Apple DROPS AI BOMBSHELL: LLMS CANNOT Reason
Summary
TLDRThe video discusses recent research revealing that many AI models, including advanced systems like GPT-4, primarily function as sophisticated pattern matchers rather than true reasoners. This finding highlights a significant reasoning gap, with performance drops observed under slight variations. The speaker emphasizes the need for AI researchers to reevaluate their methodologies, suggesting that scaling data alone may not resolve these issues. Despite the challenges, there is an optimistic outlook that understanding these limitations can lead to effective solutions, encouraging innovative approaches to enhance AI's reasoning capabilities.
Takeaways
- 😀 AI models, especially from Apple's research, primarily function as sophisticated pattern matchers rather than true reasoners.
- 📉 A significant performance drop of 17.5% in reasoning tasks raises concerns about AI's reasoning capabilities.
- 📊 Research from Consequent AI highlights a reasoning gap of 58% to 80% among leading models on static benchmarks.
- 🔍 The findings suggest that simply scaling data and computational resources may not effectively address the reasoning gap.
- 🤔 Apple researchers assert that AI behavior is better explained by fragile pattern matching, which can be easily affected by minor changes.
- 💡 Identifying the reasoning gap is seen as a critical first step in improving AI's capabilities and developing solutions.
- 🧩 Potential solutions may include adjusting how questions are posed to AI models to enhance reasoning performance.
- 📝 The discussion around AI reasoning is evolving, with researchers needing to reassess their approaches based on these findings.
- 📖 Other benchmarks, such as the Simple Bench Reasoning Benchmark, are being explored to further assess AI reasoning capabilities.
- 🚀 The recognition of a significant discrepancy in reasoning ability presents an opportunity for AI developers to innovate and find effective fixes.
Q & A
What was the main focus of the research discussed in the transcript?
-The research focused on the reasoning capabilities of advanced AI models, particularly highlighting their function as pattern matchers rather than true reasoners.
What surprising conclusion did the Apple research reach regarding AI models?
-The research concluded that AI models, despite their sophistication, primarily rely on pattern matching, which may not effectively address reasoning tasks.
What is the 'reasoning gap' mentioned in the discussion?
-The 'reasoning gap' refers to the significant performance disparity observed among state-of-the-art AI models when evaluated on reasoning tasks, with some models showing a gap of 58% to 80%.
How does scaling data impact the performance of AI models according to the findings?
-The findings suggest that merely scaling data or adjusting parameters does not necessarily lead to improved reasoning capabilities and can reveal the fragility of these models.
What did the speaker suggest regarding the overlooked research on AI reasoning?
-The speaker noted that there are existing studies, like one from Consequent AI, that discuss reasoning performance and gaps, which have not received sufficient attention in the AI community.
What potential solutions did the speaker propose to address the reasoning limitations of AI models?
-The speaker proposed exploring new methodologies, such as repeated questioning or other strategies, to enhance the reasoning abilities of AI models.
Why does the speaker view the recognition of AI's reasoning limitations as a positive development?
-The speaker sees it as a positive development because acknowledging the problem provides a clearer direction for researchers to explore solutions and improve AI capabilities.
What type of reasoning problems did the speaker mention in relation to AI benchmarks?
-The speaker referred to benchmarks involving simple reasoning problems that assess models' abilities to handle various reasoning tasks, indicating a need for robust evaluation metrics.
What is the significance of the performance drop mentioned in the transcript?
-The significance of the performance drop, specifically a 17.5% decrease after certain adjustments, emphasizes the fragility of AI models and their reliance on specific data patterns.
How does the speaker encourage audience engagement regarding AI reasoning?
-The speaker encourages audience engagement by inviting feedback and thoughts on their experiences with AI models and their reasoning capabilities, promoting a dialogue about AI research.
Outlines
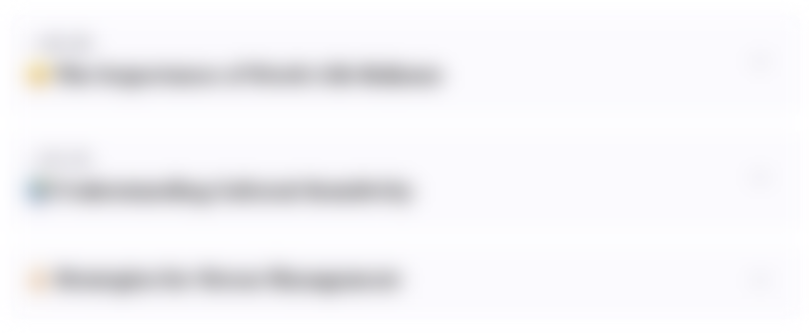
このセクションは有料ユーザー限定です。 アクセスするには、アップグレードをお願いします。
今すぐアップグレードMindmap
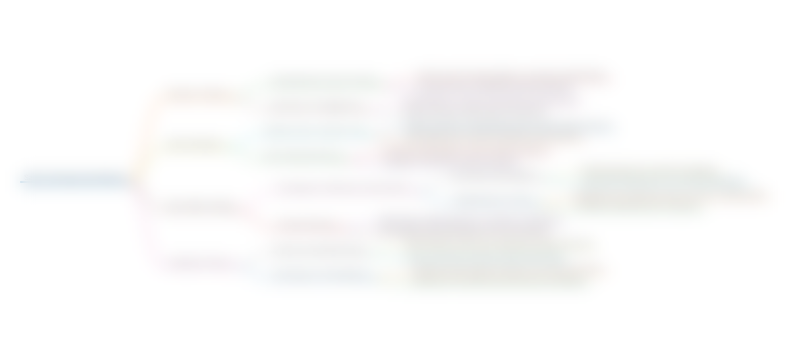
このセクションは有料ユーザー限定です。 アクセスするには、アップグレードをお願いします。
今すぐアップグレードKeywords
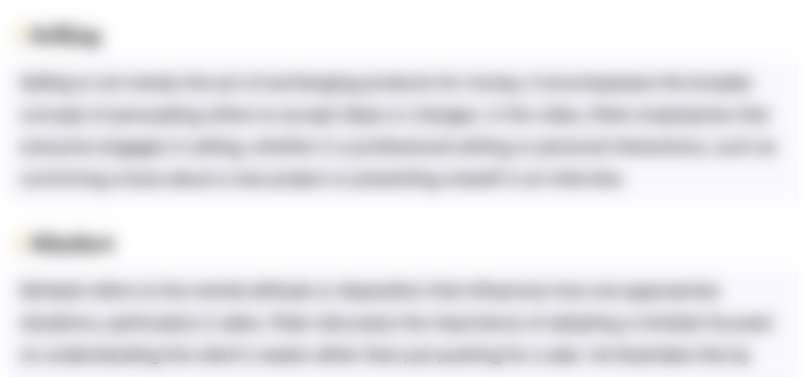
このセクションは有料ユーザー限定です。 アクセスするには、アップグレードをお願いします。
今すぐアップグレードHighlights
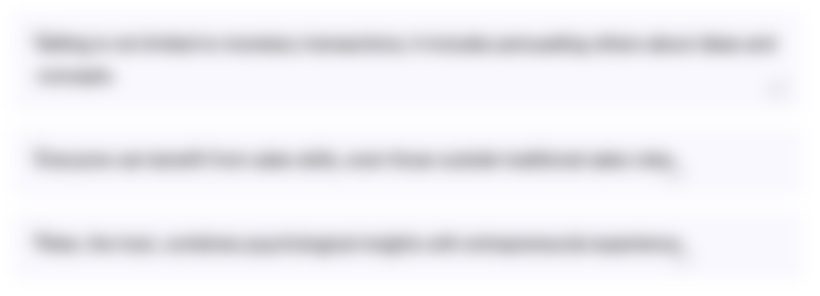
このセクションは有料ユーザー限定です。 アクセスするには、アップグレードをお願いします。
今すぐアップグレードTranscripts
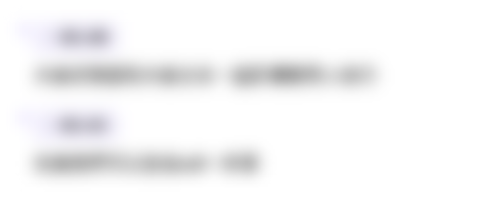
このセクションは有料ユーザー限定です。 アクセスするには、アップグレードをお願いします。
今すぐアップグレード関連動画をさらに表示
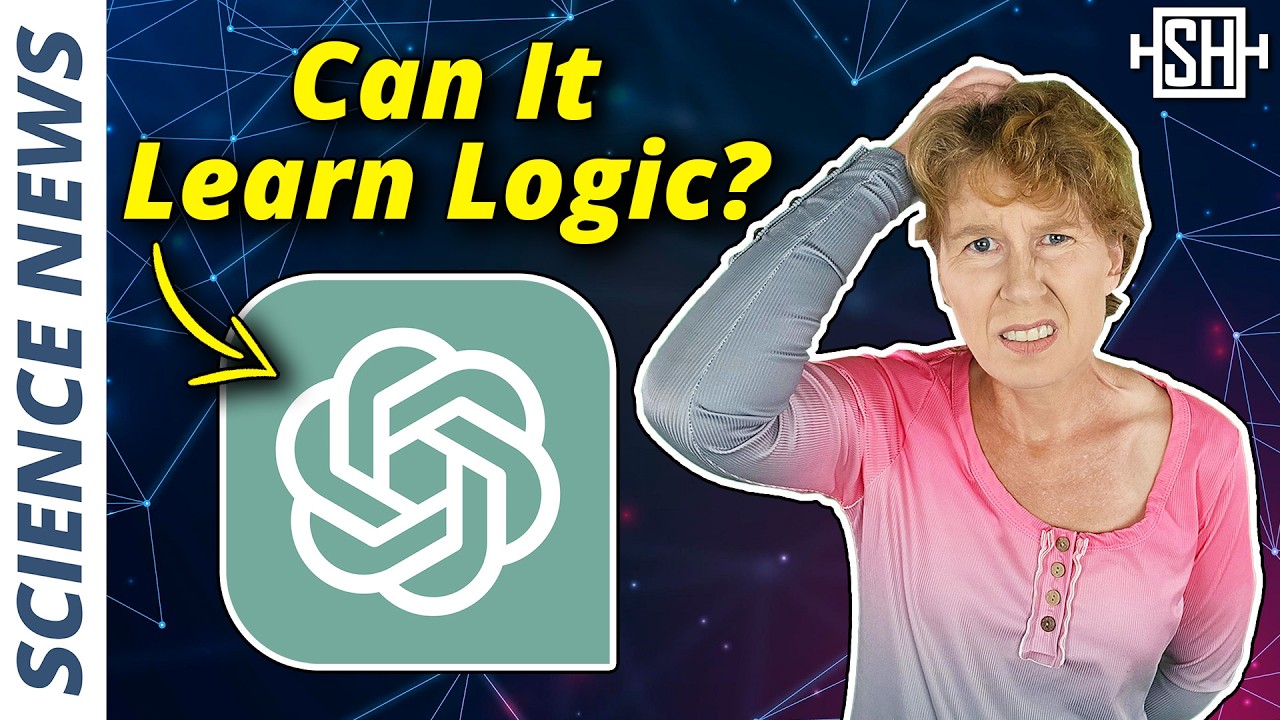
AI Can’t Reason. Should It Drive Cars?
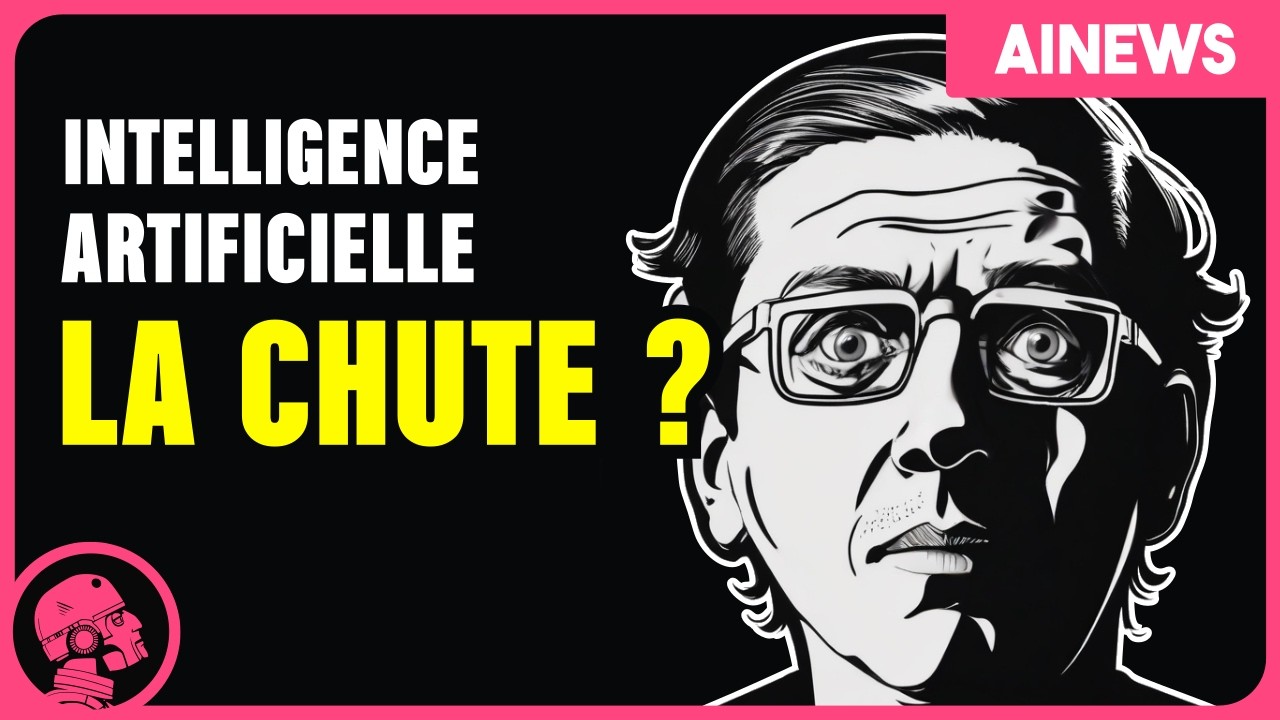
Intelligence Artificielle: la fin du bullshit ? (AI News)
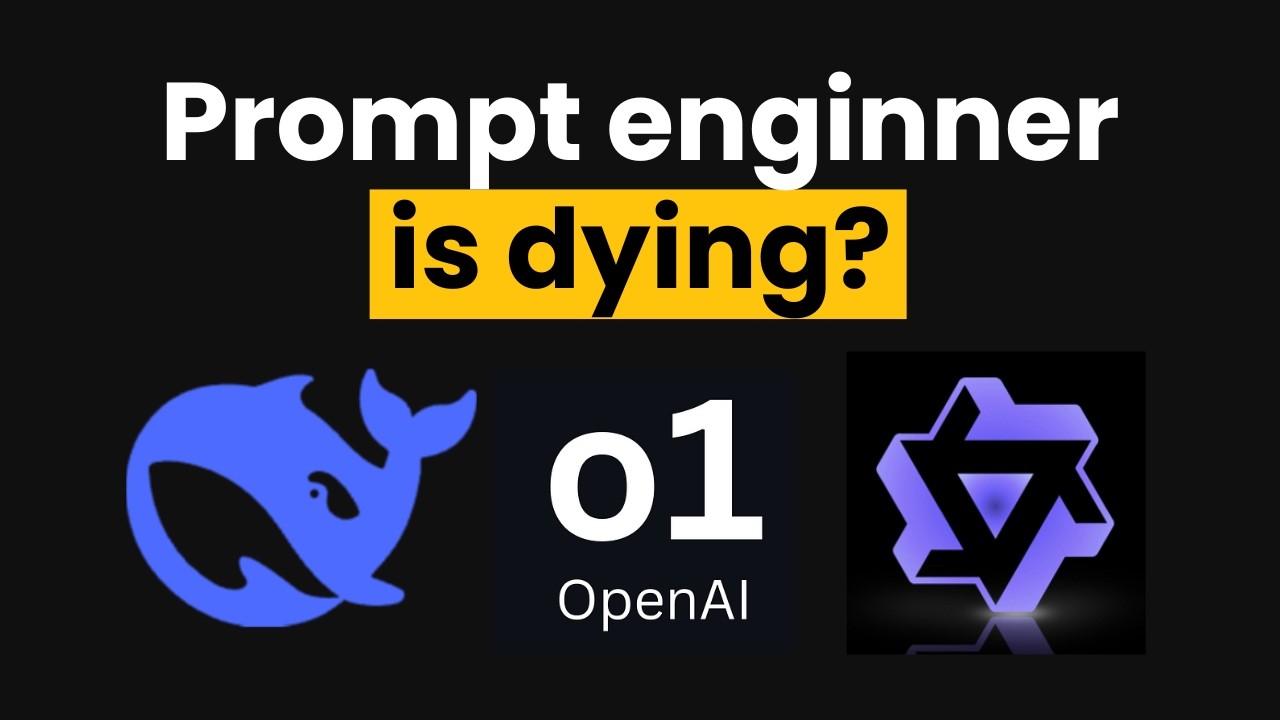
Deepseek R1 - The Era of Reasoning models
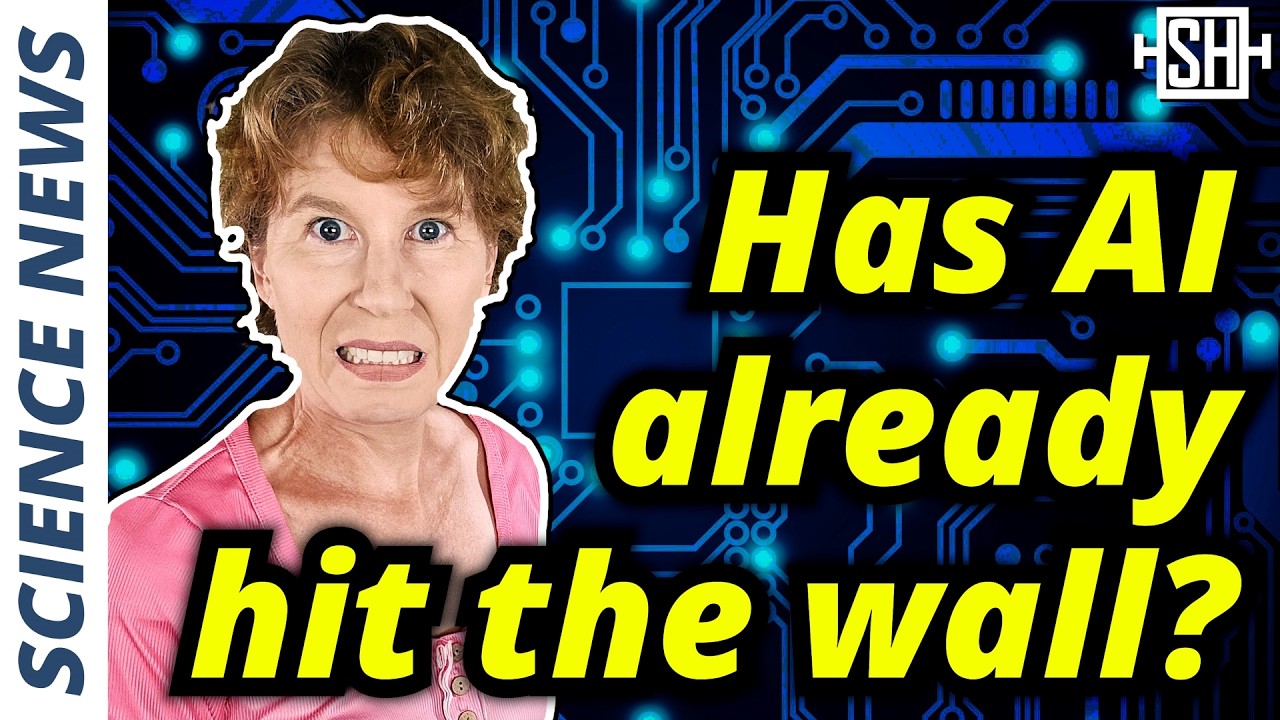
AI Scaling Hits Wall, Rumours Say. How Serious is it?
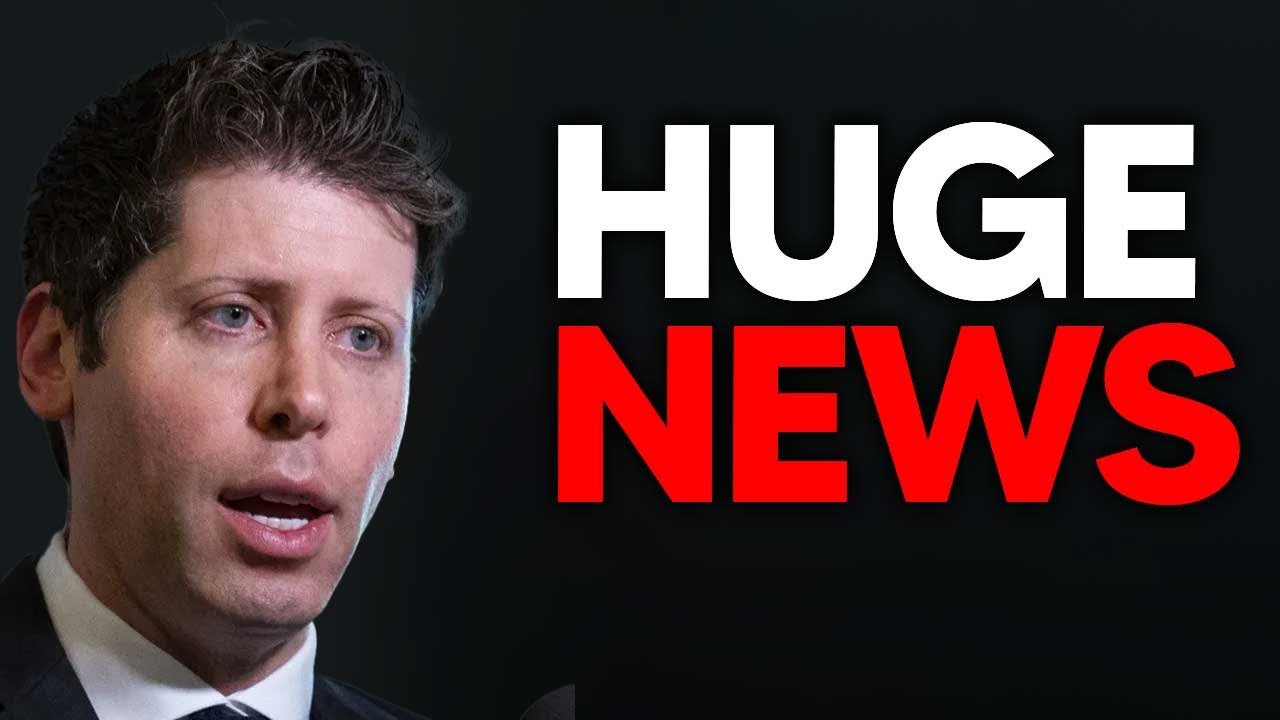
HUGE AI NEWS: AGI Benchmark BROKEN ,OpenAIs Agents Leaked , Automated AI Research And More
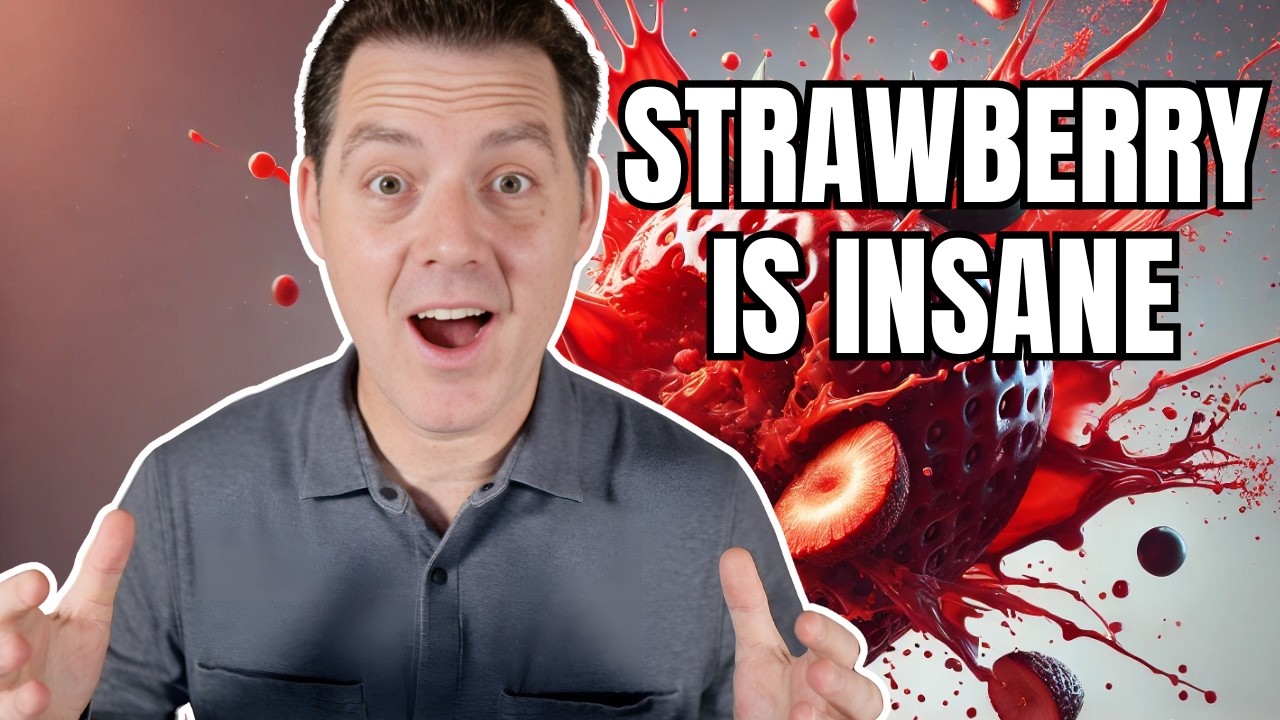
OpenAI Releases GPT Strawberry 🍓 Intelligence Explosion!
5.0 / 5 (0 votes)