Nick Emmons: Allora
Summary
TLDRIn this video, Nick, co-founder of Aora, introduces their self-improving decentralized AI network. The platform aims to address the challenge of siloed machine intelligence by enabling different AI models to learn from each other in a modular, decentralized way. Aora creates a collective intelligence network, optimizing machine learning objectives and producing aggregated outputs that outperform individual models. With applications in finance, AI-powered data feeds, and DeFi, the network is designed to continuously improve through a combination of inference and forecasting workers, all while preserving privacy and scalability.
Takeaways
- 🧠 The AI stack can be broken down into four core layers: data, compute, intelligence, and execution.
- 🛠️ Decentralized AI networks, like blockchain, are built modularly for increased coordination and composability.
- 📊 Aura aims to solve the problem of siloed machine intelligence by creating a decentralized AI network that allows models to learn from one another.
- 🤖 The network uses an inference synthesis mechanism, which stitches together model outputs for a more intelligent and performant outcome.
- 💡 Aura's core focus is on turning intelligence into a digital commodity and enabling models to work together to achieve collective intelligence.
- 🔐 The network supports both open and closed-source models without revealing proprietary data, making it suitable for financial settings where privacy is crucial.
- 📈 Financial applications, especially AI-powered price feeds for longtail assets, are a key area where decentralized AI networks like Aura can provide value.
- 💸 Aura sees decentralized finance (DeFi) and AI agents as the next frontier for executing advanced trading and risk management strategies.
- 📉 AI-driven risk modeling can help address complex risks in decentralized finance, especially for staking and liquidity provisioning.
- 🚀 Aura is currently in the testnet phase, focusing on onboarding machine intelligence to enhance the network's collective intelligence before the mainnet launch.
Q & A
What is the core concept behind Aora?
-Aora is a self-improving, decentralized AI Network that aims to turn intelligence into a digital commodity by allowing different AI models to learn from one another and become more composable or compatible in a decentralized setting.
How does Aora address the issue of siloed machine intelligence?
-Aora addresses the issue of siloed machine intelligence by enabling models to learn from each other and collectively optimize different machine learning objectives, resulting in an aggregate model output that outperforms any individual model within the network.
What are the four core layers of the decentralized AI stack as described in the script?
-The four core layers of the decentralized AI stack are Data, Compute, Intelligence, and Execution.
What is the role of blockchains in the decentralized AI stack according to the script?
-Blockchains serve as powerful coordination mechanisms that can turn potentially nebulous resources into more tangible digital commodities, facilitating the modular construction of the decentralized AI stack.
How does Aora's network work at a high level?
-Aora's network operates through an inference synthesis mechanism that stitches together different model outputs to create a more intelligent network-level output. It involves inference workers responding to requests, forecasting workers predicting the loss of inference workers, and reputers evaluating model performance.
What is the significance of the forecasting workers in Aora's network?
-Forecasting workers in Aora's network are crucial for introducing context awareness, allowing the network to outperform individual workers by learning the contexts in which different models perform well, thus enhancing the network's overall performance.
Why is the ability to support open and closed-source models important in Aora's network?
-Supporting open and closed-source models is important because it allows the network to maintain a wide range of use cases, especially in financial settings where preserving proprietary information of models is crucial.
What are the four core features of Aora as mentioned in the script?
-The four core features of Aora are collective intelligence, iterative learning at both the network and model levels, contextual awareness, and privacy protection that supports closed-source model participation.
What are some initial focus areas for Aora's application?
-Some initial focus areas for Aora's application include AI-powered data feeds, particularly for longtail assets, AI agents for decentralized finance, and risk modeling in the context of reaking and other financial primitives.
What is the current phase of Aora's development as per the script?
-As per the script, Aora is currently in the test net phase one, with test net phase 2 launching in about a week and a half, followed by the main net soon after.
What is the primary focus of Aora Labs at the moment?
-The primary focus of Aora Labs at the moment is onboarding workers and model creators to bring a critical mass of machine intelligence onto the network, as the network's intelligence increases with more models participating.
Outlines
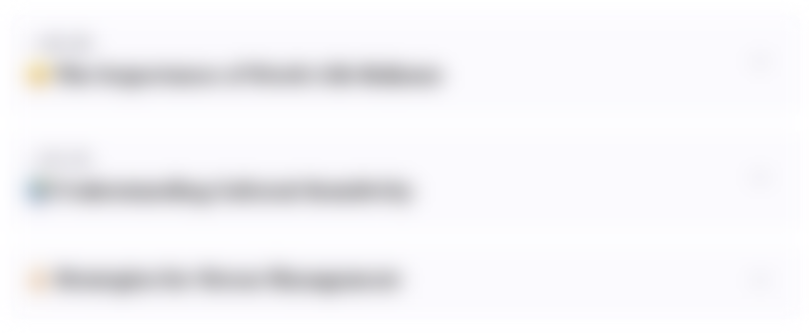
Этот раздел доступен только подписчикам платных тарифов. Пожалуйста, перейдите на платный тариф для доступа.
Перейти на платный тарифMindmap
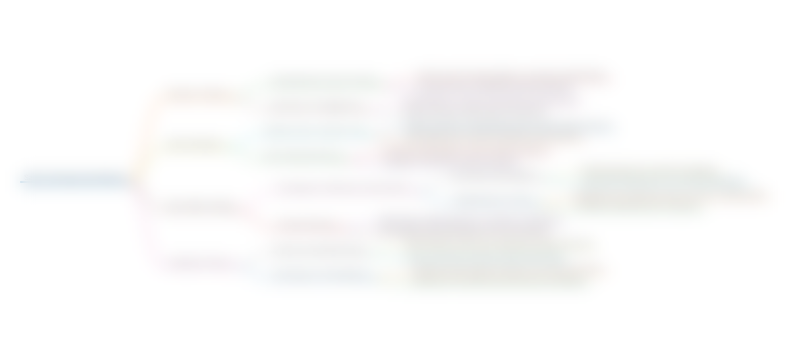
Этот раздел доступен только подписчикам платных тарифов. Пожалуйста, перейдите на платный тариф для доступа.
Перейти на платный тарифKeywords
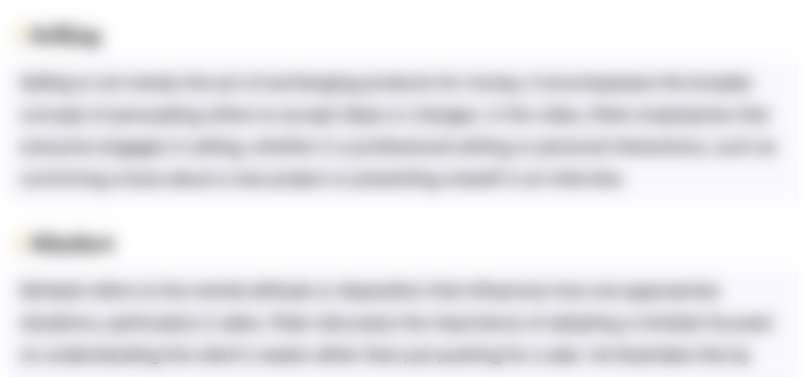
Этот раздел доступен только подписчикам платных тарифов. Пожалуйста, перейдите на платный тариф для доступа.
Перейти на платный тарифHighlights
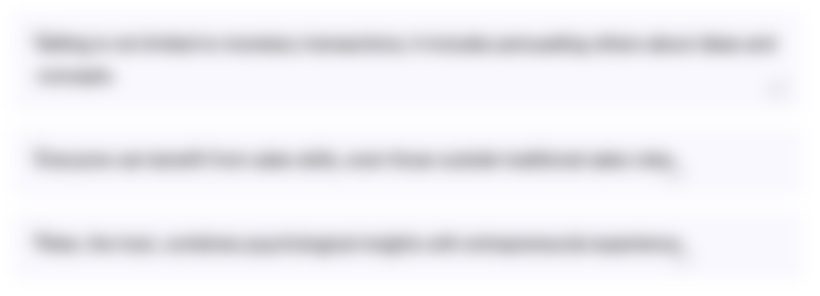
Этот раздел доступен только подписчикам платных тарифов. Пожалуйста, перейдите на платный тариф для доступа.
Перейти на платный тарифTranscripts
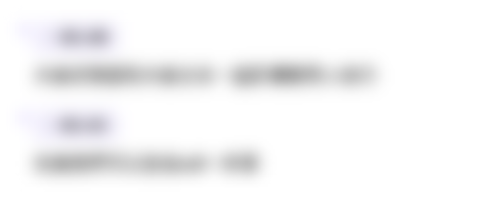
Этот раздел доступен только подписчикам платных тарифов. Пожалуйста, перейдите на платный тариф для доступа.
Перейти на платный тарифПосмотреть больше похожих видео
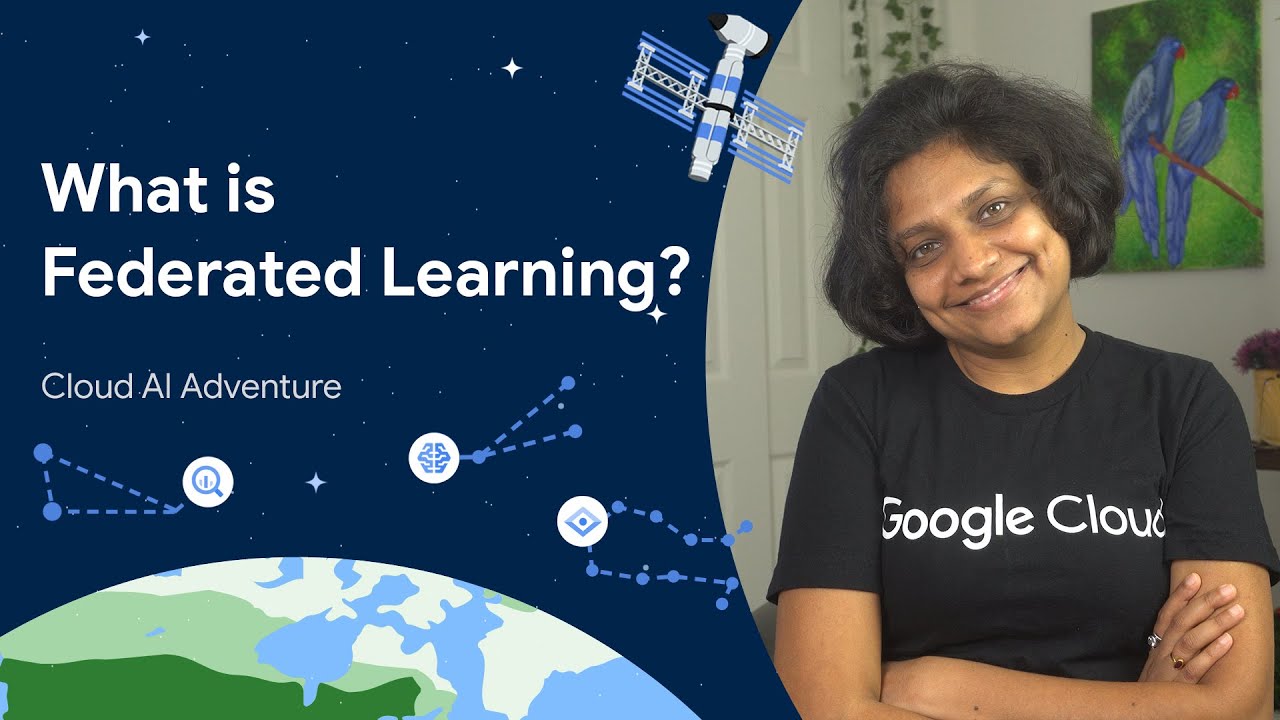
What is Federated Learning?
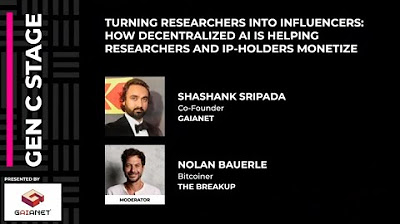
Exploring the Value of Knowledge in Web3 | #Consensus2024 AI Summit Recap
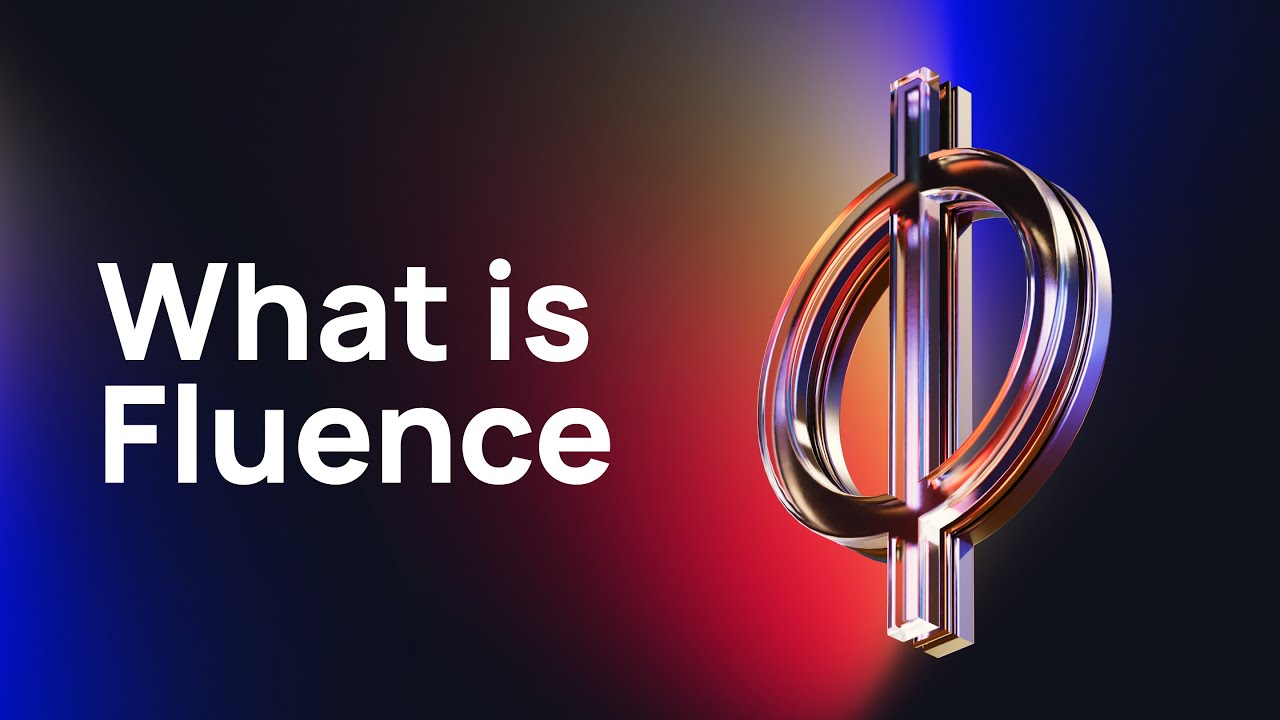
Fluence: Cloudless DePIN Computing Platform

How Federated Learning works? Clearly Explained|

CAT: Redbelly Network Announces Compliant Asset Tokenisation Solution
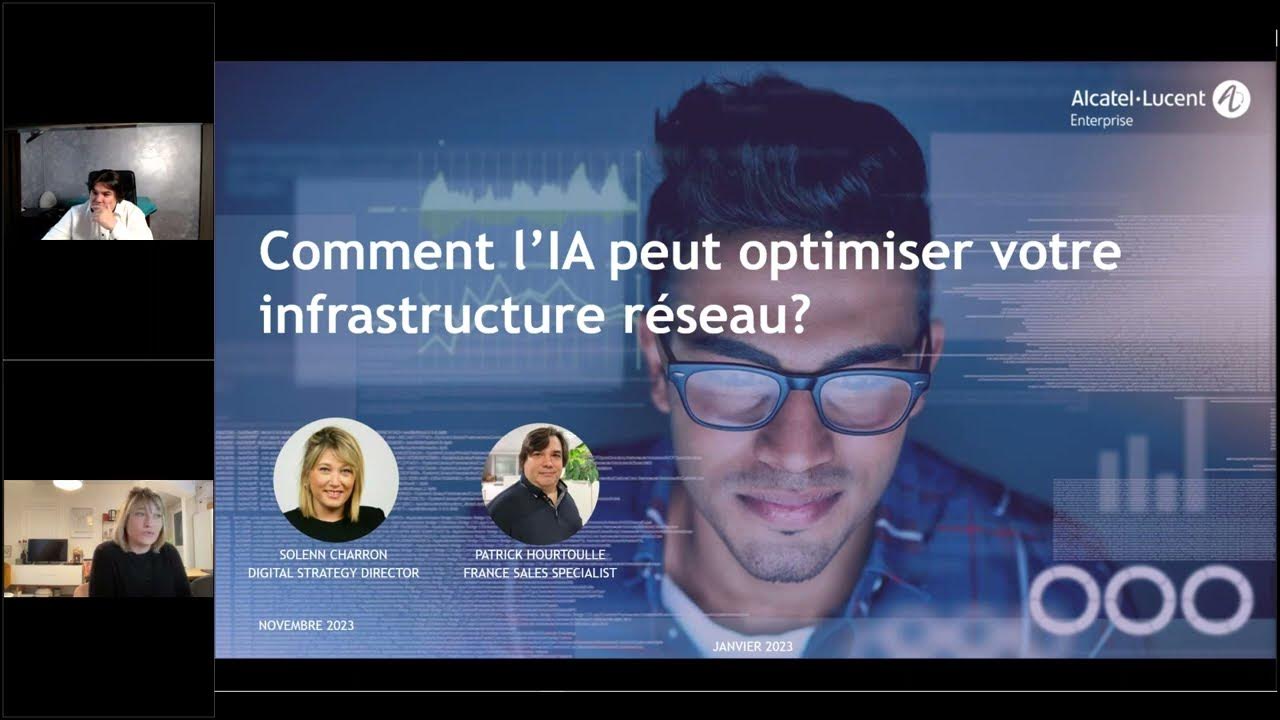
Avec OmniVista Network Advisor, mettez l’IA au service de votre réseau
5.0 / 5 (0 votes)