7. Frank Block, Head of IT Data Science at Roche, Switzerland
Summary
TLDRDer Vortrag von Frank umfasst sein persönliches Engagement in der KI-Branche, beginnend mit seiner Ausbildung als Physiker und seiner Zeit am CERN. Er diskutiert die Bedeutung von Datenqualität, die auch nach Jahrzehnten immer noch eine Herausforderung darstellt, und betont die Notwendigkeit, den Fokus auf die Produktivität und den Nutzen von KI-Anwendungen zu legen. Er reflektiert über die Entwicklung von KI von Expertensystemen über neuronale Netzwerke hin zu heutigen automatisierten Modellen und unterstreicht die Bedeutung von Interdisziplinarität, Soft Skills und der Bedeutung von Qualität und Kundenzentrierung in der KI-Entwicklung.
Takeaways
- 🔬 Datenqualität ist ein immerwährendes Thema, das die Produktivität von AI-Teams und die Skalierung von KI-Projekten beeinträchtigt.
- 🧠 Die Industrielle Nutzung von KI erfordert tiefgreifendes Verständnis der Geschäftslogik und der Wertschöpfung für Stakeholder.
- 💡 Die technische Seite erfordert fortlaufendes Lernen und gute Kenntnisse in Datenwissenschaft, Cloud-Architekturen und fortgeschrittenen Analysewerkzeugen.
- 🛠️ Technologie ist ein Enabler, aber das zentrale Ziel ist es, Probleme zu lösen und nicht nur um der Technologie willen zu arbeiten.
- 🤝 Interdisziplinarität ist entscheidend für erfolgreiche KI-Projekte, da sie die Komplementarität verschiedener Perspektiven und Fähigkeiten nutzt.
- 💼 Soft Skills wie das Fähigkeiten zu netzwerken und die Fähigkeit, Geschichten in der Sprache der Stakeholder zu erzählen, sind für KI-Spezialisten wichtig.
- 👨🔬 Die Rolle des Data Scientists hat sich von der reinen Forschung hin zu einer Produktions- und Operations-Orientierung entwickelt.
- 🔍 Die KI-Industrie hat sich von der Ersetzung von Menschen durch KI hin zu einer stärkeren Betonung der Verstärkung menschlicher Fähigkeiten entwickelt.
- 🏢 Organisationsbarriere und mangelnde Bereitschaft der mittleren Management-Ebene, KI zu adoptieren, können die Skalierung von KI-Projekten verlangsamen.
- 📈 Die Bewegung von Daten als Produkt und die Einführung von DevOps-ähnlichen Prinzipien in der KI-Entwicklung sind wichtige Entwicklungen.
Q & A
Wie hat die Erfahrung des Redners bei CERN seine Karriere beeinflusst?
-Der Redner ist Physiker ausgebildet und hat bei CERN, dem größten Beschleuniger der Welt, seine Masterarbeit geschrieben. Diese Erfahrung hat ihn stark beeinflusst und ihn für die Arbeit mit großen Datenmengen und der Analyse solcher Daten interessiert gemacht.
Was ist der Large Electron-Positron Collider und wie wichtig war er für die Forschung?
-Der Large Electron-Positron Collider (LEP) ist ein 27 Kilometer umfassendes Gerät, das 100 Meter unter der Erdoberfläche liegt. Es war ein gigantisches Instrument, das für die Forschung zum Verständnis der Bedingungen des Urknalls von großer Bedeutung war.
Wie viele Protonen-Proton-Kollisionen pro Sekunde wurden im Large Hadron Collider gemessen?
-Im Large Hadron Collider wurden zwei Milliarden Protonen-Proton-Kollisionen pro Sekunde gemessen, was enorme Mengen an Daten erzeugt hat.
Wie hat sich die Einstellung des Redners zur künstlichen Intelligenz (KI) entwickelt?
-Der Redner begann mit der Arbeit an Expertensystemen und wechselte dann zu neuronalen Netzwerken, nachdem bewiesen wurde, dass nicht-lineare Neuronen fast alles modellieren können, was zu einer Renaissance der KI-Forschung führte.
Was ist die Bedeutung von Datenqualität für KI-Anwendungen?
-Datenqualität ist ein konstanter Faktor, der die Skalierung von KI beeinträchtigt. Ohne hochwertige Daten ist es schwierig, KI-Teams produktiv zu gestalten und die volle Potenzial von KI zu nutzen.
Wie wichtig sind Soft Skills im Bereich der künstlichen Intelligenz?
-Soft Skills sind sehr wichtig, da sie das Verständnis und die Kommunikation zwischen verschiedenen Stakeholdern und Teams erleichtern. Dazu gehören Fähigkeiten wie das Aufbauen von Netzwerken, das Erzählen von Erfolgsgeschichten und das Anpassen des Tempos der Innovation an die Bedürfnisse anderer.
Was sind die Herausforderungen beim Übergang von Prototypen zu Produktion in der KI-Entwicklung?
-Ein Hauptherausforderung ist die Verkürzung der Zeit von der Erstellung eines Prototypen bis zur Produktion. Es ist wichtig, Plattformen zu nutzen, die einen nahtlosen Übergang ermöglichen, um die Zeit bis zur Produktion zu reduzieren.
Wie kann die Lücke zwischen verfügbaren Data Scientists und Marktbedarf überbrückt werden?
-Durch die Förderung von Self-Service-Analytik und Citizen Data Scientists kann die Lücke schrittweise überbrückt werden. Dies erfordert jedoch eine hohe Datenqualität, um sicherzustellen, dass die Ergebnisse der Analysen verlässlich sind.
Wie wichtig ist die Interdisziplinarität in KI-Projekten?
-Interdisziplinarität ist sehr wichtig, da sie die Zusammenarbeit verschiedener Fachbereiche wie Business, Data Science und Engineering ermöglicht, um komplexe Probleme zu lösen und umfassende KI-Lösungen zu entwickeln.
Was sind die aktuellen Entwicklungen, die der Redner in der KI-Branche beobachtet hat?
-Der Redner beobachtet eine Verschiebung von KI als Ersatzmöglichkeit für Menschen hin zu KI, die unsere Fähigkeiten erweitert und unsere Arbeit interessanter macht. Er betont auch die Notwendigkeit, Datenqualität und Governance zu verbessern, um KI erfolgreich zu skalieren.
Outlines
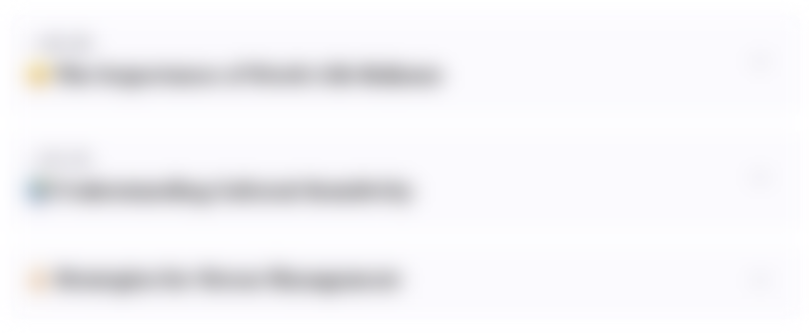
Этот раздел доступен только подписчикам платных тарифов. Пожалуйста, перейдите на платный тариф для доступа.
Перейти на платный тарифMindmap
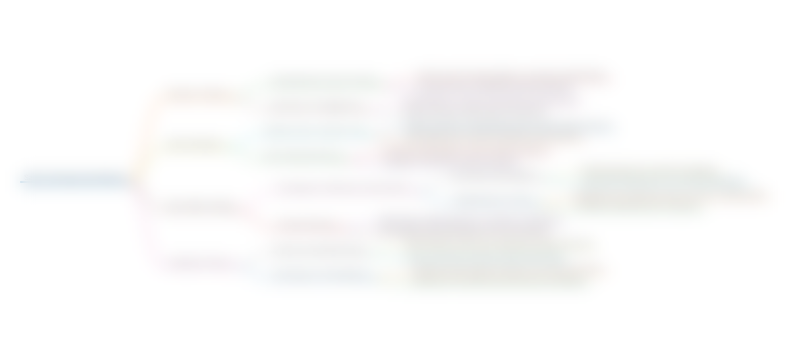
Этот раздел доступен только подписчикам платных тарифов. Пожалуйста, перейдите на платный тариф для доступа.
Перейти на платный тарифKeywords
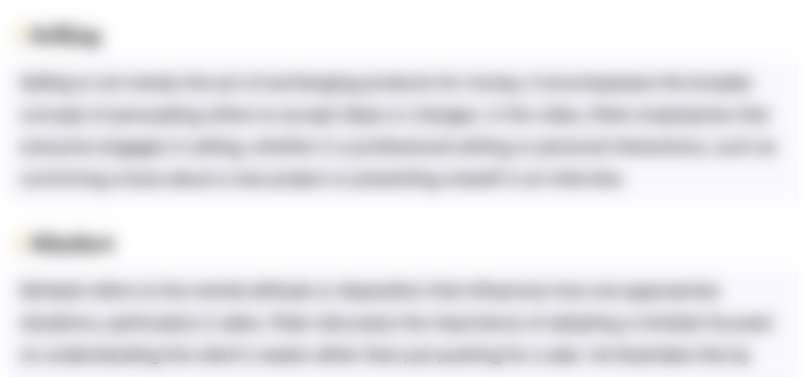
Этот раздел доступен только подписчикам платных тарифов. Пожалуйста, перейдите на платный тариф для доступа.
Перейти на платный тарифHighlights
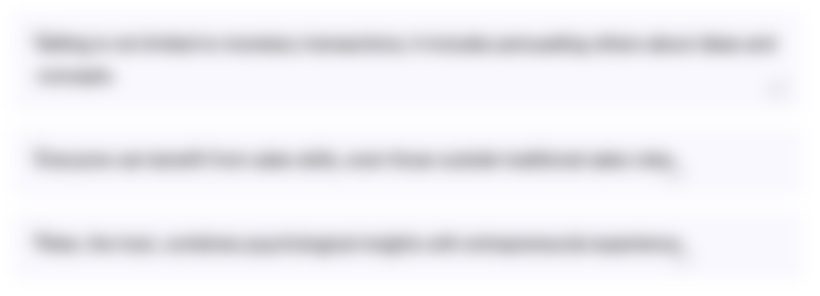
Этот раздел доступен только подписчикам платных тарифов. Пожалуйста, перейдите на платный тариф для доступа.
Перейти на платный тарифTranscripts
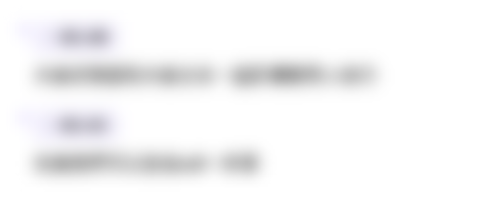
Этот раздел доступен только подписчикам платных тарифов. Пожалуйста, перейдите на платный тариф для доступа.
Перейти на платный тарифПосмотреть больше похожих видео
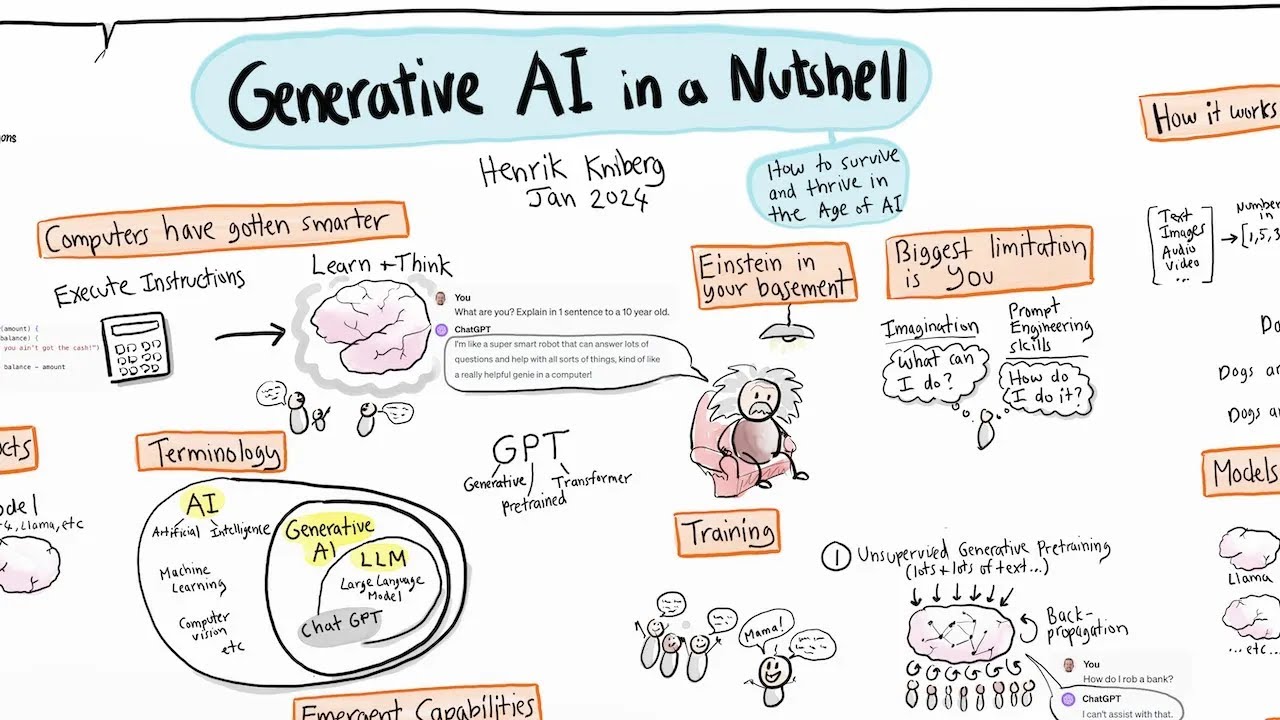
Generative KI auf den Punkt gebracht – Wie man im KI-Zeitalter besteht und erfolgreich ist (AI dub)
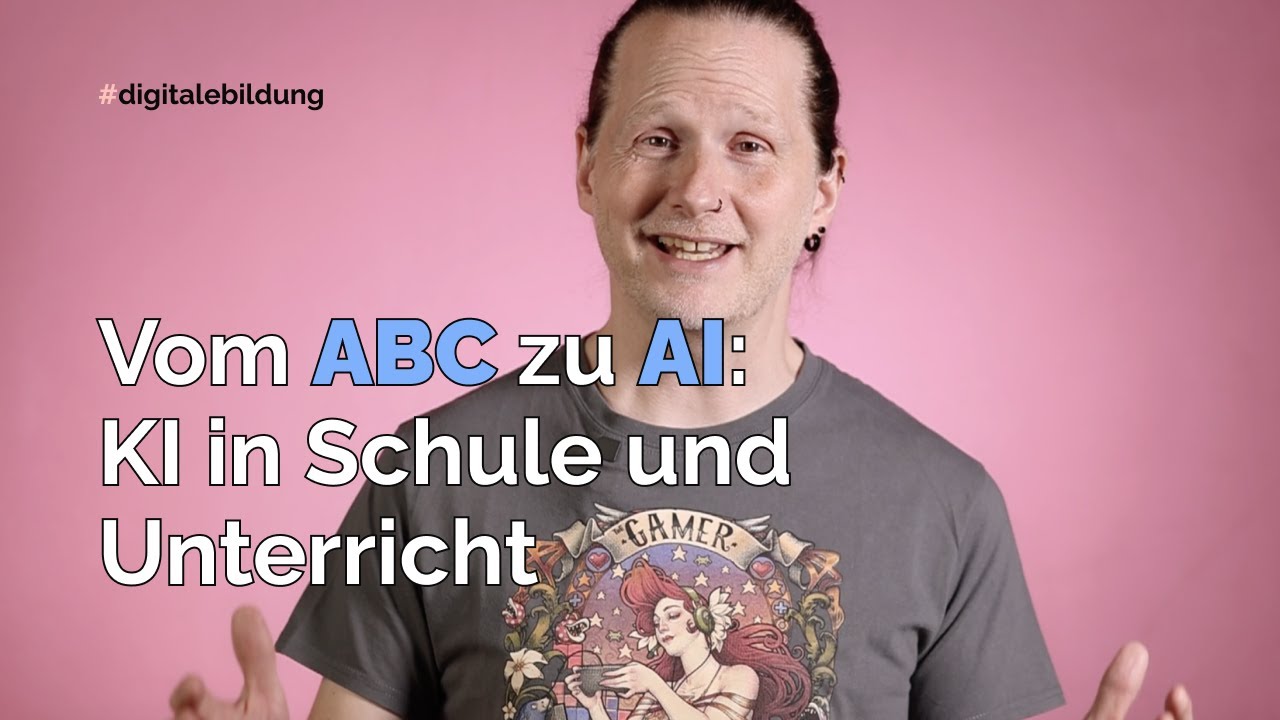
Vom ABC zu AI: KI in Schule und Unterricht
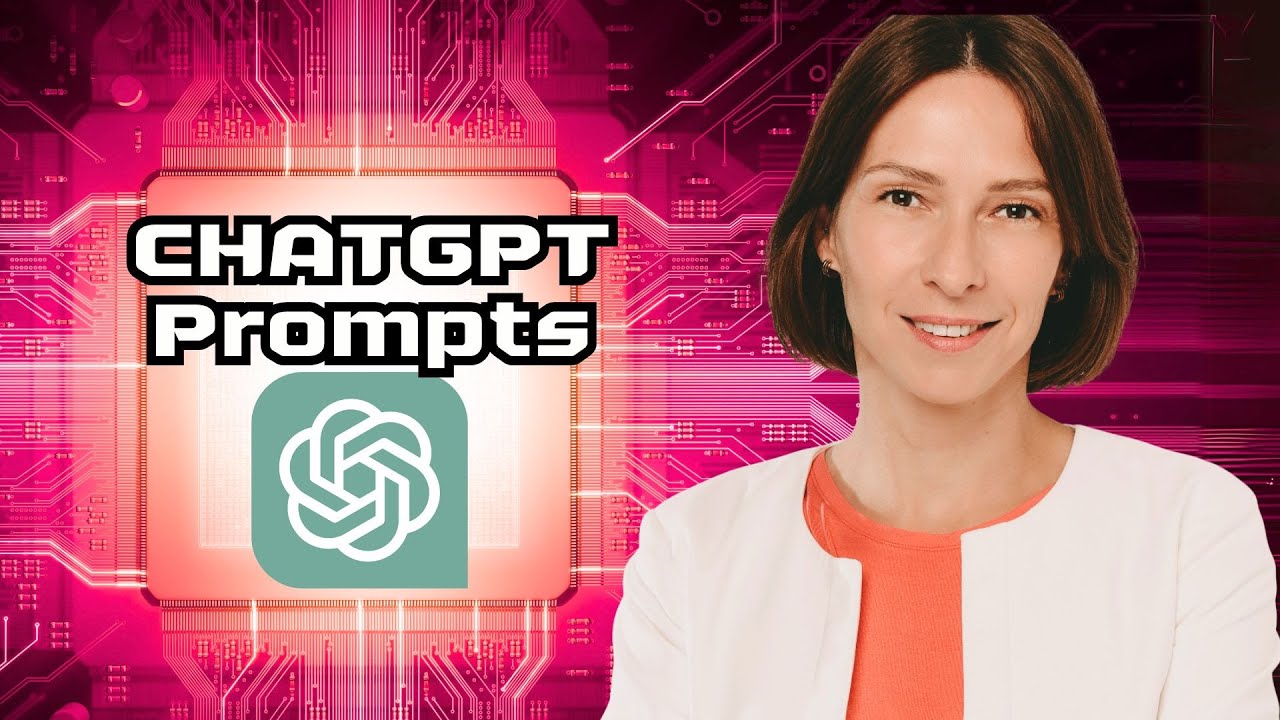
Wie erstelle ich gute Prompts für ChatGPT & Co?
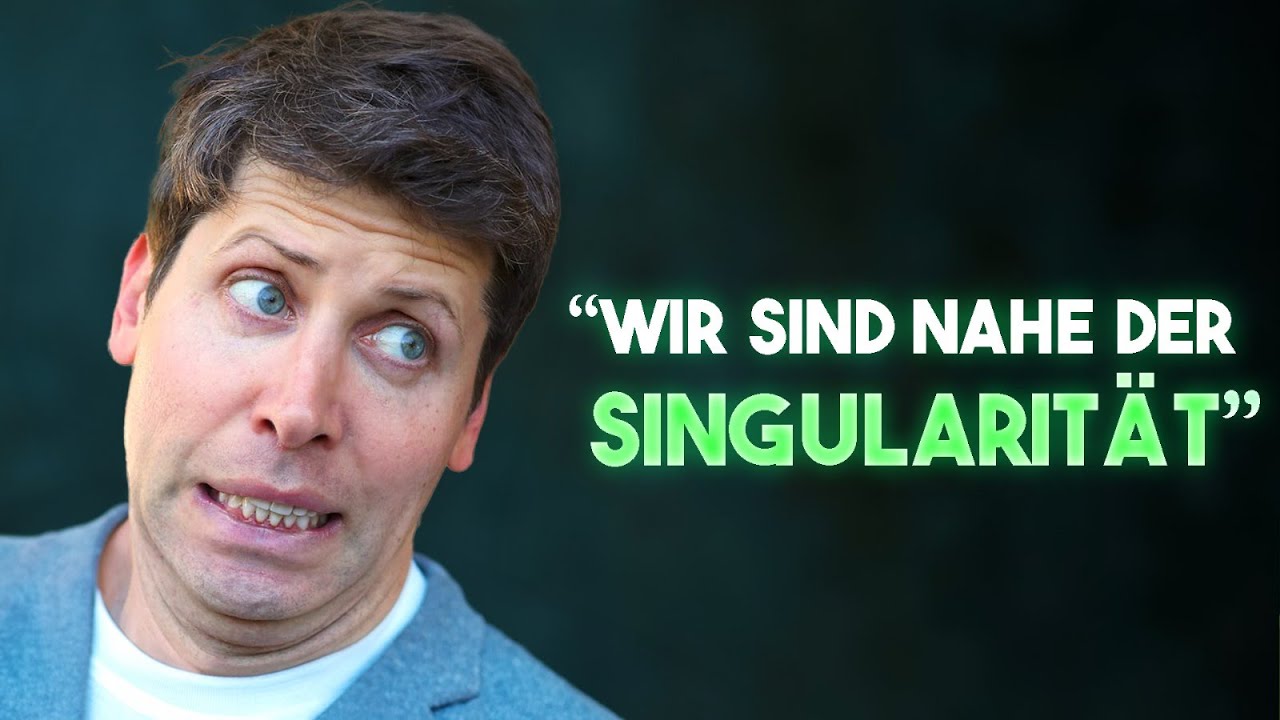
OpenAI Könnte die Singularität Ausgelöst Haben
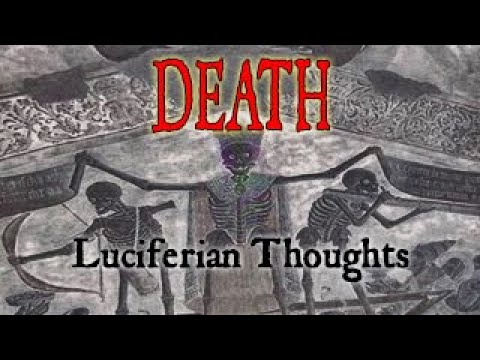
Luciferian Thoughts on Death that Will Make You Reevaluate Life
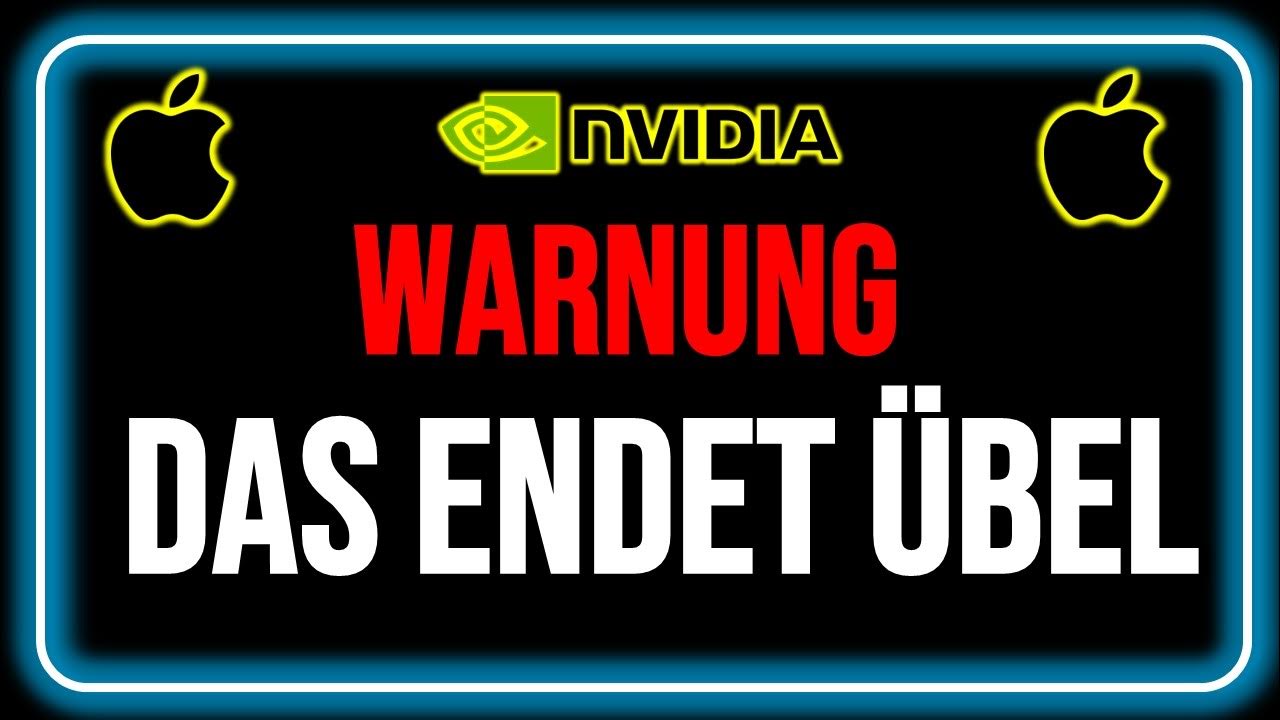
Warnzeichen auf dem Aktienmarkt....
5.0 / 5 (0 votes)