What is Genetic Algorithm? | Matlab Code of Genetic Algorithm
Summary
TLDRThis video introduces the concept of genetic algorithms, a popular optimization method inspired by natural selection from Charles Darwin's theory of evolution. Genetic algorithms involve evolving a population of solutions through generations, using crossover and mutation to create offspring and selecting the fittest individuals. Over time, the algorithm converges on an optimal or near-optimal solution. The video also offers MATLAB code for implementing genetic algorithms and provides links for more advanced techniques. The channel promises regular updates on solving optimization problems.
Takeaways
- 📚 Genetic algorithms are meta-heuristic, stochastic optimization algorithms inspired by Charles Darwin's theory of natural evolution.
- 🧬 The algorithm was introduced by Holland in 1975 and is widely used in research communities.
- 🧪 Genetic algorithms begin with a random set of solutions called the population, where each solution is a chromosome.
- 🔄 Chromosomes evolve over generations, being evaluated for fitness at each stage.
- 👥 New chromosomes (offspring) are created by either crossover (combining two chromosomes) or mutation (modifying one chromosome).
- 📊 Selection is based on fitness, where fitter chromosomes have a higher chance of being carried forward into the next generation.
- 🎯 The algorithm aims to converge to the best chromosome, which represents the optimal or near-optimal solution to the problem.
- 📝 The script discusses the traditional genetic algorithm but mentions more advanced versions with MATLAB and Python implementations.
- 🔗 Viewers are directed to download the MATLAB code and are encouraged to check out more advanced topics on the creator's YouTube channel or blog.
- 👍 The script promotes subscribing to the channel for weekly videos on solving optimization problems.
Q & A
What is a genetic algorithm?
-A genetic algorithm is a meta-heuristic stochastic optimization algorithm inspired by the natural selection process described by Charles Darwin. It is used to find optimal or near-optimal solutions to optimization problems.
Who introduced the genetic algorithm and when?
-The genetic algorithm was introduced by John Holland in 1975.
What is the initial set of random solutions in a genetic algorithm called?
-The initial set of random solutions in a genetic algorithm is called a population.
What is the term for each individual in the population of a genetic algorithm?
-Each individual in the population is called a chromosome, which represents a potential solution to the problem.
How do chromosomes evolve in a genetic algorithm?
-Chromosomes evolve through successive iterations called generations, where they are evaluated and modified using crossover and mutation operators.
What is the purpose of the fitness measure in a genetic algorithm?
-The fitness measure is used to evaluate the chromosomes during each generation to determine their quality as solutions to the problem.
How are offspring created in a genetic algorithm?
-Offspring are created by either merging two chromosomes from the current generation using a crossover operator or by modifying a chromosome using a mutation operator.
How is the new generation formed in a genetic algorithm?
-The new generation is formed by selecting some of the parents and offspring based on their fitness values and rejecting others to maintain a constant population size.
What happens to the fitter chromosomes in the selection process?
-Fitter chromosomes have a higher probability of being selected for the next generation, as they are more likely to represent better solutions.
What is the expected outcome after several generations in a genetic algorithm?
-After several generations, the algorithm converges to the best chromosome, which is hoped to represent the optimum or sub-optimal solution to the problem.
Where can one find more advanced versions of genetic algorithms with MATLAB and Python codes?
-More advanced and robust versions of genetic algorithms with MATLAB and Python codes can be found on the presenter's YouTube channel and blog at learnwithpanda.com.
What is the presenter's advice for those interested in optimization problems?
-The presenter suggests subscribing to their YouTube channel for more videos on solving optimization problems every week.
Outlines
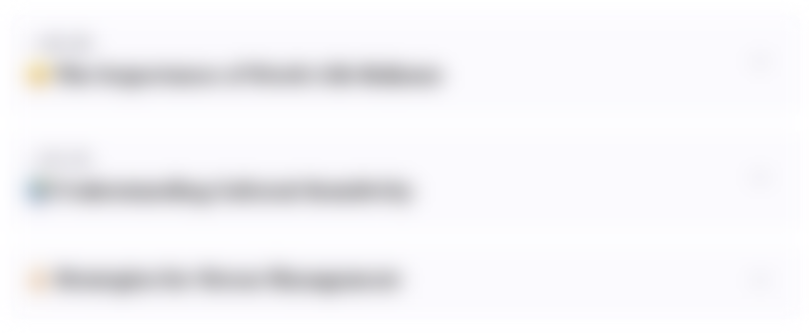
Этот раздел доступен только подписчикам платных тарифов. Пожалуйста, перейдите на платный тариф для доступа.
Перейти на платный тарифMindmap
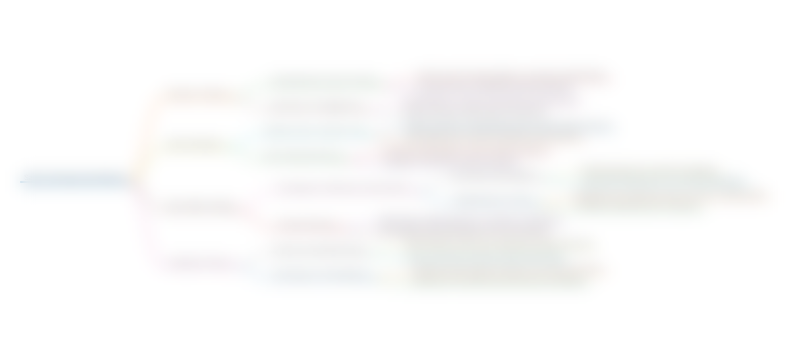
Этот раздел доступен только подписчикам платных тарифов. Пожалуйста, перейдите на платный тариф для доступа.
Перейти на платный тарифKeywords
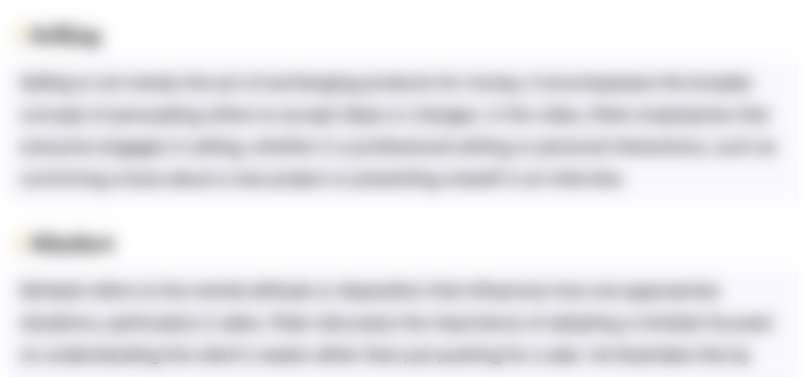
Этот раздел доступен только подписчикам платных тарифов. Пожалуйста, перейдите на платный тариф для доступа.
Перейти на платный тарифHighlights
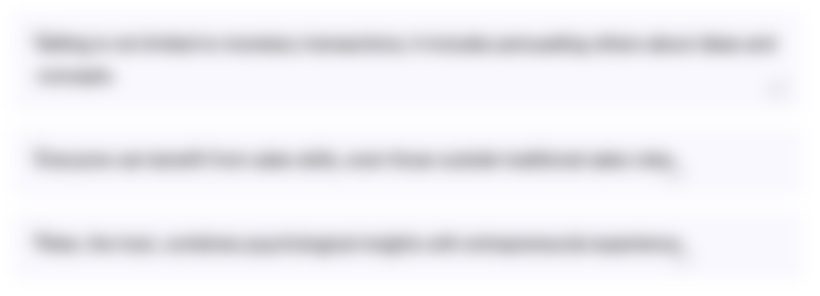
Этот раздел доступен только подписчикам платных тарифов. Пожалуйста, перейдите на платный тариф для доступа.
Перейти на платный тарифTranscripts
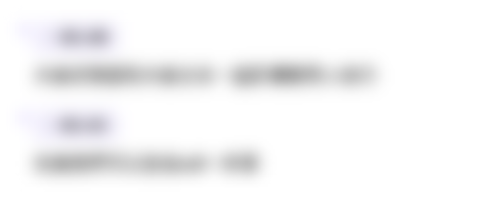
Этот раздел доступен только подписчикам платных тарифов. Пожалуйста, перейдите на платный тариф для доступа.
Перейти на платный тарифПосмотреть больше похожих видео
5.0 / 5 (0 votes)