How to get better at video games, according to babies - Brian Christian
Summary
TLDRIn 2013, DeepMind researchers set out to create an AI capable of mastering all Atari games. They developed Deep Q Networks (DQN), which achieved superhuman scores in most games but struggled with 'Montezuma’s Revenge' due to its complexity. To overcome this, the team incorporated a novelty-based reward system inspired by human behavior, particularly how babies are drawn to new stimuli. This change helped DQN explore the game effectively. The research highlights how AI and human intelligence inform each other, revealing insights into curiosity, creativity, and motivation.
Takeaways
- 🎯 In 2013, DeepMind researchers aimed to create an AI system that could beat every Atari game.
- 🕹️ They developed a system called Deep Q Networks (DQN), which became superhuman in less than two years.
- 🏆 DQN outperformed professional human testers, achieving scores 13 to 25 times better in games like 'Breakout,' 'Boxing,' and 'Video Pinball.'
- 🚨 However, DQN struggled with 'Montezuma’s Revenge,' unable to score a single point despite weeks of gameplay.
- 🔄 DQN used reinforcement learning, where it tried to maximize in-game points without modeling the game environment explicitly.
- 🤔 The challenge with 'Montezuma’s Revenge' was that scoring required complex, non-random sequences of actions, making trial-and-error button-mashing ineffective.
- 👶 Researchers discovered that AI, like babies, needed a sense of novelty to explore and learn effectively.
- 🧠 They enhanced DQN with novelty-based rewards, where unusual on-screen images were as valuable as in-game points.
- 🔑 With this novelty-seeking behavior, DQN explored and advanced in 'Montezuma’s Revenge,' overcoming its previous limitations.
- ⚠️ However, the novelty-based approach also had challenges, such as diminishing motivation over time or getting overwhelmed by constant new stimuli.
Q & A
What is Deep Q Networks (DQN) and why was it significant?
-Deep Q Networks (DQN) is an AI system developed by DeepMind to play and master multiple Atari games using reinforcement learning. It was significant because it achieved superhuman performance in games like 'Breakout' and 'Boxing,' demonstrating the potential of AI in solving complex tasks without a pre-defined model of the game environment.
How did DQN perform in most Atari games, and what was an exception?
-DQN performed exceptionally well in most Atari games, achieving scores far beyond human testers. For instance, it performed 13 times better at 'Breakout' and 17 times better at 'Boxing.' However, it struggled with 'Montezuma's Revenge,' where it couldn't score a single point, highlighting a limitation in its approach.
Why was 'Montezuma’s Revenge' particularly challenging for DQN?
-'Montezuma’s Revenge' was challenging because it required a precise sequence of actions to score points. DQN's random button-mashing approach made it difficult to navigate the game and reach reward states, as even small mistakes resulted in game failure.
How does reinforcement learning work in the context of playing Atari games?
-Reinforcement learning in Atari games involves the system maximizing a numerical reward (game points) by learning which actions to take. The AI tries different button presses and observes the consequences, gradually optimizing its actions to maximize its score.
What is the difference between model-based and model-free approaches in AI systems?
-Model-based approaches involve having an internal model of the environment, which allows the system to predict the outcome of its actions before executing them. Model-free approaches, like DQN, do not model the environment but instead learn from trial and error based on the feedback from their actions.
How did DQN learn which buttons to press in games?
-DQN learned which buttons to press through trial and error. It started by pressing buttons randomly and gradually learned which sequences of actions led to higher rewards (points) by predicting the future rewards associated with different button presses.
What role did novelty play in helping DQN succeed in 'Montezuma's Revenge'?
-Novelty played a crucial role in DQN's success when researchers added a preference for exploring new or unusual screen images as a reward. This motivated DQN to explore the game environment not just for points but out of curiosity, which led it to perform better in 'Montezuma's Revenge.'
How did the novelty-based reward system change DQN’s behavior?
-The novelty-based reward system encouraged DQN to explore the game environment more thoroughly. Instead of focusing solely on earning points, it sought out new rooms and keys in 'Montezuma’s Revenge,' which allowed it to progress further than it had before.
What are some potential downsides to using a novelty-based reward system in AI?
-One downside is that if the AI has explored all possible novelties in the environment, it may lose motivation and stop exploring. Additionally, if it encounters continuously novel stimuli, like a television screen, it can become paralyzed, unable to focus on a goal due to constant distractions.
How has the intersection of AI and human intelligence research benefited both fields?
-The intersection has been mutually beneficial. AI researchers have borrowed concepts like novelty-seeking behavior from studies of human intelligence to solve practical problems in AI. Meanwhile, insights from AI, such as how systems get stuck or unstuck, are helping researchers understand human phenomena like curiosity, creativity, and even mental health challenges like boredom and addiction.
Outlines
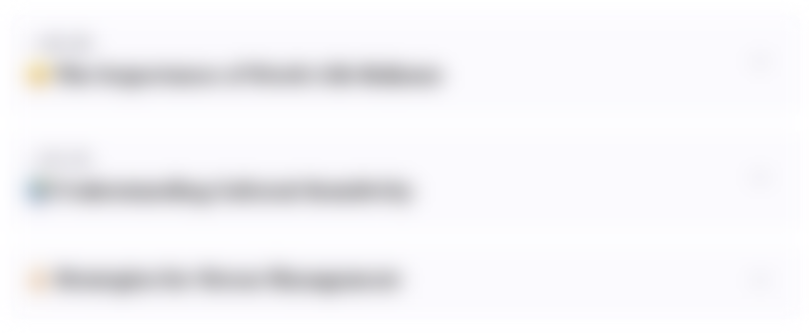
Этот раздел доступен только подписчикам платных тарифов. Пожалуйста, перейдите на платный тариф для доступа.
Перейти на платный тарифMindmap
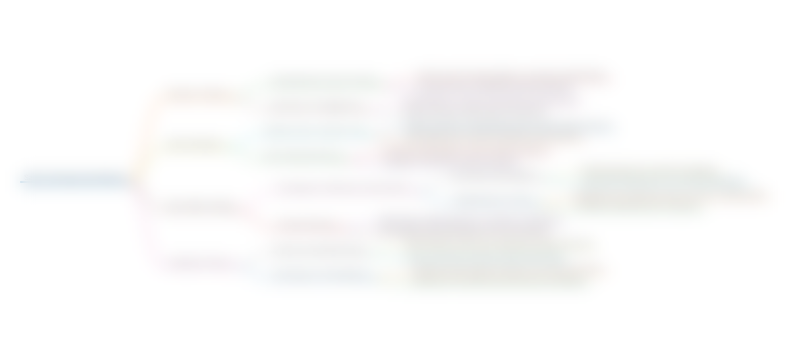
Этот раздел доступен только подписчикам платных тарифов. Пожалуйста, перейдите на платный тариф для доступа.
Перейти на платный тарифKeywords
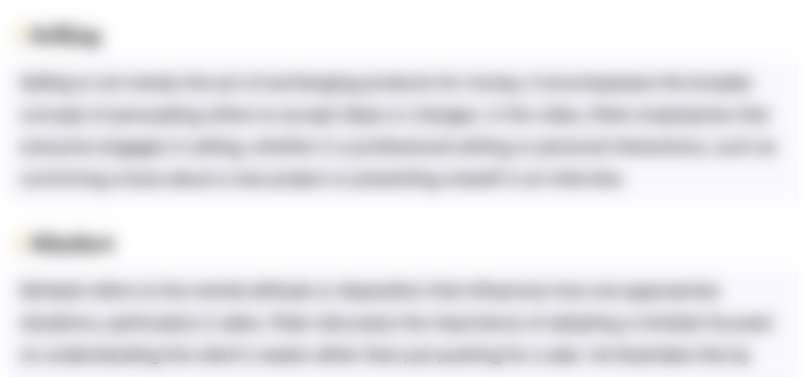
Этот раздел доступен только подписчикам платных тарифов. Пожалуйста, перейдите на платный тариф для доступа.
Перейти на платный тарифHighlights
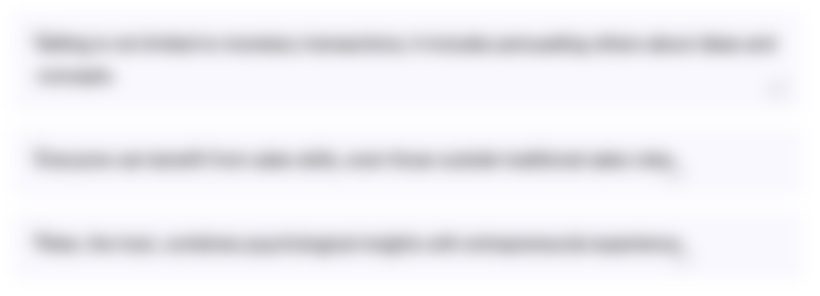
Этот раздел доступен только подписчикам платных тарифов. Пожалуйста, перейдите на платный тариф для доступа.
Перейти на платный тарифTranscripts
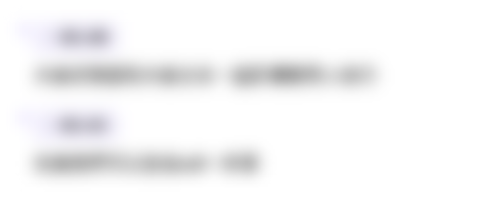
Этот раздел доступен только подписчикам платных тарифов. Пожалуйста, перейдите на платный тариф для доступа.
Перейти на платный тарифПосмотреть больше похожих видео
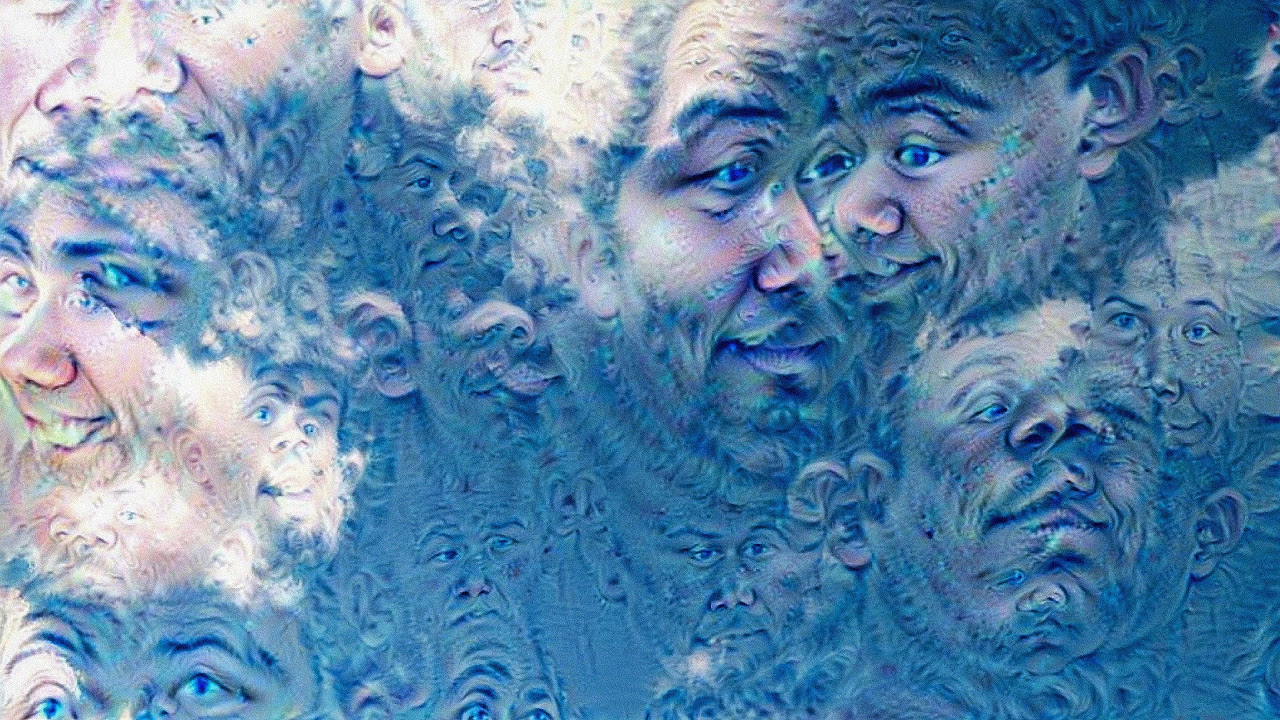
How computers are learning to be creative | Blaise Agüera y Arcas
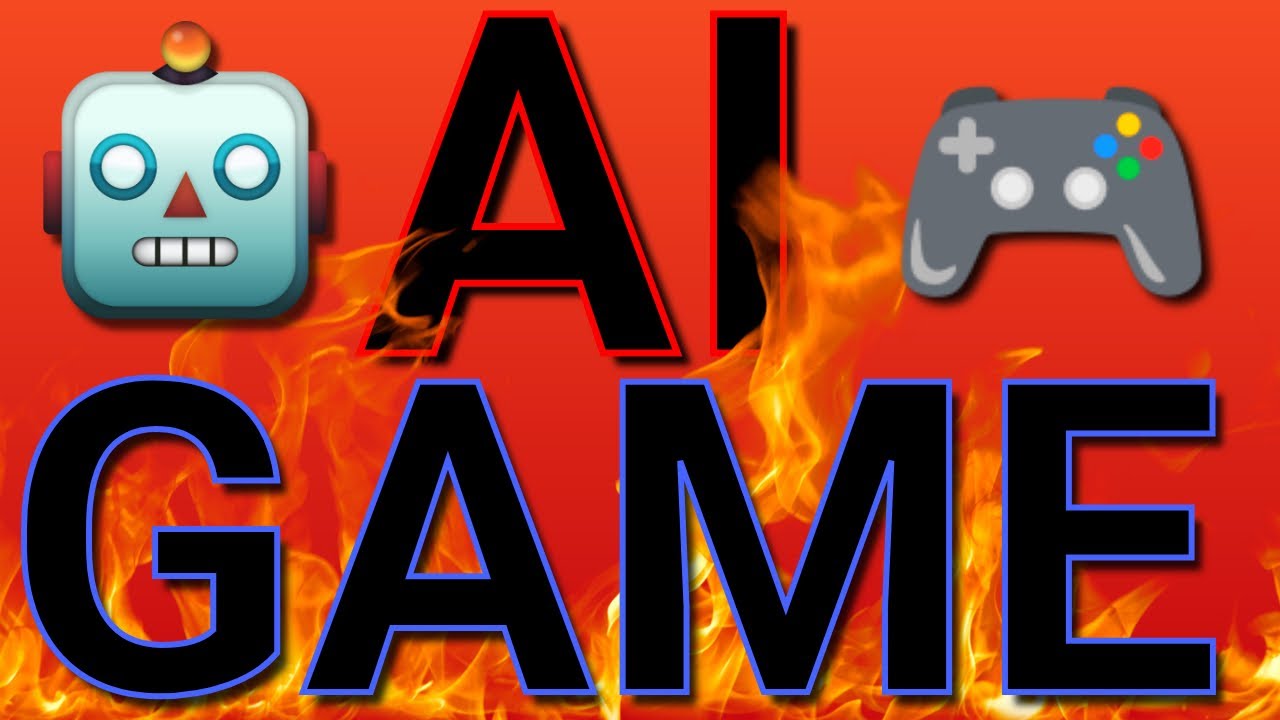
I Created a New Game Using AI (No Coding Experience)
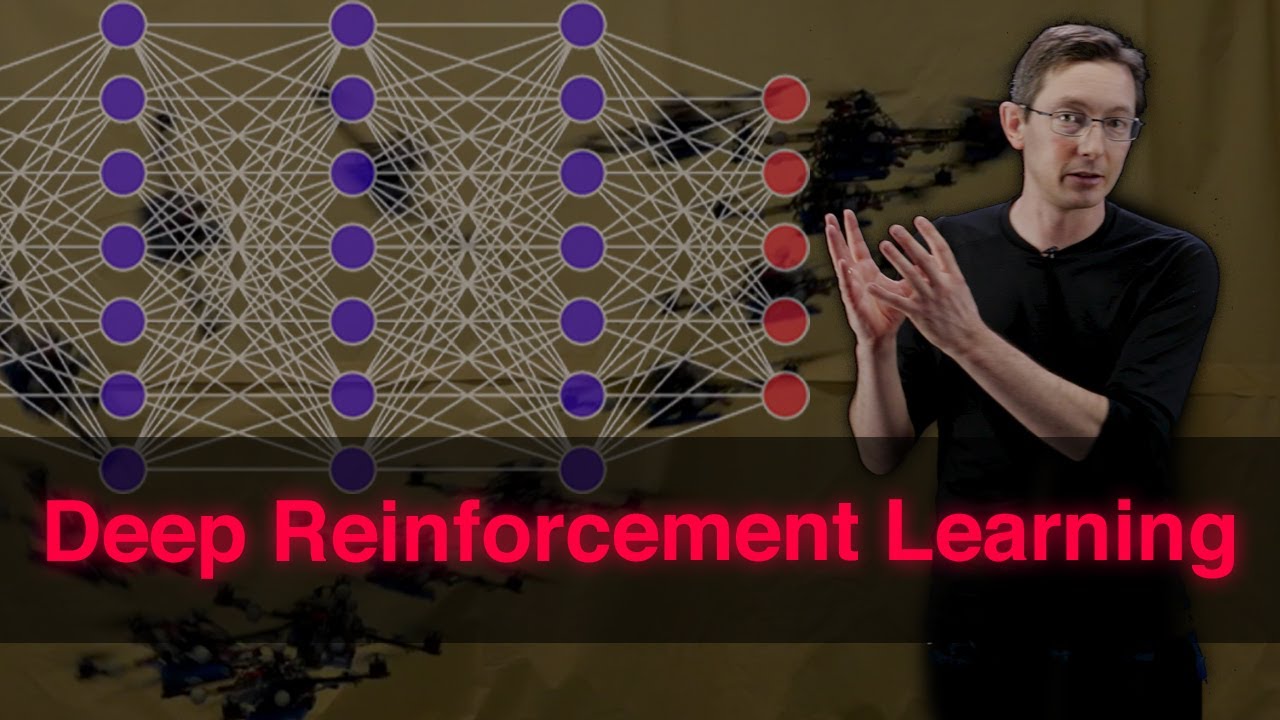
Deep Reinforcement Learning: Neural Networks for Learning Control Laws

How AI could help us talk to animals
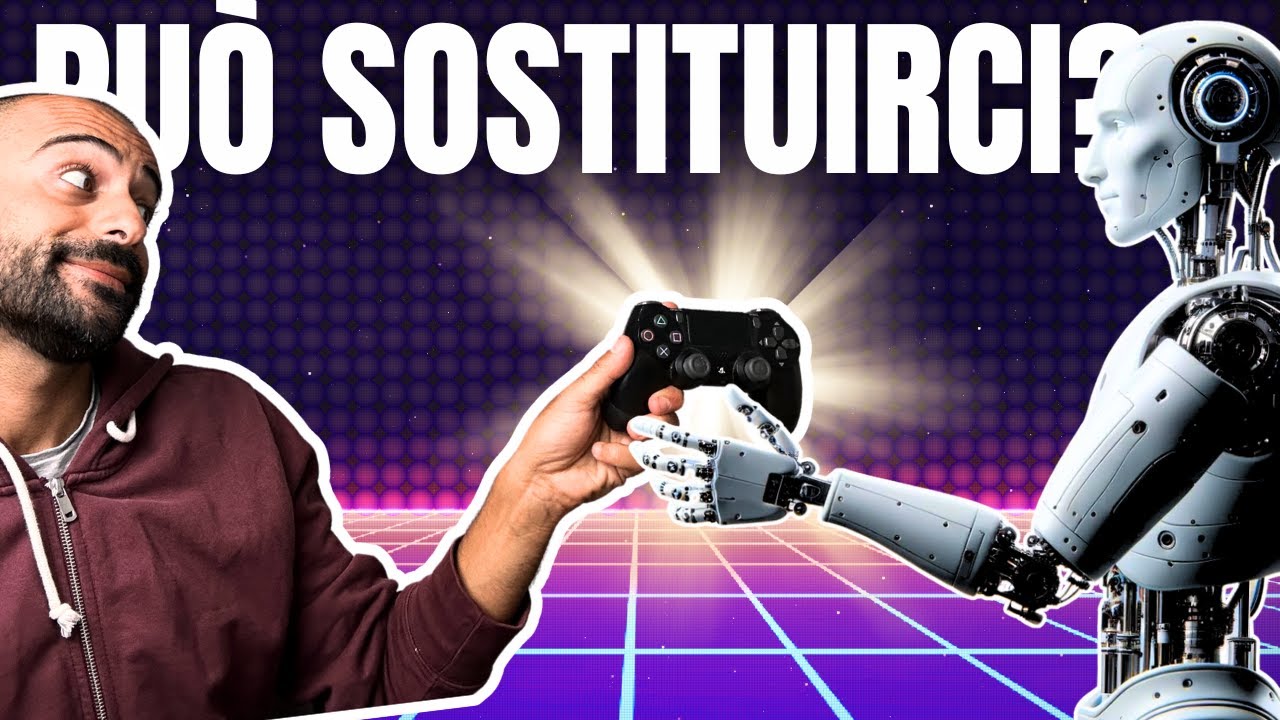
L' AI che GIOCA ai VIDEOGAME potrà LAVORARE al NOSTRO POSTO? (reaction Google SIMA)
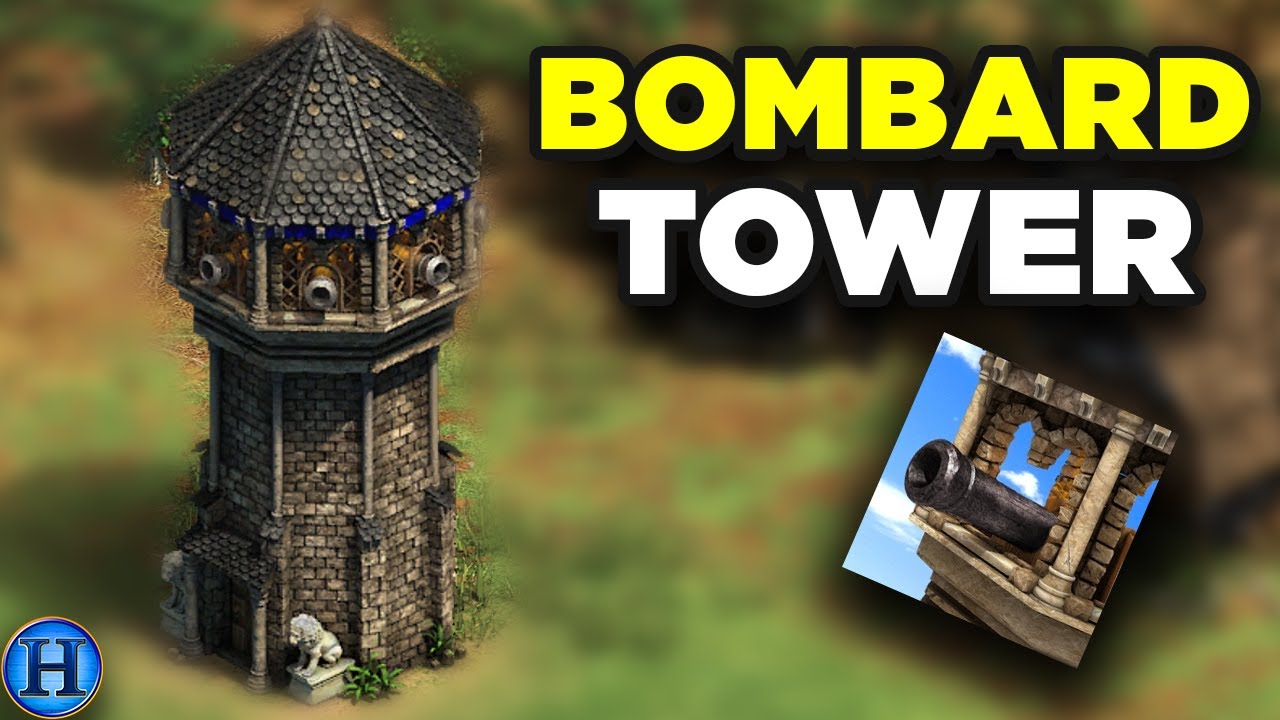
Lets Talk About Bombard Towers | AoE2
5.0 / 5 (0 votes)