Lec-7: kNN Classification with Real Life Example | Movie Imdb Example | Supervised Learning
Summary
TLDRThe video script is an educational tutorial on machine learning concepts, specifically focusing on classification and regression. It uses examples like predicting movie genres based on IMDb ratings and duration to explain the process of data collection, calculation of Euclidean distance, and the nearest neighbor algorithm. The script emphasizes the importance of understanding the concepts behind machine learning and provides a practical example of classifying a movie into genres like action or comedy.
Takeaways
- 📚 The video is aimed at explaining machine learning concepts, focusing on numerical examples to help students understand better.
- 🔢 It emphasizes the importance of taking notes and understanding the mathematical calculations involved in machine learning concepts.
- 💡 The video discusses classification and regression as two fundamental types of predictions in machine learning, with examples provided.
- 📈 The Nearest Neighbor algorithm is introduced as a simple yet important algorithm in machine learning, using distance calculations to make predictions.
- 📝 The process of data collection is highlighted as the first step in making predictions, using real-time data from movies as an example.
- 🎬 IMDB ratings, duration, and genre are used as data points to demonstrate how to apply machine learning concepts to real-world data.
- 📊 The Euclidean distance formula is explained in detail to calculate the distance between data points, which is crucial for the Nearest Neighbor algorithm.
- 📐 The concept of 'k' in k-Nearest Neighbors is explored, discussing how to determine the number of neighbors to consider for a prediction.
- 🗳️ The video uses a voting system to finalize predictions, illustrating how majority voting can be used in classification tasks.
- 📝 It stresses the importance of understanding the underlying math behind machine learning to effectively implement algorithms.
- 🔑 The video concludes by summarizing the key steps in applying machine learning, from data collection to prediction and validation.
Q & A
What is the main topic of the video script?
-The main topic of the video script is explaining the concept of classification and regression in machine learning, using examples like predicting movie genres based on IMDb ratings and duration.
What does the speaker suggest students should do while learning machine learning concepts?
-The speaker suggests that students should take notes while learning machine learning concepts, emphasizing the importance of understanding the mathematical calculations involved.
What is the example used to explain the concept of classification?
-The example used to explain classification is predicting whether an item, like an apple, is a fruit or a vegetable based on certain features.
What is the purpose of calculating the Euclidean distance in the context of the video?
-The purpose of calculating the Euclidean distance is to find the closest point or class in a dataset, which helps in making predictions in classification tasks.
How does the speaker describe the process of data collection for the machine learning example?
-The speaker describes the process of data collection by mentioning the use of real-time data from movies released in 2023, including IMDb ratings, duration, and genres.
What is the significance of IMDb ratings and duration in the given machine learning example?
-IMDb ratings and duration are significant as they are features used to classify or predict the genre of a movie in the machine learning example.
What is the method used to calculate the distance between data points in the script?
-The method used to calculate the distance between data points is the Euclidean distance, calculated using the formula √((x2 - x1)² + (y2 - y1)²).
What is the final step in the classification process after calculating distances?
-The final step is to select the nearest neighbor or class based on the minimum distance calculated, which helps in making the prediction for the classification.
How does the speaker address the challenge of selecting the 'k' value in k-nearest neighbors?
-The speaker suggests taking the best value as low as possible, such as 3 or 4, but not taking 5 due to the limited data available, as it might not always provide the exact solution.
What is the importance of majority voting in the context of the k-nearest neighbors algorithm?
-Majority voting is important as it helps in deciding the final class or genre of the movie by considering the most frequent class among the k-nearest neighbors.
Outlines
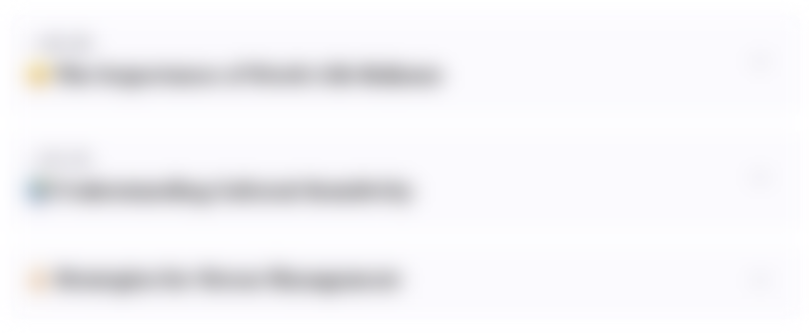
このセクションは有料ユーザー限定です。 アクセスするには、アップグレードをお願いします。
今すぐアップグレードMindmap
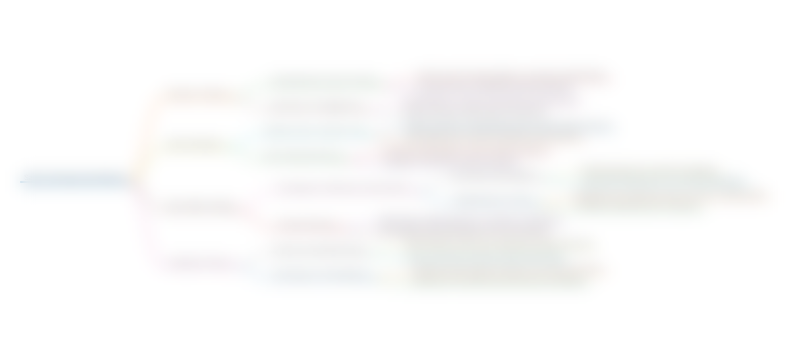
このセクションは有料ユーザー限定です。 アクセスするには、アップグレードをお願いします。
今すぐアップグレードKeywords
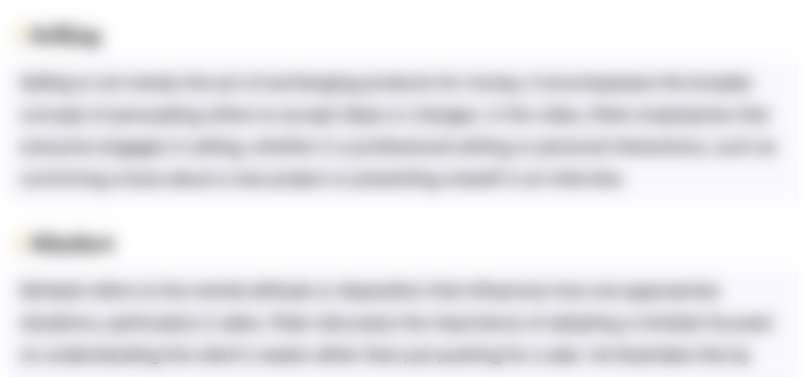
このセクションは有料ユーザー限定です。 アクセスするには、アップグレードをお願いします。
今すぐアップグレードHighlights
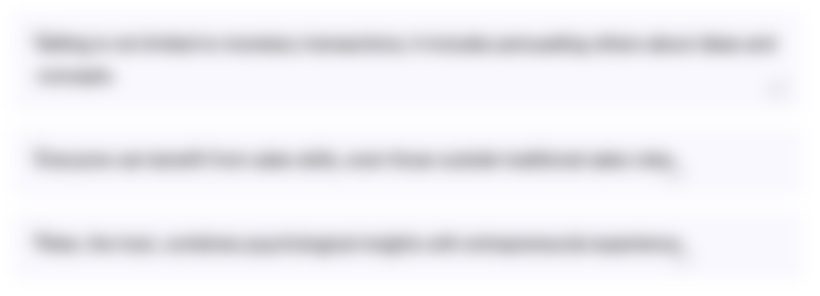
このセクションは有料ユーザー限定です。 アクセスするには、アップグレードをお願いします。
今すぐアップグレードTranscripts
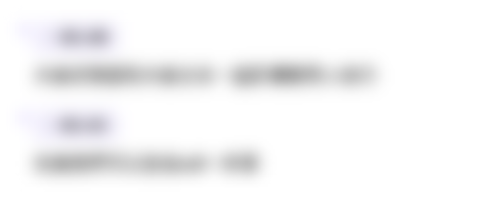
このセクションは有料ユーザー限定です。 アクセスするには、アップグレードをお願いします。
今すぐアップグレード関連動画をさらに表示
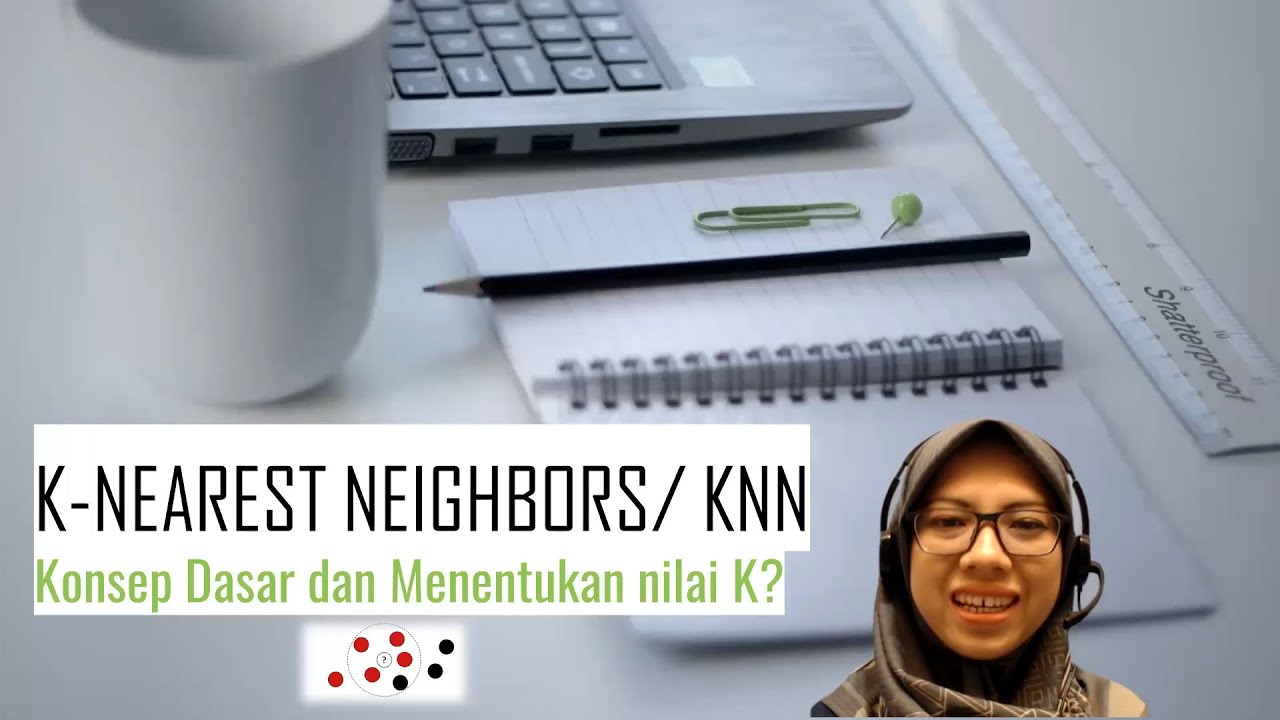
Konsep Algoritma KNN (K-Nearest Neigbors) dan Tips Menentukan Nilai K
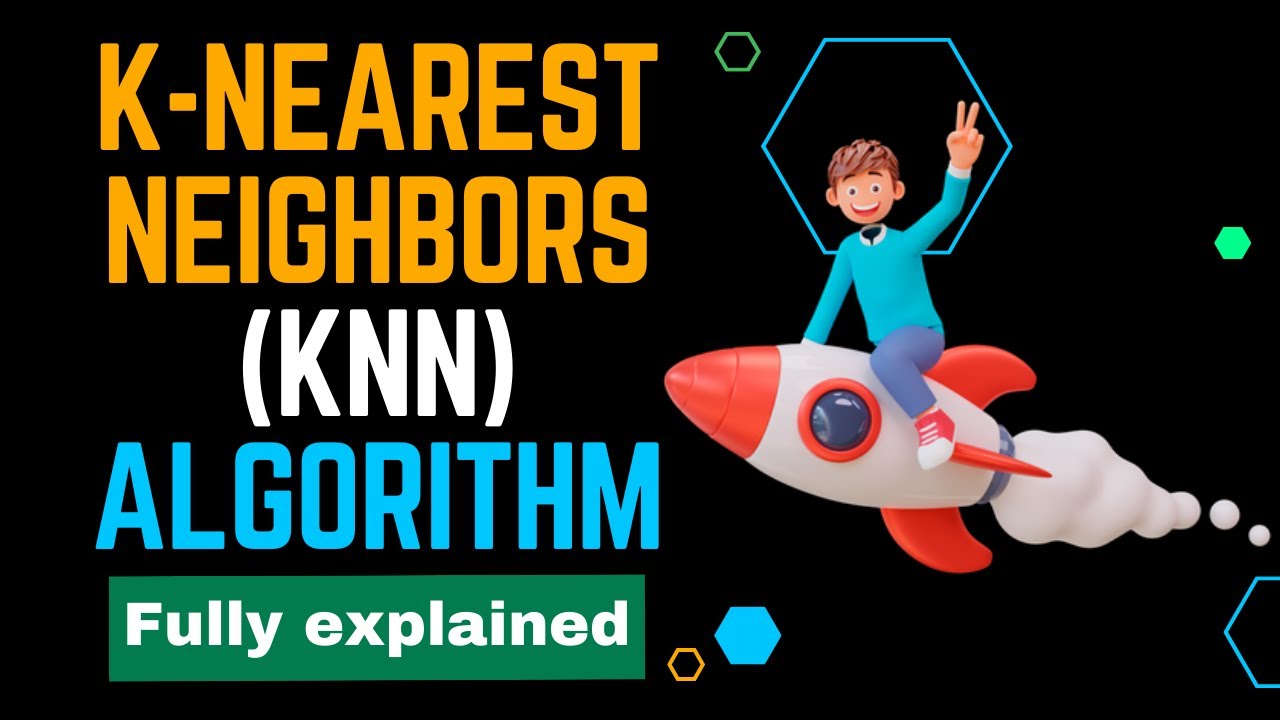
Lecture 3.4 | KNN Algorithm In Machine Learning | K Nearest Neighbor | Classification | #mlt #knn

Python Exercise on kNN and PCA

Lec-3: Introduction to Regression with Real Life Examples
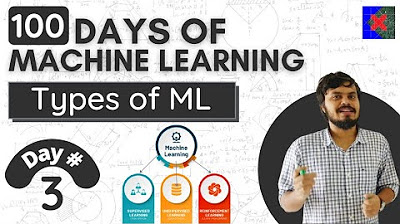
Types of Machine Learning for Beginners | Types of Machine learning in Hindi | Types of ML in Depth
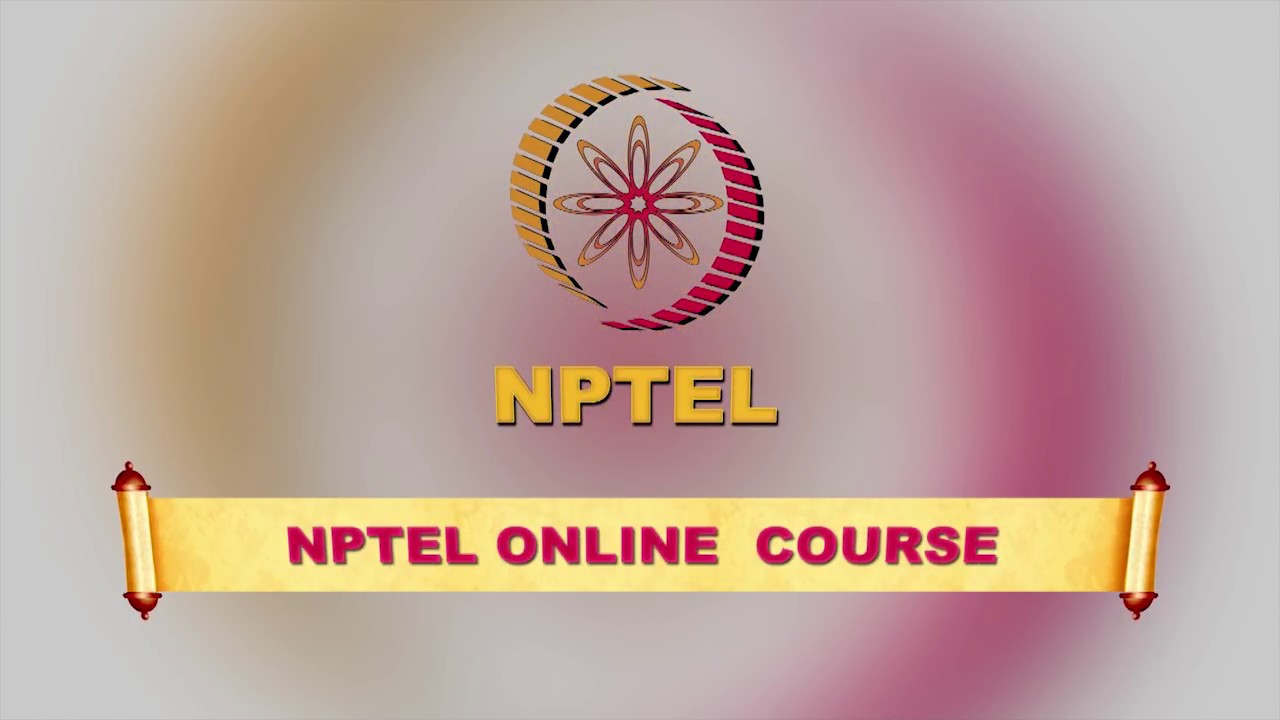
Deep Learning(CS7015): Lec 2.5 Perceptron Learning Algorithm
5.0 / 5 (0 votes)