Walmart Data Science Case Study Mock Interview: Underpricing Algorithm
Summary
TLDRIn this insightful discussion, the focus is on diagnosing why an e-commerce pricing algorithm is undervaluing certain consumer products. The conversation delves into the factors influencing product pricing, such as demand, availability, and logistics costs. It explores potential causes for the price drop, including changes in consumer behavior, external factors like new laws, and improvements in logistics. The dialogue also touches on the importance of demand patterns, search algorithm changes, and the impact of UI updates on product visibility. The conversation concludes with considerations for manual intervention in the pricing algorithm based on analysis outcomes.
Takeaways
- 🔍 The script discusses diagnosing an issue where an e-commerce pricing algorithm is underpricing certain products, focusing on factors like availability, demand, and logistics cost.
- 📈 Demand information for pricing is sourced from internal data, such as historical sales and user engagement metrics like clicks and searches on the website.
- 📉 The algorithm's pricing discrepancy was not due to seasonal or time-based factors, but rather a recent and significant drop in price over the past few months.
- 📱 The product in question is an electronic consumer good, which may have different pricing dynamics compared to other types of products.
- 📊 A significant 50% price drop was observed compared to five months prior, indicating a potential issue in the pricing model that needs investigation.
- 🔍 The discussion suggests that a change in customer behavior, negative reviews, or new regulations could affect demand and thus pricing.
- 🛍️ The company's logistics improvements, such as new distribution centers or partnerships, could reduce costs and contribute to lower product pricing.
- 📝 The script highlights the importance of monitoring search query results and product visibility on the website to assess changes in demand and algorithm behavior.
- 📉 If demand remains constant but price drops, it might indicate external factors not captured by the algorithm, such as changes in consumer reviews or regulations.
- 🛑 Manual intervention in the algorithm may be necessary if there's a fundamental change in logistics or supply chain not reflected in the pricing model.
- 🔧 The script suggests potential areas for improvement, such as retraining the model with more emphasis on logistic costs or incorporating feedback loops to adjust pricing based on historical data.
Q & A
What is the primary role of a data scientist in the context of the e-commerce pricing problem discussed in the script?
-The primary role of a data scientist in this context is to diagnose why the algorithm is underpricing certain products, considering factors such as product availability, demand, and logistics costs.
How does the demand information for products get collected in the scenario described in the script?
-The demand information is collected from the company's internal data, which includes historical sales data and user engagement metrics like clicks and searches on the website.
What are some potential reasons for a sudden drop in the price of a product as mentioned in the script?
-Potential reasons include a significant change in demand, external factors not captured by the algorithm such as consumer reviews or new laws, or changes in the company's logistics infrastructure that reduce costs.
How can the time aspect of the price drop provide insights into the underlying issue?
-The timing of the price drop can indicate whether the issue is related to seasonal changes, macroeconomic factors, or specific events such as product bans or negative reviews.
What type of product was discussed in the script as experiencing the pricing issue?
-The product discussed was an electronic consumer good, which could be a phone or similar device.
How might changes in the product's demand pattern affect the pricing algorithm?
-If the demand pattern remains constant but the price drops, it suggests that the algorithm may not be accounting for external factors that have reduced the product's appeal to consumers.
What could be some external factors affecting the product's demand aside from the product itself?
-External factors could include negative consumer reviews, changes in legislation that affect the product's viability, or shifts in consumer behavior due to new competitor products.
How can the company determine if changes in logistics costs are responsible for the price drop?
-By analyzing whether there have been improvements in logistics infrastructure, such as new distribution centers or partnerships with freight forwarding companies, which could reduce shipping costs.
What actions might a data scientist take if they find that the pricing algorithm is not accurately reflecting increased logistics costs?
-The data scientist might retrain the model to give more weight to logistics costs or implement a feedback loop in the model to better account for changes in these costs over time.
What is the decision-making process a data scientist might follow when deciding whether to adjust the pricing algorithm manually?
-The data scientist would consider whether the current pricing is beneficial to customers, whether demand is consistent, and if the company is still gaining profits. Manual intervention would be considered if there's a fundamental change in the supply chain or logistics that the algorithm isn't accounting for.
What did the speaker in the script suggest could be missing from the algorithm's consideration that might be causing the underpricing?
-The speaker suggested that the algorithm might not be considering external factors like consumer reviews, end-user experience, or changes in laws that could affect the product's appeal and, consequently, its pricing.
Outlines
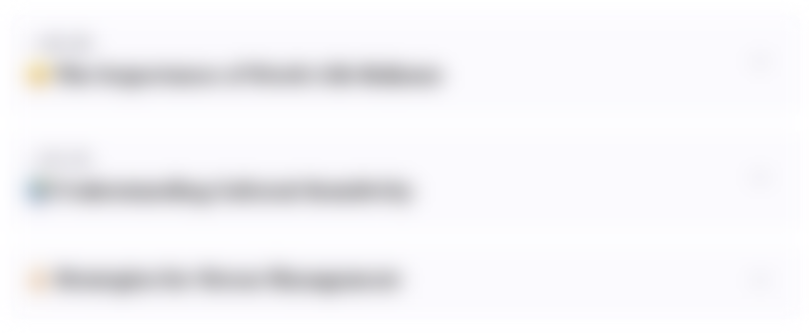
このセクションは有料ユーザー限定です。 アクセスするには、アップグレードをお願いします。
今すぐアップグレードMindmap
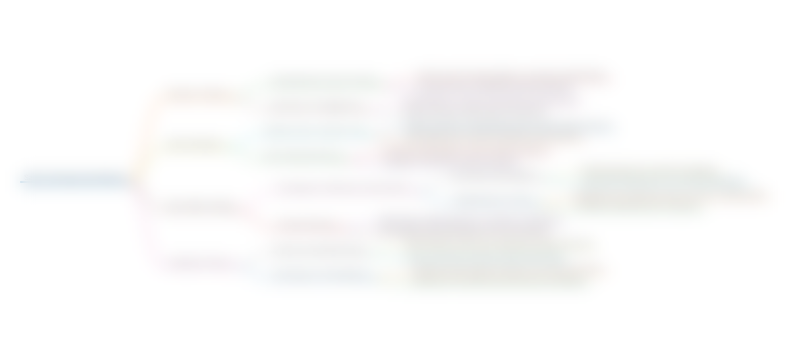
このセクションは有料ユーザー限定です。 アクセスするには、アップグレードをお願いします。
今すぐアップグレードKeywords
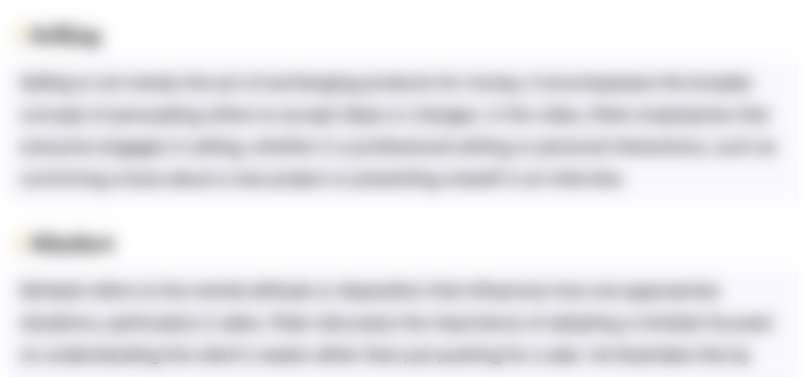
このセクションは有料ユーザー限定です。 アクセスするには、アップグレードをお願いします。
今すぐアップグレードHighlights
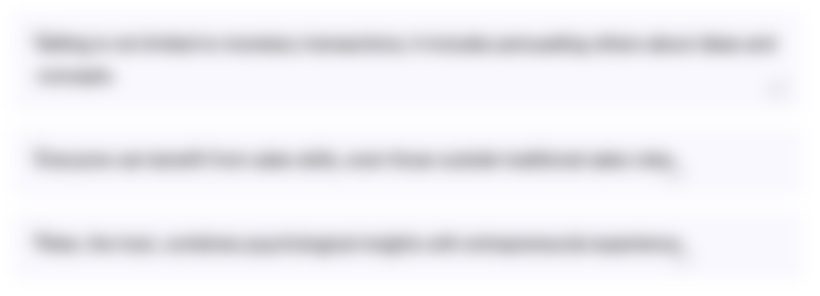
このセクションは有料ユーザー限定です。 アクセスするには、アップグレードをお願いします。
今すぐアップグレードTranscripts
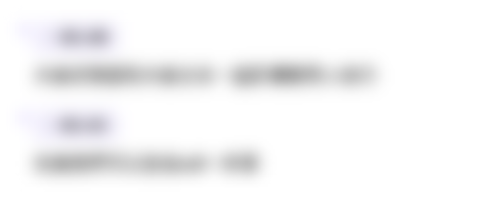
このセクションは有料ユーザー限定です。 アクセスするには、アップグレードをお願いします。
今すぐアップグレード関連動画をさらに表示

Penetapan Harga Produk Berbasis Digital by Melya Yosita (E Marketing)
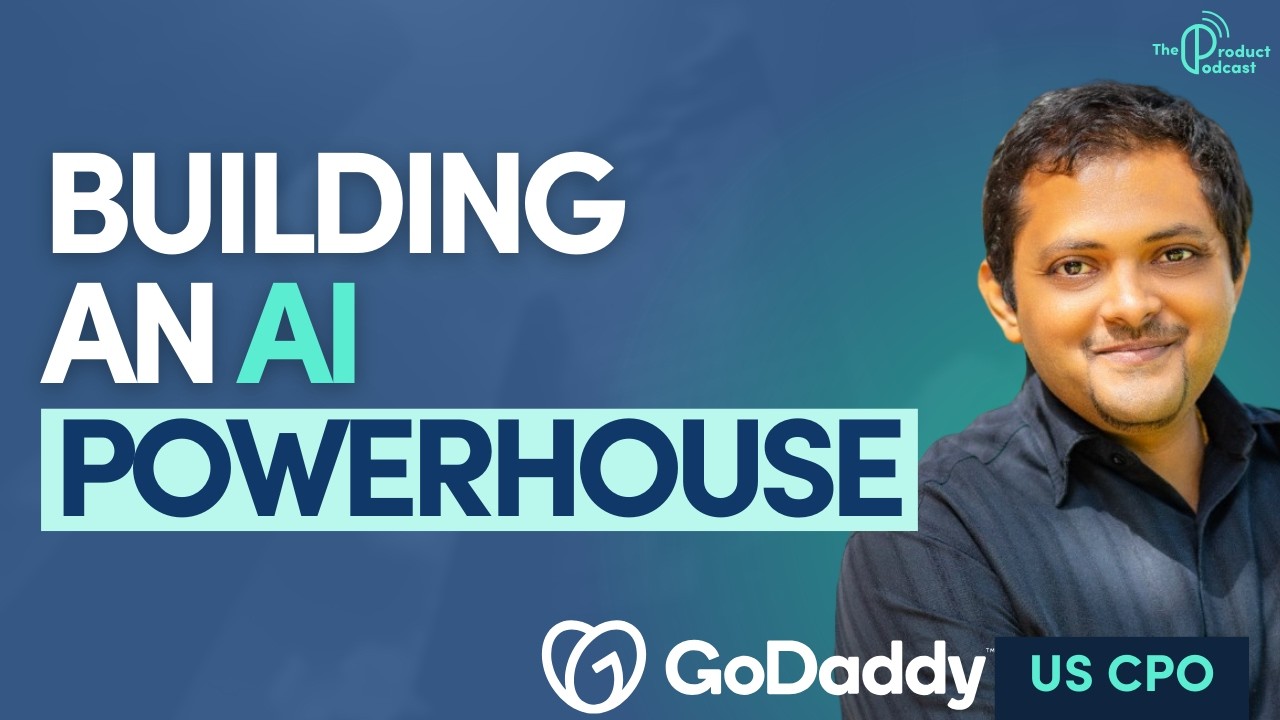
27 y/o Business to AI Powerhouse | Vinod Suresh, US CPO, GoDaddy
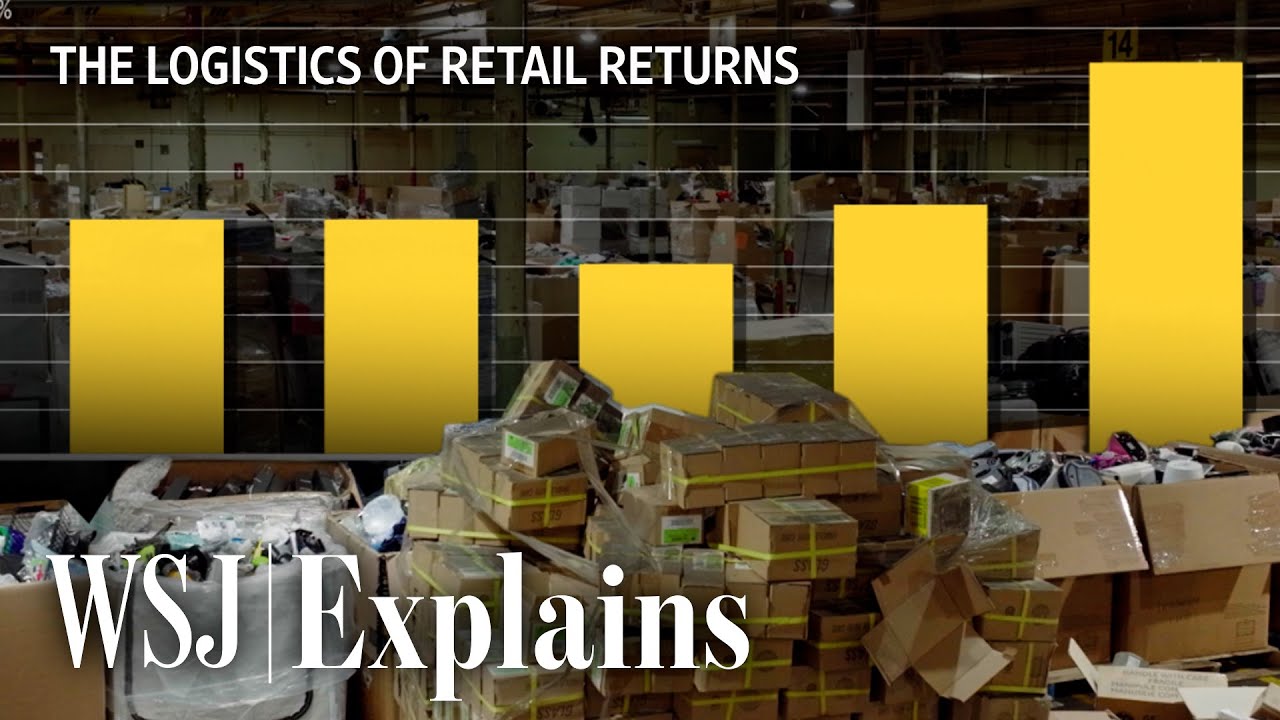
Returns Are the Retail Industry’s Quietly Mounting Logistics Problem | WSJ

Commercial Refrigeration Expected To Recover In H2FY25 & EMP Biz Expected To Grow At 20%: Blue Star
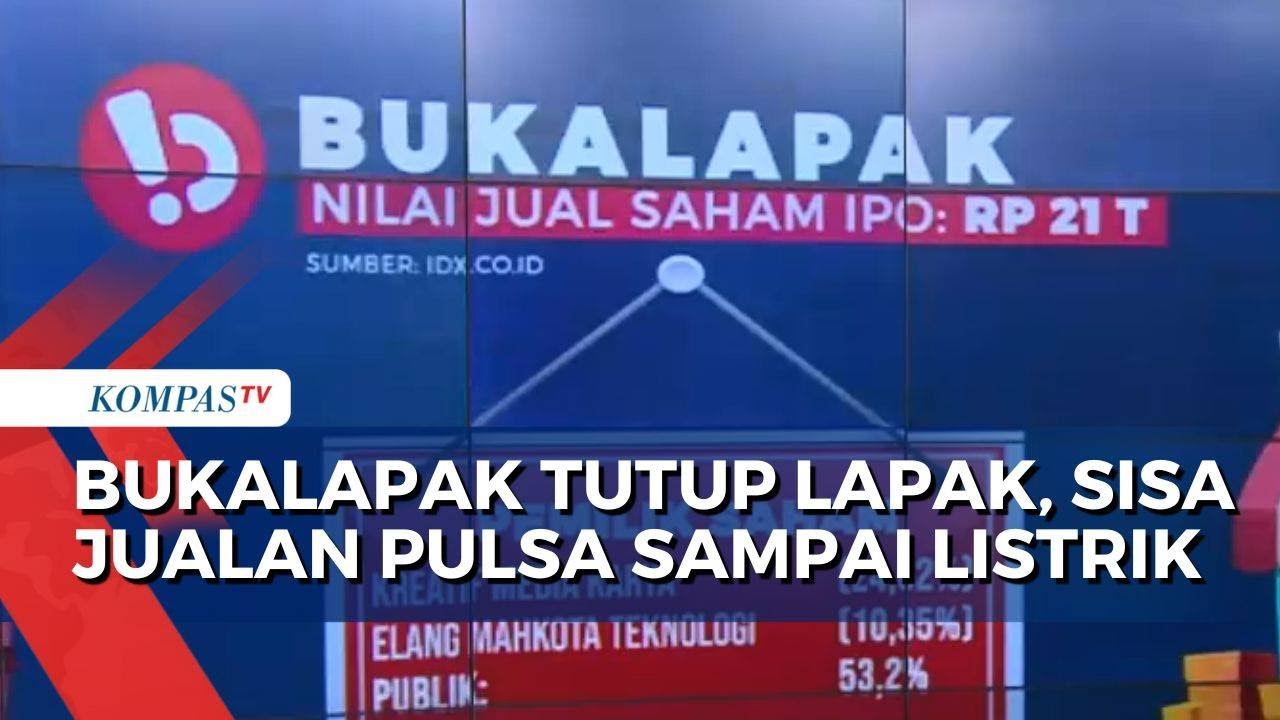
Bukalapak, Unicorn Pertama yang Tutup Lapak
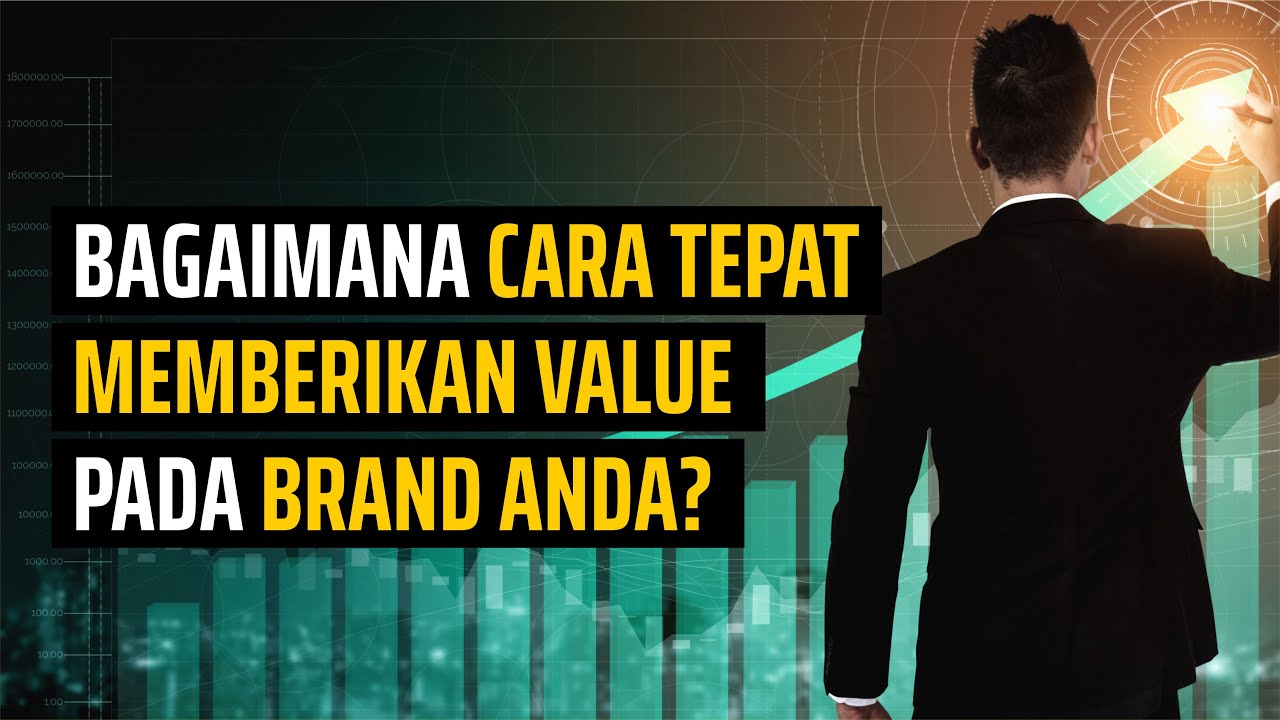
🛑 Bagaimana cara tepat memberikan Value pada Brand Anda? | Brand Strategi UKM Dodi Zulkifli
5.0 / 5 (0 votes)