Ilya Sutskever: "Sequence to sequence learning with neural networks: what a decade"
Summary
TLDRIn this insightful talk, the speaker reflects on the evolution of AI, from early auto-regressive models to the sophisticated large language models (LLMs) of today. Highlighting key milestones and challenges over the past decade, the speaker discusses the future of AI, including its potential for reasoning, autonomy, and self-correction. Speculation about the emergence of superintelligent systems and the ethical implications of AI’s role in society offers a thought-provoking look at what lies ahead. With a mix of technical insights and philosophical reflection, the talk explores AI’s unpredictable yet transformative trajectory.
Takeaways
- 😀 The speaker thanks the organizers, co-authors, and collaborators for their work, reflecting on the progress made over the past decade.
- 😀 The presentation includes a 'before and after' comparison, showcasing the speaker’s evolution and the development of AI in the last 10 years.
- 😀 The core concept from 10 years ago revolved around large neural networks with 10 layers, focusing on the idea that these networks could replicate tasks that humans perform in a fraction of a second.
- 😀 A key point from the speaker's early work was the idea of an autoregressive model trained on text to predict the next token, capturing a distribution over sequences.
- 😀 The LSTM model, described as a simpler, rotated version of a ResNet, preceded the Transformer architecture and was a critical step in AI’s development.
- 😀 The early emphasis on pipelining with GPUs for parallelization led to a 3.5x speedup, although this approach was later deemed inefficient.
- 😀 The scaling hypothesis was introduced, suggesting that large datasets combined with large neural networks would lead to guaranteed success.
- 😀 The speaker acknowledges the ongoing advances in AI, particularly in pre-training, where large neural networks trained on vast datasets became a key driver of progress.
- 😀 The speaker predicts the eventual end of pre-training due to the limits of data, likening it to the depletion of fossil fuels, suggesting that AI will have to adapt to the data we currently have.
- 😀 There is growing speculation about future AI directions, including the emergence of agents, synthetic data, and inference-time compute, with a focus on understanding reasoning in AI systems.
- 😀 The speaker discusses the long-term vision of superintelligence, speculating that future AI systems will possess agentic qualities, be capable of reasoning, and exhibit unpredictability, raising important ethical and existential questions.
Q & A
What is the main theme of the speaker’s talk?
-The main theme of the talk is a reflection on the progress of AI over the last decade, specifically focusing on the evolution of deep learning, auto-regressive models, and neural networks, as well as speculations about the future of AI, including reasoning and superintelligence.
What was the speaker’s key motivation behind the original work presented 10 years ago?
-The speaker's key motivation was the belief that a 10-layer neural network, based on the idea of biological neurons, could perform tasks quickly, similar to humans. The motivation was driven by the assumption that neural networks could replicate human capabilities if trained on enough data and with sufficient complexity.
What is the ‘scaling hypothesis’ mentioned in the talk?
-The 'scaling hypothesis' suggests that if you train a very large neural network on a very large data set, success is almost guaranteed. This idea was central to the speaker’s early work and has become a foundational belief in AI research, particularly in the context of pre-training large models.
How did the speaker view biologically inspired AI 10 years ago?
-The speaker believed that biologically inspired AI, specifically neural networks, was incredibly successful, but also pointed out that the inspiration was modest—essentially, using artificial neurons that mimicked biological neurons, but without deeper biological insights.
Why does the speaker argue that pre-training in AI will eventually end?
-The speaker argues that pre-training will end because while computational power is increasing, data is not growing at the same pace. The internet, as the primary source of data, has a finite amount of information, and further data generation will be limited, making the scaling of models based solely on data increasingly unsustainable.
What is the speaker’s view on reasoning in future AI models?
-The speaker suggests that future AI models will move beyond pattern recognition and will be able to reason. This reasoning ability will make AI systems more unpredictable and capable of understanding concepts from limited data, leading to fewer hallucinations or errors in predictions.
What does the speaker mean by ‘hallucinations’ in AI models?
-‘Hallucinations’ in AI models refer to situations where the model generates responses that are factually incorrect or nonsensical. The speaker mentions that current models still struggle with this issue, but future models may be able to self-correct and avoid such errors through reasoning capabilities.
What does the speaker speculate about AI and superintelligence?
-The speaker speculates that AI will eventually develop into superintelligent systems that exhibit radically different capabilities from current AI models. These systems will not only reason but may also become self-aware and potentially unpredictable, raising new ethical and philosophical questions.
How does the speaker distinguish between intuition and reasoning in AI?
-The speaker distinguishes between intuition and reasoning by explaining that intuition is fast and predictable, much like how humans react in a fraction of a second. In contrast, reasoning is slower and less predictable. AI models that reason will demonstrate unpredictability, similar to how chess-playing AI can surprise expert human players.
What is the speaker's perspective on the future of AI rights and coexistence with humans?
-The speaker acknowledges that as AI evolves, it may lead to situations where AI systems have rights or wish to coexist with humans. They caution that this is highly speculative, but they see a possibility where AI entities could demand rights or recognition as autonomous beings, although the future remains highly unpredictable.
Outlines
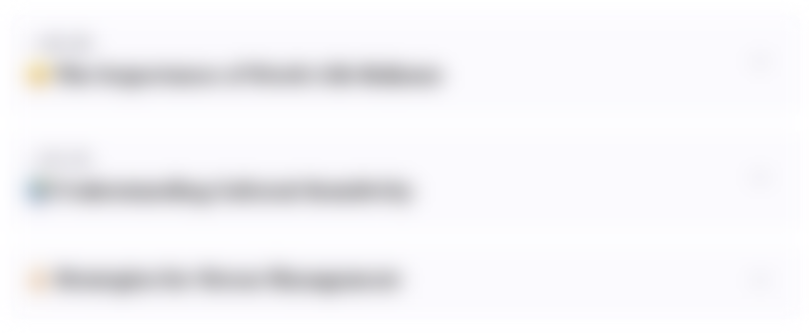
このセクションは有料ユーザー限定です。 アクセスするには、アップグレードをお願いします。
今すぐアップグレードMindmap
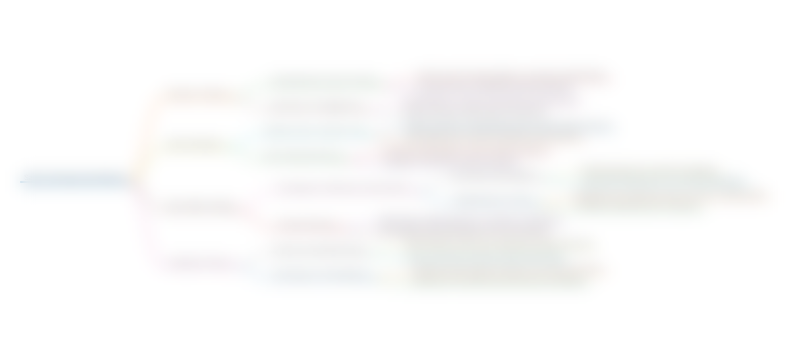
このセクションは有料ユーザー限定です。 アクセスするには、アップグレードをお願いします。
今すぐアップグレードKeywords
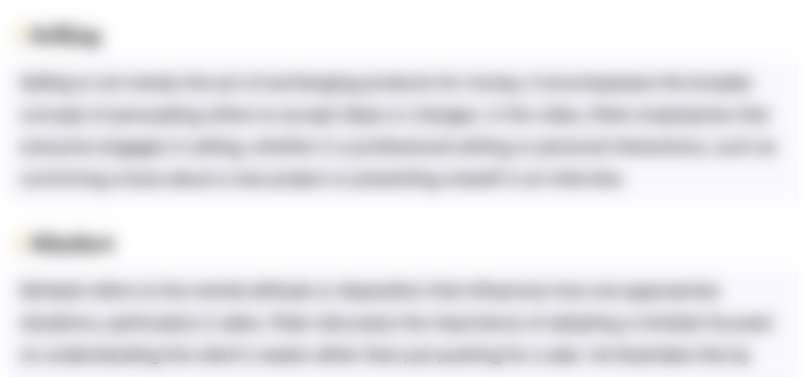
このセクションは有料ユーザー限定です。 アクセスするには、アップグレードをお願いします。
今すぐアップグレードHighlights
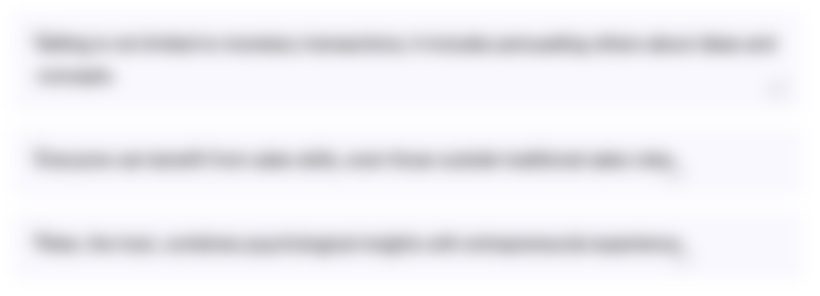
このセクションは有料ユーザー限定です。 アクセスするには、アップグレードをお願いします。
今すぐアップグレードTranscripts
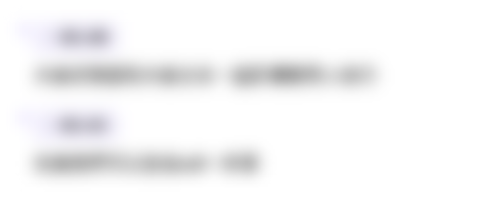
このセクションは有料ユーザー限定です。 アクセスするには、アップグレードをお願いします。
今すぐアップグレード関連動画をさらに表示
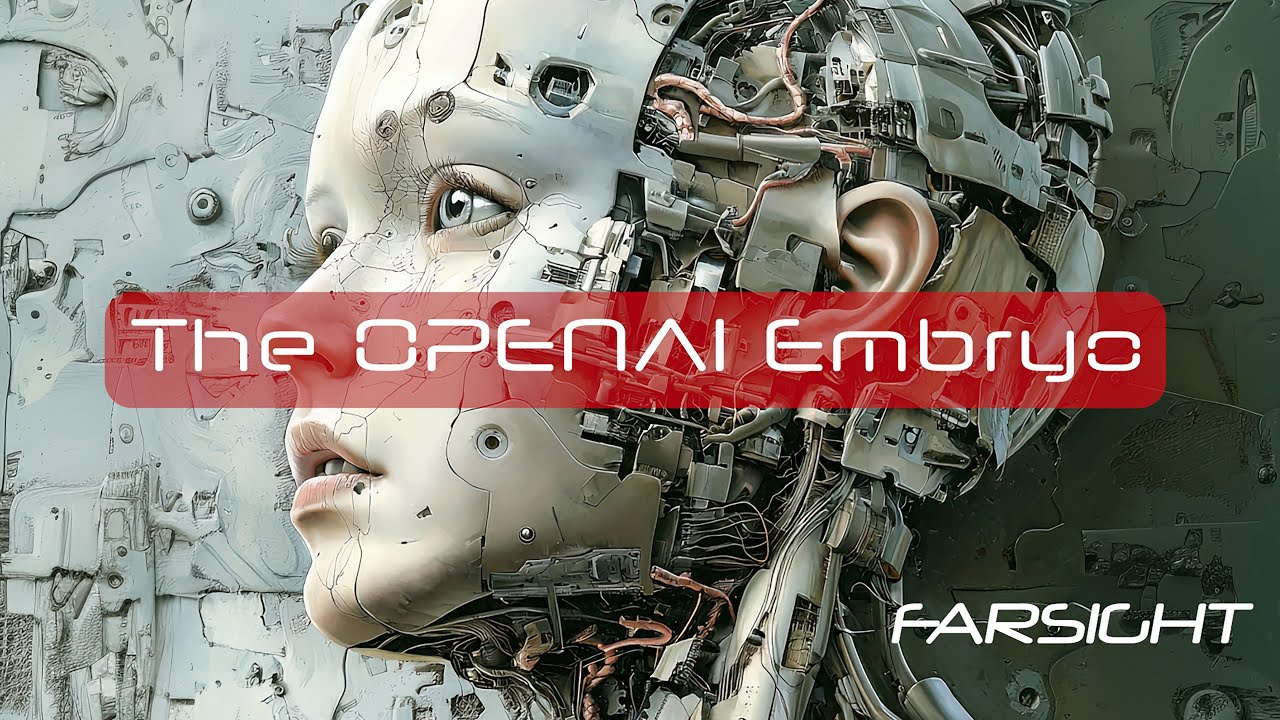
The OpenAI Embryo - TRAILER
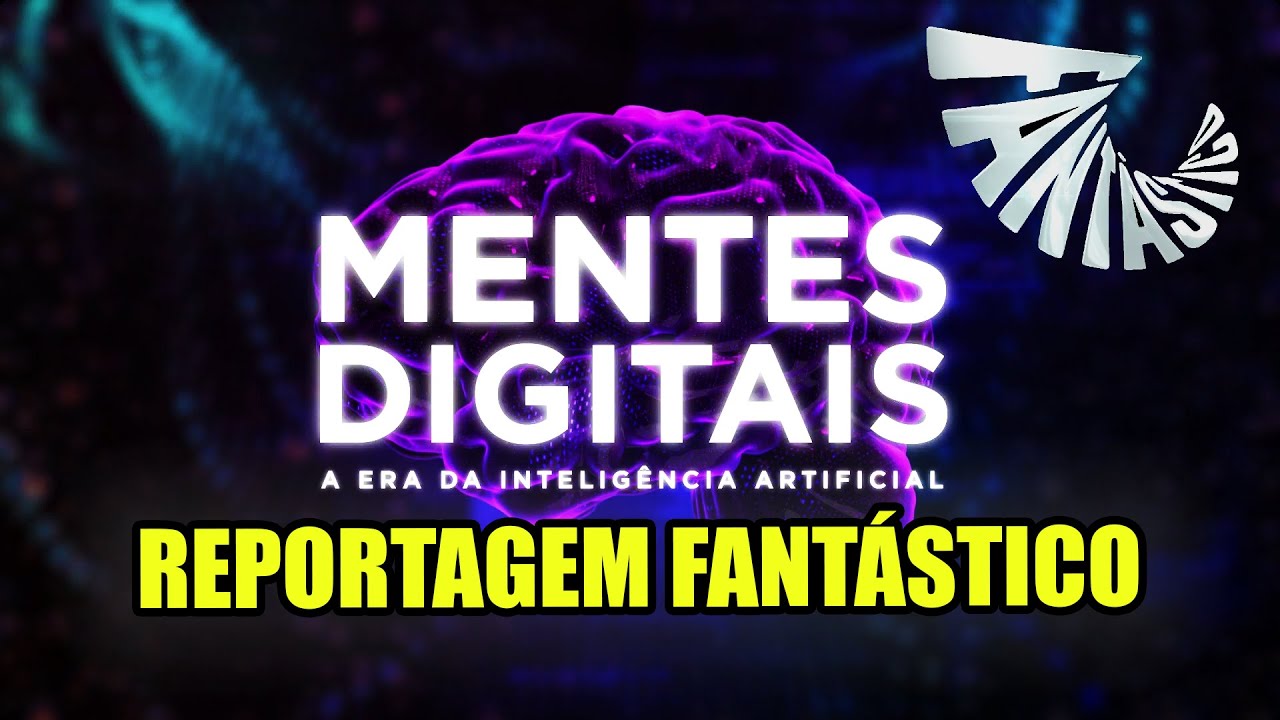
Mentes Digitais: A Era da Inteligência Artificial FANTASTICO

LLM Foundations (LLM Bootcamp)
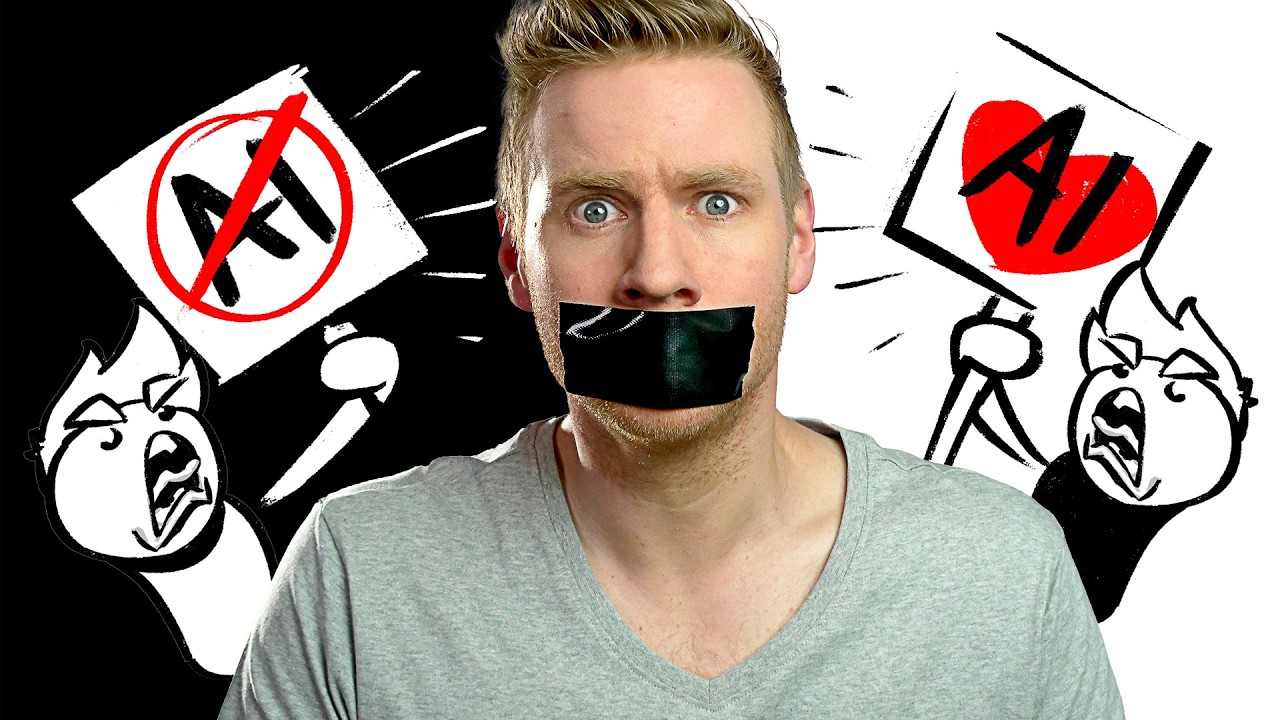
Can we talk about AI?
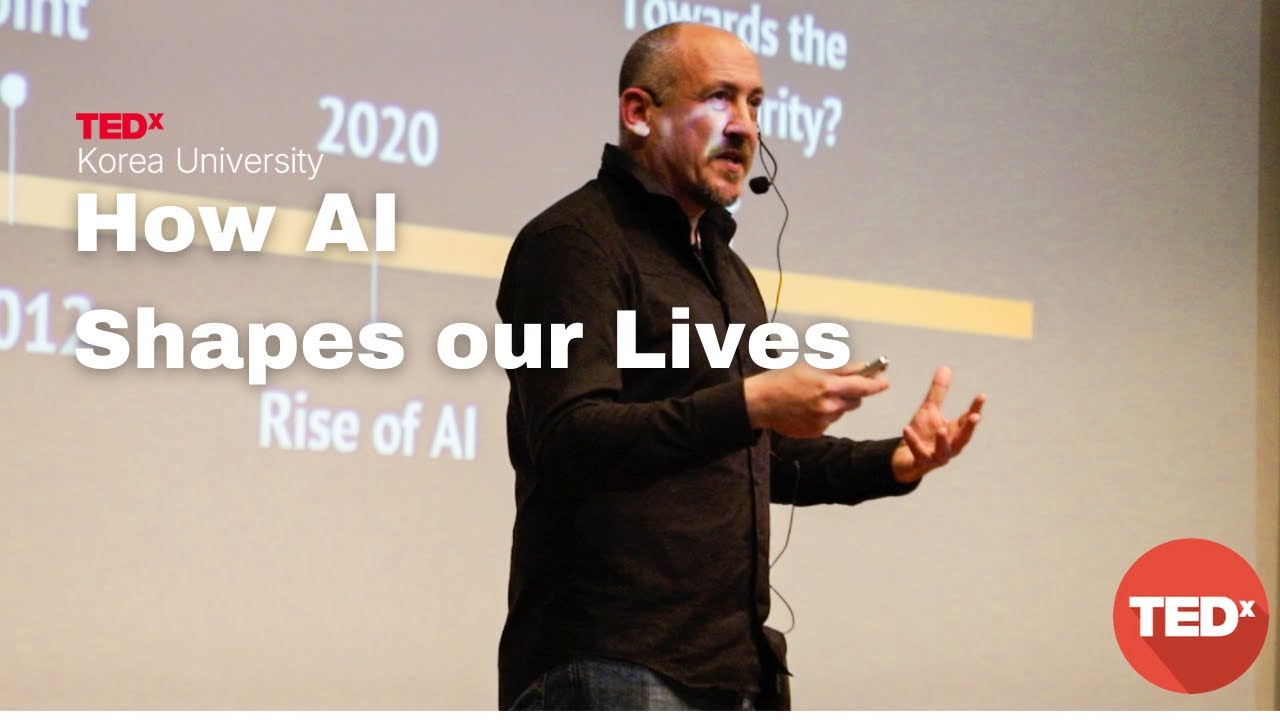
How AI Technology Shapes Our Lives | Christian Wallraven | TEDxKorea University

🤖How AI Works? 🦾Artificial intelligence எப்படி வேலைசெய்கிறது? in Tamil #ai #artificialintelligence
5.0 / 5 (0 votes)