BIOL 4330 Unit 2 1 2 Bayesian Analysis and Markov Chain Monte Carlo
Summary
TLDRThe transcript explains Bayesian analysis, particularly its use in phylogenetic studies. It highlights how complex probability distributions are sampled using the Markov Chain Monte Carlo (MCMC) method to identify optimal evolutionary trees. The analogy of searching for the highest peak in a rugged landscape is used to illustrate how random starting points help explore possible outcomes. Bayesian analysis iteratively refines the model of evolution and parameters, improving accuracy while being computationally efficient. The process is compared with other methods like maximum likelihood and parsimony, emphasizing Bayesian analysis' speed and ability to provide support values.
Takeaways
- 📊 Statistical analysis requires understanding the distribution of outcomes, which can be simple or complex depending on the population.
- 🌳 For human height, a normal distribution is suitable, but phylogenetic analysis involves more complex, multi-dimensional probability distributions.
- 🔍 Markov Chain Monte Carlo (MCMC) is used to estimate complex probability distributions by simulating random walks through the search space.
- 🗺 The MCMC process starts with a random point and explores nearby areas, using an algorithm to move towards better scoring areas, akin to finding the highest peak in a rugged landscape.
- 📈 The process involves running the MCMC, pausing to re-estimate parameters, and repeating until a plateau of scores is reached, indicating the best overall solution.
- 🌐 Phylogenetic search space is multi-dimensional and large, requiring extensive sampling to ensure the best solutions are found.
- 🔄 The Bayesian analysis involves an iterative process of estimating parameters, running MCMC, and re-estimating parameters until convergence.
- 🌲 The 'burn-in' period discards initial trees with lower scores, focusing on saving trees with high scores that represent the best solutions.
- 📊 A consensus tree is made from the saved trees, with support values indicating how often certain relationships appear, providing a measure of confidence in the phylogeny.
- 🤔 Bayesian analysis is favored for its computational efficiency and ability to provide support measures, although other methods like maximum likelihood and parsimony are also discussed.
Q & A
What is the primary purpose of using statistical analysis in phylogenetic studies?
-The primary purpose is to estimate the probability distribution of phylogenetic outcomes due to the complexity of phylogenies and the vast number of possible trees. Statistical analysis helps in identifying the most likely phylogenetic trees that represent evolutionary relationships.
Why are normal distributions insufficient for phylogenetic studies?
-Normal distributions are insufficient for phylogenetic studies because phylogenies involve complex, multidimensional probability distributions, unlike simpler distributions like human height, which follow a normal distribution.
What is the Markov Chain Monte Carlo (MCMC) method, and why is it useful in phylogenetics?
-MCMC is a statistical approach used to estimate probability distributions by sampling nearby regions in a complex search space. In phylogenetics, it helps simulate and estimate the likelihood of various phylogenetic trees and outcomes, even in highly rugged topologies with multiple local maxima.
How does the analogy of searching for the highest peak relate to phylogenetic tree optimization?
-The analogy compares phylogenetic search space to a landscape with peaks. In MCMC, a random starting point is selected, and the algorithm explores surrounding areas to find the highest peak, much like trying to optimize a phylogenetic tree by improving its score step-by-step.
What is the 'burn-in period' in Bayesian phylogenetic analysis?
-The burn-in period refers to the early stage of MCMC sampling where the trees with lower scores are discarded as the algorithm converges on better-scoring phylogenetic trees. During this phase, poorer solutions are filtered out.
How do Bayesian analyses differ from maximum likelihood and parsimony analyses?
-Bayesian analyses not only search for the best trees based on a model of evolution but also provide a posterior probability for relationships, giving a measure of support for different parts of the tree. Maximum likelihood and parsimony do not automatically provide this support for relationships.
What is the role of the model of evolution in Bayesian phylogenetic analysis?
-The model of evolution provides the framework for estimating the likelihood of different phylogenetic trees. Bayesian analysis refines the parameters of this model iteratively to improve tree scores, ultimately producing a tree that best fits the model.
Why is it important to re-estimate parameters during the Bayesian analysis?
-Re-estimating parameters during Bayesian analysis allows for the adjustment of the model of evolution to better fit the data. This iterative process helps in converging toward the best phylogenetic tree with improved accuracy.
What does it mean when the analysis reaches a plateau phase?
-The plateau phase occurs when repeated iterations of the Bayesian analysis no longer improve the tree scores, indicating that the best solutions have been found, and further iterations will not lead to better trees.
How does a consensus tree in Bayesian analysis provide support values for relationships?
-In Bayesian analysis, after saving the trees with good scores, a consensus tree is created by comparing all saved trees. Relationships found in most trees are given higher support values, while those found in fewer trees are given lower values or collapsed into polytomies.
Outlines
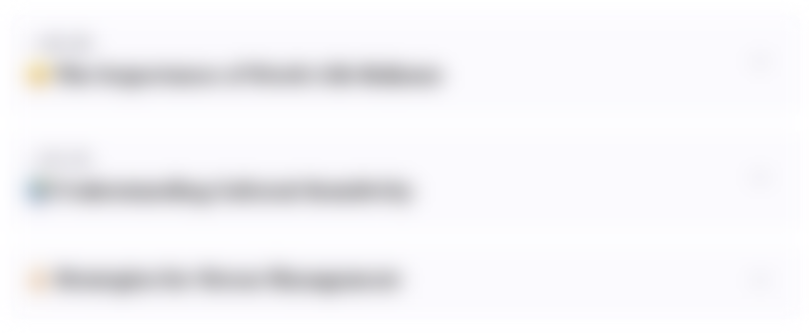
このセクションは有料ユーザー限定です。 アクセスするには、アップグレードをお願いします。
今すぐアップグレードMindmap
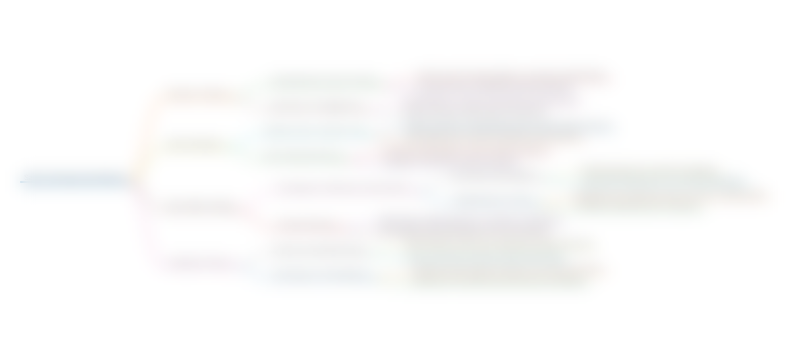
このセクションは有料ユーザー限定です。 アクセスするには、アップグレードをお願いします。
今すぐアップグレードKeywords
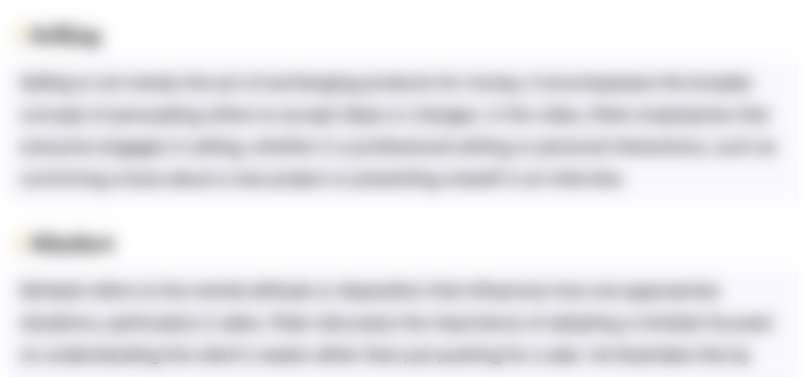
このセクションは有料ユーザー限定です。 アクセスするには、アップグレードをお願いします。
今すぐアップグレードHighlights
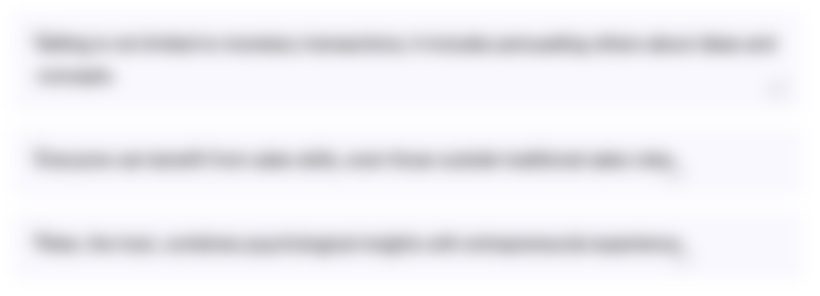
このセクションは有料ユーザー限定です。 アクセスするには、アップグレードをお願いします。
今すぐアップグレードTranscripts
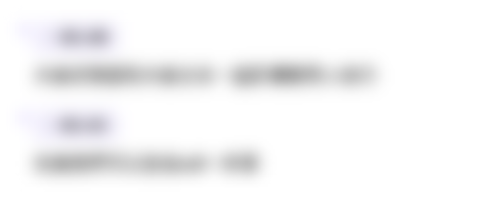
このセクションは有料ユーザー限定です。 アクセスするには、アップグレードをお願いします。
今すぐアップグレード関連動画をさらに表示
5.0 / 5 (0 votes)