W01 Clip 06
Summary
TLDRThe video script discusses the importance of dense encoding matrices in word embeddings to capture context, semantic meaning, and relationships between words. It uses a table to illustrate how words are represented numerically based on shared attributes or latent factors, such as 'vehicle' or 'luxury'. The script explains how similar values in the matrix indicate word similarity and how distance measures can reveal relationships. It also touches on the challenge of defining clear factors with a large vocabulary and many dimensions, and hints at the process of learning these word embeddings.
Takeaways
- 📊 The encoding matrix should be dense to effectively represent words in a context-rich manner.
- 🔢 Words are represented as numbers that express underlying factors or features, such as association and relationship between words.
- 🚗 The first row in the table captures a latent factor like 'vehicle' due to the high and similar values for car, bike, Mercedes-Benz, and Harley-Davidson.
- 🏎️ The second row's high values for Mercedes-Benz and Harley-Davidson suggest a 'luxury' factor as the differentiating attribute.
- 🍊 The third row indicates 'fruit' as a latent factor with high values for orange and mango.
- 🏢 The fourth row could represent 'company' as a factor, considering orange and mango are also names of companies.
- 🌐 Columns in the table show the weights of words across all dimensions, with similar values indicating similarity between words.
- 🔗 Words with closely related meanings, like 'orange' and 'mango', have similar values across most factors.
- 📏 Distance measures such as Euclidean or Manhattan can be used to infer the association between words based on their columnar values.
- 🔑 The number of factors is a hyperparameter that can be adjusted (e.g., 50, 100, 300, 500) to capture more relationships but also increases computation.
- 🤖 In real-world scenarios, it's challenging to distinctly identify single factors due to potential overlaps in a large vocabulary with limited dimensions.
Q & A
What is the significance of a dense encoding or embedding matrix in word representation?
-A dense encoding or embedding matrix is significant because it captures the context, semantic meaning, and relationships between words. It represents words as numbers that express underlying factors or features, which account for the association between words.
How does the magnitude of word vectors in the matrix relate to the common attributes among words?
-The magnitude of word vectors in the matrix is high and similar for words that share common attributes. For example, words like 'car', 'bike', 'Mercedes-Benz', and 'Harley-Davidson' have high and similar magnitudes because they all share the attribute of being vehicles.
What is a latent factor in the context of word embeddings?
-A latent factor in word embeddings refers to an underlying characteristic or feature that is captured by the rows of the matrix. It helps to differentiate and relate words based on their common attributes, such as 'luxury' for 'Mercedes-Benz' and 'Harley-Davidson'.
How does the table's column represent the weights of words?
-The columns of the table represent the weights of words across all dimensions or axes. Similar values across the columns indicate that the words are similar, and points in the dimensional space would be close to each other.
What can be inferred from the similarity of values across factors for words like 'car' and 'bike'?
-From the similarity of values across factors, it can be inferred that words like 'car' and 'bike' are closely related, and the relationship is stronger than that between words like 'car' and 'orange'.
How does the distance between columnar values help in understanding word associations?
-The distance between columnar values, measured using distance measures like Euclidean or Manhattan distance, helps infer the underlying association between words. Similar values indicate a strong association, such as between 'Mercedes-Benz' and 'Harley-Davidson'.
What is the role of factors like 'vehicle', 'luxury', 'fruit', and 'company' in word embeddings?
-Factors like 'vehicle', 'luxury', 'fruit', and 'company' in word embeddings help in capturing the semantic relationships and categorizations of words. They allow the model to understand and represent the contextual meaning of words more accurately.
How does the number of factors in the matrix affect the computation?
-The number of factors in the matrix is a hyperparameter that determines the complexity of the model. More factors can capture more nuances in word relationships but also increase computational requirements.
Why is it challenging to identify underlying factors in real-world scenarios?
-It is challenging to identify underlying factors in real-world scenarios because factors often overlap, especially with a large vocabulary and limited dimensions. This makes it difficult to distinctly attribute a single row to a single factor.
How can word vectors be visualized in two dimensions to show relationships between words?
-Word vectors can be visualized in two dimensions by plotting them based on their distances. Words that are closely related, like 'car' and 'bike', would be placed near each other, while less related words, like 'orange' and 'mango', would be farther but still closer to each other than to the vehicle-related words.
What is the process of learning word embeddings?
-Learning word embeddings involves training a model to represent words as vectors in a high-dimensional space such that the relative positions of the vectors capture semantic meanings and relationships between words.
Outlines
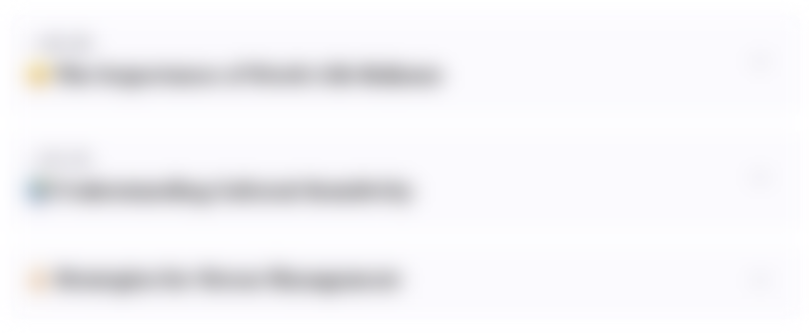
Этот раздел доступен только подписчикам платных тарифов. Пожалуйста, перейдите на платный тариф для доступа.
Перейти на платный тарифMindmap
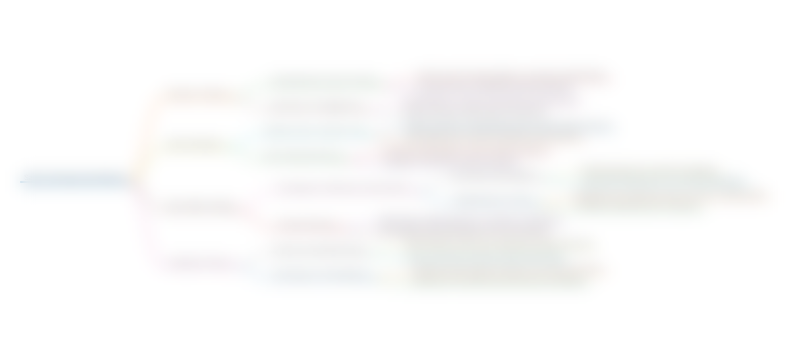
Этот раздел доступен только подписчикам платных тарифов. Пожалуйста, перейдите на платный тариф для доступа.
Перейти на платный тарифKeywords
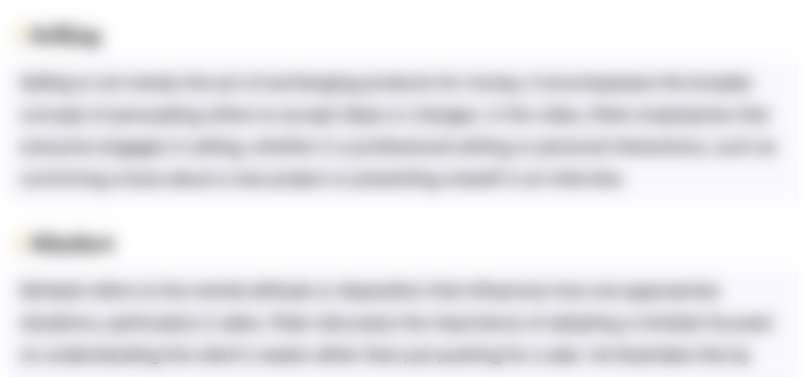
Этот раздел доступен только подписчикам платных тарифов. Пожалуйста, перейдите на платный тариф для доступа.
Перейти на платный тарифHighlights
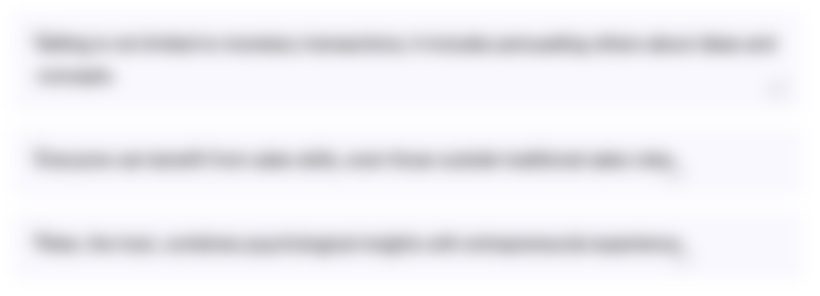
Этот раздел доступен только подписчикам платных тарифов. Пожалуйста, перейдите на платный тариф для доступа.
Перейти на платный тарифTranscripts
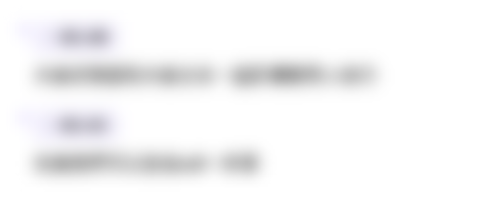
Этот раздел доступен только подписчикам платных тарифов. Пожалуйста, перейдите на платный тариф для доступа.
Перейти на платный тарифПосмотреть больше похожих видео
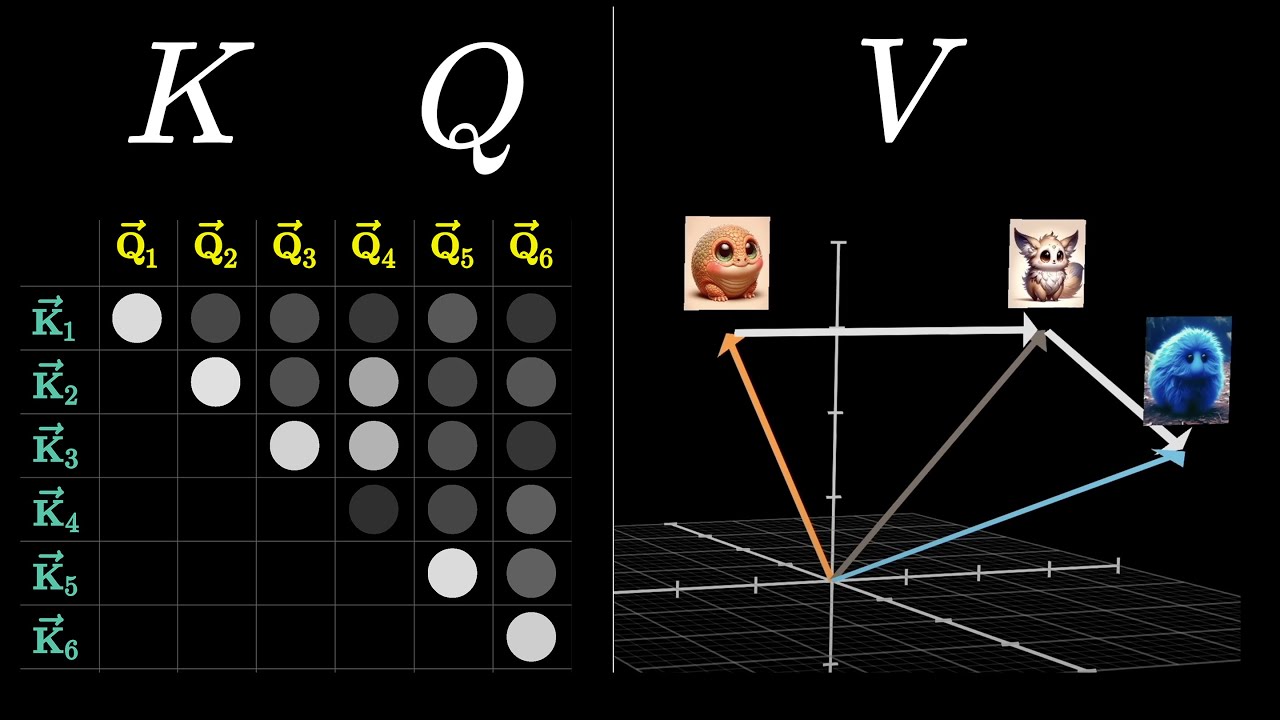
Visualizing Attention, a Transformer's Heart | Chapter 6, Deep Learning

Practical Intro to NLP 23: Evolution of word vectors Part 2 - Embeddings and Sentence Transformers
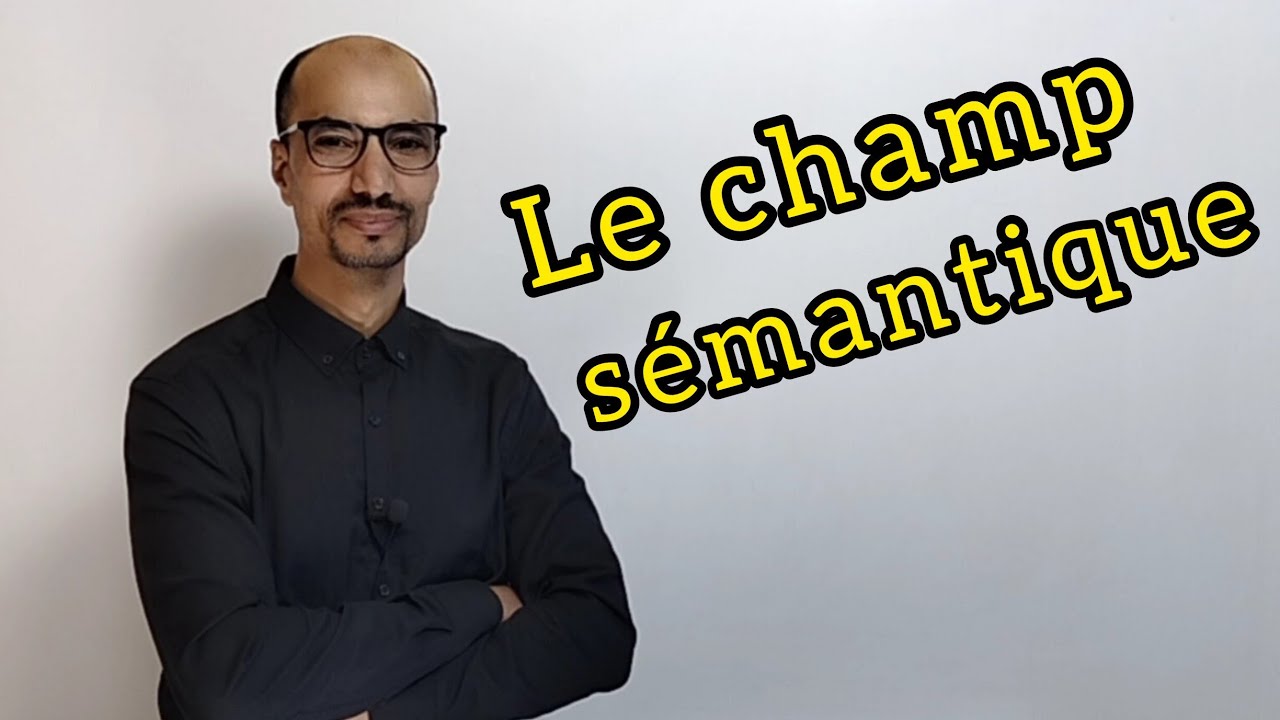
Le champ sémantique

Techniques to Enhance Learning and Memory | Nancy D. Chiaravalloti | TEDxHerndon
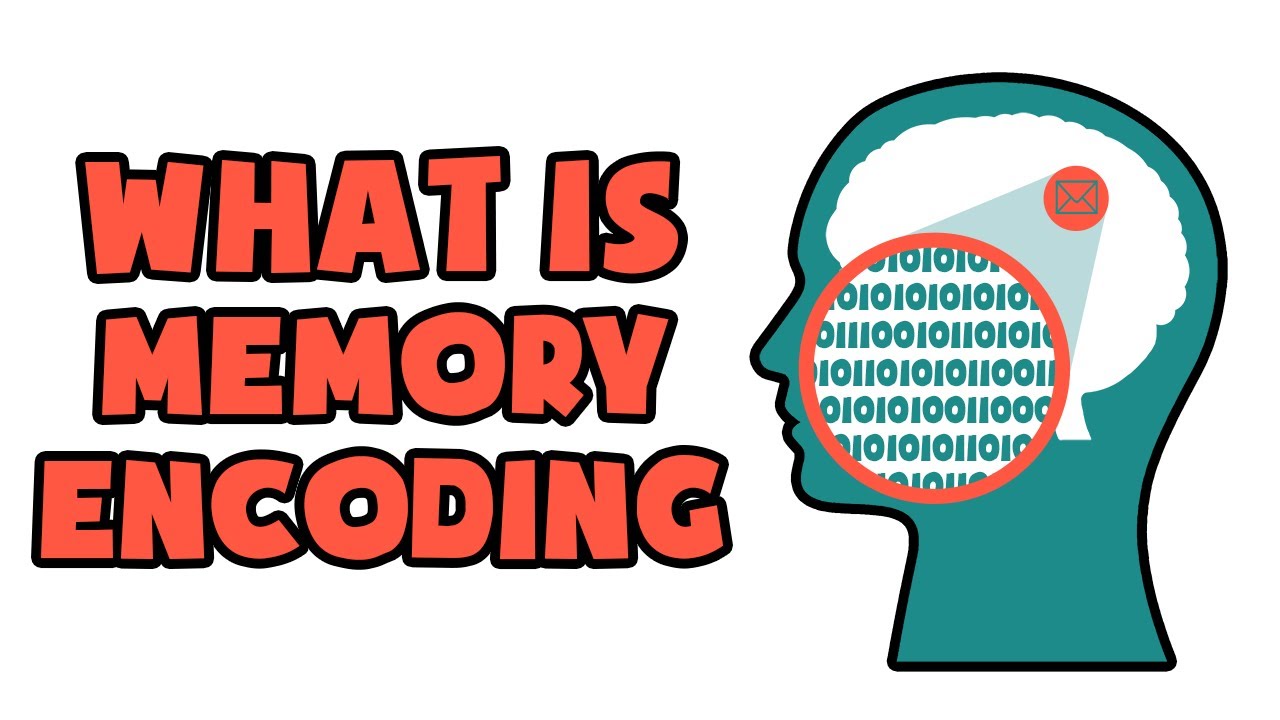
What is Memory Encoding | Explained in 2 min

SEM101 - Word Semantics
5.0 / 5 (0 votes)