Parametric and Nonparametric Statistical Tests
Summary
TLDRIn this video, Dr. Grande explores the distinctions between parametric and nonparametric tests in counseling research. He outlines common tests, such as the independent samples t-test and Mann-Whitney U test, emphasizing the importance of assessing data against specific assumptions. Parametric tests generally require larger sample sizes and are more powerful in detecting true differences, while nonparametric tests are more flexible but less powerful. Dr. Grande provides clear guidelines for selecting the appropriate test based on data characteristics, underscoring the need for careful evaluation to ensure valid statistical analysis.
Takeaways
- 📊 Parametric tests, such as the independent samples t-test and one-way ANOVA, are commonly used in counseling research.
- 🔍 Nonparametric tests, including Mann-Whitney and Kruskal-Wallis tests, are alternatives when data does not meet parametric assumptions.
- ✅ The choice between parametric and nonparametric tests depends on analyzing the data and its adherence to specific assumptions.
- 🔑 Key assumptions for parametric tests include random independent samples, interval or ratio measurement levels, and normally distributed data.
- 📉 Parametric tests are generally more powerful, meaning they are better at detecting true differences and are less likely to produce Type II errors.
- ⚖️ Nonparametric tests have fewer assumptions but can be more conservative and may lead to Type II errors more frequently.
- 📋 Common assumptions for nonparametric tests include random independent samples and specific distribution shapes for certain tests.
- 🔄 The Mann-Whitney test corresponds to the independent samples t-test when parametric assumptions are not met.
- 🔁 The Wilcoxon signed-rank test serves as the nonparametric equivalent for the paired samples t-test.
- 📝 Always check the assumptions of both parametric and nonparametric tests before choosing which one to use in research.
Q & A
What are parametric tests?
-Parametric tests are statistical tests that assume a specific distribution for the data, typically the normal distribution. They require certain assumptions to be met regarding the data's level of measurement and distribution.
What are some examples of parametric tests mentioned in the video?
-The video mentions three common parametric tests: independent samples t-test, paired samples t-test (or dependent samples t-test), and one-way ANOVA.
What are nonparametric tests?
-Nonparametric tests are statistical tests that do not assume a specific distribution for the data. They are used when the assumptions for parametric tests are not met and are generally more flexible.
Can you provide examples of nonparametric tests?
-Examples of nonparametric tests mentioned in the video include the Mann-Whitney test, Wilcoxon signed-rank test, and Kruskal-Wallis test.
Why are parametric tests preferred over nonparametric tests?
-Parametric tests are generally preferred because they have greater statistical power, meaning they are more likely to detect a true difference when one exists and are less prone to type 2 errors.
What are some common assumptions for parametric tests?
-Common assumptions for parametric tests include having random independent samples, using interval or ratio level measurement, ensuring the data is normally distributed, having no outliers, and satisfying the homogeneity of variance.
What happens if the assumptions for parametric tests are not met?
-If the assumptions for parametric tests are not met, researchers may opt to use nonparametric tests, which have fewer and less stringent assumptions.
What is a type 2 error?
-A type 2 error occurs when a statistical test fails to reject the null hypothesis when it is actually false, meaning that a difference that does exist is not detected.
How do the assumptions for nonparametric tests compare to those for parametric tests?
-Nonparametric tests have fewer assumptions than parametric tests and are less rigid, but they still require some conditions to be met, such as having random independent samples and certain distributional properties.
What is the significance of checking assumptions before conducting statistical tests?
-Checking assumptions is crucial because violating them can lead to incorrect conclusions, such as accepting or rejecting a hypothesis based on unreliable results. Both parametric and nonparametric tests have their own assumptions that must be verified.
Outlines
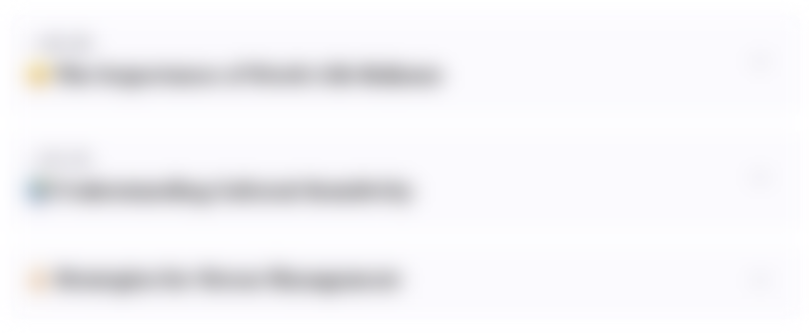
このセクションは有料ユーザー限定です。 アクセスするには、アップグレードをお願いします。
今すぐアップグレードMindmap
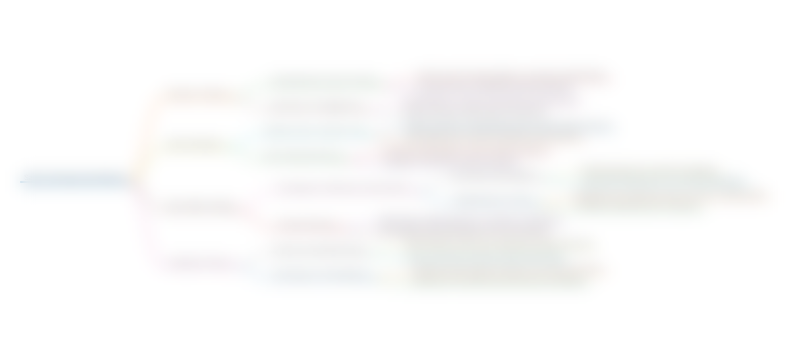
このセクションは有料ユーザー限定です。 アクセスするには、アップグレードをお願いします。
今すぐアップグレードKeywords
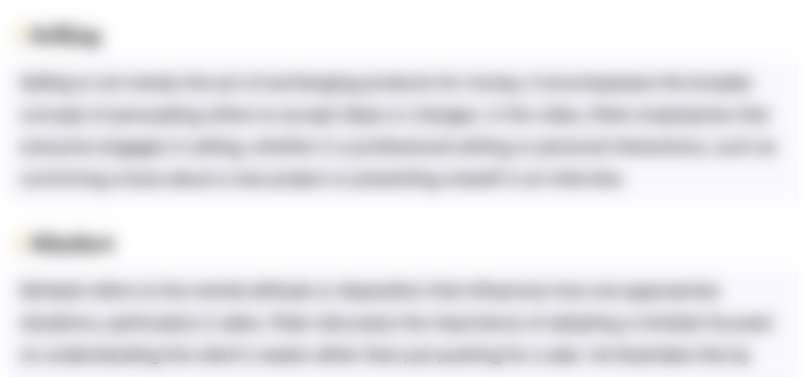
このセクションは有料ユーザー限定です。 アクセスするには、アップグレードをお願いします。
今すぐアップグレードHighlights
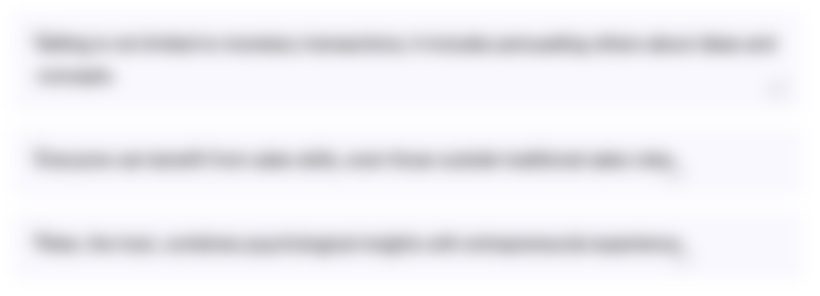
このセクションは有料ユーザー限定です。 アクセスするには、アップグレードをお願いします。
今すぐアップグレードTranscripts
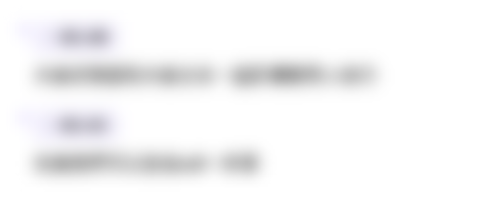
このセクションは有料ユーザー限定です。 アクセスするには、アップグレードをお願いします。
今すぐアップグレード関連動画をさらに表示
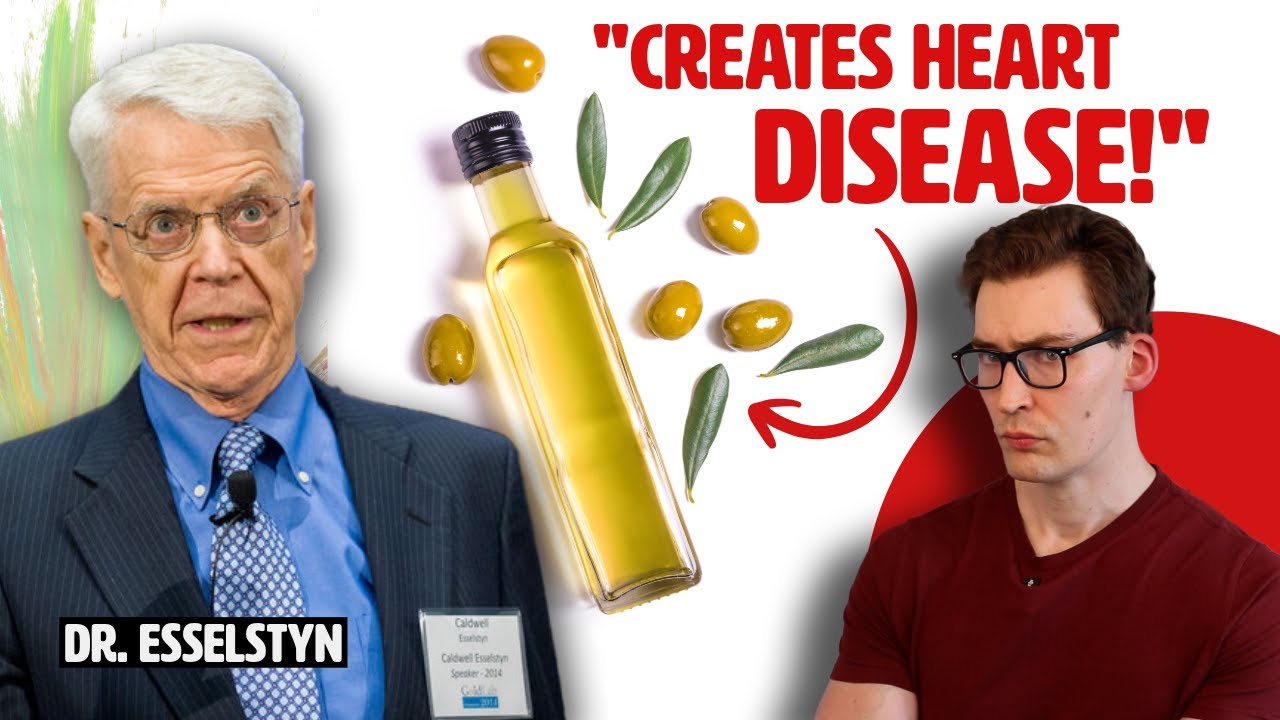
Dr. Esselstyn: “Mediterranean Diet (and Olive Oil) creates Heart Disease!”

Should Letter Grades Be Abandoned?

Think Cultural Health Case Study: Cultural and religious beliefs
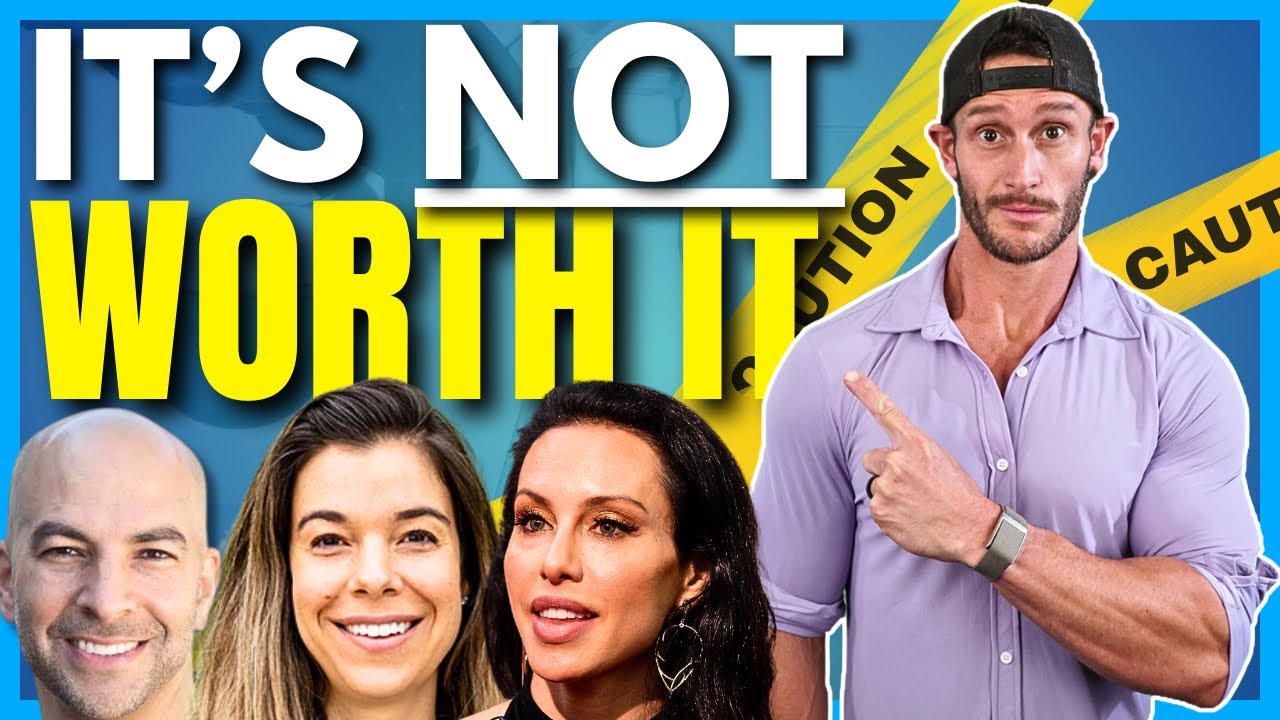
Why Experts are Warning Against Fasting - Dr. Peter Attia, Dr. Rhonda Patrick, Dr. Gabrielle Lyon

Dr G Medical Examiner - Internal Exam
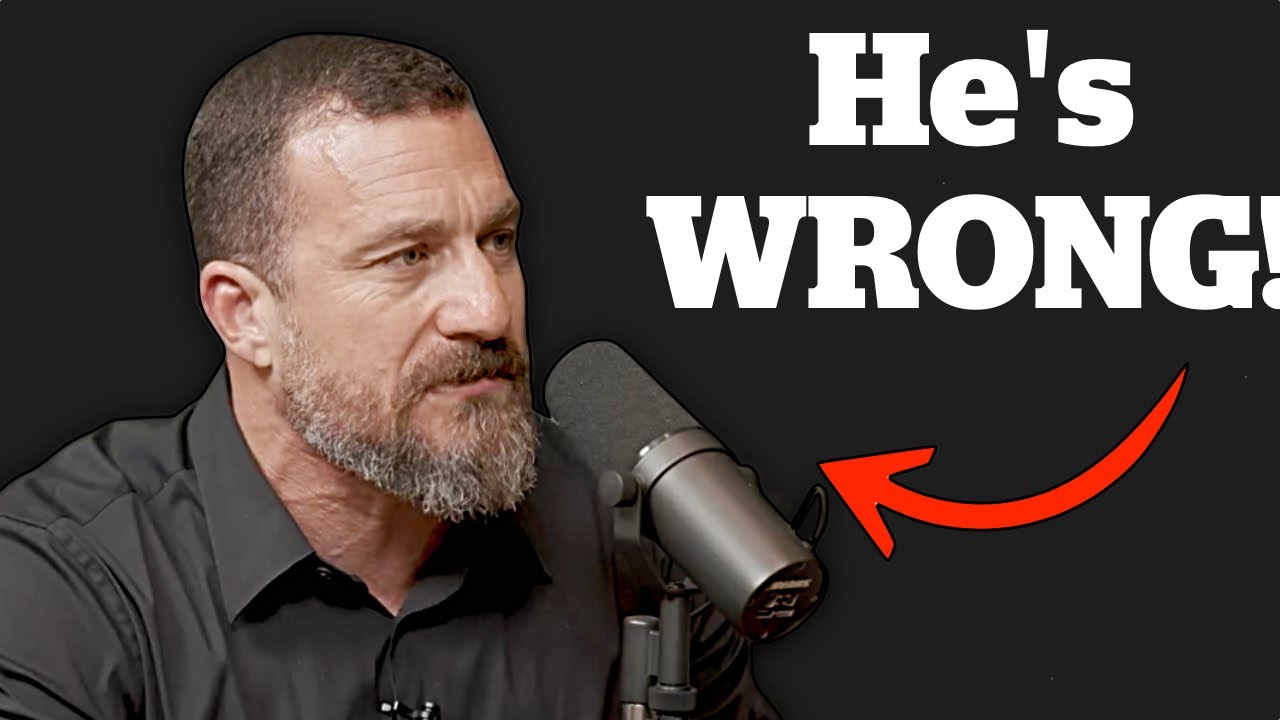
Don’t Listen to Andrew Huberman About this Fitness Advice
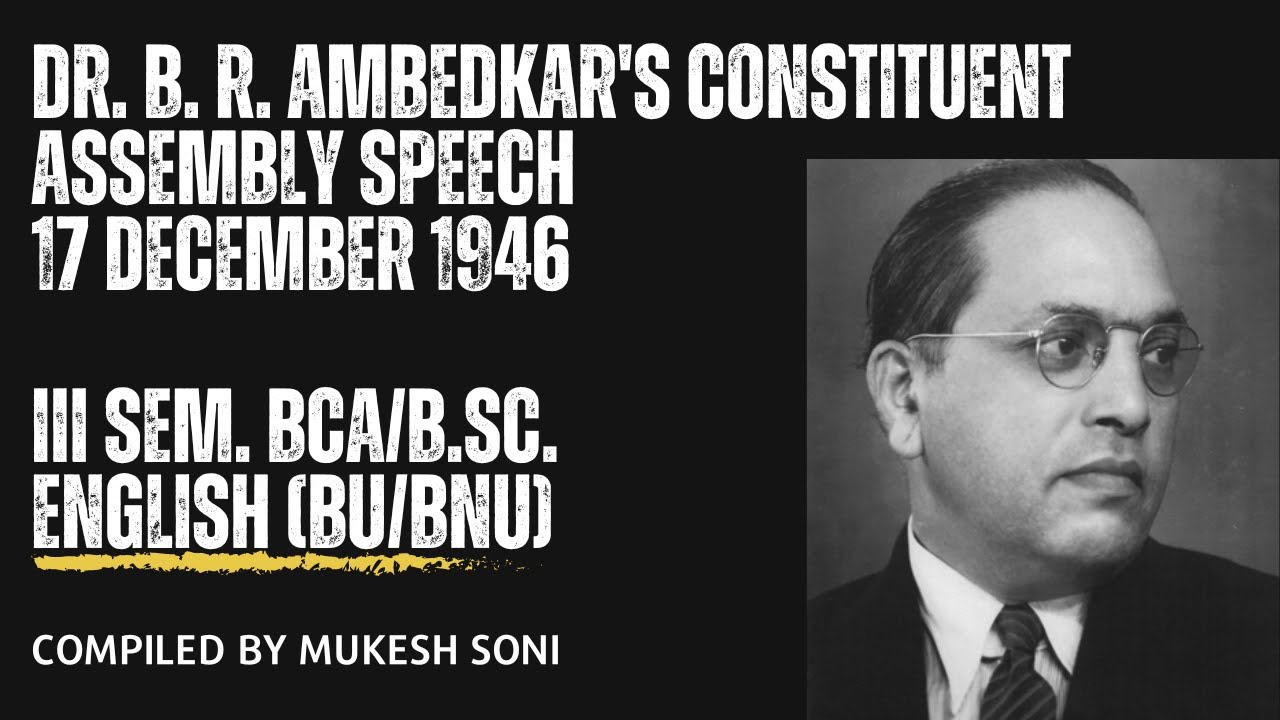
#ambedkarspeech #17december1946#3rdsembscbca #bu #bnu #bcu#university of mysore
5.0 / 5 (0 votes)