SHOCKING Robots EVOLVE in the SIMULATION plus OpenAI Leadership Just... LEAVES?
Summary
TLDRThe video script discusses recent advancements in AI, focusing on the rapid progress of Figure 1's AI and robotics, particularly their robot's ability to perform tasks like handling an orange using visual reasoning. It also touches on the ethical considerations of training robots by kicking them and the potential legal issues surrounding AI and copyright. The script introduces Dr. Eureka, an AI agent that automates the process of training robots in simulation and bridging the gap to real-world deployment. Additionally, it covers the departure of two executives from OpenAI and the potential implications for AI-generated content and copyright law. The video also explores the concept of multi-token prediction to improve language models' efficiency and the release of Devon 2.0, an AI agent capable of performing complex tasks. Finally, it mentions the development of wearable AI devices, such as open-source AI glasses that can provide real-time information and assistance.
Takeaways
- 🤖 The advancements in AI robotics are significant, with Figure 01 showcasing an AI-driven robot that can perform tasks like identifying healthy food options through visual reasoning.
- 📈 Figure One is utilizing pre-trained models via OpenAI to output common sense reasoning, indicating a trend towards integrating AI with robotics for enhanced functionality.
- 🔧 The robot's ability to grapple an orange is facilitated by an in-house trained neural network, highlighting the role of neural networks in translating visual data into physical actions.
- 📱 Concerns are raised about the practice of 'kicking' robots for demonstration purposes and the ethical implications of training AI through adversarial means.
- 🐕 Dr. Jim Fan discusses training a robot dog to balance on a yoga ball using simulation, emphasizing the potential for zero-shot learning transfers to the real world without fine-tuning.
- 🌐 The introduction of Dr. Eureka, an LLM agent that writes code for robot skill training in simulation and bridges the simulation-reality gap, represents a step towards automating the entire robot learning pipeline.
- 📚 Eureka's ability to generate novel rewards for complex tasks suggests that AI can devise solutions that differ from human approaches, potentially offering better outcomes for advanced tasks.
- 📉 Two senior executives from OpenAI, Diane Yun and Chris Clark, have left the company, raising questions about the reasons behind their departure and the impact on the organization.
- 📄 A paper by Ethan M discusses copyright issues for AI-generated content, proposing a framework for compensating copyright owners based on their contribution to AI generative content.
- 🔑 The paper suggests that the act of 'reading' or training on copyrighted material by AI models may not be copyright infringement in itself, but rather the reproduction of similar works.
- 📈 Research indicates that training language models to predict multiple future tokens at once can lead to higher sample efficiency and faster inference times, which could significantly improve the performance of large language models.
- 🧊 Devon 2.0, an AI agent, is capable of performing complex tasks such as creating a website to play chess against a language model and visualizing data, although it may encounter bugs that need fixing.
Q & A
What is the significance of Figure 01's robot and its AI capabilities as mentioned in the transcript?
-Figure 01's robot, equipped with AI, is significant because it demonstrates the integration of robots and AI as the next frontier in technology. The robot showcased on '60 Minutes' is capable of common sense reasoning and can perform tasks like selecting a healthy food item over an unhealthy one based on visual cues, which is a step towards more autonomous and intelligent machines.
How does the robot in the transcript determine which object to pick based on the request for something healthy?
-The robot uses visual reasoning via its cameras to identify objects within its field of view. It is connected to a pre-trained model via Open AI, which helps it to output common sense reasoning. When asked to hand over something healthy, it recognizes the orange as the healthy choice instead of the chips.
What is the role of Dr. Jim Fan in the development of AI and robots as described in the transcript?
-Dr. Jim Fan is involved in training robots using simulations and transferring those skills to the real world without fine-tuning. He is also associated with the development of Dr. Eureka, an LLM agent that writes code to train robot skills in simulation and bridges the simulation-reality gap, automating the pipeline from new skill learning to real-world deployment.
What is the concern raised about training AI on internet footage?
-The concern raised is the ethical implications of training AI systems using footage that may have been obtained without consent, such as recording robots being kicked or abused and then using that footage to train AI. This raises questions about consent, privacy, and the potential for misuse of such technology.
How does the proposed Dr. Eureka system differ from traditional simulation-to-real transfer methods?
-The Dr. Eureka system automates the process of transferring skills from simulation to the real world, which traditionally required domain randomization and manual adjustments by expert roboticists. Instead of tedious manual work, Dr. Eureka uses AI to search over a vast space of sim-to-real configurations, enabling more efficient and effective training of robots.
What is the potential impact of GPT-5 on the process described in the transcript?
-The potential impact of GPT-5, as inferred from the capabilities of GPT-4, could be significant. It suggests that with the advancement to GPT-5, the process of sim-to-real transfer and the tuning of physical parameters such as friction, damping, and gravity could become even more efficient and accurate, potentially leading to better performance in real-world applications.
What is the main idea behind training robots in simulation as discussed in the transcript?
-The main idea is to allow robots to learn and master various skills in a simulated environment that mimics the real world's physics. This enables the robots to learn complex tasks like walking, balancing, opening doors, and picking up objects, which can then be transferred to real-world scenarios, increasing efficiency and reducing the need for physical trials and errors.
What is the role of Nvidia's Isaac SIM in the context of the transcript?
-Nvidia's Isaac SIM is mentioned as a platform where the physics of the simulated world exist just like in the real world, but it allows for running simulations at a much faster pace. This high-speed simulation capability is crucial for training robots efficiently and testing various scenarios before deploying them in the real world.
How does the Eureka algorithm contribute to the automation of the robot learning pipeline?
-The Eureka algorithm contributes by teaching a robot hand to perform complex tasks like pen spinning within a simulation. It takes the process further by automating the entire pipeline from learning new skills in simulation to deploying those skills in the real world, reducing the need for human intervention in the training process.
What is the proposed framework for dealing with copyright issues for AI-generated content as mentioned in the transcript?
-The proposed framework aims to compensate copyright owners based on their contribution to the creation of AI-generated content. The metric for contributions is determined quantitatively by leveraging the probabilistic nature of modern generative AI models, suggesting a potential solution for the debate on copyright infringement in AI training.
What is the potential impact of multi-token prediction in training language models as discussed in the transcript?
-Multi-token prediction could lead to higher sample efficiency and improved performance on generative benchmarks, especially in tasks like coding. It also suggests that models trained this way can have up to 3 times faster inference, even with large batch sizes, which could significantly enhance the development of algorithmic reasoning capabilities in AI.
Outlines
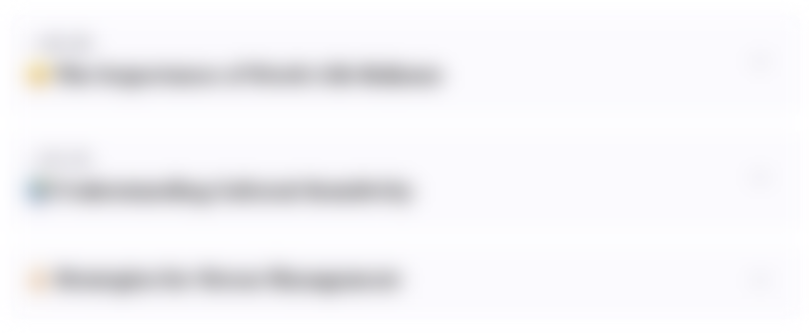
このセクションは有料ユーザー限定です。 アクセスするには、アップグレードをお願いします。
今すぐアップグレードMindmap
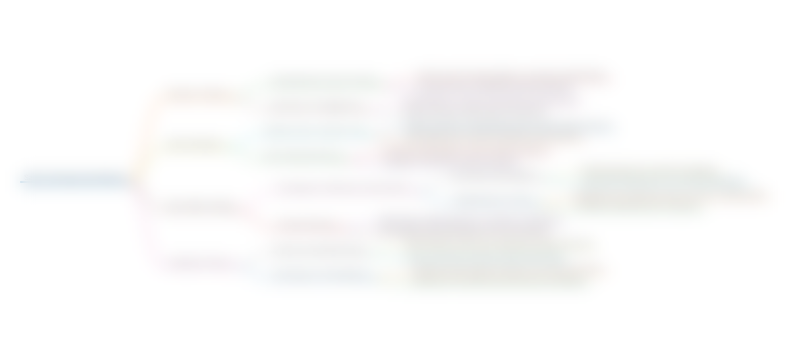
このセクションは有料ユーザー限定です。 アクセスするには、アップグレードをお願いします。
今すぐアップグレードKeywords
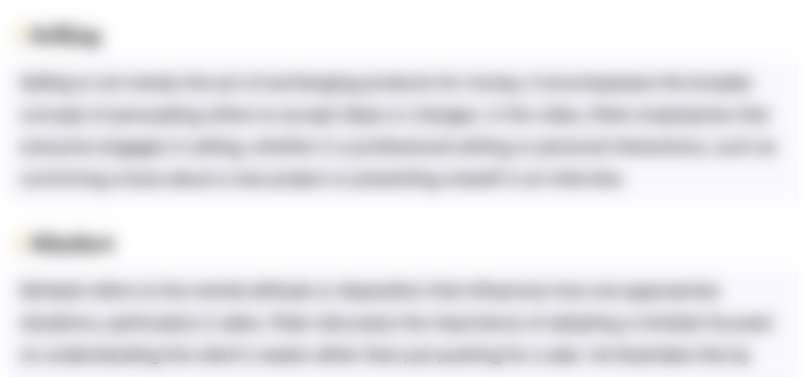
このセクションは有料ユーザー限定です。 アクセスするには、アップグレードをお願いします。
今すぐアップグレードHighlights
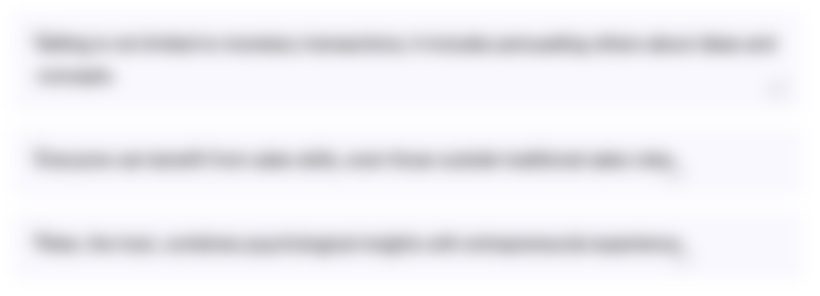
このセクションは有料ユーザー限定です。 アクセスするには、アップグレードをお願いします。
今すぐアップグレードTranscripts
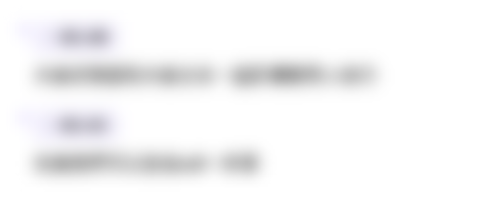
このセクションは有料ユーザー限定です。 アクセスするには、アップグレードをお願いします。
今すぐアップグレード関連動画をさらに表示
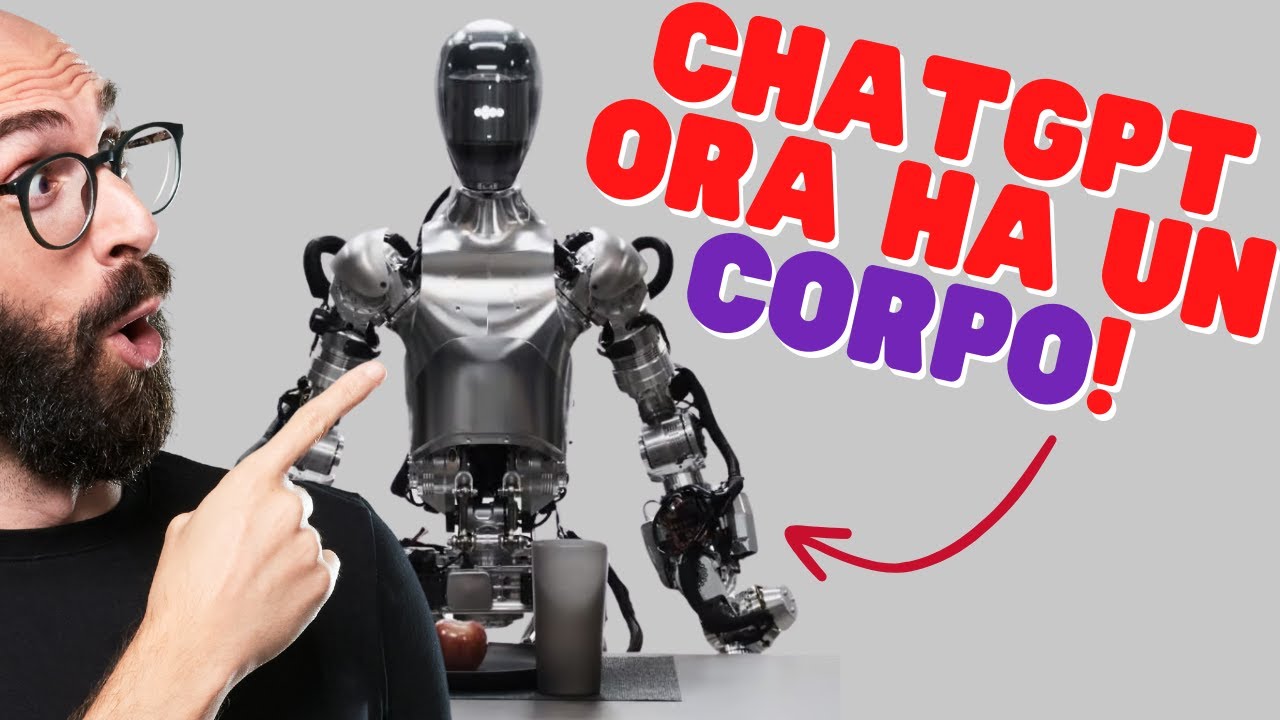
Questo robot vede, sente e parla grazie a ChatGPT [Figure 01]

OpenAI's 'AGI Robot' Develops SHOCKING NEW ABILITIES | Sam Altman Gives Figure 01 Get a Brain
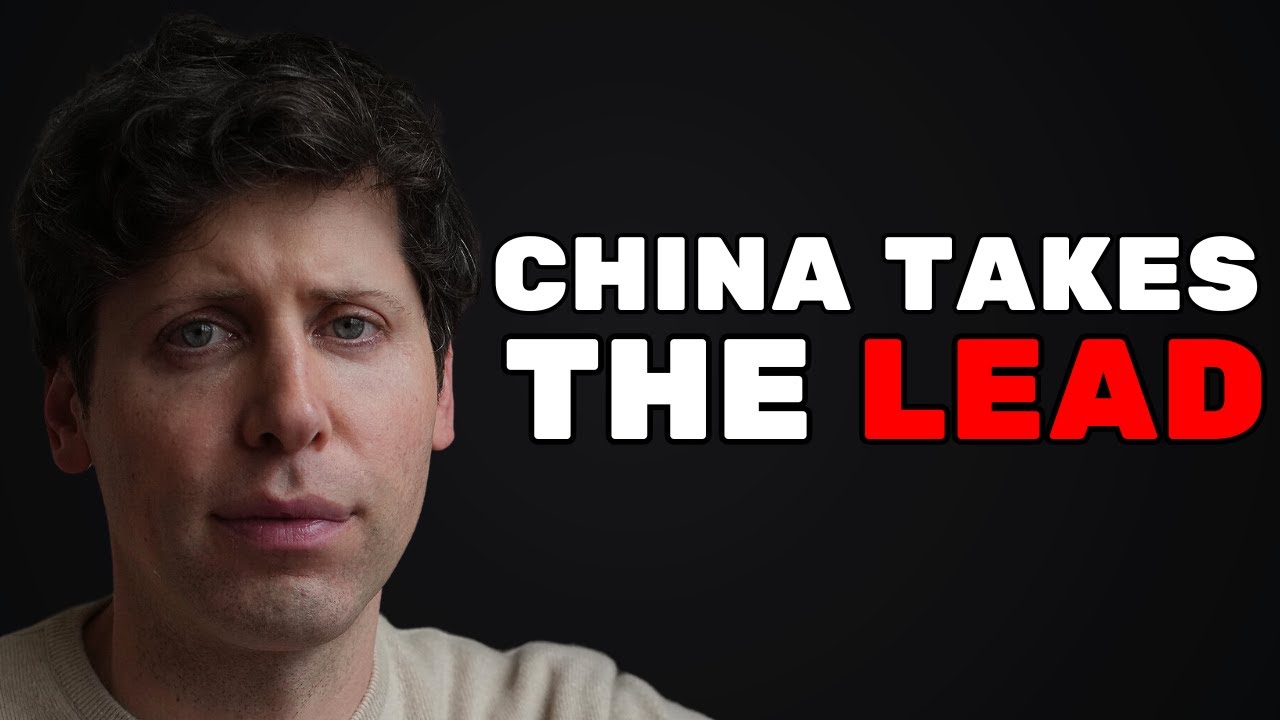
China's 'DeepSeek R1' DEFEATS OpenAI's o1! AI Art Turing Test, Figure 02 Update
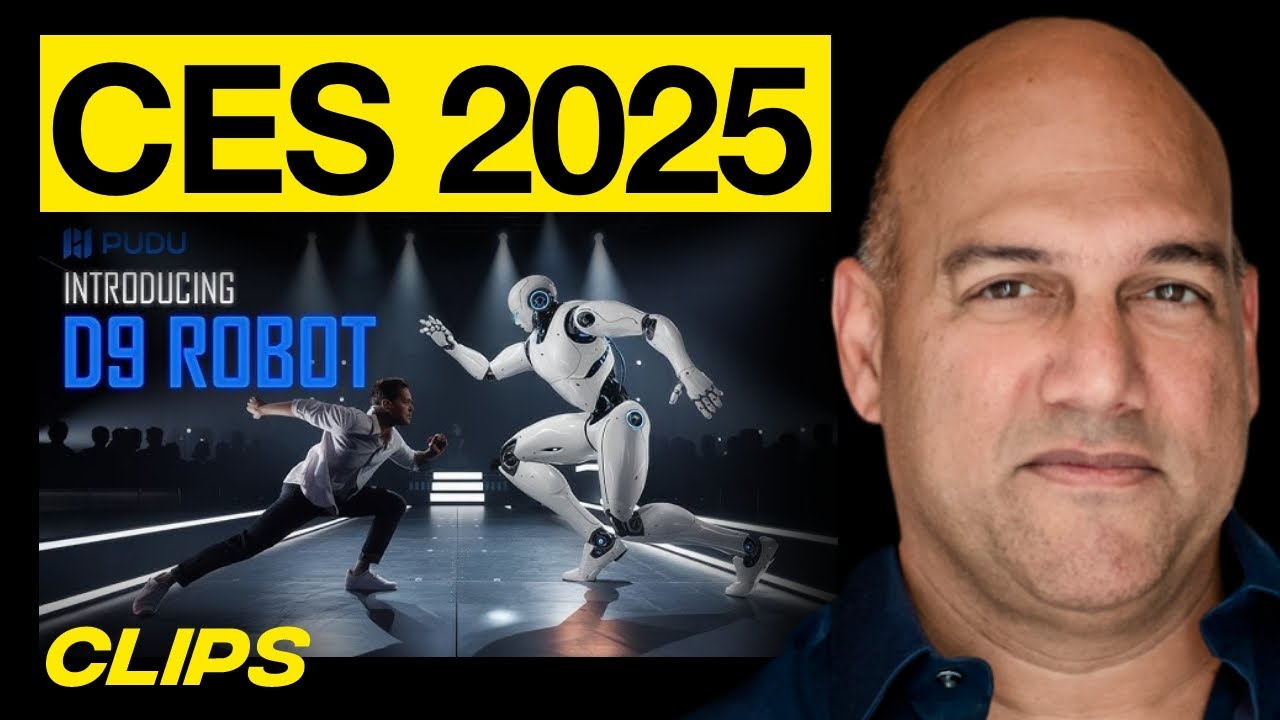
The Insane Tech You Missed From CES 2025 | MOONSHOTS
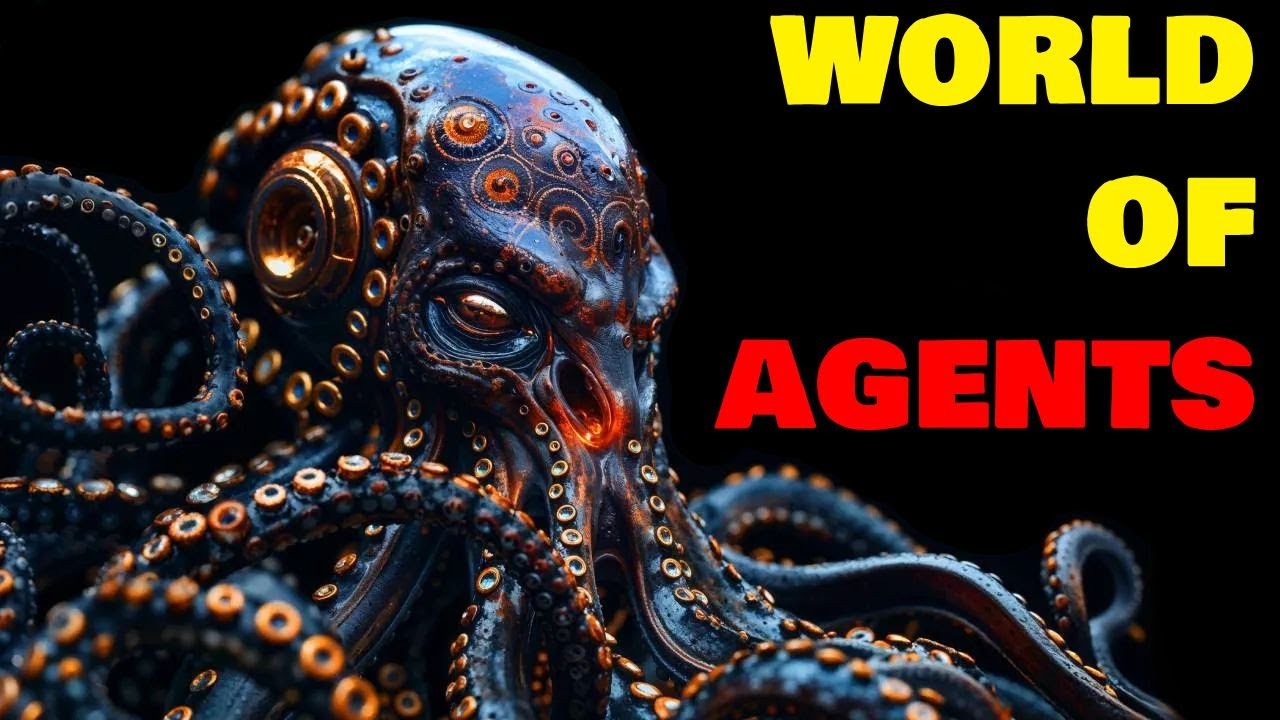
STUNNING Step for Autonomous AI Agents PLUS OpenAI Defense Against JAILBROKEN Agents
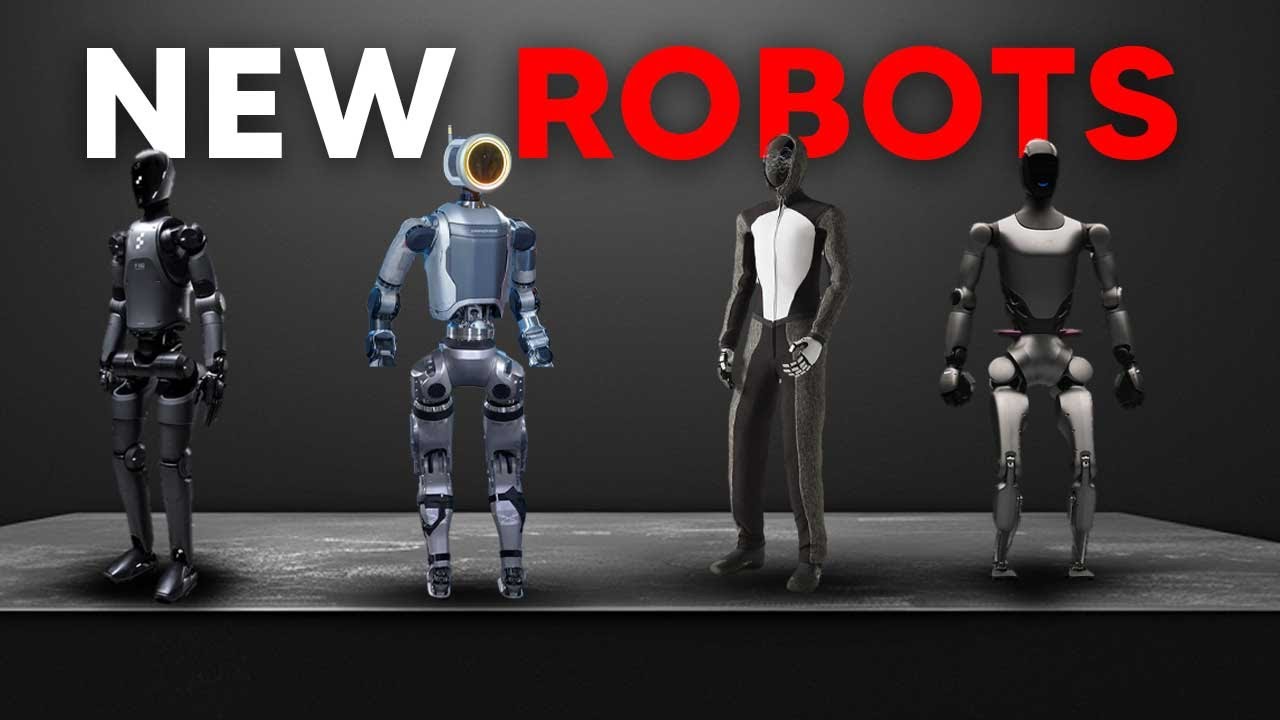
Top 10 NEW Humanoid Robots of 2024 (Updated)
5.0 / 5 (0 votes)