Llama 3.2 Deep Dive - Tiny LM & NEW VLM Unleashed By Meta
Summary
TLDRIn the latest Meta Connect, Meta announced the release of LLaMA 3.2, featuring a diverse range of models, including lightweight text-only models and groundbreaking vision-language models. The 1B and 3B models excel in long-context tasks and summarization, making them ideal for on-device applications. However, while the vision capabilities of the 11B and 90B models show promise, they do not outperform competitors like Quin2 VL and NVM in benchmarks. The video also discusses the advantages of local deployment for privacy and performance, alongside Nvidia's optimizations for efficient use across various hardware. Overall, LLaMA 3.2 represents a significant advancement in multimodal AI technology.
Takeaways
- 😀 Meta announced LLaMA 3.2, introducing a variety of new language models, including lightweight text-only and multimodal versions.
- 📊 The 1B and 3B text-only models are derived from the 3.28B model, utilizing synthesized data from a larger 45B model.
- 💡 The 1B model excels in long context retrieval and summarization tasks, outperforming larger models like the 5.3B Mini Instruct in specific benchmarks.
- ⚖️ The 3B model offers improved capabilities but faces significant competition, making it less distinctive than the 1B model.
- 📈 Both text-only models support a remarkable 128k context window, a feature uncommon for models of their size.
- 💾 The 1B model is designed for efficiency, requiring only 2.5GB of VRAM for full precision, suitable for mobile device deployment.
- 🖼️ The 11B and 90B models are Meta's first multimodal models, integrating vision capabilities while maintaining the 128k context window.
- 🔍 Benchmarks show LLaMA 3.2's vision models may not lead the market but provide valuable advantages for local usage and privacy.
- 🔗 Nvidia is collaborating with Meta to optimize LLaMA 3.2 for local deployment, ensuring efficiency across various devices.
- 🔄 Future improvements are anticipated in multimodal models, particularly in refining cross-attention mechanisms for handling multiple modalities.
Q & A
What are the key new models introduced in LLaMA 3.2?
-LLaMA 3.2 introduces four models: two lightweight text-only models (1B and 3B) and two multimodal models with vision capabilities (11B and 90B).
How do the 1B and 3B models differ in terms of architecture?
-The 1B model has 1.24 billion parameters and the 3B model has 3.24 billion parameters. Both are pruned from LLaMA 3.28B and utilize synthesized data, but the 3B model has improved capabilities and is fine-tuned for tool use.
What is unique about the context window size of the 1B and 3B models?
-Both the 1B and 3B models feature an unprecedented 128k context window, which allows them to process much longer sequences of text compared to other models of similar size.
In which tasks does the 1B model excel compared to the 5.3B models?
-The 1B model outperforms the 5.3B models in benchmarks focused on long context information retrieval, summarization, and question answering.
What are the memory requirements for running the LLaMA 3.2 models?
-The 1B model requires 2.5 GB of VRAM for FP16 and 1.3 GB for 8-bit integer quantization, while the 3B model requires 6.4 GB for FP16 and 3.4 GB for AP quantization.
What are the parameter counts for the multimodal models?
-The LLaMA 3.2 multimodal models have parameter counts of 10.6 billion for the 11B model and 88.6 billion for the 90B model.
How does LLaMA 3.2 compare to other existing vision language models?
-In benchmark comparisons, LLaMA 3.2 generally underperformed against other models like Quin2 VL and NVM, particularly in vision capabilities, though it maintains strong performance in text tasks.
What training architecture is used in LLaMA 3.2 for multimodal models?
-LLaMA 3.2 uses a vision transformer called ViT H14 and freezes the text transformer during training to preserve text generation quality while integrating vision capabilities.
What advantages does running models locally offer?
-Running models locally provides better privacy since sensitive data, such as emails and messages, are processed without being sent to external servers, reducing the risk of data leaks.
What optimizations have been made by NVIDIA for LLaMA 3.2?
-NVIDIA has optimized LLaMA 3.2 for efficient performance on their RTX GPUs and developed a multimodal RAG pipeline, allowing for better indexing and querying of information based on visual input.
Outlines
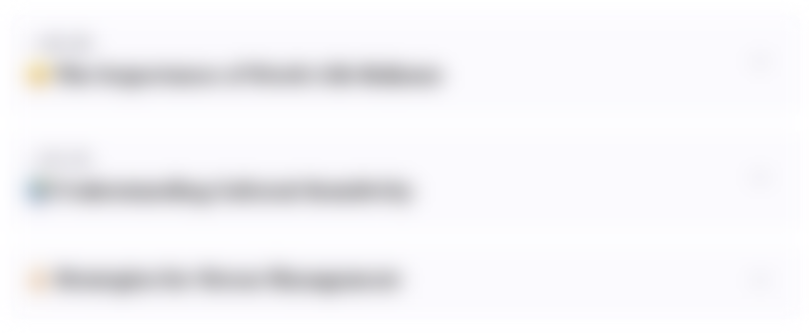
Этот раздел доступен только подписчикам платных тарифов. Пожалуйста, перейдите на платный тариф для доступа.
Перейти на платный тарифMindmap
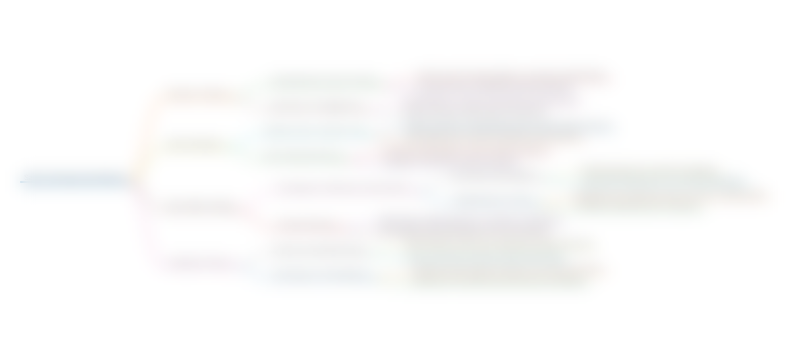
Этот раздел доступен только подписчикам платных тарифов. Пожалуйста, перейдите на платный тариф для доступа.
Перейти на платный тарифKeywords
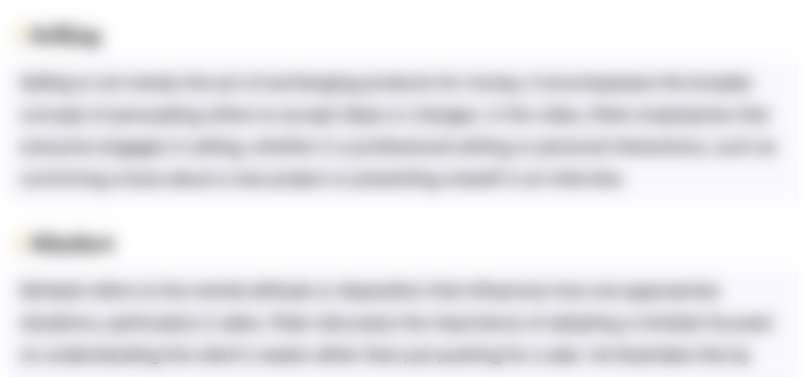
Этот раздел доступен только подписчикам платных тарифов. Пожалуйста, перейдите на платный тариф для доступа.
Перейти на платный тарифHighlights
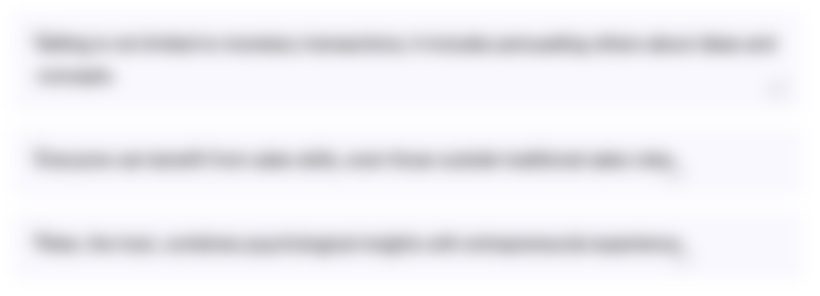
Этот раздел доступен только подписчикам платных тарифов. Пожалуйста, перейдите на платный тариф для доступа.
Перейти на платный тарифTranscripts
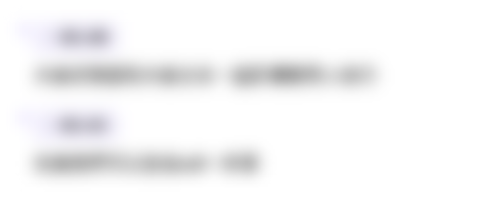
Этот раздел доступен только подписчикам платных тарифов. Пожалуйста, перейдите на платный тариф для доступа.
Перейти на платный тарифПосмотреть больше похожих видео

🚨BREAKING: LLaMA 3 Is HERE and SMASHES Benchmarks (Open-Source)
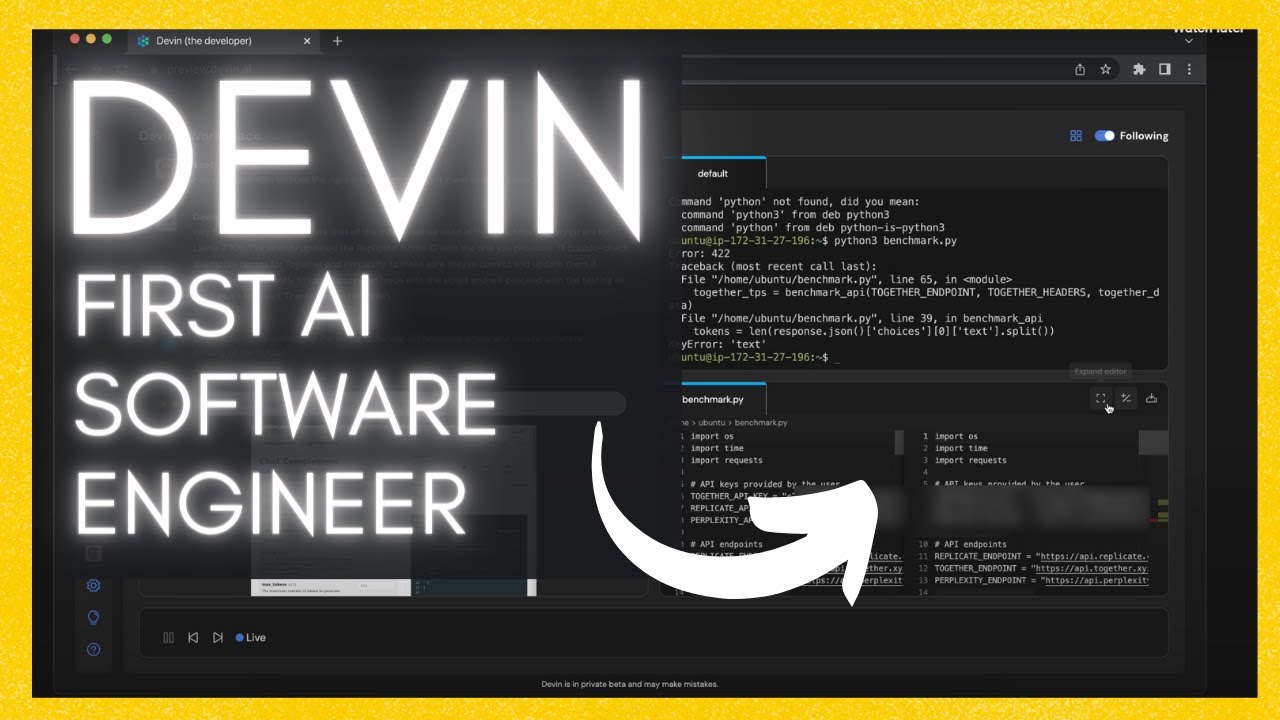
Devin: The First AI Software Engineer - Builds & Deploy Apps End-to-End!
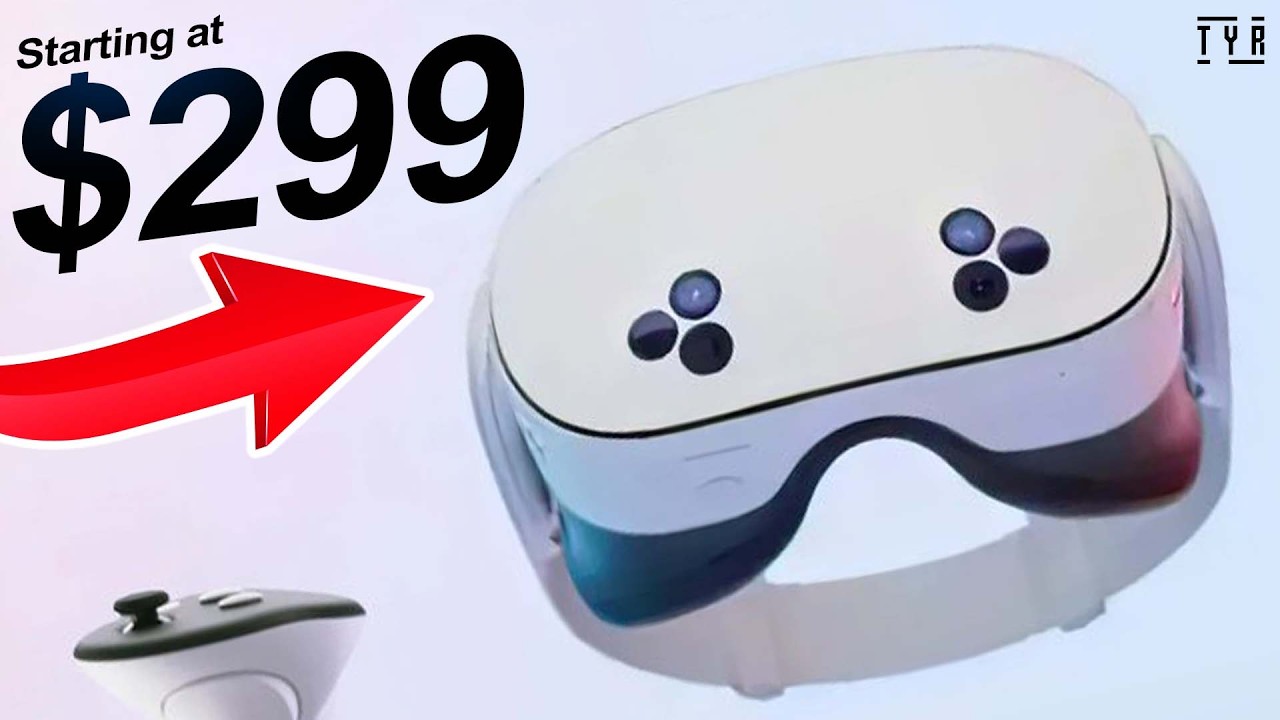
EVERYTHING About the Meta QUEST 3S

"40만원짜리?" 요새 폼 미친 메타의 홀로그램과 라마AI! / 메타 커넥트 2024리뷰
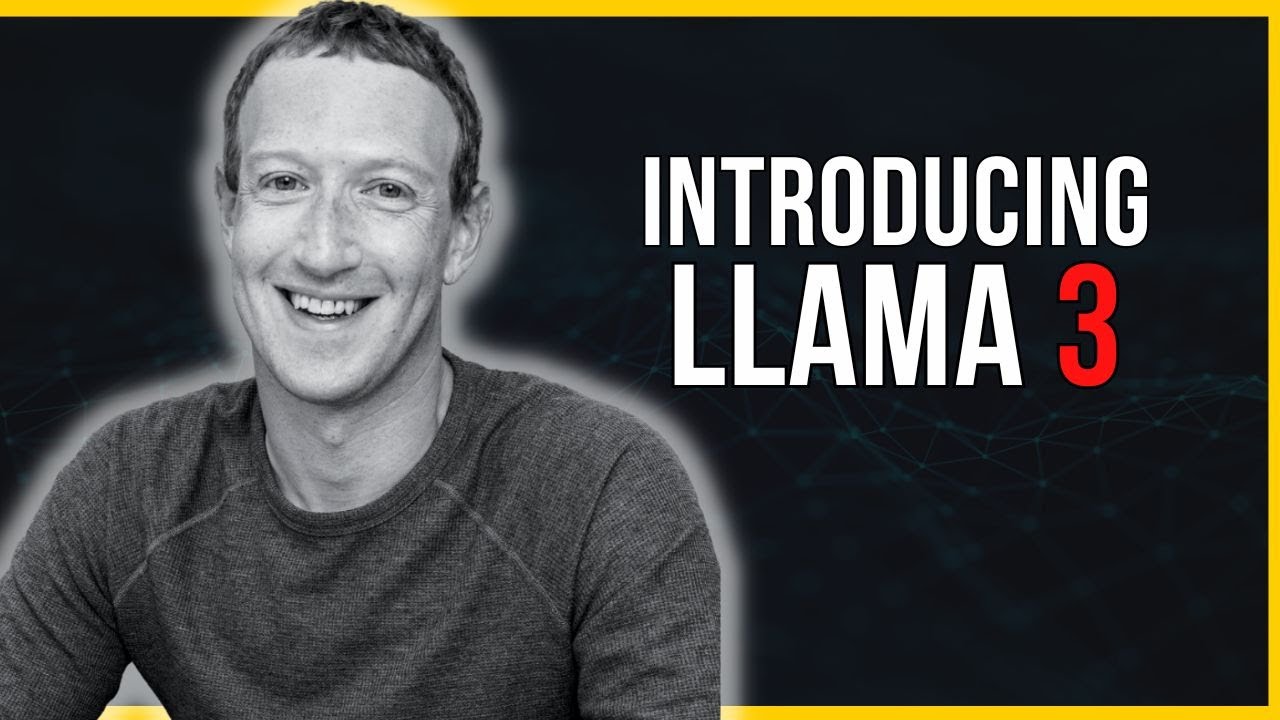
LLAMA 3 Released - All You Need to Know
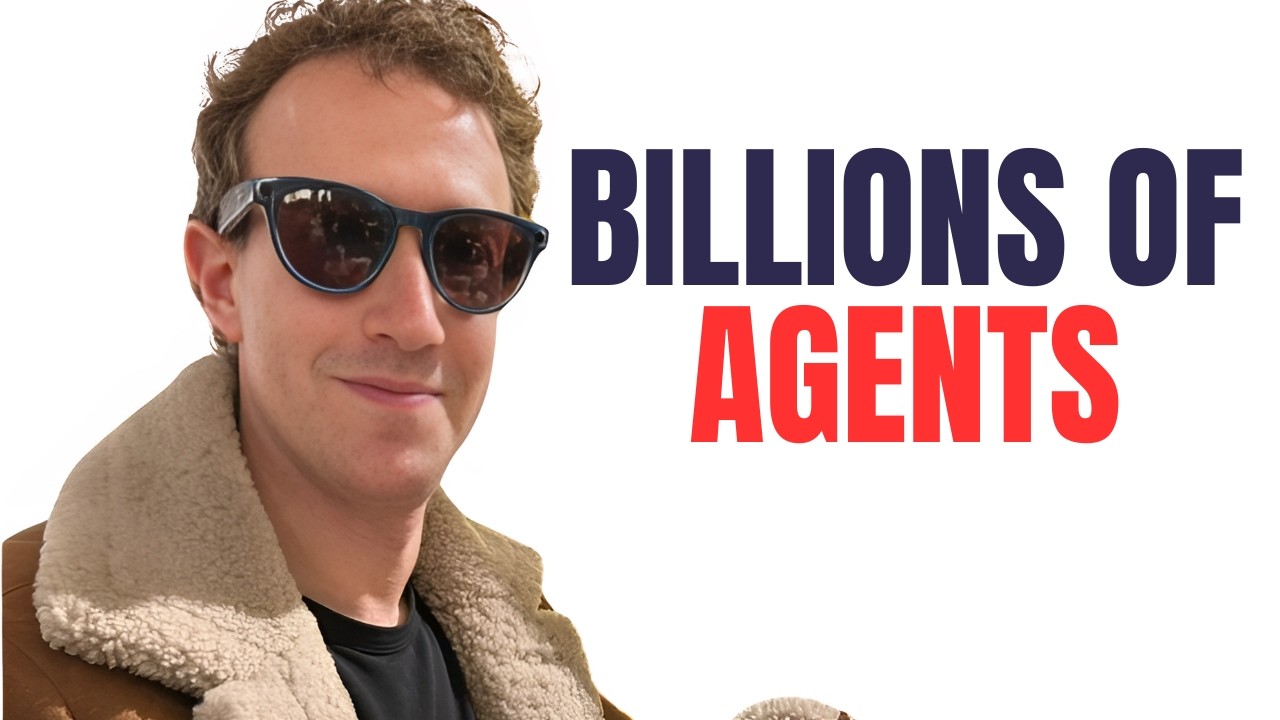
Zuckerberg's "Scorched Earth" LLaMA AI Strategy (Interview Breakdown)
5.0 / 5 (0 votes)