Tutorial #7 Regression + Survey weights
Summary
TLDRThis tutorial explains how to run a regression analysis using political data, focusing on the relationship between party identification and leader ratings. It demonstrates the creation of dummy variables for political parties (Liberals, Conservatives, NDP) and shows how to apply survey weights to correct for sampling biases in the data. Emphasis is placed on the importance of using weights in regression analysis, particularly for datasets with disproportionate sampling. The tutorial also touches on the limitations of weighted crosstabs and encourages students to understand and use weights in survey data analysis for more accurate results.
Takeaways
- 😀 You can use party identification data as dummy variables in a regression model to predict leader ratings (e.g., for Justin Trudeau).
- 😀 Survey data often requires the use of weights to correct for over- and under-represented groups, such as youth or Quebec residents in the Canadian Election Study (CES).
- 😀 In a regression, a constant value represents the average rating of Justin Trudeau for those who do not identify with major political parties (Liberals, Conservatives, or NDP).
- 😀 Dummy variables (e.g., for Liberals, Conservatives, and NDP) allow you to assess the impact of party identification on the evaluation of political leaders.
- 😀 The use of weights in regression can affect the model's explanatory power (R-squared), but generally results in more accurate and representative estimates.
- 😀 Applying a survey weight is important when analyzing CES data because it accounts for the disproportionate stratified random sampling technique used in the study.
- 😀 For weighted regressions, you can specify the weight by using commands like 'pweight' in Stata, which adjusts the results according to the sampling design.
- 😀 The effect of party affiliation on leader ratings is substantial; for instance, Liberal supporters rate Justin Trudeau significantly higher than non-partisans or minor-party supporters.
- 😀 Although weights can slightly alter the coefficients, they generally do not dramatically change the overall interpretation of the model's results.
- 😀 When using weighted crosstabs, it’s important to recognize that while the technique corrects for sampling issues, it complicates statistical analysis and should be approached with caution.
- 😀 Grad students working with CES data should always apply the weight in regressions to ensure accurate results, but may avoid weighted crosstabs due to their complexity and limitations in measuring association.
Q & A
What is the main focus of the tutorial in the transcript?
-The tutorial focuses on running a regression analysis using survey data, particularly to examine how party identification influences leader evaluation ratings. It also discusses the importance of incorporating survey weights to adjust for disproportionate stratified random sampling in the data.
Why is party identification transformed into dummy variables for the regression?
-Party identification is transformed into dummy variables so that it can be used in the regression analysis. This allows for a comparison between the different party affiliations (Liberals, Conservatives, NDP) and those who do not identify with the major parties.
What does the constant in the regression model represent?
-The constant in the regression model represents the average leader evaluation rating for respondents who do not identify with any of the major political parties (Liberals, Conservatives, or NDP), essentially those who belong to minor parties or do not identify with any party at all.
How does party affiliation affect the leader evaluation in the regression model?
-The regression model shows that being affiliated with a particular party significantly impacts leader evaluation ratings. For example, being a Liberal increases the rating of Justin Trudeau by about 37 points on a 0-100 scale, while being a Conservative decreases the rating by about 27 points, compared to individuals who do not identify with the major parties.
What is the purpose of using survey weights in the regression analysis?
-Survey weights are used to correct for the overrepresentation or underrepresentation of certain groups in the survey sample. This ensures that the regression results are more reflective of the actual population, especially when the survey data is drawn from a disproportionate stratified random sample.
What is the main difference between unweighted and weighted regression results?
-The main difference is that weighted regression adjusts for biases in the sampling design, typically leading to a slight decrease in the R-squared value and small shifts in the coefficients. For instance, while the effect of being a Liberal on leader evaluation stays roughly the same, the effect of the NDP may change slightly when weights are applied.
Why do the results from crosstabs with survey weights not include chi-square or association measures?
-When survey weights are applied to crosstabs, traditional measures like chi-square or Kramer’s V cannot be calculated because weighted crosstabs do not allow for these statistical measures. This is why weighted regressions are generally preferred over crosstabs when working with survey weights.
What are the challenges with using crosstabs when applying survey weights?
-Crosstabs become less reliable and harder to interpret when survey weights are applied. While they can still be used to display counts and percentages, they don't provide as robust statistical measures of association as regressions do, particularly when dealing with weighted survey data.
Why is it important to use survey weights in the Canadian Election Study (CES)?
-The CES uses a disproportionate stratified random sampling method, oversampling certain groups like Quebec residents and youth to ensure sufficient representation. Applying survey weights corrects for these sampling biases, ensuring that the results are representative of the overall population.
What should you do if you're unsure whether to apply weights in your survey analysis?
-Always check the documentation of the dataset you're using. If the data comes from a source like Statistics Canada, weights may not be necessary, but if you're using data from studies like the CES, applying weights is typically required. It's always a good practice to confirm with the dataset's guidelines.
Outlines
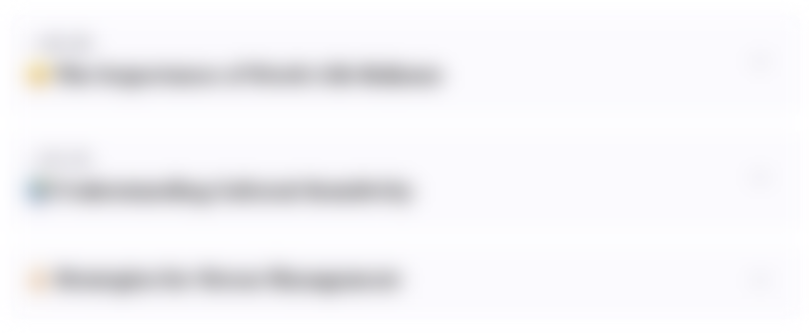
Этот раздел доступен только подписчикам платных тарифов. Пожалуйста, перейдите на платный тариф для доступа.
Перейти на платный тарифMindmap
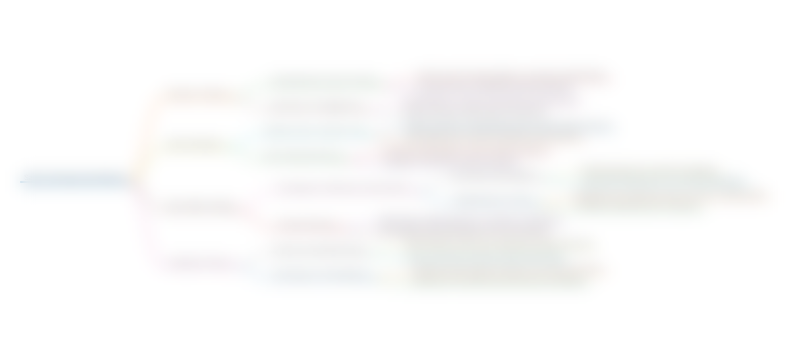
Этот раздел доступен только подписчикам платных тарифов. Пожалуйста, перейдите на платный тариф для доступа.
Перейти на платный тарифKeywords
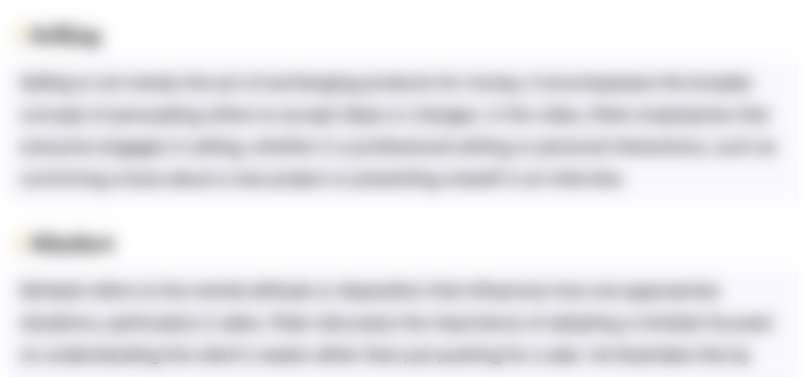
Этот раздел доступен только подписчикам платных тарифов. Пожалуйста, перейдите на платный тариф для доступа.
Перейти на платный тарифHighlights
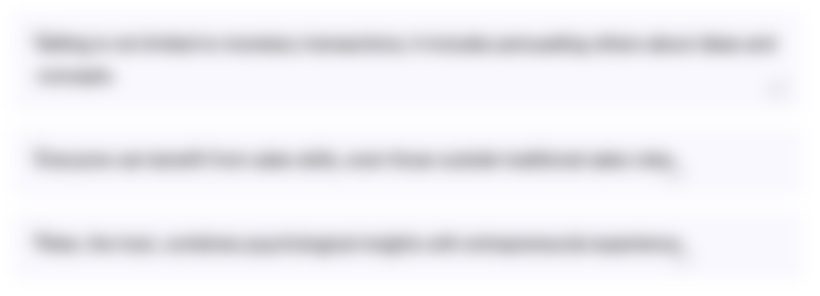
Этот раздел доступен только подписчикам платных тарифов. Пожалуйста, перейдите на платный тариф для доступа.
Перейти на платный тарифTranscripts
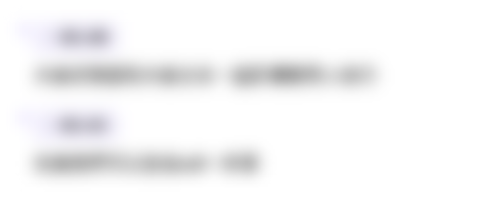
Этот раздел доступен только подписчикам платных тарифов. Пожалуйста, перейдите на платный тариф для доступа.
Перейти на платный тарифПосмотреть больше похожих видео

Excel Regression Analysis through the Toolpak
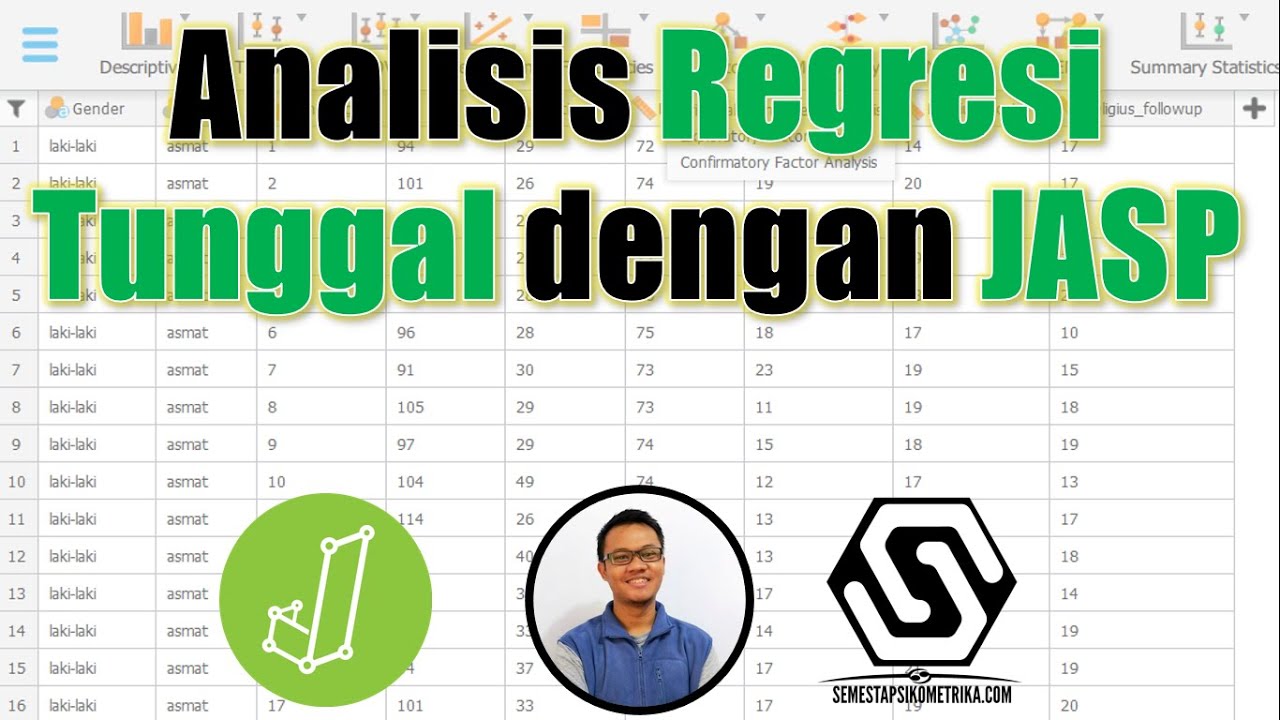
Tutorial Analisis Regresi Tunggal dengan JASP
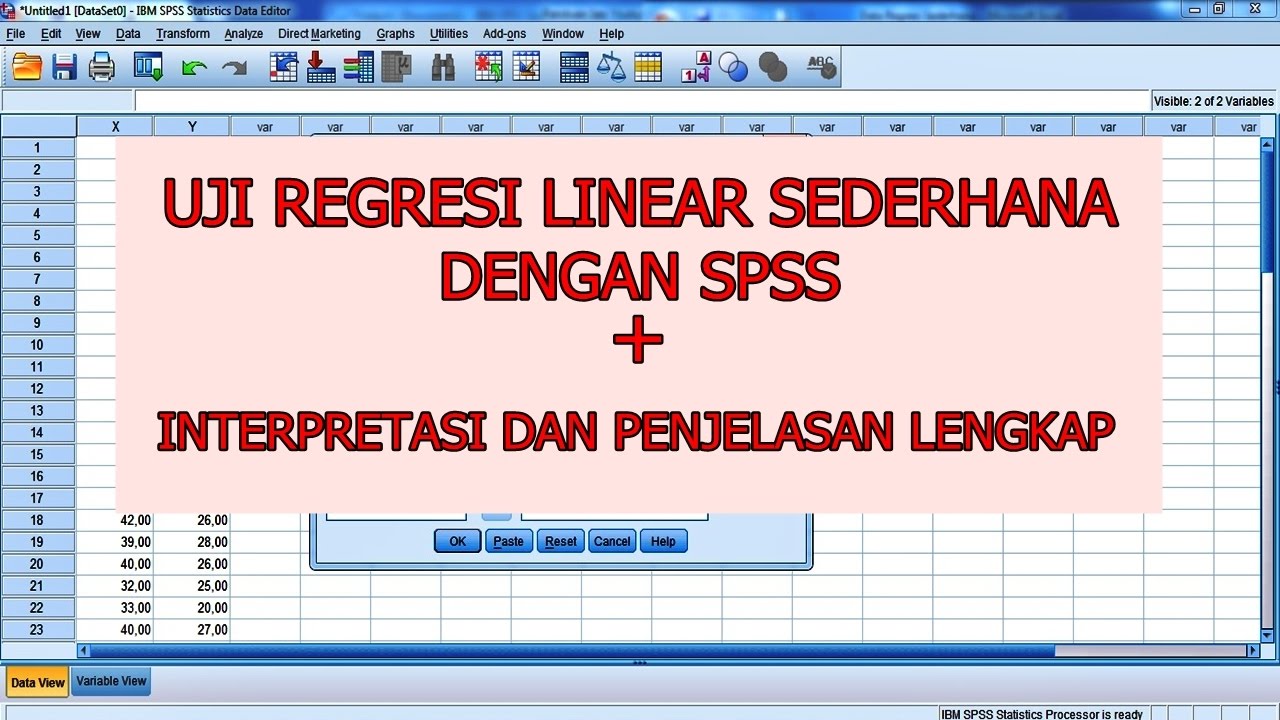
Uji Regresi Linear Sederhana dengan SPSS Sangat Detail
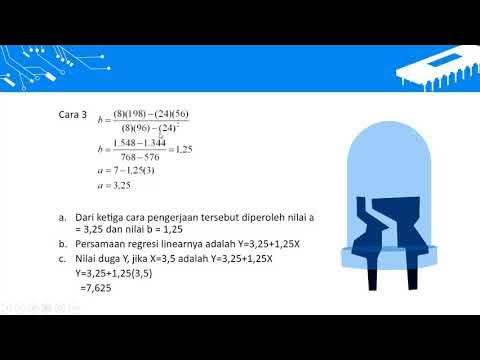
METODE NUMERIK 11 REGRESI LINIER
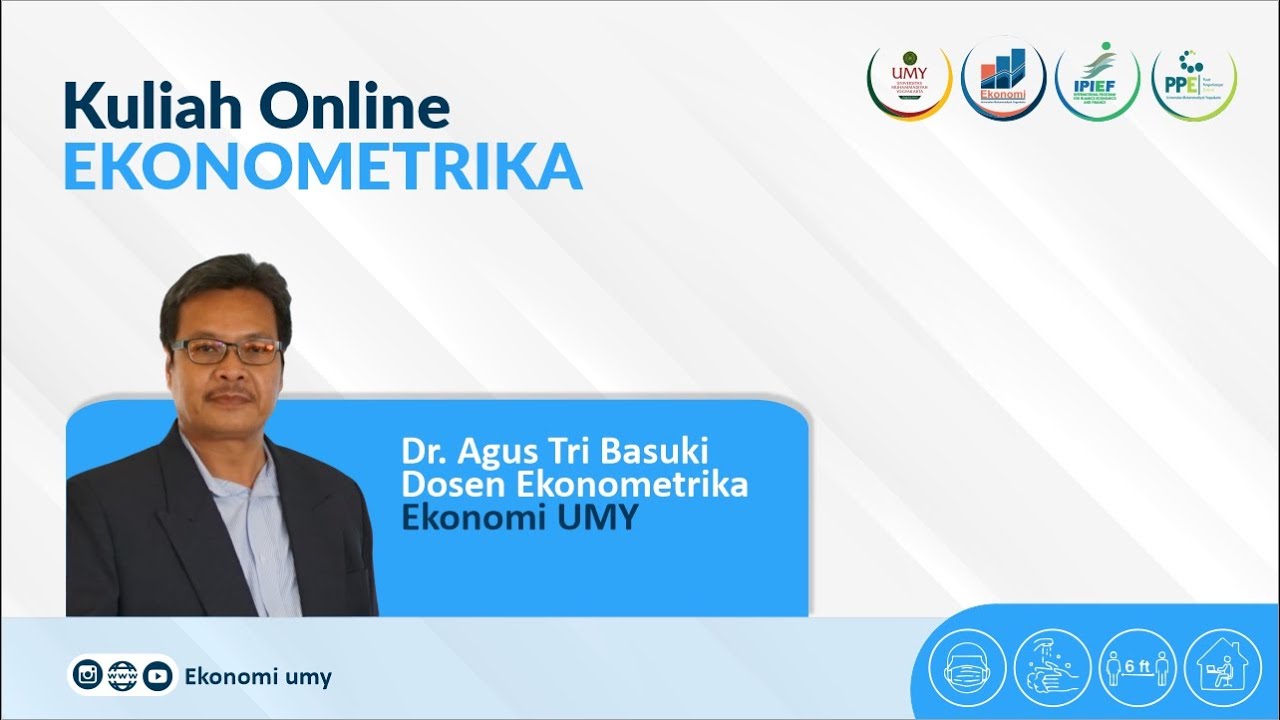
REGRESI DENGAN DUMMY VARIABEL LEBIH DARI 2 KATEGORI Oleh Agus Tri Basuki Part 2
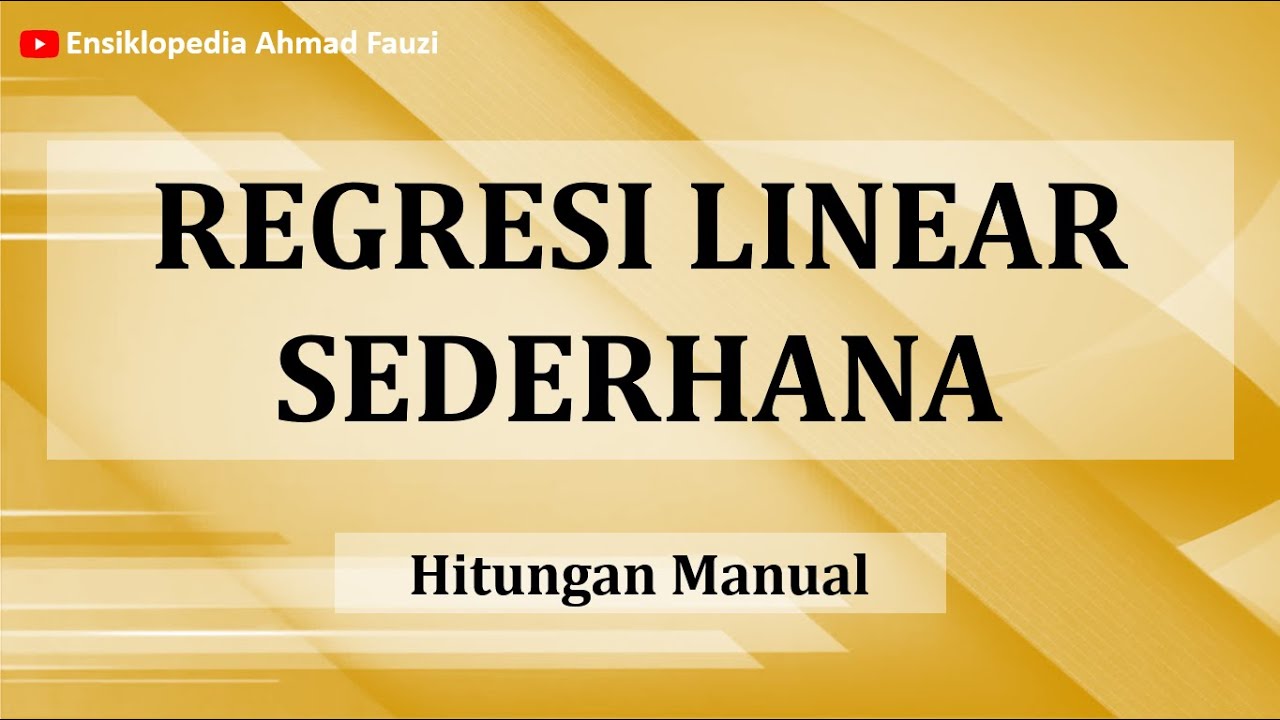
Cara Menghitung Analisis Regresi Sederhana secara Manual
5.0 / 5 (0 votes)