Lec-1: Introduction to Data Science & ML | Roadmap to Learn Data Science & ML
Summary
TLDRIn the digital age, data science is crucial for understanding and leveraging data across various platforms. This video outlines the roadmap to becoming a data scientist, emphasizing the importance of grasping business problems, collecting relevant data, cleaning it for accuracy, building predictive models, and extracting actionable insights. Key skills such as SQL, Excel, and fundamental statistics are essential for success in this field. By mastering these concepts and techniques, aspiring data scientists can effectively analyze data and drive business growth, making informed decisions based on solid insights.
Takeaways
- 😀 Data science is becoming increasingly relevant in today's world, with applications in various technologies like smartwatches and mobile apps.
- 📈 The first step to becoming a data scientist is understanding business problems and how data can provide insights to address these challenges.
- 🗂️ Collecting data from multiple sources is crucial, whether it's user behavior on platforms like Netflix or transaction data from apps.
- 🧹 Data cleaning is essential to ensure accuracy, as raw data can often contain errors, outliers, or inconsistencies.
- 🔍 Once data is cleaned, the next step involves building predictive models to analyze and forecast outcomes based on the data.
- 📊 Visualization tools, such as Power BI, can help present data insights effectively, aiding in decision-making processes.
- 🔑 Understanding SQL and having knowledge of statistical concepts are fundamental skills for aspiring data scientists.
- 🚀 Emphasizing the importance of big data, the script notes that traditional tools like Excel may not suffice for handling large datasets.
- 📊 The road to becoming a data scientist involves following structured steps from understanding business needs to data collection, cleaning, modeling, and insight extraction.
- 🎯 Finally, the ultimate goal is to derive actionable insights from data, contributing to business growth and informed decision-making.
Q & A
What is the significance of data in our daily lives according to the transcript?
-The transcript highlights that we are surrounded by data from various devices, such as smartwatches and mobile phones, which track our activities and interactions, generating a large amount of data.
How does the speaker define data science?
-Data science is defined as the process of extracting valuable insights from data, which can inform business decisions and strategies.
What is the first step in the roadmap to becoming a data scientist?
-The first step is to understand the business problem, which involves knowing what the business needs and what kind of data is required to address those needs.
Why is data collection important in data science?
-Data collection is crucial because it allows data scientists to gather relevant information from various sources, which is necessary for analysis and insight generation.
What does the data cleaning process involve?
-Data cleaning involves removing errors, duplicates, and inconsistencies from the collected data to ensure that the analysis is based on accurate and reliable information.
What are the key tools mentioned for data analysis?
-The transcript mentions SQL for database management, Excel for data manipulation, and data visualization tools to present the analysis effectively.
What role does modeling play in data science?
-Modeling is used to analyze the cleaned data and build predictive models, which help in understanding patterns and making forecasts.
How should insights be presented after analysis?
-Insights should be presented through dashboards or reports to inform and support business decisions and strategies.
What basic statistical concepts should a data scientist be familiar with?
-A data scientist should understand basic statistical concepts such as mean, median, standard deviation, and variance.
Why is continuous learning important in the field of data science?
-Continuous learning is important because the field of data science is rapidly evolving with new tools and technologies, and staying updated is essential for adapting to new challenges and improving skills.
Outlines
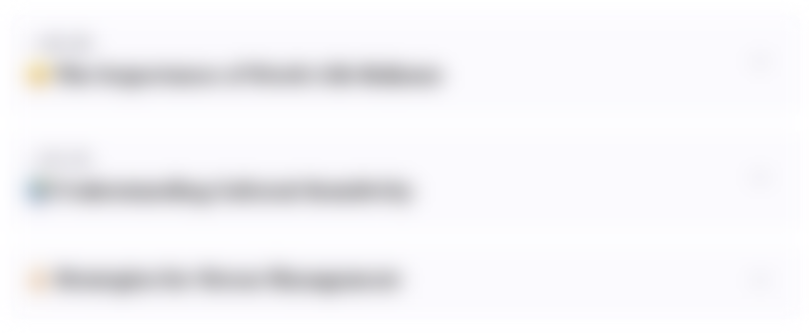
このセクションは有料ユーザー限定です。 アクセスするには、アップグレードをお願いします。
今すぐアップグレードMindmap
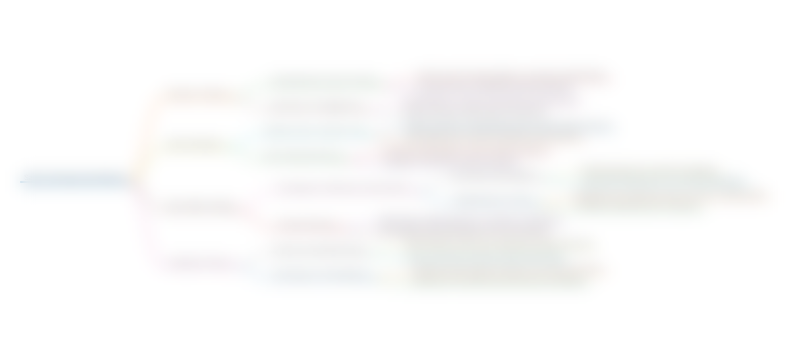
このセクションは有料ユーザー限定です。 アクセスするには、アップグレードをお願いします。
今すぐアップグレードKeywords
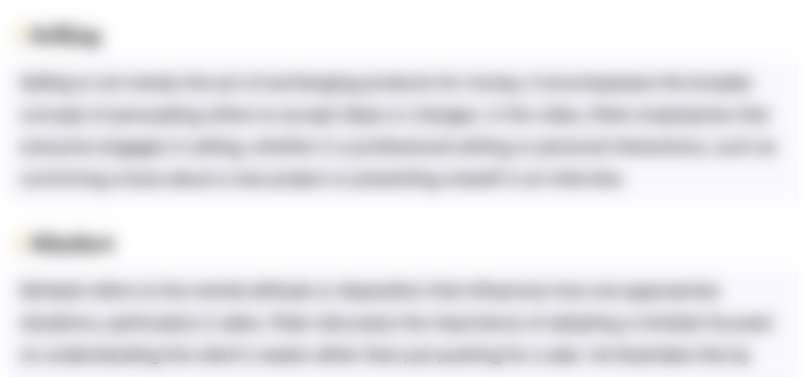
このセクションは有料ユーザー限定です。 アクセスするには、アップグレードをお願いします。
今すぐアップグレードHighlights
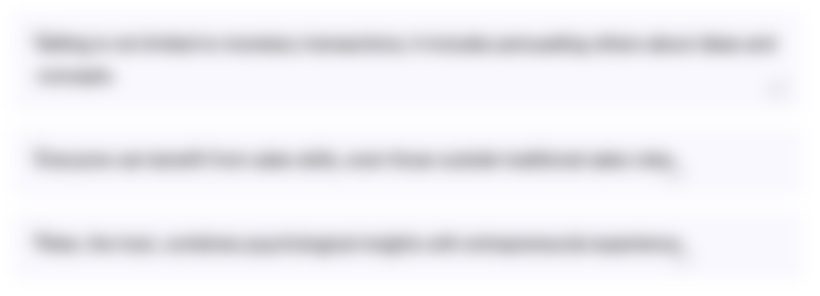
このセクションは有料ユーザー限定です。 アクセスするには、アップグレードをお願いします。
今すぐアップグレードTranscripts
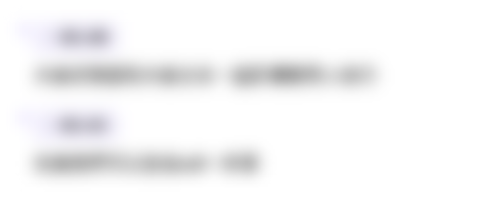
このセクションは有料ユーザー限定です。 アクセスするには、アップグレードをお願いします。
今すぐアップグレード関連動画をさらに表示

04 Privacy and Social Media

Pengantar Sains Data 01 - Pendahuluan Sains Data & Big Data
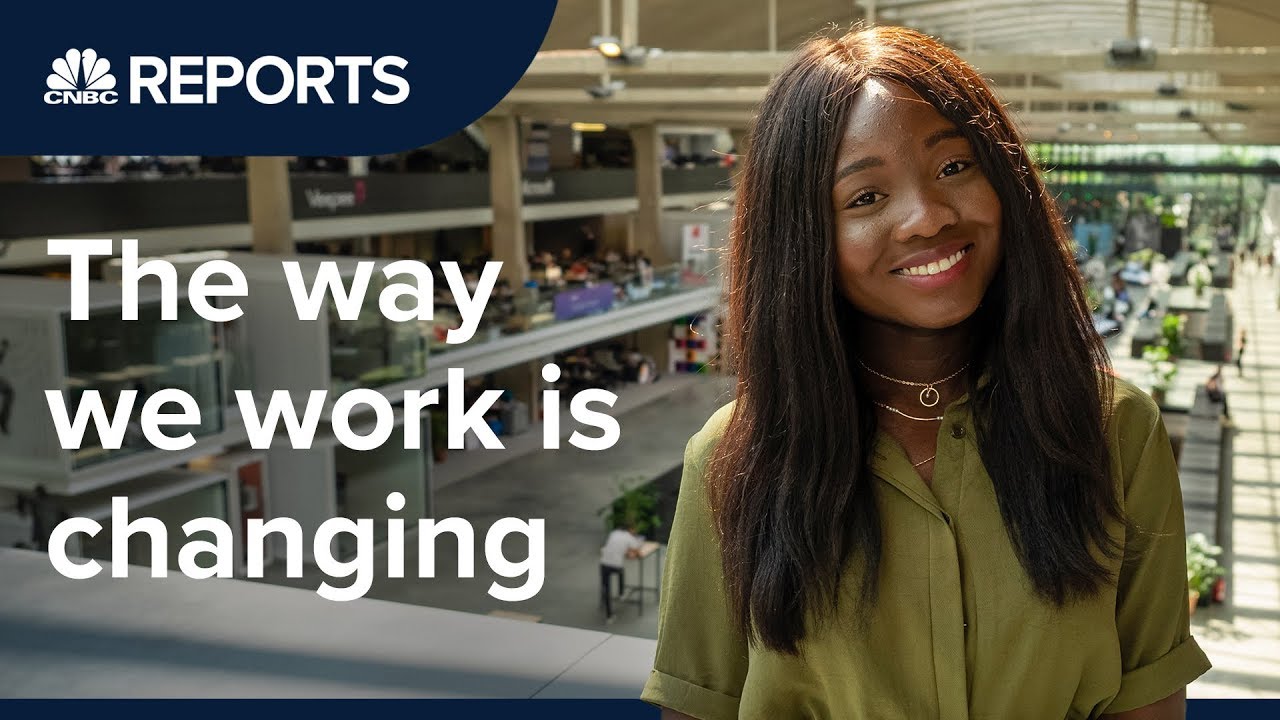
More freedom, less privacy: The digital economy is changing the way we work | CNBC Reports
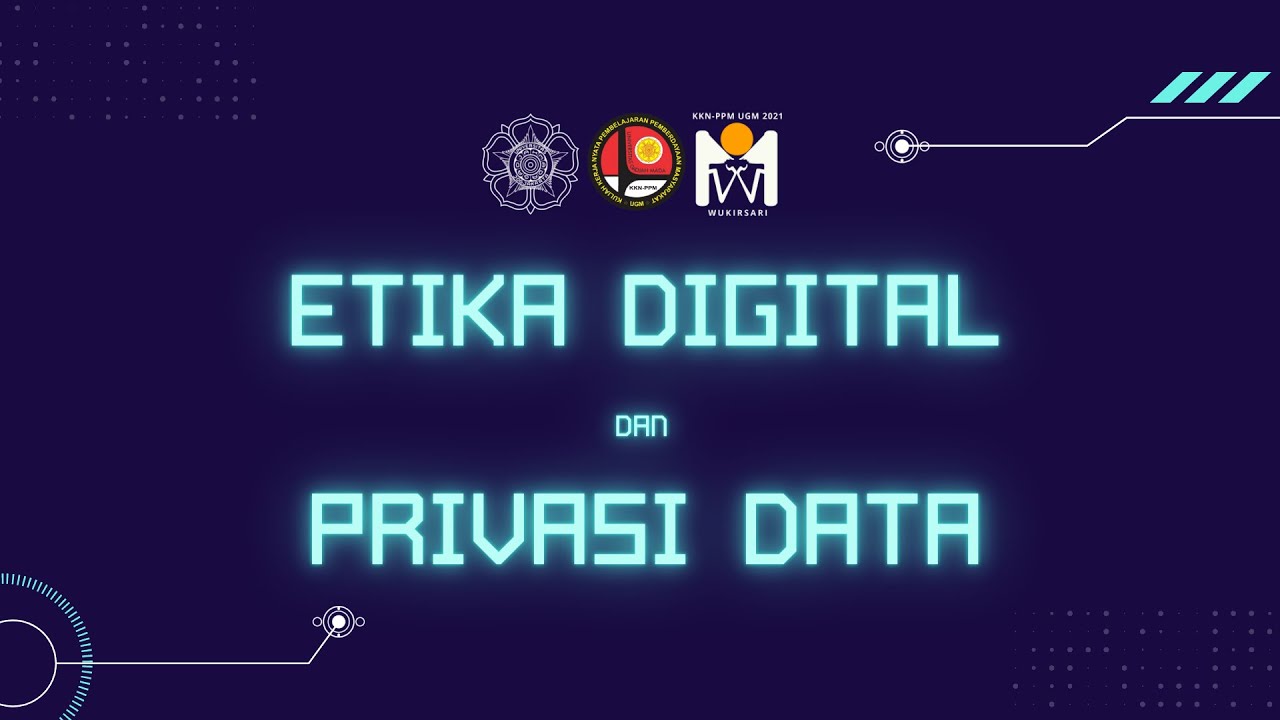
Etika Digital dan Privasi Data

Data Science + Politics & International Relations: Democracy in the Age of Big Data
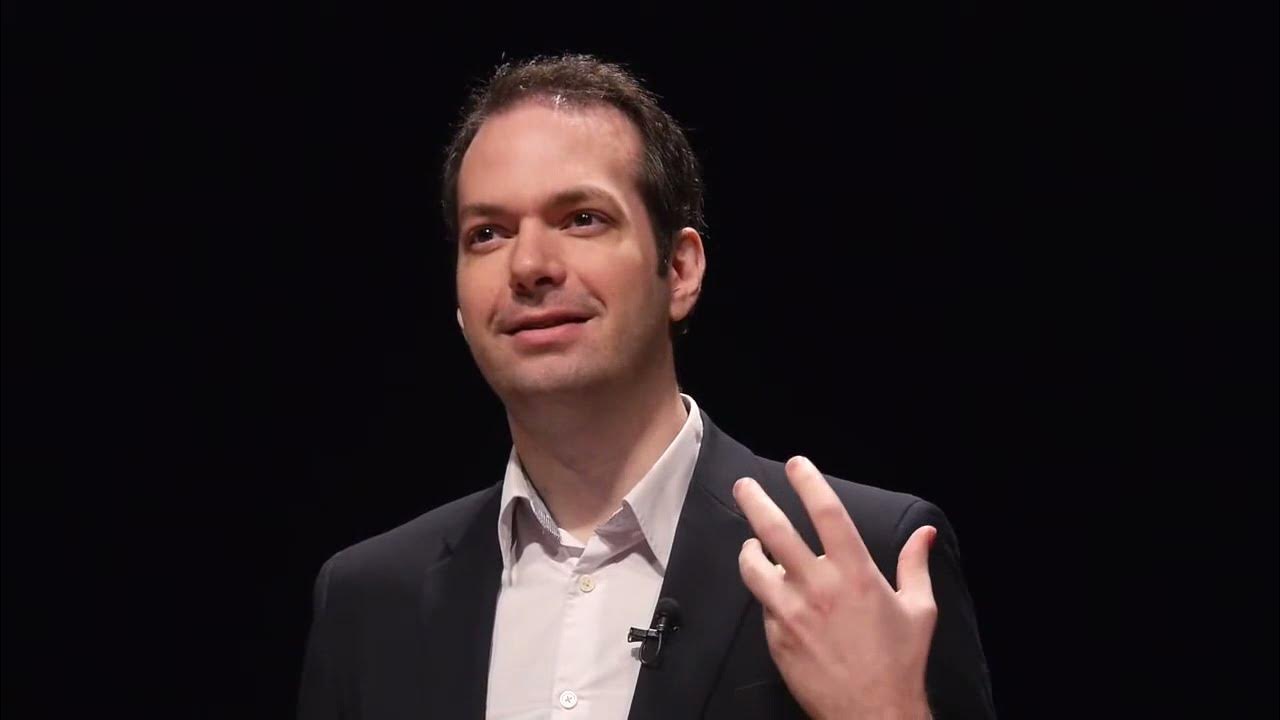
DFS101: 1.1 Introduction to digital forensics
5.0 / 5 (0 votes)