一口气了解英伟达,芯片新王凭什么是他?
Summary
TLDRThe video traces Nvidia and CEO Jensen Huang's rise from graphics card company to AI powerhouse. It examines how Nvidia leveraged its GPUs for parallel computing, invested heavily in R&D, and developed CUDA software to make GPUs programmable. This enabled breakthroughs in AI. However, Nvidia owes much success to luck from the crypto mining boom. Now Nvidia dominates training of AI models. With AI's vast potential, tech giants aim to compete, but Nvidia's enduring monopoly may be hard to challenge.
Takeaways
- 😀 Nvidia started off by specializing in graphics processing chips and established itself as a leader in the gaming industry
- 🤓 Jensen Huang had the vision to make Nvidia's GPUs more versatile through the CUDA software platform
- 🔥 Cryptocurrency mining created huge demand for Nvidia's GPUs from 2018-2021
- 🧠 Nvidia GPUs proved enormously effective for AI model training after 2012, cementing Nvidia's dominance
- 💰 Nvidia's data center segment now generates over half its revenues due to AI demand
- 👷♂️ Chipmakers need to iterate very fast - Nvidia stays ahead through huge R&D investments
- 🏭 The chip industry has high barriers to entry and tends to concentrate power
- 🚘 Nvidia is expanding into self-driving car computers and other growth areas
- 🗺️ Major tech firms see Nvidia as a strategic asset and are wary of its dominance
- ❓ It remains to be seen if Nvidia can maintain its edge as the AI market evolves
Q & A
When was Nvidia founded and by whom?
-Nvidia was founded in 1993 by Jensen Huang, Chris Malachowsky, and Curtis Priem.
What breakthrough product established Nvidia as an industry leader in 1999?
-The GeForce256 graphics card established Nvidia as an industry leader in 1999. It was the first 'GPU' that was designed specifically for graphics processing.
How did the rise of Bitcoin mining benefit Nvidia?
-The rise in Bitcoin mining brought huge demand for mining hardware. Since graphics cards are very efficient for crypto mining, this greatly benefited Nvidia's graphics card business.
What is the significance of Nvidia's CUDA platform?
-CUDA made Nvidia GPUs programmable and expanded their use for general purpose computing beyond just graphics processing. This laid the foundation for using GPUs in AI.
Why did the AlexNet deep learning model represent an important breakthrough?
-The AlexNet model that won the 2012 ImageNet competition utilized GPUs for its neural network training. This showed the potential of GPU accelerated deep learning.
How has Nvidia tailored its GPU products for AI workloads?
-Nvidia has optimized its GPU architecture, created software frameworks like CUDA, and launched specialized products like the A100 GPU specifically for accelerating AI workloads.
What are some key moves Nvidia has made to expand beyond GPUs?
-Major moves include the acquisitions of Mellanox and ARM, investments in automotive tech, and initiatives in areas like Metaverse.
Why is it difficult to build an enduring competitive advantage in the chip industry?
-The rapid pace of innovation means advantages don't last long before new technologies emerge. Nvidia tries to stay ahead with heavy R&D spending and continuous new product introduction.
How are tech giants responding to Nvidia’s dominance in AI chips?
-Large tech firms like Google, Meta, and Microsoft are conducting their own AI chip research and development to reduce reliance on Nvidia GPUs.
What does Nvidia’s future success depend on in the face of rising competition?
-Nvidia's future hinges on sustaining technological leadership in GPUs via continuous R&D innovation, expanding into new high-growth markets like AI and automotive, and leveraging vertical integration synergies across hardware and software stack.
Outlines
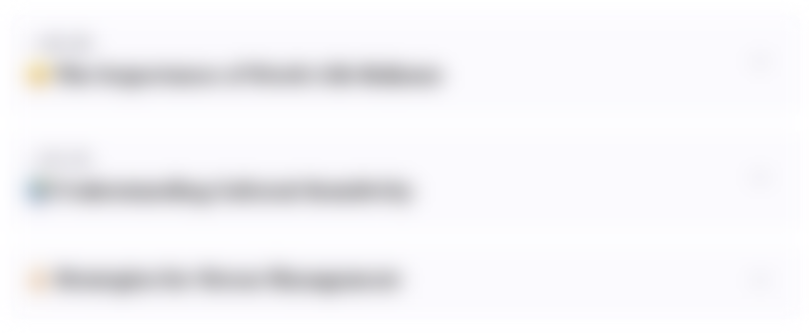
このセクションは有料ユーザー限定です。 アクセスするには、アップグレードをお願いします。
今すぐアップグレードMindmap
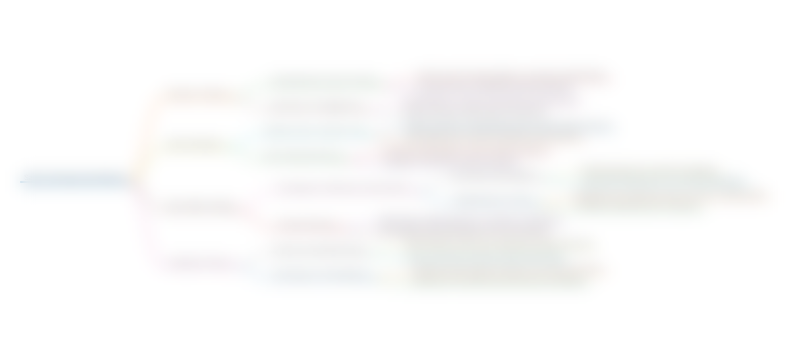
このセクションは有料ユーザー限定です。 アクセスするには、アップグレードをお願いします。
今すぐアップグレードKeywords
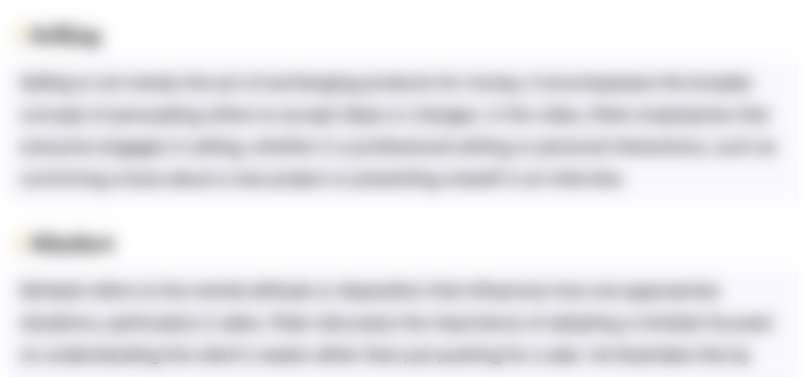
このセクションは有料ユーザー限定です。 アクセスするには、アップグレードをお願いします。
今すぐアップグレードHighlights
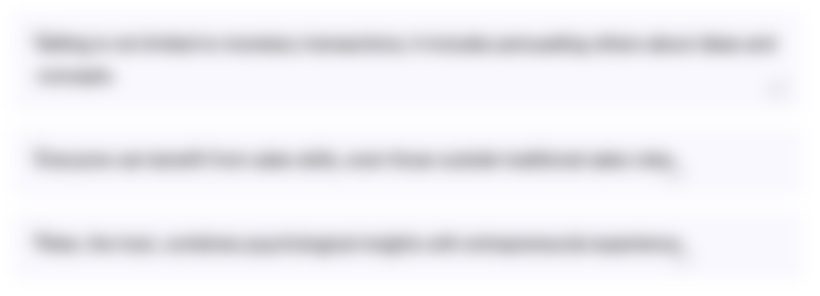
このセクションは有料ユーザー限定です。 アクセスするには、アップグレードをお願いします。
今すぐアップグレードTranscripts
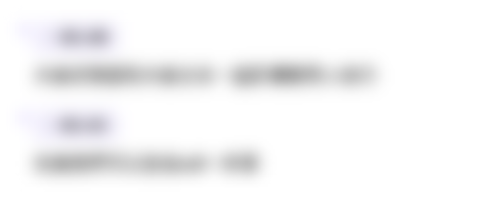
このセクションは有料ユーザー限定です。 アクセスするには、アップグレードをお願いします。
今すぐアップグレード関連動画をさらに表示
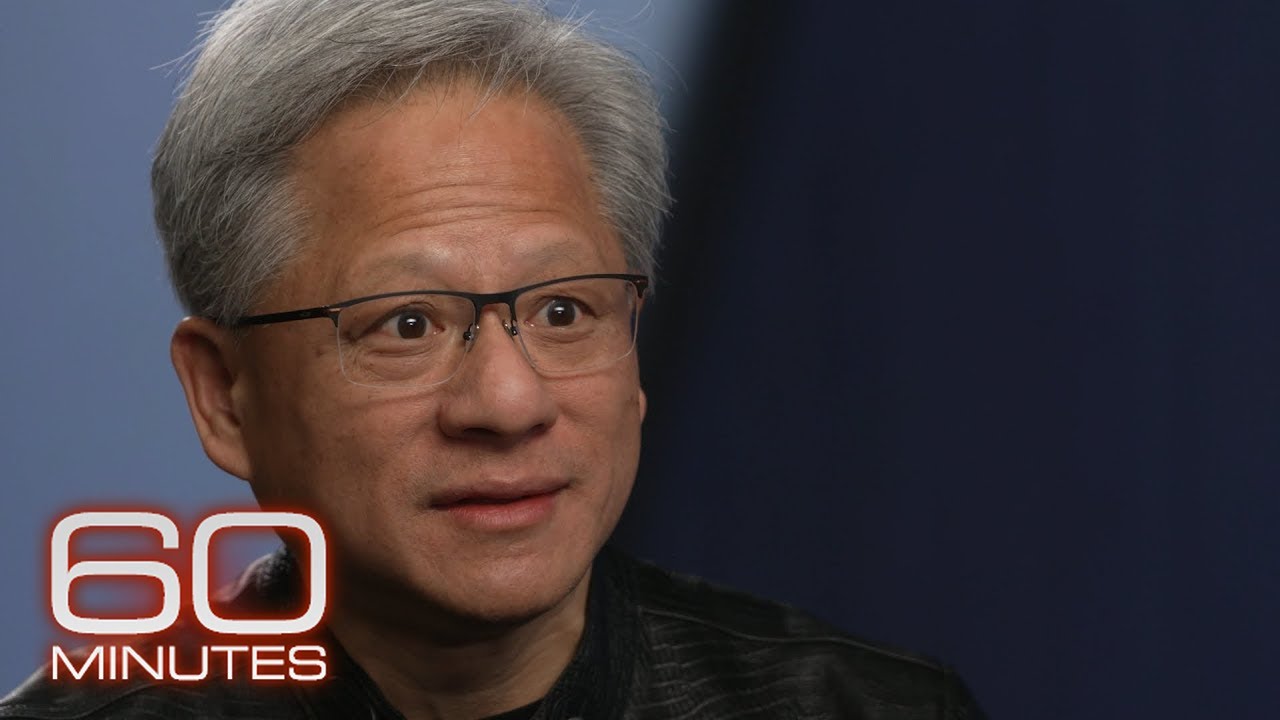
Nvidia CEO Jensen Huang and the $2 trillion company powering today's AI | 60 Minutes

The Rise of Jensen Huang, NVIDIA’s Co-founder, President & CEO
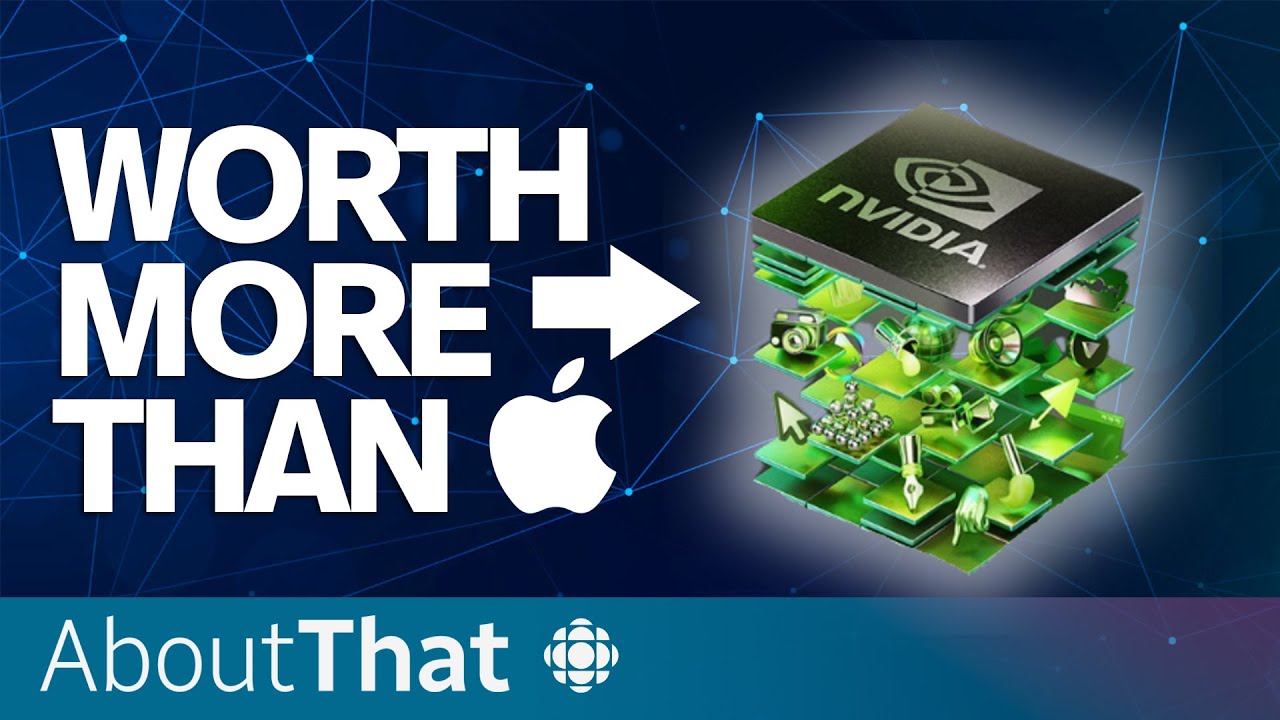
Nvidia's meteoric rise to $3 trillion | About That

The Growth of Nvidia
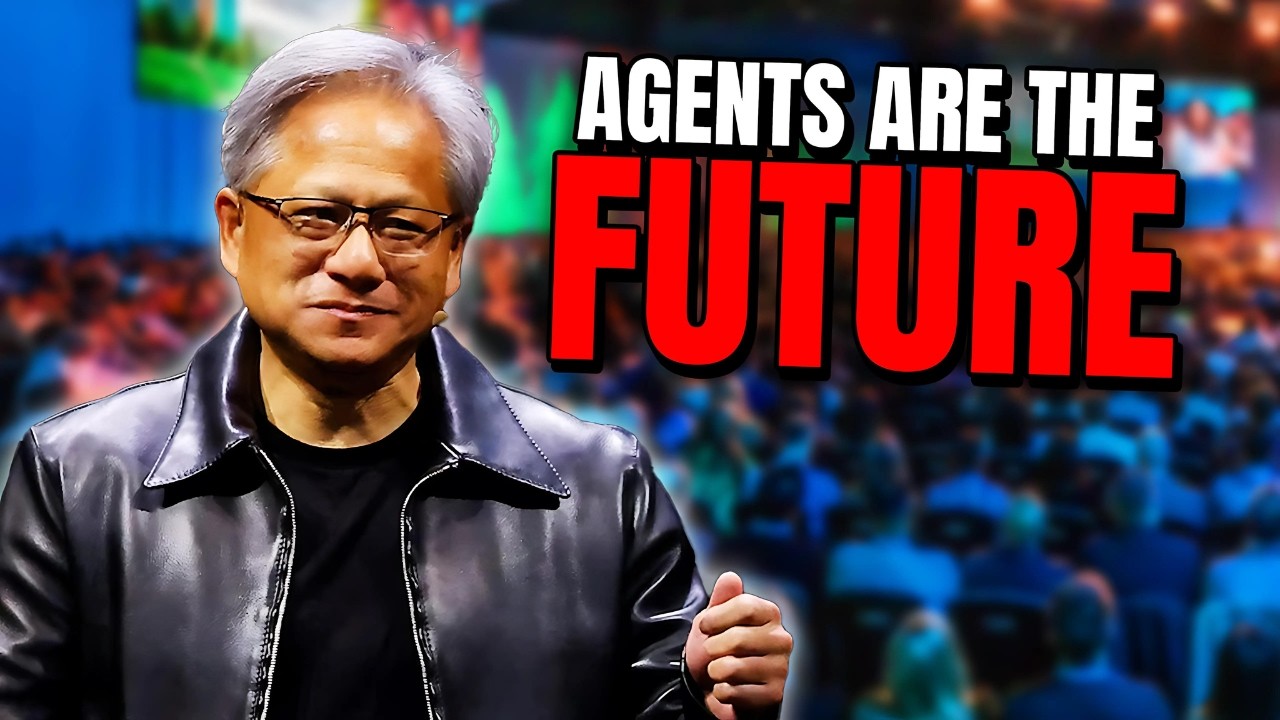
NVIDIA CEO on Agents Being the Future of AI
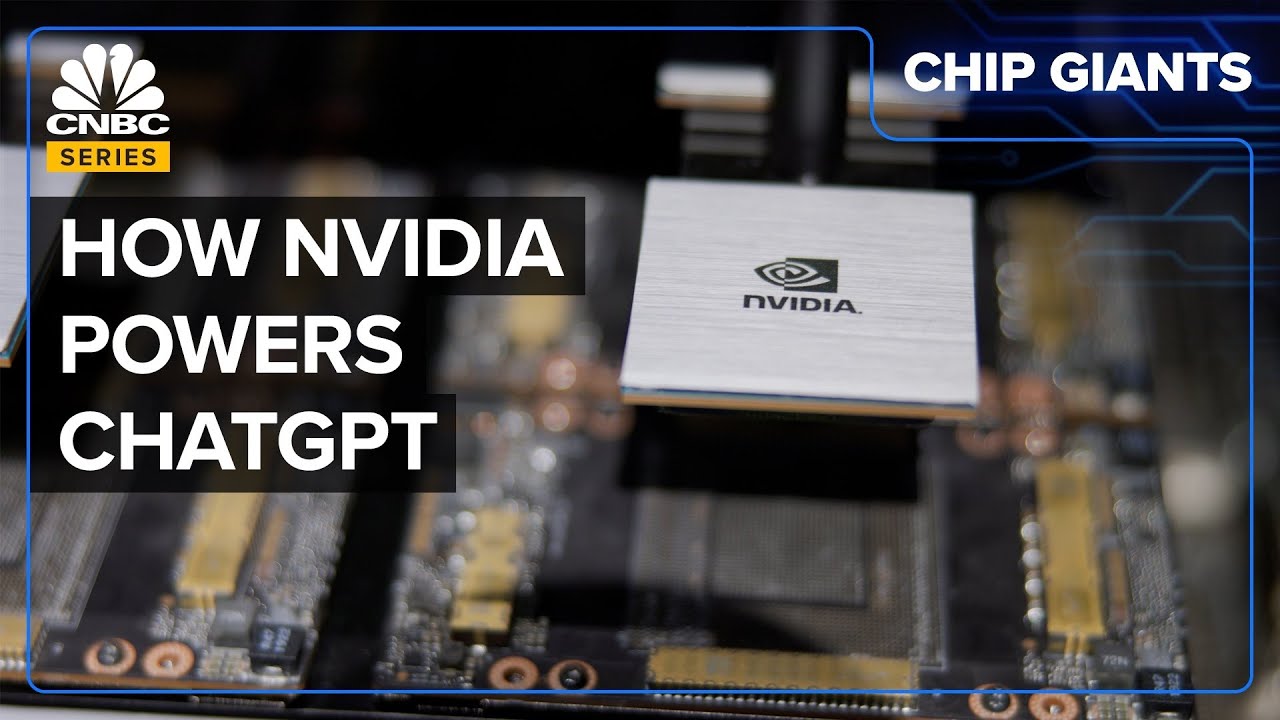
How Nvidia Grew From Gaming To A.I. Giant, Now Powering ChatGPT
5.0 / 5 (0 votes)