Abstract vector spaces | Chapter 16, Essence of linear algebra
Summary
TLDRВ этом видео скрипте автор освещает фундаментальные вопросы теории векторов, начиная с их описания как стрелочков на плоскости и пары действительных чисел, до их более глубокого понимания как объектов в пространстве. Рассматриваются функции как альтернативные типы векторов, демонстрируя, что они подчиняются тем же операциям сложения и умножения на скаляр, что и классические векторы. Автор также обсуждает линейные преобразования и свойства линейности, подводя итог к понятию векторного пространства и его аксиоматическому определению, что позволяет применять теорию линейной алгебры к различным типам векторов, включая функции и другие абстрактные объекты.
Takeaways
- 🔍 Векторы могут быть представлены в различных формах, таких как стрелки на плоскости, пары вещественных чисел или как функции.
- 📏 Определение векторов как списков вещественных чисел дает четкий и недвусмысленный образ, что упрощает работу с многомерными векторами.
- 🌐 Векторы, в сущности, могут быть рассмотрены как существующие независимо от координатных систем, координаты являются условными и зависят от выбранных базисных векторов.
- 📊 Основные понятия линейной алгебры, такие как определители и собственные векторы, не зависят от выбора координатной системы.
- 📚 Функции также могут рассматриваться как тип векторов, где можно выполнять операции сложения и умножения на скаляр.
- 🔄 Преобразования линейные, такие как производная, являются примером оператора, который преобразует функции в соответствии с определенными свойствами.
- 📐 Определение линейности включает в себя свойства аддитивности и масштабирования, которые позволяют преобразованием сохранять структуру операций с векторами.
- 📈 Применение линейных преобразований к функциям, таким как производная, подтверждается тем, что они соответствуют определению линейности.
- 📝 Векторы могут быть представлены как функции с бесконечным числом координат, что позволяет использовать матричные операции для их преобразования.
- 📚 Векторы в современной линейной алгебре определяются не конкретной формой, а аксиомами, которые описывают правила для сложения и умножения на скаляр.
- 🌟 Основываясь на аксиомах, математические концепты и методы линейной алгебры могут быть применены к различным типам векторных пространств, включая не только пространственные векторы, но и функции.
Q & A
Что такое векторы в математике?
-Векторы в математике - это объекты, которые могут быть представлены как стрелки в пространстве или списки чисел, и которые подчиняются определенным правилам сложения и умножения на скаляр.
Почему векторы могут быть представлены как стрелки на плоскости?
-Векторы могут быть представлены как стрелки на плоскости для удобства описания их направления и размера, что позволяет визуализировать их геометрические свойства.
Какие свойства определяют, что что-то является вектором?
-Чтобы что-то считалось вектором, оно должно удовлетворять определенным аксиомам векторного пространства, таким как законы сложения векторов и умножения на скаляр.
Почему функции могут быть рассмотрены как тип векторов?
-Функции могут быть рассмотрены как тип векторов, так как существует понятие сложения функций и умножения их на скаляр, что аналогично операциям над векторами.
Что такое линейная трансформация и как она связана с векторами?
-Линейная трансформация - это функция, которая преобразует один вектор в другой, сохраняя свойства аддитивности и скалярного умножения, что делает ее линейной.
Как определяется аддитивность линейной трансформации?
-Трансформация называется аддитивной, если приложенная к сумме двух векторов дает тот же результат, что и сумма примененных к этим векторам трансформаций.
Что такое свойство скалярного умножения в контексте линейных трансформаций?
-Свойство скалярного умножения означает, что при умножении вектора на скаляр и последующем применении линейной трансформации, результат будет тем же, что и при применении трансформации к умноженному на скаляр вектору.
Как матрица описывает линейную трансформацию векторов?
-Матрица описывает линейную трансформацию, указывая, как каждая базисная векторная компонента трансформируется, и позволяет посредством матричного умножения найти трансформированный вектор.
Чем отличается понятие векторного пространства в современной теории линейной алгебры?
-Векторное пространство в современной теории линейной алгебры определяется как множество объектов, удовлетворяющих аксиомам векторного пространства, что позволяет применять теорию к различным типам векторов, включая функции и другие абстрактные структуры.
Почему математические тексты часто используют абстрактные термины при описании линейной алгебры?
-Абстрактные термины позволяют охватить широкий спектр применений линейной алгебры, не ограничиваясь одним конкретным случаем, и обеспечивают универсальность теории.
Outlines
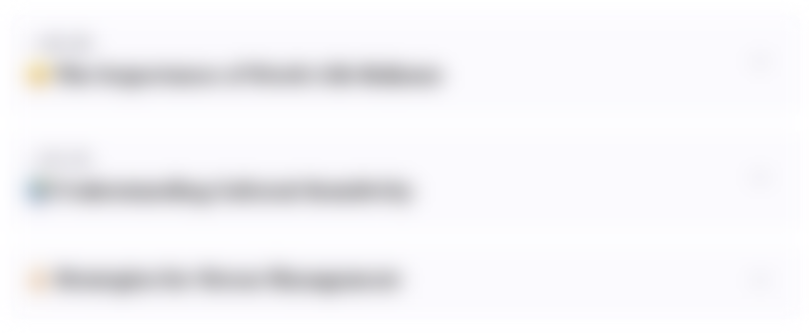
Этот раздел доступен только подписчикам платных тарифов. Пожалуйста, перейдите на платный тариф для доступа.
Перейти на платный тарифMindmap
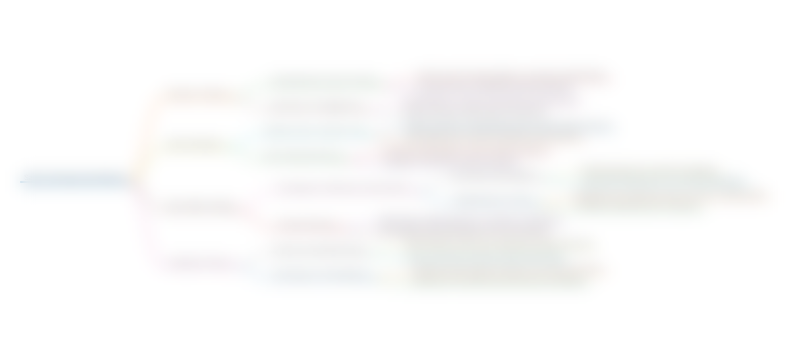
Этот раздел доступен только подписчикам платных тарифов. Пожалуйста, перейдите на платный тариф для доступа.
Перейти на платный тарифKeywords
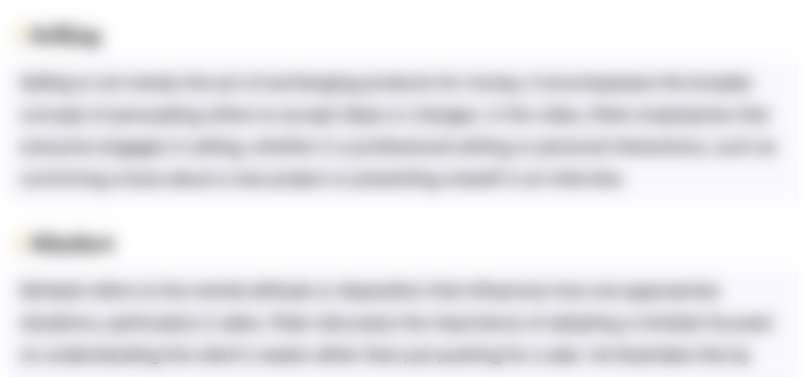
Этот раздел доступен только подписчикам платных тарифов. Пожалуйста, перейдите на платный тариф для доступа.
Перейти на платный тарифHighlights
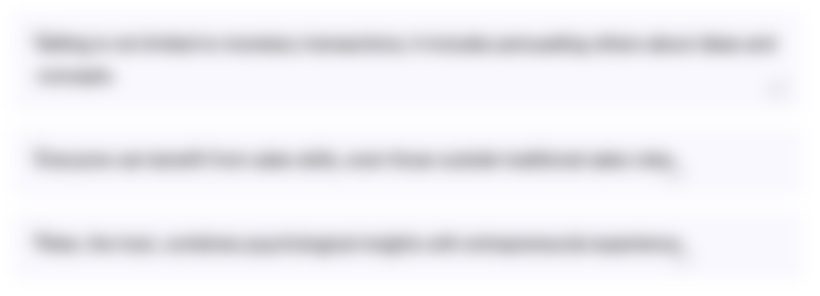
Этот раздел доступен только подписчикам платных тарифов. Пожалуйста, перейдите на платный тариф для доступа.
Перейти на платный тарифTranscripts
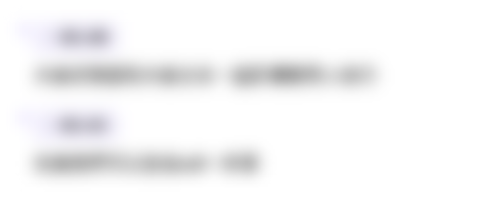
Этот раздел доступен только подписчикам платных тарифов. Пожалуйста, перейдите на платный тариф для доступа.
Перейти на платный тарифПосмотреть больше похожих видео
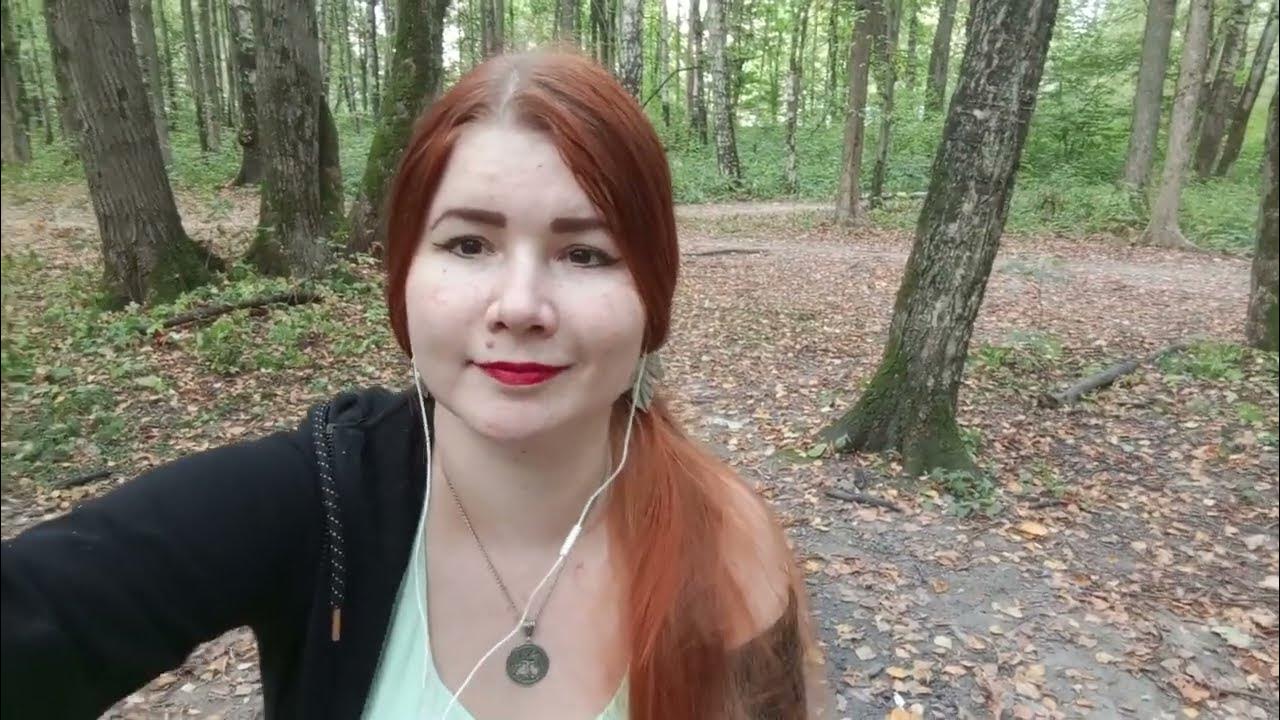
Вас просто не выбрали. Какая разница, что там за тип привязанности?
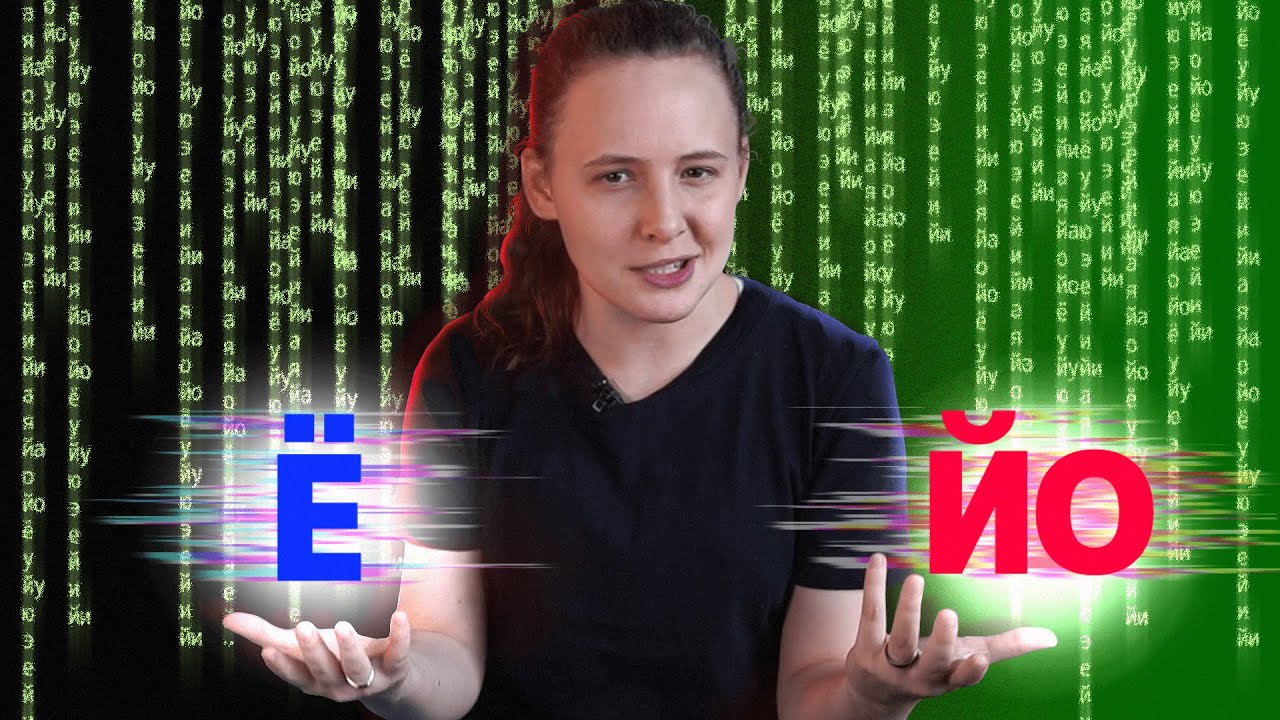
Зачем Ё, если есть ЙО?
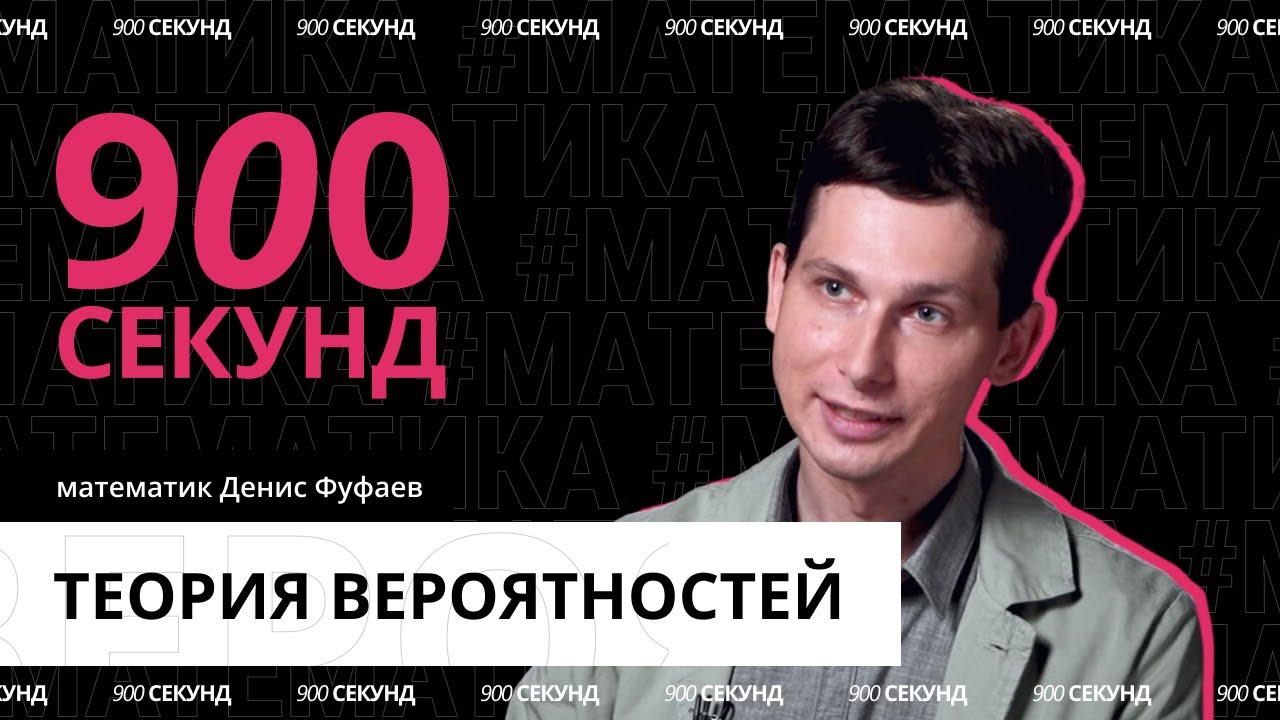
Вся суть теории вероятностей — за 900 секунд!
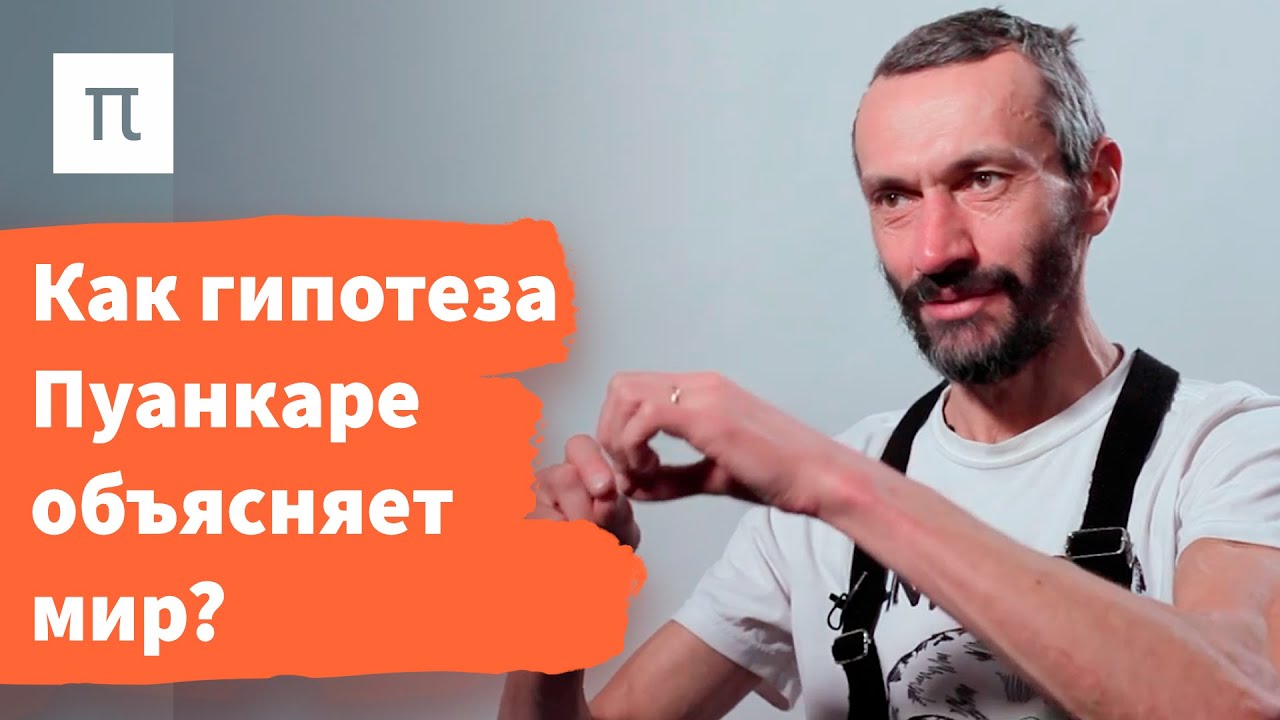
Гипотеза Пуанкаре — Алексей Савватеев на ПостНауке
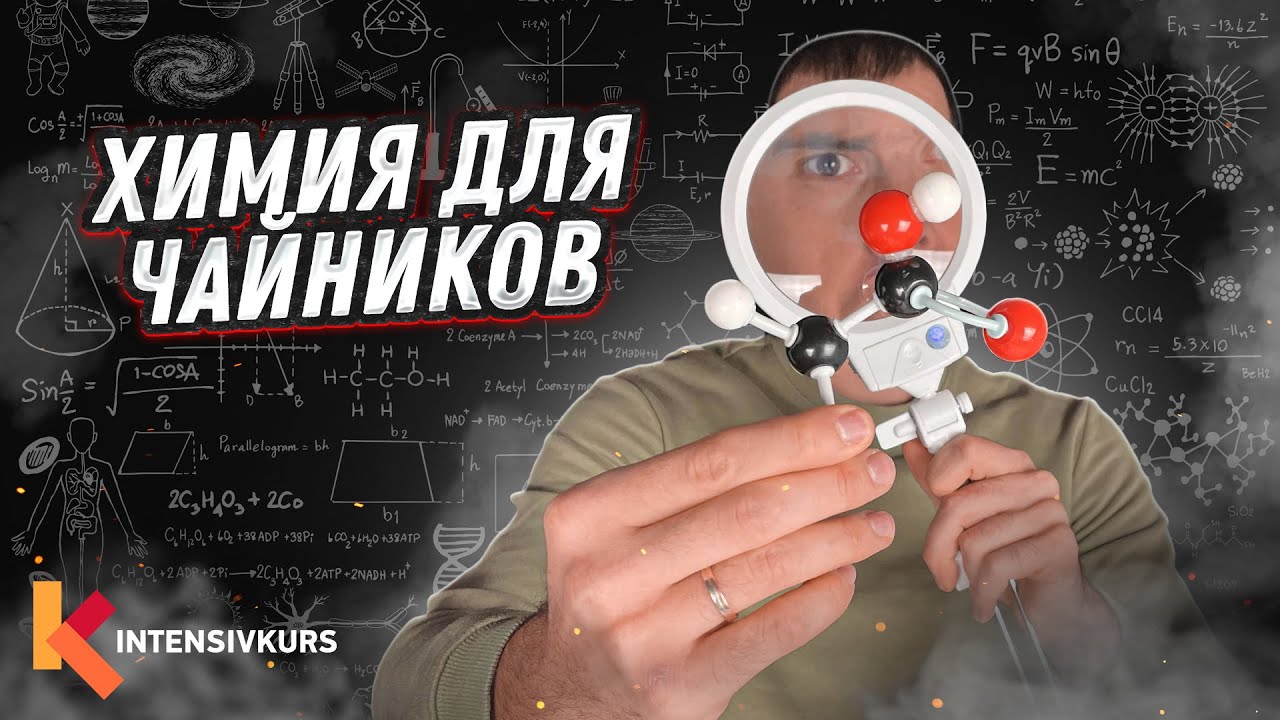
ЭТО НУЖНО ЗНАТЬ — Химия с нуля, Основные Химические Понятия
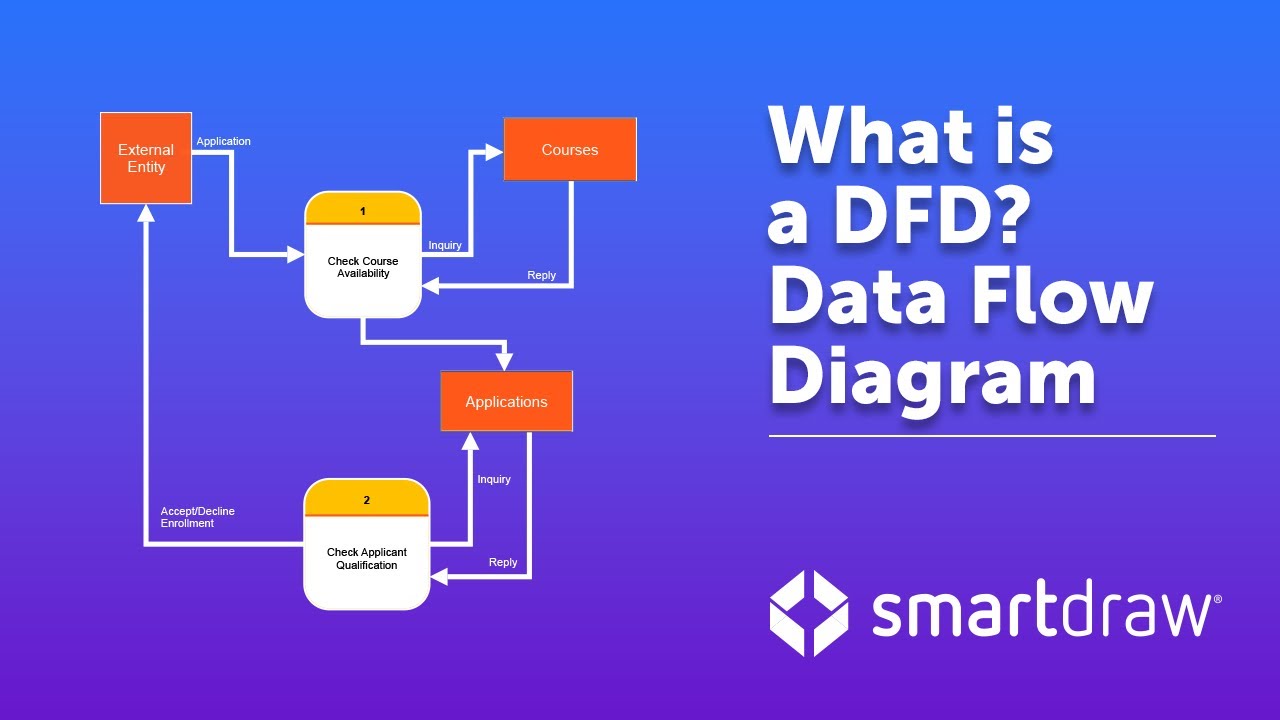
Data Flow Diagrams - What is DFD? Data Flow Diagram Symbols and More
5.0 / 5 (0 votes)