StatQuest: One or Two Tailed P-Values
Summary
TLDRIn this Stat Quest, brought to you by the Genetics Department at UNC Chapel Hill, the focus is on one-tailed vs. two-tailed tests in statistics. Using a cancer treatment trial as an example, the video explains that a one-tailed test shows a p-value of 0.03, indicating significance, while a two-tailed test shows 0.06, not significant. The importance of choosing the test type before the experiment to avoid false positives is emphasized. The video concludes that a two-tailed test is generally preferable to get a complete picture of the data.
Takeaways
- 📊 One-tailed tests focus on whether one treatment is better than another, while two-tailed tests evaluate if there is any difference, either better or worse.
- 🔬 In clinical trials, it's crucial to decide on the type of test and p-value threshold before conducting the experiment to avoid bias.
- 📉 A one-tailed test may produce a smaller p-value because it doesn't account for the possibility of the new treatment being worse.
- ⚖️ Good statistical practice involves using a two-tailed test to get a complete picture of the treatment's effectiveness.
- 🛑 Waiting to choose the type of test until after seeing the data can lead to p-hacking and false positives.
- 🔍 A false positive occurs when a test indicates a significant result when there is none, which can be influenced by the type of test used.
- 📈 Using a one-tailed test inappropriately can increase the probability of reporting false positives from 5% to 8%.
- 💡 Always aim to understand both sides of the data story, not just the side that seems favorable.
- 🔎 Some statistical tests do not offer a choice between one-tailed and two-tailed, but when given a choice, the two-tailed test is generally preferable.
- 🎉 The end message is clear: for accurate and unbiased results, always use a two-tailed test when you have the option.
Q & A
What is the primary topic discussed in the transcript?
-The primary topic discussed is the difference between one-tailed and two-tailed tests in statistical analysis and when to use each type.
Why might someone choose to use a one-tailed test?
-A one-tailed test is used when the hypothesis specifically predicts that one treatment or condition will have a better outcome than another.
What p-value did the one-tailed test yield in the example given?
-The one-tailed test yielded a p-value of 0.03.
What p-value did the two-tailed test yield in the example given?
-The two-tailed test yielded a p-value of 0.06.
Why is the two-tailed p-value generally preferred over the one-tailed p-value?
-The two-tailed p-value is preferred because it tests whether the new treatment is better, worse, or not significantly different from the standard treatment, providing a more comprehensive analysis.
What is the risk associated with deciding the type of test after seeing the data?
-Deciding the type of test after seeing the data can lead to 'p-hacking,' increasing the probability of reporting false positives.
What is a false positive in the context of statistical testing?
-A false positive occurs when the test indicates a significant effect when there is none, typically expected to happen 5% of the time in a two-tailed test.
How did the false positive rate change when switching to a one-tailed test after seeing favorable data?
-The false positive rate increased from 5% to 8% when switching to a one-tailed test after seeing favorable data.
What lesson does the transcript emphasize regarding the choice of statistical tests?
-The transcript emphasizes the importance of deciding which statistical test to use before conducting the experiment to avoid p-hacking and ensure accurate results.
What is the recommended practice when you have a choice between a one-tailed and a two-tailed test?
-The recommended practice is to always choose a two-tailed test to fully understand both sides of the data and avoid biased results.
Outlines
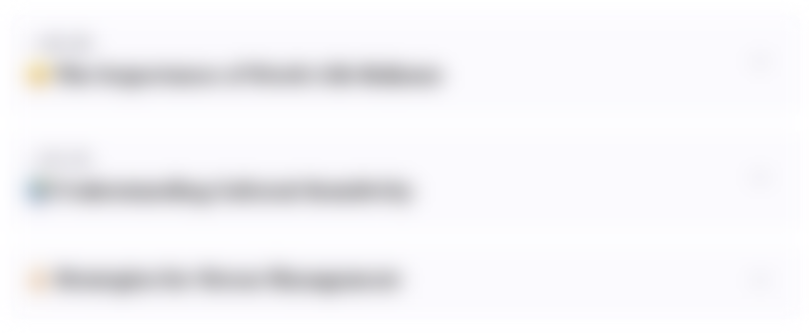
このセクションは有料ユーザー限定です。 アクセスするには、アップグレードをお願いします。
今すぐアップグレードMindmap
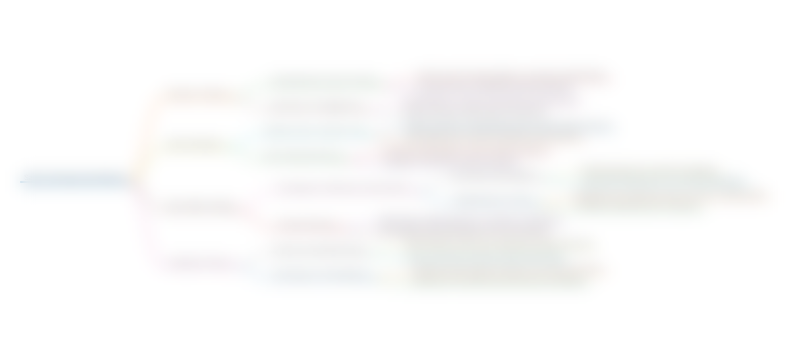
このセクションは有料ユーザー限定です。 アクセスするには、アップグレードをお願いします。
今すぐアップグレードKeywords
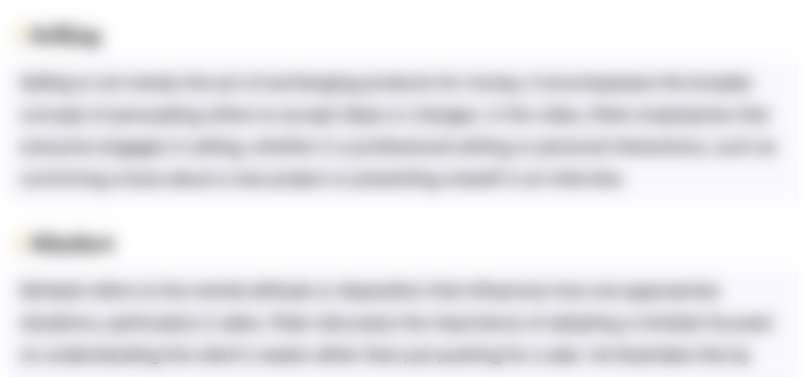
このセクションは有料ユーザー限定です。 アクセスするには、アップグレードをお願いします。
今すぐアップグレードHighlights
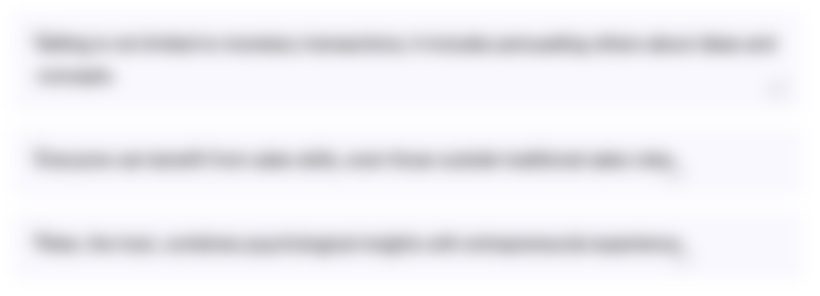
このセクションは有料ユーザー限定です。 アクセスするには、アップグレードをお願いします。
今すぐアップグレードTranscripts
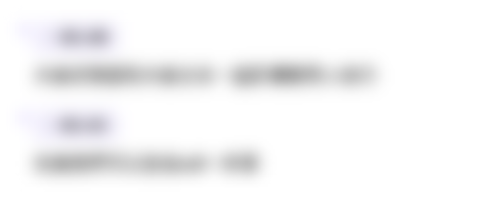
このセクションは有料ユーザー限定です。 アクセスするには、アップグレードをお願いします。
今すぐアップグレード5.0 / 5 (0 votes)