Multiple Regression, Clearly Explained!!!
Summary
TLDRIn this StatQuest episode, host Josh Starmer explains multiple regression, emphasizing it's not much different from simple linear regression. He reviews key concepts like fitting a plane to data, calculating R-squared, and adjusting for additional parameters. The episode also covers calculating p-values and F-values, comparing simple and multiple regression to determine the value of collecting more data, like tail length in mice. A companion video teaches how to perform multiple regression in R, detailing important aspects of the output.
Takeaways
- 📚 StatQuest is an educational series focused on statistics, hosted by Josh Starmer.
- 🔍 The episode discusses multiple regression, building on the concepts introduced in the linear regression episode.
- 📈 Simple regression involves fitting a line to data, while multiple regression involves fitting a plane or higher-dimensional object.
- 📊 R-squared is used to evaluate the fit of the model to the data, and its calculation remains the same for both simple and multiple regression.
- ⚖️ For multiple regression, R-squared is adjusted to account for additional parameters in the model.
- 🧮 Calculating the p-value involves comparing the sums of squares around the fit and the mean.
- 🔢 The number of parameters estimated (P fit) changes with the complexity of the regression model.
- 🆚 Multiple regression allows for comparison between models with different numbers of predictors to determine if additional data is beneficial.
- 📊 The F value is calculated similarly for both simple and multiple regression, but with different parameters.
- 💻 An additional StatQuest episode demonstrates how to perform multiple regression in R, detailing the interpretation of the output.
Q & A
What is the main topic of this StatQuest episode?
-The main topic of this StatQuest episode is multiple regression, which is explained as an extension of linear regression.
Who is the presenter of StatQuest?
-Josh Stommer is the presenter of StatQuest.
What is the relationship between simple linear regression and multiple regression?
-Simple linear regression is fitting a line to data, while multiple regression involves fitting a plane or higher-dimensional object to data, which essentially means adding more variables to the model.
What is the purpose of R-squared in the context of regression?
-R-squared is used to evaluate how well the regression model fits the data, and it is calculated in the same way for both simple and multiple regression.
How does the addition of more data affect the calculation of R-squared in multiple regression?
-The R-squared value is adjusted to compensate for the additional parameters in the equation when more data is added to the model.
What is the role of the p-value in regression analysis?
-The p-value is used to determine the statistical significance of the model, and it is calculated by comparing the sums of squares around the fit and the mean.
What does 'P fit' represent in the context of calculating the F-value for regression?
-'P fit' represents the number of parameters that least-squares has to estimate in the regression equation.
Why is it necessary to compare simple and multiple regression?
-Comparing simple and multiple regression helps determine if adding additional variables to the model is worthwhile, by assessing if the increase in R-squared and the decrease in p-value are significant.
What is the significance of a large difference in R-squared values between simple and multiple regression?
-A large difference in R-squared values between simple and multiple regression indicates that including additional variables significantly improves the model's fit.
What does a small p-value suggest when comparing simple and multiple regression?
-A small p-value when comparing simple and multiple regression suggests that the improvement in the model with additional variables is statistically significant.
Is there a follow-up StatQuest episode that demonstrates how to perform multiple regression in R?
-Yes, there is a follow-up episode that shows how to perform multiple regression in R, detailing the interpretation of the output.
Outlines
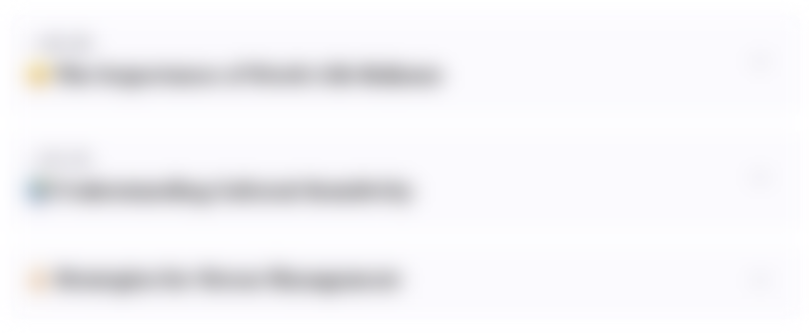
Этот раздел доступен только подписчикам платных тарифов. Пожалуйста, перейдите на платный тариф для доступа.
Перейти на платный тарифMindmap
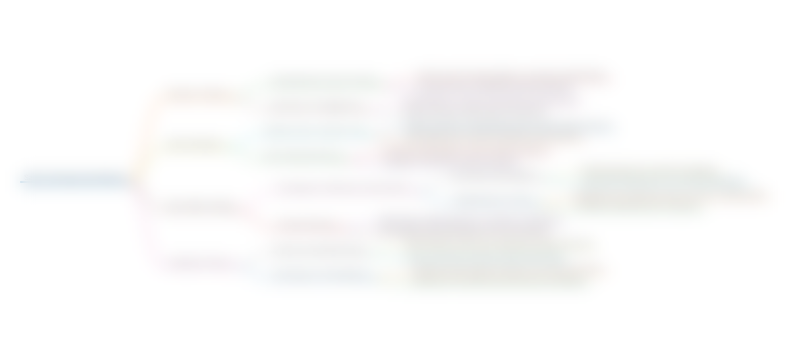
Этот раздел доступен только подписчикам платных тарифов. Пожалуйста, перейдите на платный тариф для доступа.
Перейти на платный тарифKeywords
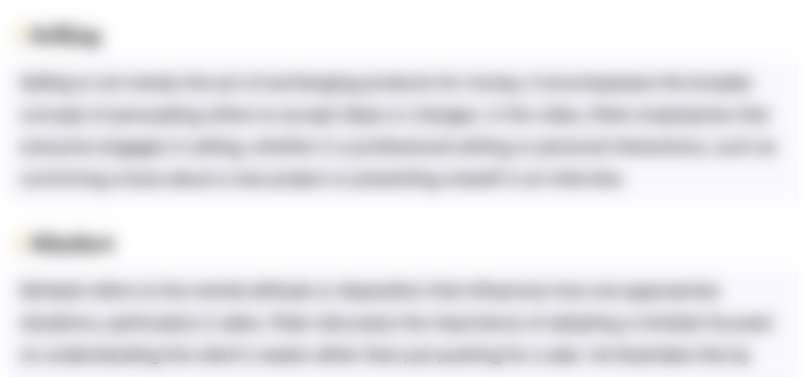
Этот раздел доступен только подписчикам платных тарифов. Пожалуйста, перейдите на платный тариф для доступа.
Перейти на платный тарифHighlights
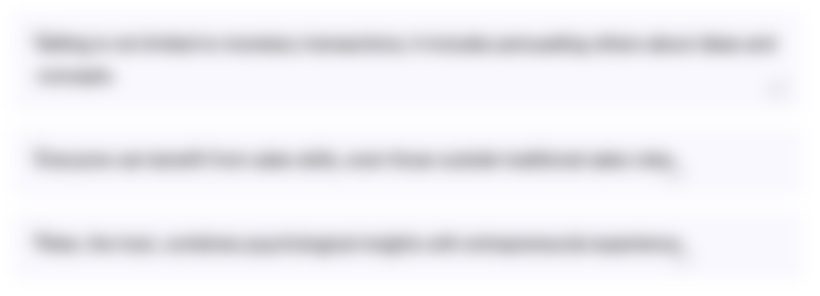
Этот раздел доступен только подписчикам платных тарифов. Пожалуйста, перейдите на платный тариф для доступа.
Перейти на платный тарифTranscripts
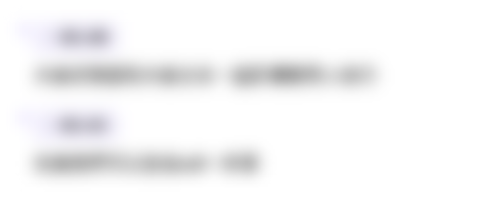
Этот раздел доступен только подписчикам платных тарифов. Пожалуйста, перейдите на платный тариф для доступа.
Перейти на платный тариф5.0 / 5 (0 votes)