Do You REALLY Need A Data Warehouse - What Are The Benefits Of A Data Warehouse?
Summary
TLDRThis video discusses the decision-making process of whether to build a data warehouse. It covers the benefits of data warehouses, including improved accessibility, historical tracking, and data integration, and explores use cases like reporting, automation, and machine learning. The speaker also outlines situations where a data warehouse may not be necessary, such as small-scale reporting or limited budgets. Alternative solutions like Tinybird, Rockset, and Excel are suggested for companies with simpler needs. Ultimately, the video encourages businesses to assess their data requirements and resources before committing to a data warehouse project.
Takeaways
- 😀 Building a data warehouse is a critical decision for companies at a stage where they have complex data sets and need deeper insights across various departments.
- 😀 A data warehouse centralizes and integrates data from different sources, making it easier to access and analyze for decision-making purposes.
- 😀 Not every company needs a data warehouse. If you're only doing minimal reporting, alternatives like Excel, direct solutions like Tableau, or smaller platforms might be sufficient.
- 😀 Data warehouses make data more accessible by reorganizing complex and normalized data into simpler formats like the 'fact and dimension' model, which is easier for analysts to understand.
- 😀 A key benefit of a data warehouse is tracking historical data. It ensures that you can analyze trends over time, even if the source systems don't track historical changes.
- 😀 Data warehouses are essential when data needs to be integrated from multiple, often siloed, systems. This avoids inconsistencies and errors when data is manually joined.
- 😀 Automation is another strong reason to build a data warehouse, especially when you need to streamline reporting and decision-making processes.
- 😀 Use cases for data warehouses include reporting and dashboarding, automating small decisions, and supporting machine learning models with reliable data.
- 😀 Machine learning and data science often rely on data warehouses as they provide clean, processed, and reliable datasets, making them an ideal foundation for model development.
- 😀 However, building a data warehouse can be expensive, both in terms of initial setup and ongoing maintenance, which might not be justified for smaller companies with minimal reporting needs.
Q & A
What is a data warehouse and what purpose does it serve?
-A data warehouse is a centralized repository for storing data from multiple sources. It integrates, organizes, and makes data accessible for analysis, reporting, and decision-making. Its purpose is to provide a unified and historical view of data that can support business intelligence activities.
What are the benefits of building a data warehouse?
-The key benefits of a data warehouse include easier data accessibility, integration of multiple data sources, tracking of historical data, improved reporting and dashboarding, and automation of reporting processes.
What does it mean for a data warehouse to track historical data?
-Tracking historical data in a data warehouse allows businesses to analyze changes over time. For example, it can store historical customer information, such as past addresses, enabling analysis of customer behavior across different time periods.
Why is data integration important in a data warehouse?
-Data integration is crucial because it allows disparate systems (e.g., CRM, finance, marketing) to be joined together. Without proper integration, it's difficult to perform meaningful analysis across these systems, leading to inconsistent and potentially inaccurate insights.
What is the 'fact dim' approach in data warehousing?
-The 'fact dim' approach involves creating fact tables (core data) and dimension tables (attributes related to the data). This approach is useful because it makes the data easier to understand for analysts, allowing them to slice and dice the data by dimensions like time, customer, or location.
When should a business consider building a data warehouse?
-A business should consider building a data warehouse when it has a significant volume of data across multiple sources, requires complex reporting and analysis, or needs to automate data processes. If data starts to grow in complexity and scale, a data warehouse can centralize the data and make it more manageable.
What are some alternatives to building a data warehouse for small businesses?
-For small businesses, alternatives to a data warehouse include using lightweight solutions like Tinybird or Rockset, or directly connecting reporting tools such as Tableau to data sources. Excel can also be sufficient for basic reporting needs.
What are the potential downsides of building a data warehouse?
-The major downsides of building a data warehouse are the high costs involved, including hiring specialized personnel (e.g., data engineers or architects) and the ongoing maintenance expenses. If the business has limited data or simple reporting needs, the investment may not justify the returns.
How does automation play a role in data warehouses?
-Automation in data warehouses helps by reducing manual reporting and data processing tasks. This includes automating data pulls, generating regular reports, and even triggering decisions based on predefined criteria, saving time and reducing the risk of human error.
Can a data warehouse be used for machine learning and data science?
-Yes, data warehouses can be used for machine learning and data science. Since the data in a well-designed warehouse is typically clean, accurate, and structured, it can serve as a reliable foundation for building and training machine learning models.
Outlines
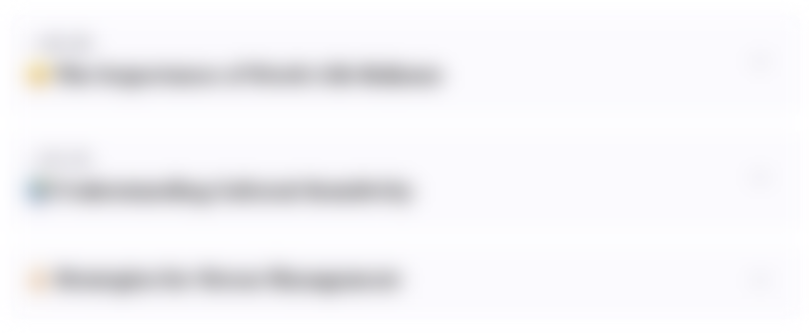
このセクションは有料ユーザー限定です。 アクセスするには、アップグレードをお願いします。
今すぐアップグレードMindmap
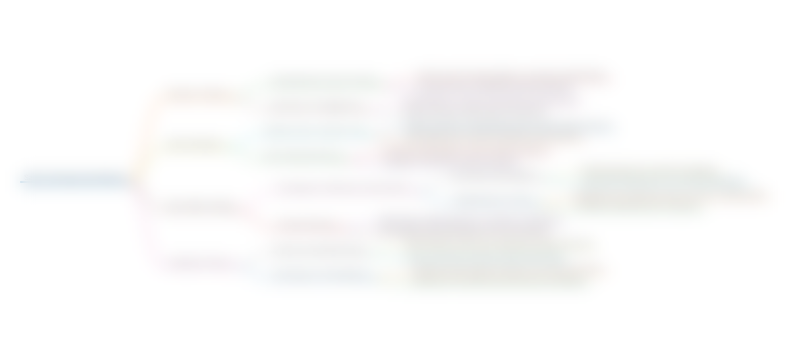
このセクションは有料ユーザー限定です。 アクセスするには、アップグレードをお願いします。
今すぐアップグレードKeywords
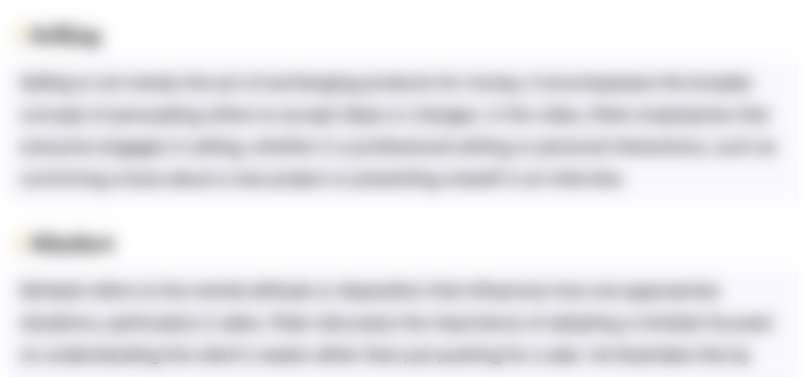
このセクションは有料ユーザー限定です。 アクセスするには、アップグレードをお願いします。
今すぐアップグレードHighlights
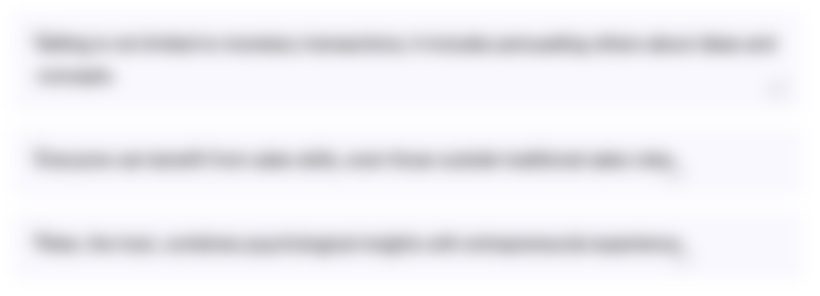
このセクションは有料ユーザー限定です。 アクセスするには、アップグレードをお願いします。
今すぐアップグレードTranscripts
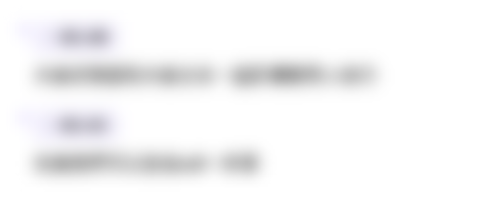
このセクションは有料ユーザー限定です。 アクセスするには、アップグレードをお願いします。
今すぐアップグレード関連動画をさらに表示

What is Warehouse Management System | WMS Software | Functions & Benefits of WMS | How WMS works
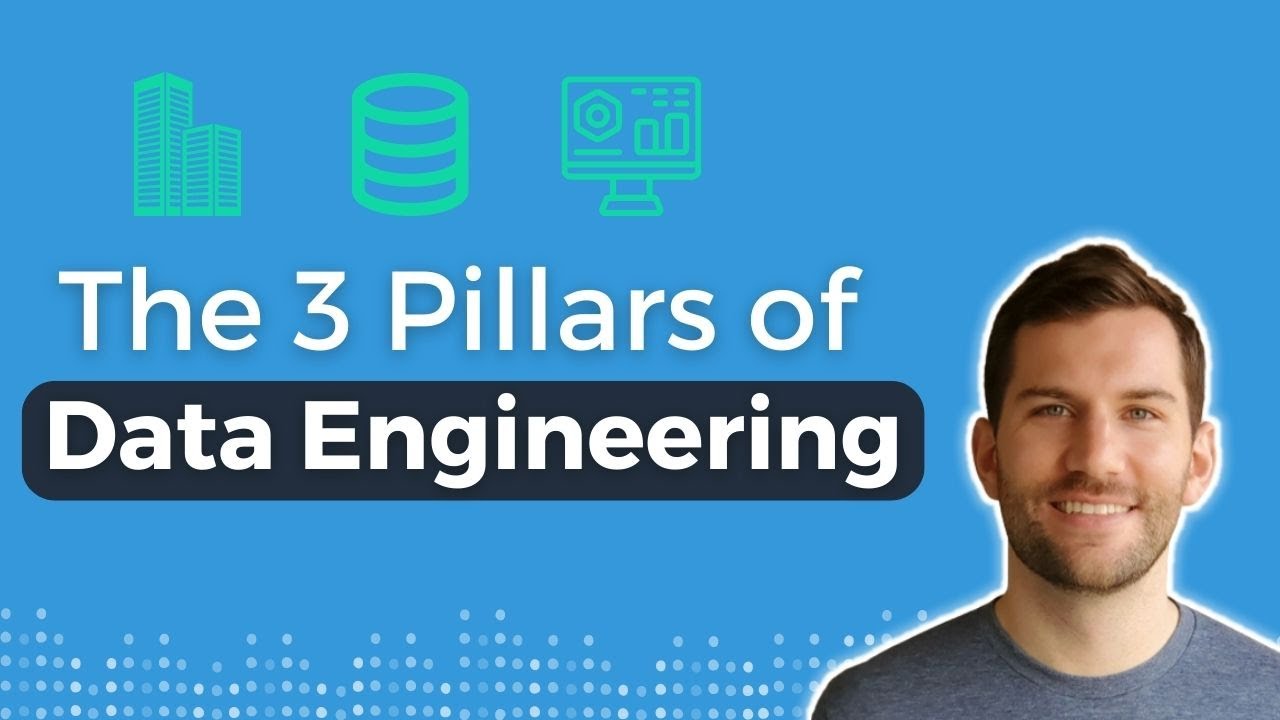
The 3 Pillars of Data Engineering
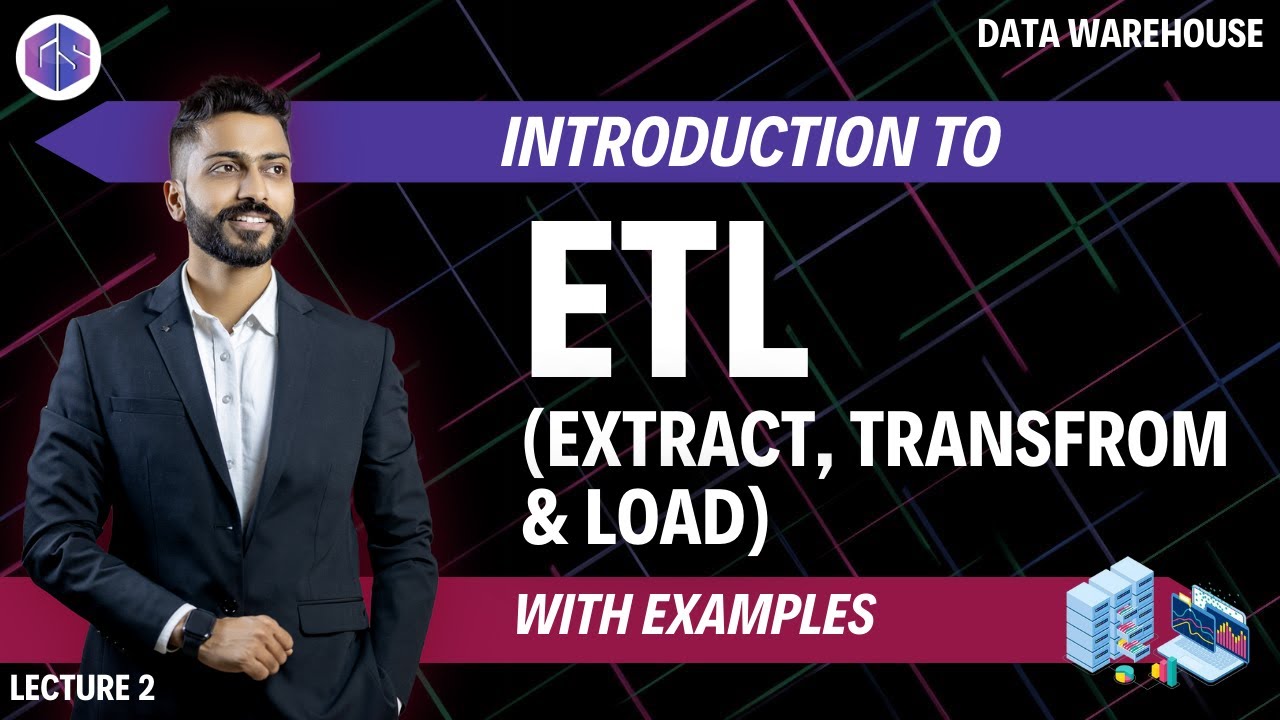
ETL (Extract, Transform, Load) | Data 📊Aggregation | Data Warehouse🏭 & Mining ⛏️
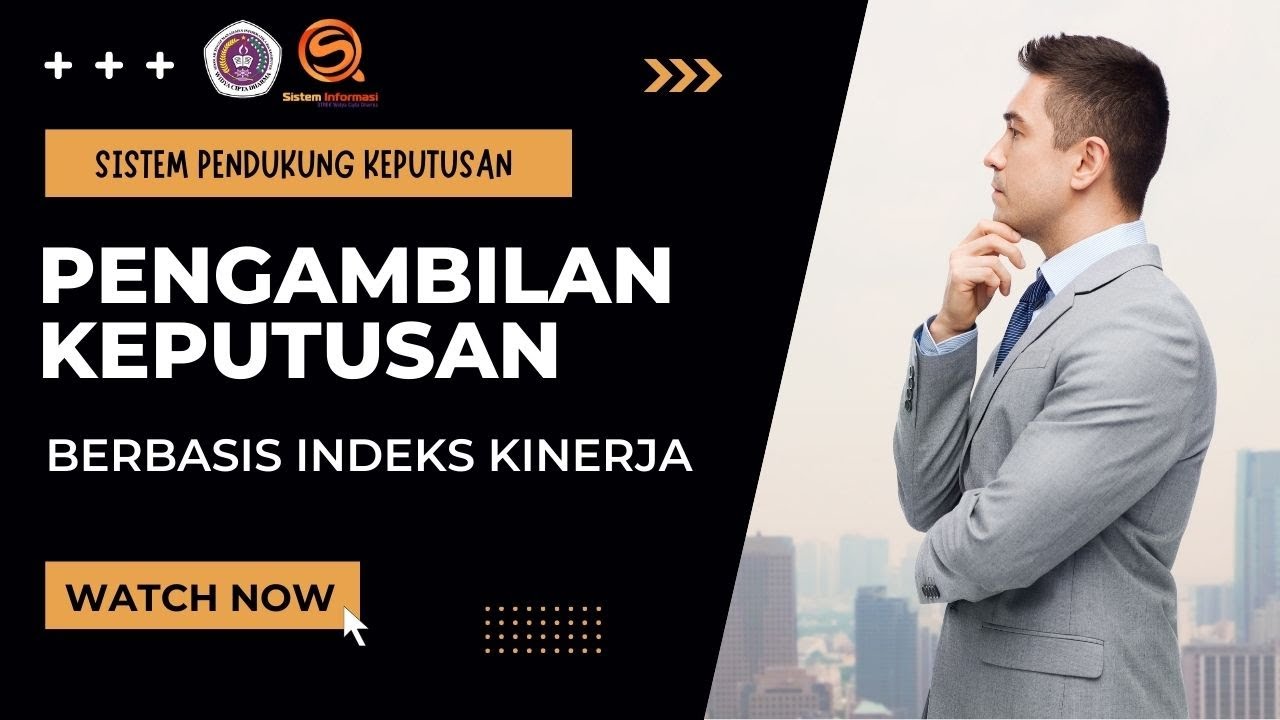
Sistem Pendukung Keputusan - Pengambilan Keputusan Berbasis Indeks Kinerja
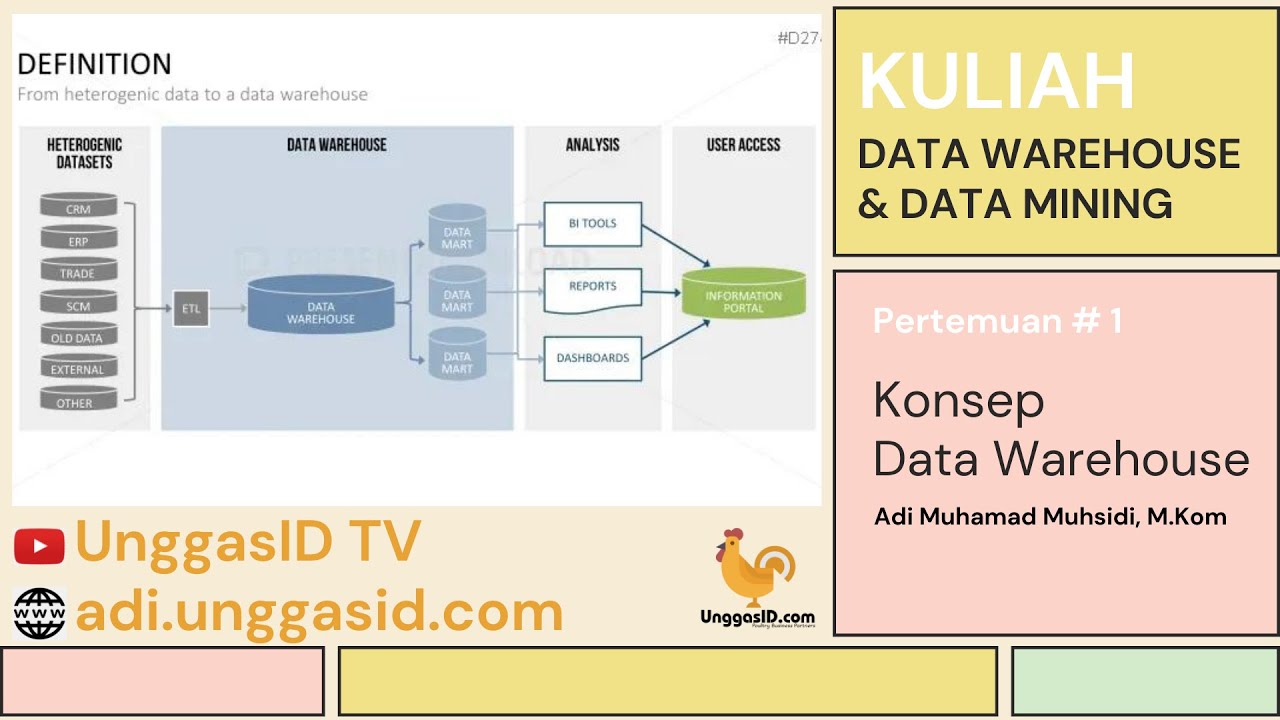
Kuliah Data Warehouse & Data Mining - 01. Konsep Data Warehouse

Riset Pemasaran - Marketing Research Process
5.0 / 5 (0 votes)