Llama 3.2 is HERE and has VISION 👀
Summary
TLDRMeta has unveiled Llama 3.2, an upgrade to its AI model with added vision capabilities. The new models include an 11 billion parameter and a 90 billion parameter version, both with vision, and text-only models of 1 billion and 3 billion parameters for edge devices. These are designed to run tasks like summarization and rewriting locally. Llama 3.2 also introduces the Llama stack for simplified development and is optimized for Qualcomm processors. Benchmarks show it outperforming peers in its class.
Takeaways
- 🚀 **Llama 3.2 Release**: Meta has released Llama 3.2, an upgrade from Llama 3.1, with new capabilities.
- 👀 **Vision Capabilities**: Llama 3.2 introduces vision capabilities to the Llama family of models.
- 🧠 **Parameter Sizes**: Two new vision-capable models are available, one with 11 billion parameters and another with 90 billion parameters.
- 🔩 **Drop-in Replacements**: The new models are designed to be drop-in replacements for Llama 3.1, requiring no code changes.
- 📱 **Edge Device Models**: Two text-only models (1 billion and 3 billion parameters) are optimized for edge devices like smartphones and IoT devices.
- 🌐 **AI at the Edge**: The script emphasizes the trend of pushing AI compute to edge devices.
- 📊 **Performance Benchmarks**: Llama 3.2 models outperform their peers in benchmark tests for summarization, instruction following, and rewriting tasks.
- 🔧 **Optimized for Qualcomm**: The models are optimized for Qualcomm and MediaTek processors, indicating a focus on mobile and edge computing.
- 🛠️ **Llama Stack Distributions**: Meta is releasing Llama Stack, a set of tools to simplify working with Llama models for production applications.
- 📈 **Synthetic Data Generation**: Llama 3.1 is used to generate synthetic data to improve the performance of Llama 3.2 models.
- 🔎 **Vision Task Support**: The largest Llama 3.2 models support image reasoning for tasks like document understanding and visual grounding.
Q & A
What is the main update in Llama 3.2?
-Llama 3.2 introduces vision capabilities to the Llama family of models, allowing them to 'see' things. This is a significant update from the previous versions which were text-only.
What are the different versions of Llama 3.2 models mentioned in the script?
-The script mentions four versions: an 11 billion parameter version with vision capabilities, a 90 billion parameter version with vision capabilities, a 1 billion parameter text-only model, and a 3 billion parameter text-only model.
What does 'drop-in replacement' mean in the context of Llama 3.2 models?
-A 'drop-in replacement' means that the new models can be used in place of the older Llama 3.1 models without requiring any changes to the existing code.
What is special about the 1 billion and 3 billion parameter models?
-These models are designed to be run on edge devices, such as smartphones, computers, and IoT devices. They are optimized for on-device AI compute, which is a growing trend in the industry.
What are some use cases for the Llama 3.2 models?
-The Llama 3.2 models are capable of tasks like summarization, instruction following, rewriting tasks, and image understanding tasks such as document level understanding, image captioning, and visual grounding.
How does the Llama 3.2 model compare to its peers in terms of performance?
-The script suggests that the Llama 3.2 models, especially the 3 billion parameter version, perform incredibly well compared to models in the same class, such as GPT-J 2B and 53.5 Mini.
What is the significance of the Llama Stack distributions released by Meta?
-The Llama Stack distributions are a set of tools that simplify how developers work with Llama models in different environments, enabling turnkey deployment of applications with integrated safety.
What are the capabilities of the Llama 3.2 models with vision?
-The 11 billion and 90 billion parameter models support image reasoning, including document understanding, image captioning, and visual grounding tasks.
How did Meta achieve the integration of vision capabilities into the Llama 3.2 models?
-Meta trained a set of adapter weights that integrate the pre-trained image encoder into the pre-trained language model, using a new technique that involves cross attention layers.
What is the purpose of the synthetic data generation mentioned in the script?
-Synthetic data generation is used to augment question and answer pairs on top of in-domain images, leveraging the Llama 3.1 model to filter and augment data, which helps improve the model's performance.
How are the smaller 1 billion and 3 billion parameter models created from the larger Llama 3.2 models?
-The smaller models are created using a combination of pruning and distillation methods on the larger 11 billion parameter model, making them lightweight and capable of running efficiently on devices.
Outlines
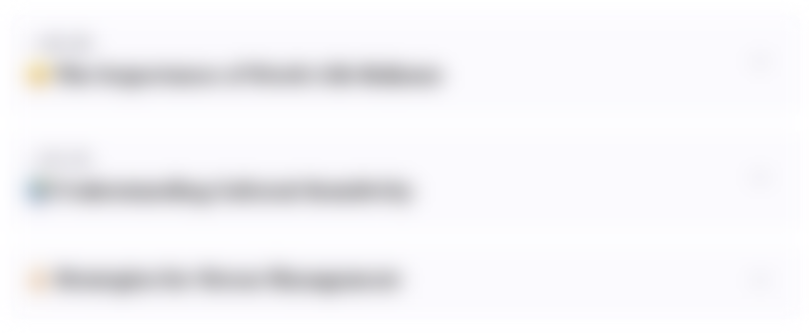
Этот раздел доступен только подписчикам платных тарифов. Пожалуйста, перейдите на платный тариф для доступа.
Перейти на платный тарифMindmap
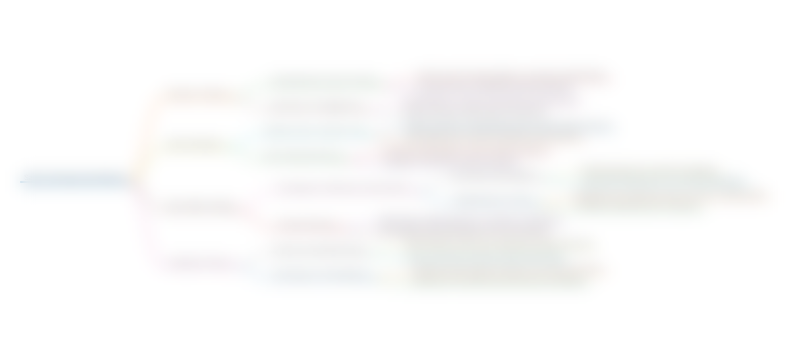
Этот раздел доступен только подписчикам платных тарифов. Пожалуйста, перейдите на платный тариф для доступа.
Перейти на платный тарифKeywords
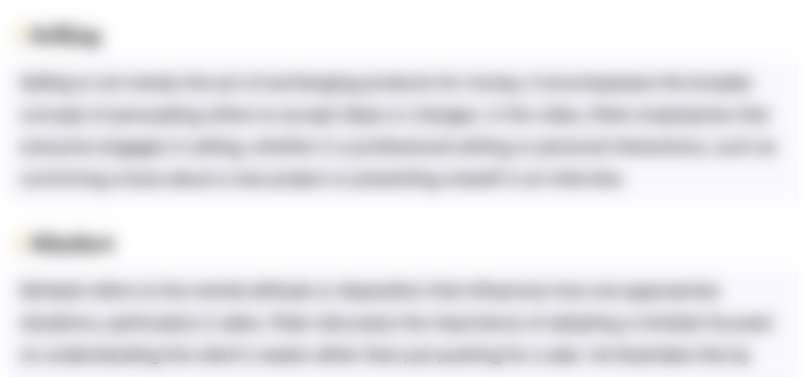
Этот раздел доступен только подписчикам платных тарифов. Пожалуйста, перейдите на платный тариф для доступа.
Перейти на платный тарифHighlights
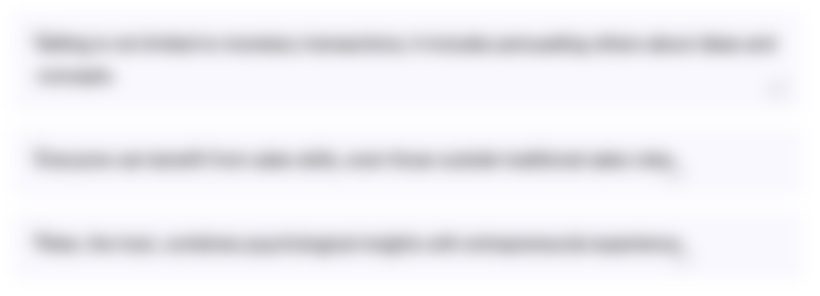
Этот раздел доступен только подписчикам платных тарифов. Пожалуйста, перейдите на платный тариф для доступа.
Перейти на платный тарифTranscripts
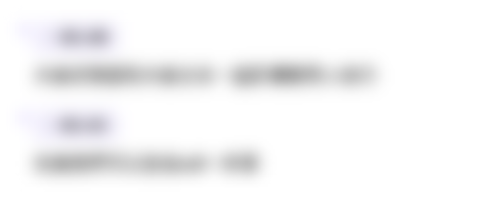
Этот раздел доступен только подписчикам платных тарифов. Пожалуйста, перейдите на платный тариф для доступа.
Перейти на платный тарифПосмотреть больше похожих видео
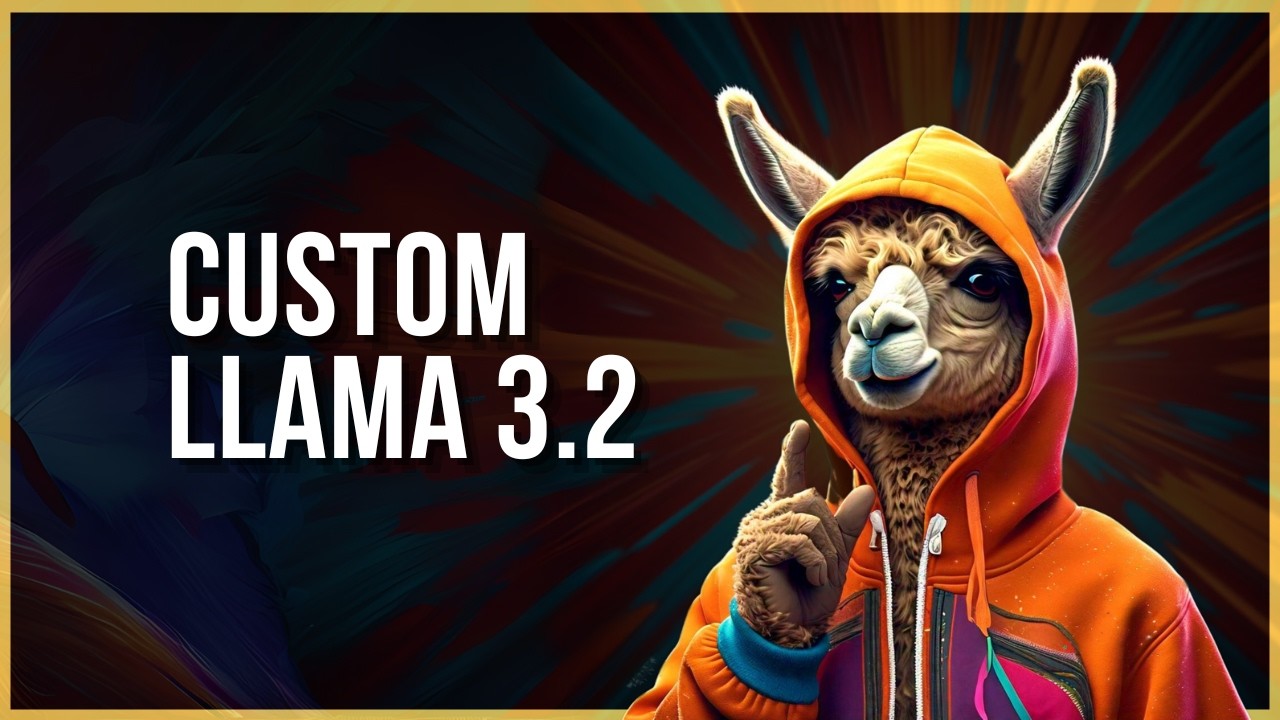
EASIEST Way to Fine-Tune LLAMA-3.2 and Run it in Ollama

Llama 3.2 is INSANE - But Does it Beat GPT as an AI Agent?
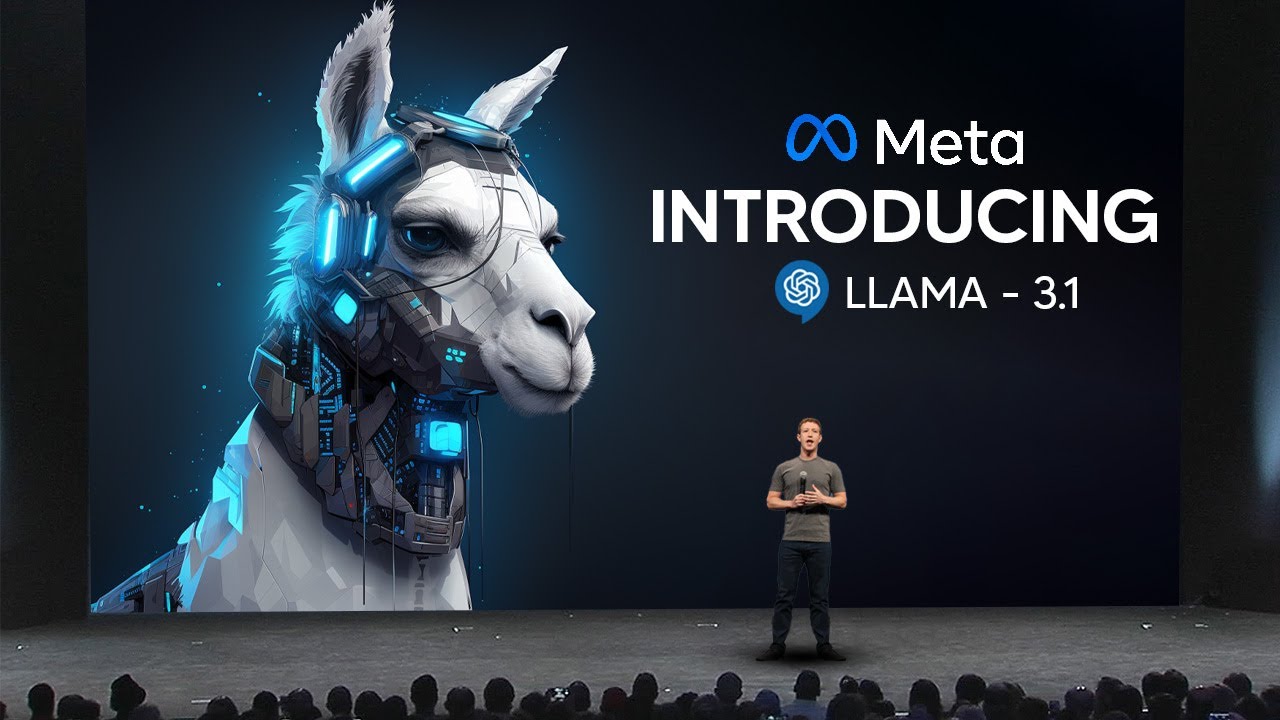
Metas LLAMA 405B Just STUNNED OpenAI! (Open Source GPT-4o)
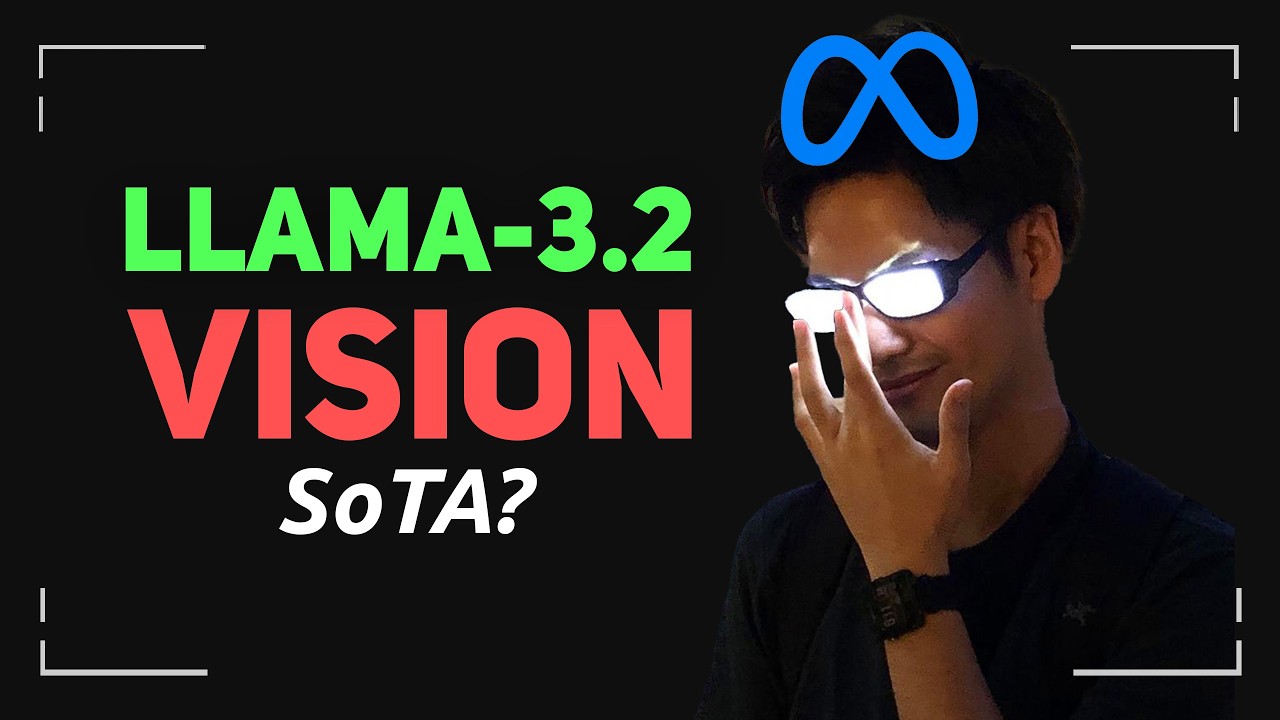
Llama 3.2 Deep Dive - Tiny LM & NEW VLM Unleashed By Meta

🚨BREAKING: LLaMA 3 Is HERE and SMASHES Benchmarks (Open-Source)
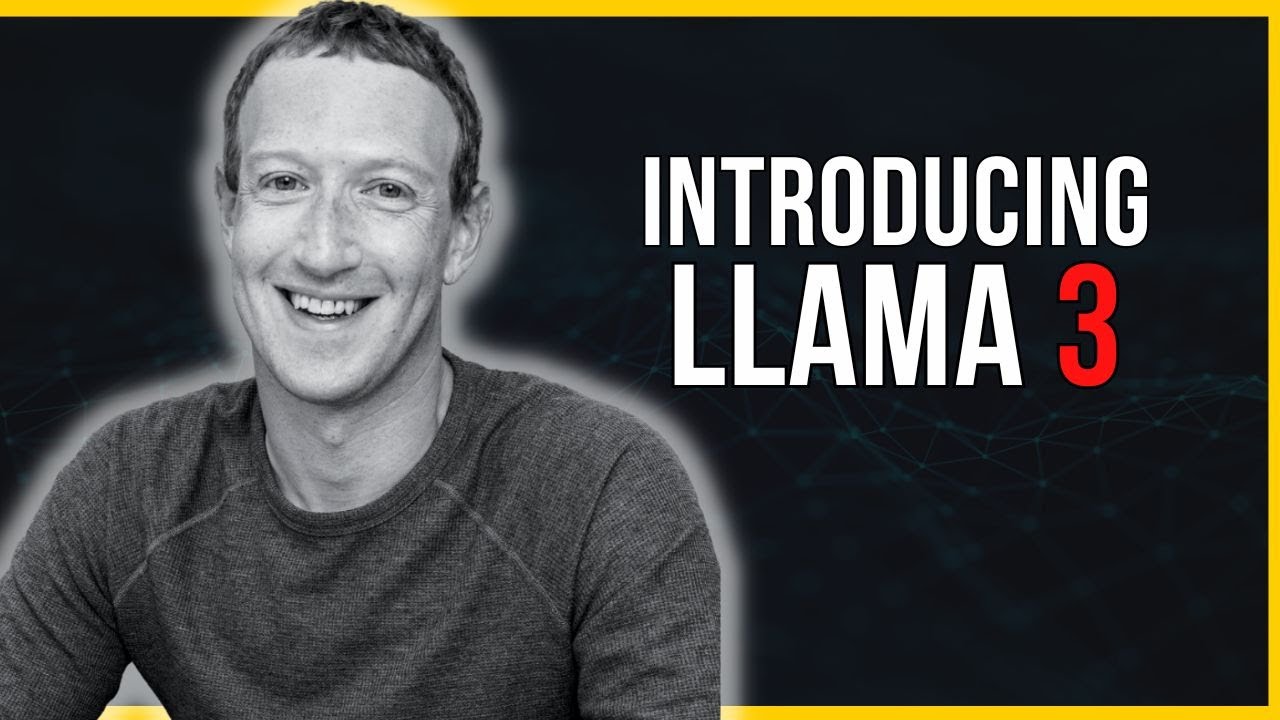
LLAMA 3 Released - All You Need to Know
5.0 / 5 (0 votes)