Statistical inference for spatial autocorrelation: Moran’s I & LISA
Summary
TLDRIn this video, the instructor explores spatial autocorrelation, focusing on Moran's I test, a key statistical tool for analyzing spatial relationships. The test measures correlation between a location's value and the average of its neighboring values. A positive Moran's I suggests clusters of similar values, while negative autocorrelation indicates outliers. The instructor also discusses Local Indicator of Spatial Association (LISA) to identify clusters of high and low values in specific areas. Monte Carlo simulations are used to assess statistical significance, providing a robust method for testing hypotheses in spatial data analysis.
Takeaways
- 😀 Moran's I test is the main method for assessing spatial autocorrelation, measuring correlation between a location's value and the average value of its neighbors.
- 😀 The Moran's I test result provides a statistic (I value) and a p-value to determine if there is significant spatial autocorrelation or if the null hypothesis (no autocorrelation) holds.
- 😀 If Moran's I value is close to zero, there is no spatial autocorrelation. A positive value suggests positive autocorrelation (similar values clustering together), and a negative value indicates negative autocorrelation (dissimilar values clustering together).
- 😀 The null hypothesis of Moran's I is that there is no spatial autocorrelation (I = 0), while the alternative hypothesis is that there is autocorrelation (I ≠ 0).
- 😀 A p-value associated with Moran's I helps assess the significance of the autocorrelation—whether the observed pattern is due to random chance or not.
- 😀 Positive autocorrelation indicates high values near high values or low values near low values, which can help identify spatial patterns like disease spread or areas with similar characteristics.
- 😀 Local Indicator of Spatial Autocorrelation (LISA) is used to identify specific locations where high values cluster with high values, and low values with low values, providing a more granular view of spatial relationships.
- 😀 LISA can identify local outliers where high values are surrounded by low values or vice versa, even in areas with a global positive autocorrelation trend.
- 😀 The significance of Moran's I is assessed using Monte Carlo simulations, where the observed data is randomly scrambled multiple times to generate a distribution of values under the null hypothesis.
- 😀 Monte Carlo simulations allow the calculation of a p-value based on how the real data's Moran's I statistic compares to the distribution of random, scrambled data, helping to confirm the statistical significance of autocorrelation.
Q & A
What is the primary focus of the video?
-The video focuses on explaining spatial autocorrelation, specifically through the use of Moran's I and Local Moran's I (LISA) tests, as tools for geographical data analysis.
What is Moran's I test used for in spatial analysis?
-Moran's I is used to measure spatial autocorrelation by comparing the value of a variable at a given location with the average values of its neighboring locations. A positive Moran’s I indicates positive autocorrelation (clusters of similar values), while a negative value suggests negative autocorrelation (high values next to low values).
How do you interpret the p-value in Moran’s I test?
-The p-value in Moran’s I test helps determine the statistical significance of the result. A low p-value (close to zero) suggests that the observed spatial autocorrelation is statistically significant, indicating that the data is not random but follows a spatial pattern.
What is the role of LISA (Local Moran’s I) in spatial autocorrelation analysis?
-LISA is used to identify localized clusters of high or low values within a study area. It provides more detailed insight by detecting local outliers where high values are surrounded by low values or vice versa, even when the global trend suggests positive autocorrelation.
How does LISA differ from Moran's I in spatial analysis?
-While Moran's I provides a global measure of spatial autocorrelation, LISA performs a local test to highlight specific areas with high or low values. LISA can uncover local clusters or outliers that Moran’s I might miss.
What does a positive Moran’s I value indicate?
-A positive Moran’s I value indicates positive spatial autocorrelation, meaning that similar values (either high or low) are grouped together in the study area, creating clusters of similar values.
What does a negative Moran’s I value imply?
-A negative Moran’s I value implies negative spatial autocorrelation, where high values are surrounded by low values, or vice versa, indicating that dissimilar values are grouped together.
Why is it important to assess local spatial patterns using LISA?
-LISA is important because it identifies specific locations or areas with significant spatial patterns, such as clusters of high values or low values. This can guide decision-making, such as prioritizing areas for intervention in public health or other fields.
How are Monte Carlo simulations used to assess the significance of Moran’s I and LISA?
-Monte Carlo simulations assess the significance of Moran’s I and LISA by repeatedly scrambling the data, creating random permutations, and calculating the Moran’s I statistic for each permutation. The actual observed statistic is then compared to this distribution to derive the p-value and determine if the spatial autocorrelation is statistically significant.
Can Moran’s I and LISA detect local outliers even when there is a global positive autocorrelation?
-Yes, even when there is an overall positive spatial autocorrelation, Moran’s I and LISA can detect local outliers, such as areas where high values are surrounded by low values, or vice versa.
Outlines
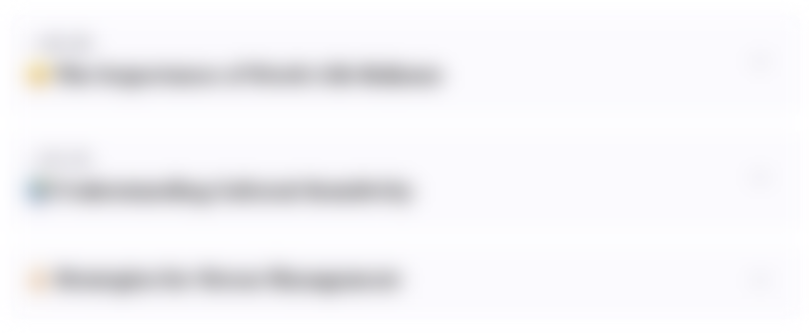
Этот раздел доступен только подписчикам платных тарифов. Пожалуйста, перейдите на платный тариф для доступа.
Перейти на платный тарифMindmap
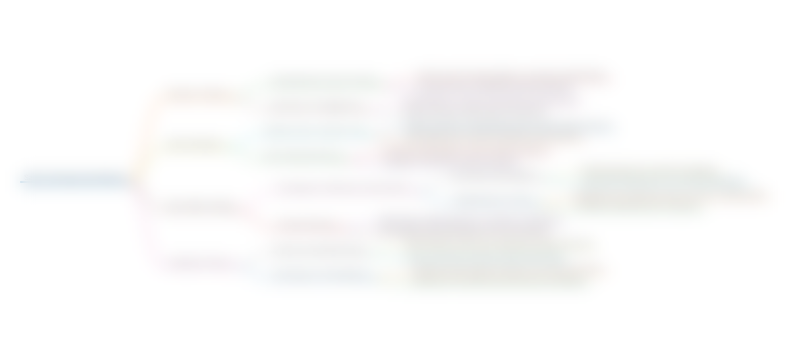
Этот раздел доступен только подписчикам платных тарифов. Пожалуйста, перейдите на платный тариф для доступа.
Перейти на платный тарифKeywords
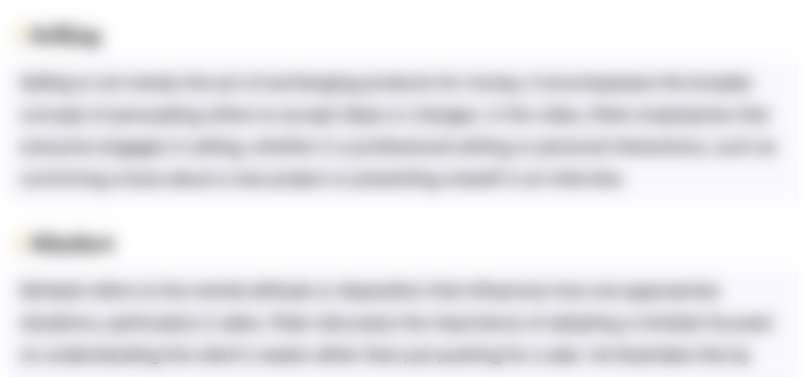
Этот раздел доступен только подписчикам платных тарифов. Пожалуйста, перейдите на платный тариф для доступа.
Перейти на платный тарифHighlights
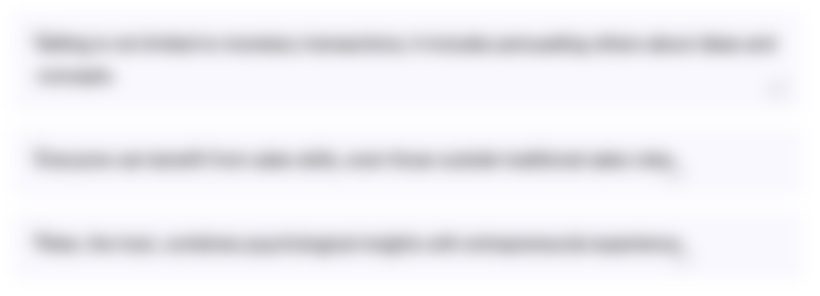
Этот раздел доступен только подписчикам платных тарифов. Пожалуйста, перейдите на платный тариф для доступа.
Перейти на платный тарифTranscripts
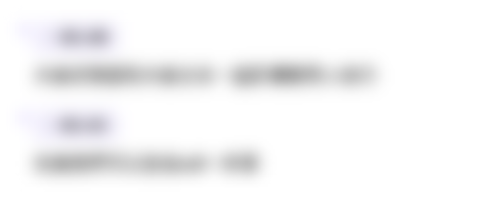
Этот раздел доступен только подписчикам платных тарифов. Пожалуйста, перейдите на платный тариф для доступа.
Перейти на платный тариф5.0 / 5 (0 votes)