Chi-squared Test
Summary
TLDRIn this informative podcast, Mr. Andersen demystifies the Chi-squared test, a statistical tool used to determine if observed data variations are due to chance or underlying variables. He introduces the concept with a coin flip experiment, explains the null hypothesis, and illustrates how to calculate the Chi-squared value and interpret critical values for decision-making. The podcast also covers degrees of freedom and provides examples with coins and dice to demonstrate the test's application in real-world scenarios, encouraging viewers to apply Chi-squared in their own experiments.
Takeaways
- 📚 The Chi-squared test is a statistical method used to determine if observed data differs from expected data due to chance or a specific variable.
- 🔍 It was developed by Carl Pearson in the early 1900s and is widely used in fields like AP Biology and other sciences.
- 📝 The formula for the Chi-squared test involves summing the differences between observed and expected values, squared and divided by the expected values.
- 🎲 The test uses the concept of 'observed data' and 'expected values', with the latter being theoretical values calculated before an experiment.
- 🤔 The Chi-squared test helps to answer questions like whether a coin flip's outcome is due to chance or a biased coin.
- ❓ The Null Hypothesis in Chi-squared testing states that there is no statistical significant difference between observed and expected frequencies.
- 🔢 'Degrees of freedom' in the test is calculated by the number of outcomes minus one, which affects the critical value used for comparison.
- 📉 Critical values are used to decide whether to accept or reject the Null Hypothesis, with common values determined for specific confidence levels like 0.05.
- 🧩 The test involves comparing the calculated Chi-squared value to a critical value from a Chi-squared distribution chart.
- 🎯 If the Chi-squared value exceeds the critical value, the Null Hypothesis is rejected, indicating a significant difference between observed and expected data.
- 📈 The Chi-squared test can be applied to various scenarios, such as coin flips, dice rolls, or animal behavior studies, to determine if outcomes are due to chance or other factors.
Q & A
What is the primary purpose of the Chi-squared test?
-The primary purpose of the Chi-squared test is to determine if the variation in collected data is due to chance or if it's due to one of the variables being tested.
Who developed the Chi-squared test and when was it developed?
-The Chi-squared test was developed by Karl Pearson in the early part of the 1900s.
What are the two main components used in the Chi-squared test to compare data?
-The two main components used in the Chi-squared test are the observed data (O) and the expected values (E).
What is the Null Hypothesis in the context of the Chi-squared test?
-The Null Hypothesis in the context of the Chi-squared test is the assumption that there is no statistical significant difference between the observed values and the expected frequencies.
What are degrees of freedom in a Chi-squared test?
-Degrees of freedom in a Chi-squared test refer to the number of values in the final calculation that are free to vary. It is calculated by subtracting one from the number of outcomes being compared.
What is a critical value in the context of the Chi-squared test?
-A critical value in the context of the Chi-squared test is the value that determines whether to accept or reject the Null Hypothesis. If the calculated Chi-squared value exceeds the critical value, the Null Hypothesis is rejected.
What does it mean to accept or reject the Null Hypothesis in a Chi-squared test?
-Accepting the Null Hypothesis means that the observed data does not show a significant difference from the expected data, suggesting no effect from the variable being tested. Rejecting the Null Hypothesis indicates that there is a significant difference, suggesting the variable has an effect.
How is the Chi-squared value calculated in a test?
-The Chi-squared value is calculated by taking the difference between the observed and expected values, squaring the result, and then dividing by the expected value. This is done for each category and the results are summed to get the total Chi-squared value.
What is the significance of the 0.05 value in the context of critical values?
-The 0.05 value, or alpha level, is a threshold used in hypothesis testing to determine statistical significance. It represents a 95% confidence level that the results are not due to chance.
Can you provide an example from the script where the Chi-squared test was applied to a coin flip experiment?
-In the script, Mr. Andersen applied the Chi-squared test to a coin flip experiment where he flipped a coin 100 times and observed 62 heads and 38 tails. The expected values were 50 heads and 50 tails. The calculated Chi-squared value was compared to the critical value to determine if the coin flip results were due to chance or not.
How does the Chi-squared test help in determining if a coin or dice is biased?
-The Chi-squared test helps in determining if a coin or dice is biased by comparing the observed frequencies of outcomes (like heads/tails or dice numbers) to the expected frequencies based on probability. If the calculated Chi-squared value exceeds the critical value, it suggests the coin or dice may be biased.
Outlines
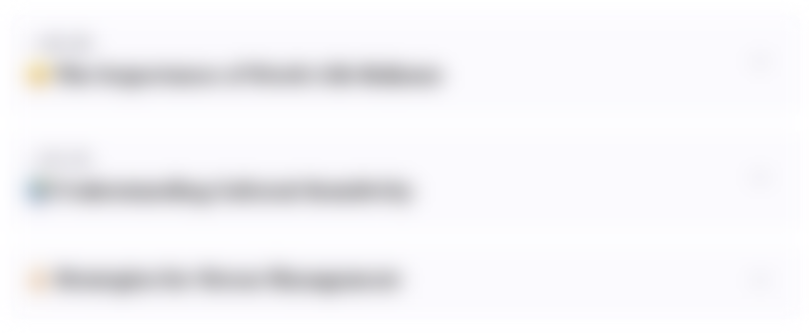
Этот раздел доступен только подписчикам платных тарифов. Пожалуйста, перейдите на платный тариф для доступа.
Перейти на платный тарифMindmap
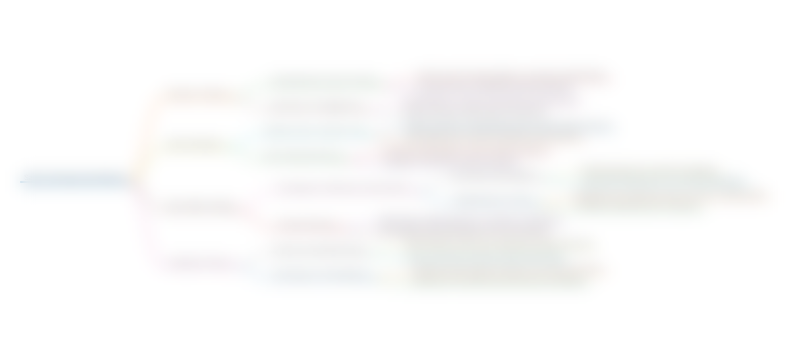
Этот раздел доступен только подписчикам платных тарифов. Пожалуйста, перейдите на платный тариф для доступа.
Перейти на платный тарифKeywords
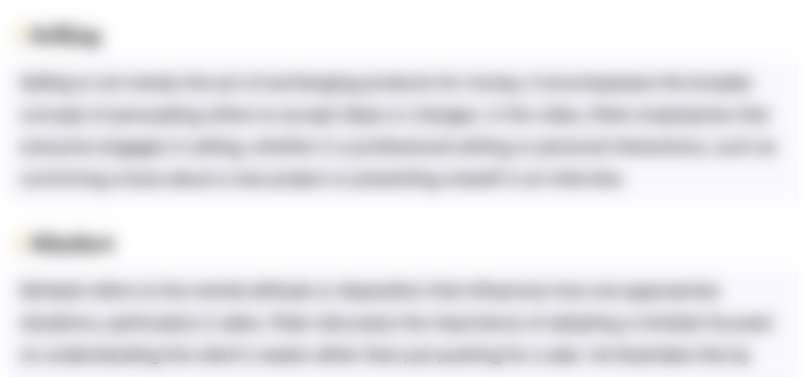
Этот раздел доступен только подписчикам платных тарифов. Пожалуйста, перейдите на платный тариф для доступа.
Перейти на платный тарифHighlights
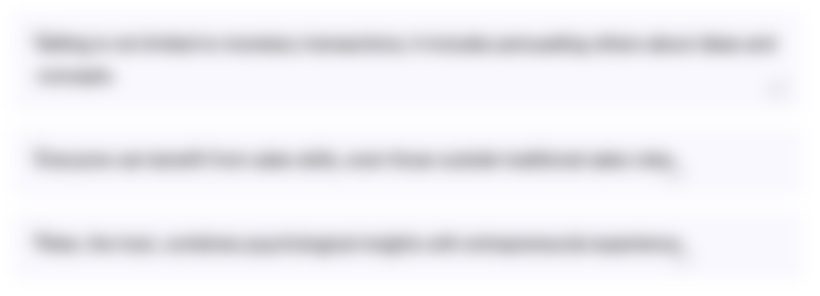
Этот раздел доступен только подписчикам платных тарифов. Пожалуйста, перейдите на платный тариф для доступа.
Перейти на платный тарифTranscripts
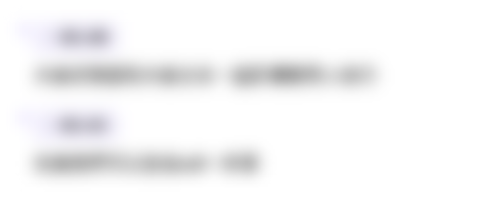
Этот раздел доступен только подписчикам платных тарифов. Пожалуйста, перейдите на платный тариф для доступа.
Перейти на платный тарифПосмотреть больше похожих видео

Chi Square in Genetics & Examples (AP Biology)
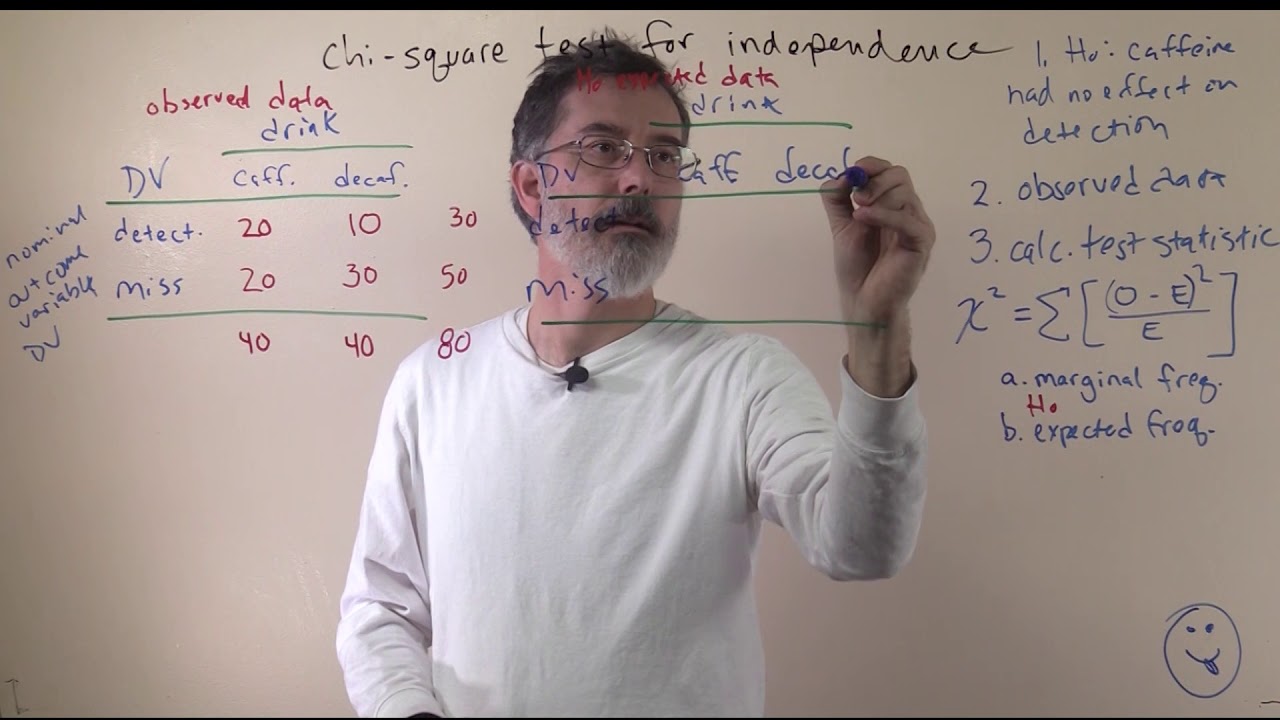
video 14.2. chi-square test of independence

Goodness of Fit
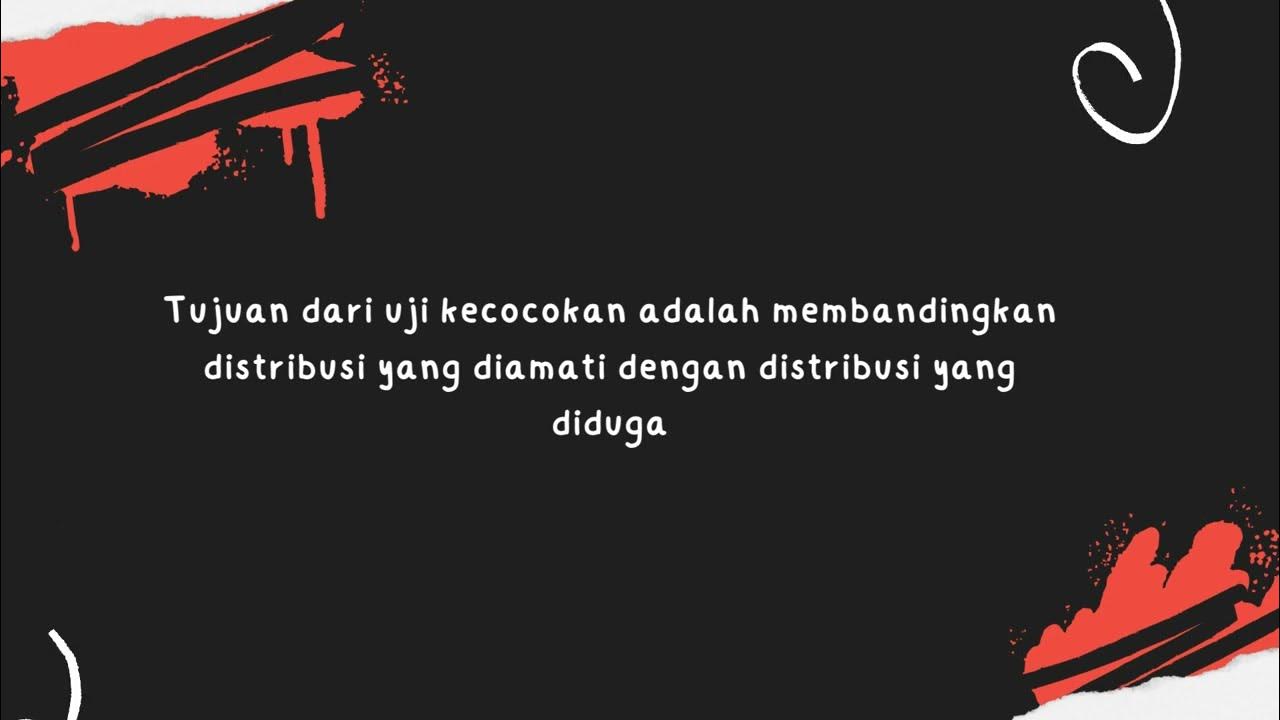
*M* Uji Kecocokan: Frekuensi yang Diduga Sama dan yang Tidak Sama dengan Microsoft Excel dan SPSS
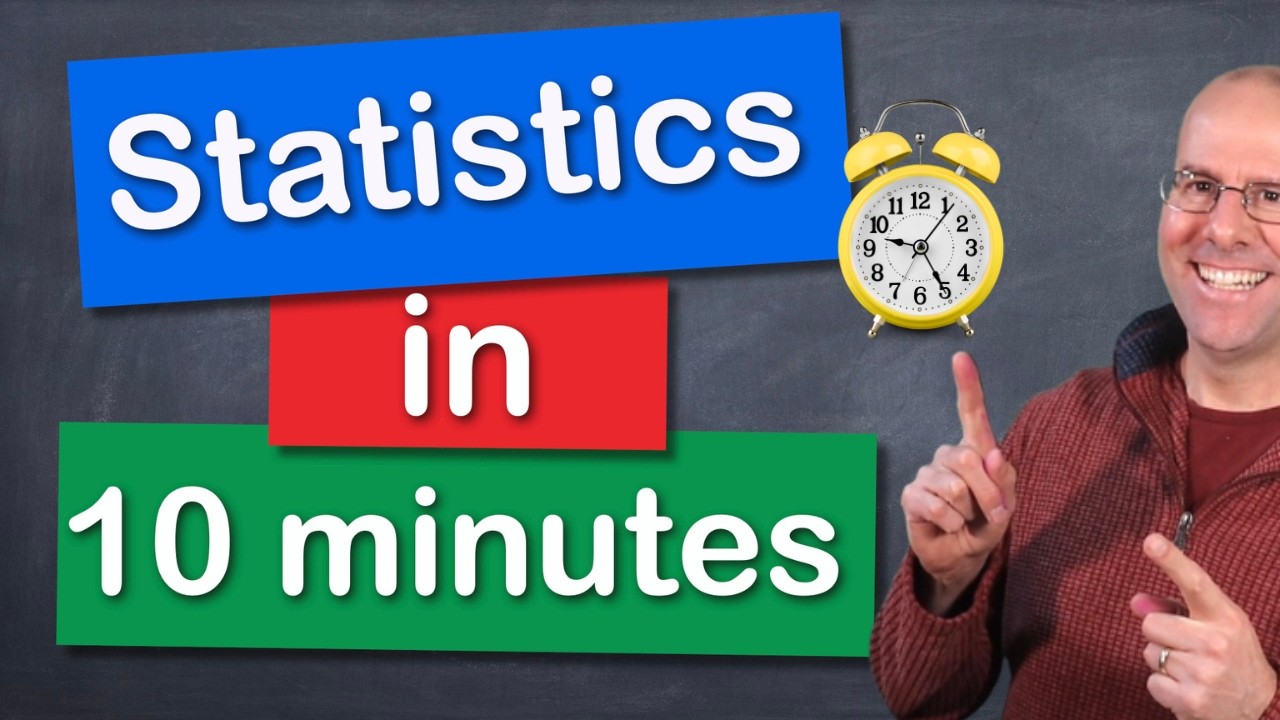
Statistics in 10 minutes. Hypothesis testing, the p value, t-test, chi squared, ANOVA and more
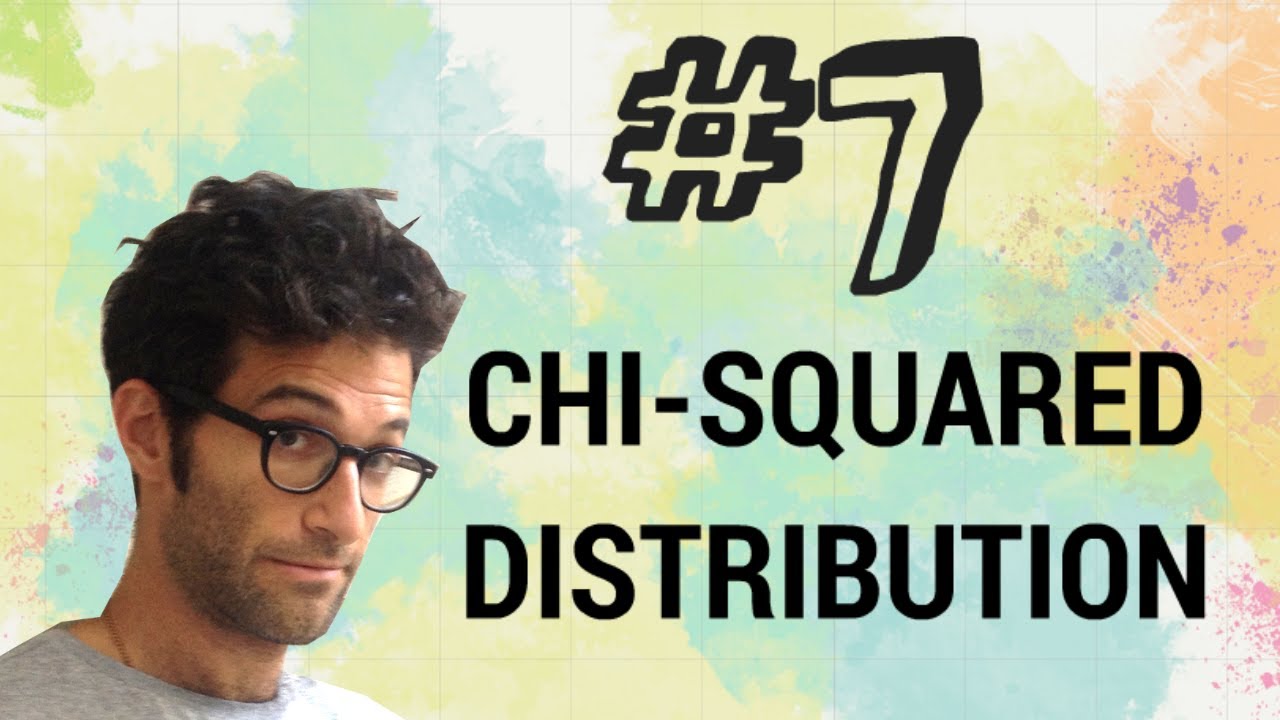
What is the Chi-Squared distribution? Extensive video!
5.0 / 5 (0 votes)