Mean and variance of Bernoulli distribution example | Probability and Statistics | Khan Academy
Summary
TLDRThe video discusses a simple example of a Bernoulli distribution, where a population is surveyed for their opinion on the president. Respondents can either give a favorable or unfavorable rating, and the mean and variance of this discrete probability distribution are calculated. The example demonstrates how to find the expected value and variance using probability-weighted sums, despite the expected value not being a possible outcome. It concludes by introducing general formulas for the mean, variance, and standard deviation in a Bernoulli distribution, setting the stage for further exploration of the binomial distribution.
Takeaways
- 📊 The speaker conducts a full survey of a population's opinion on the president, offering two possible responses: favorable or unfavorable.
- 🎯 The probability distribution is discrete with two outcomes: 40% have an unfavorable view, and 60% have a favorable view.
- 📈 The expected value (mean) of the distribution is calculated by assigning 0 to unfavorable (u) and 1 to favorable (f) views.
- 🔢 The expected value of the distribution is 0.6, which represents a probability-weighted sum of the two options.
- 🙅 No individual can have an actual value of 0.6; individuals will either have a favorable or unfavorable rating (0 or 1).
- 💡 The variance of the population is the probability-weighted sum of the squared distances from the mean.
- 🔍 Variance is calculated using the differences between each outcome (0 or 1) and the mean (0.6), resulting in a variance of 0.24.
- 📐 The standard deviation is the square root of the variance, which in this case is approximately 0.49.
- 🧠 The distribution is skewed to the right, with most individuals having a favorable view.
- 📚 This specific example introduces the Bernoulli Distribution, a special case of the binomial distribution, which is further explained in future discussions.
Q & A
What is the purpose of surveying every single member of a population in this scenario?
-The purpose is to gather data on the favorability rating of the president, with the aim of understanding the distribution of opinions within the population.
What are the two options available for the survey respondents?
-The respondents can either have an unfavorable rating or a favorable rating for the president.
What percentage of the population had an unfavorable rating according to the survey?
-According to the survey, 40% of the population had an unfavorable rating.
What percentage of the population had a favorable rating?
-60% of the population had a favorable rating.
How is the probability distribution represented in this scenario?
-The probability distribution is represented as a discrete distribution with two values: unfavorable (0) and favorable (1).
What is the expected favorability rating of a randomly picked member of the population?
-The expected favorability rating is the mean of the distribution, which is calculated as 0.4 * 0 + 0.6 * 1 = 0.6.
Why is the mean of 0.6 not a value that an individual can actually take on?
-The mean of 0.6 is not a value that an individual can take on because each person must choose either a favorable or unfavorable rating, which are represented as 1 or 0, respectively.
How is the variance of the distribution calculated?
-The variance is calculated as the probability-weighted sum of the squared distances from the mean. In this case, it is 0.4 * (0 - 0.6)^2 + 0.6 * (1 - 0.6)^2 = 0.24.
What is the standard deviation of this distribution?
-The standard deviation is the square root of the variance, which is approximately 0.49.
What does the distribution's skew to the right indicate?
-The skew to the right indicates that the distribution is not symmetric and that there is a higher concentration of favorable ratings.
What is the Bernoulli Distribution mentioned in the script?
-The Bernoulli Distribution is a discrete probability distribution that takes value 1 with success probability p and value 0 with failure probability q = 1 - p. It is the simplest case of the binomial distribution.
Outlines
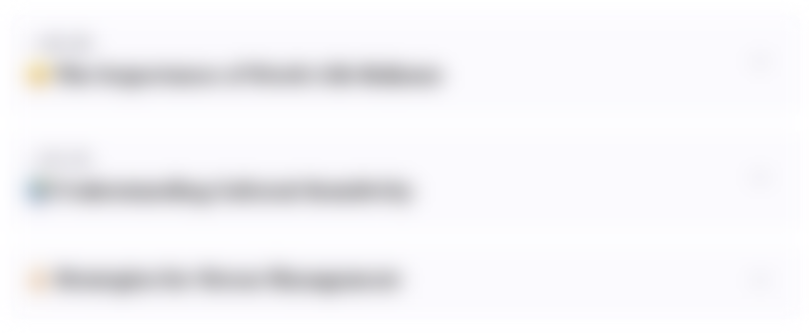
このセクションは有料ユーザー限定です。 アクセスするには、アップグレードをお願いします。
今すぐアップグレードMindmap
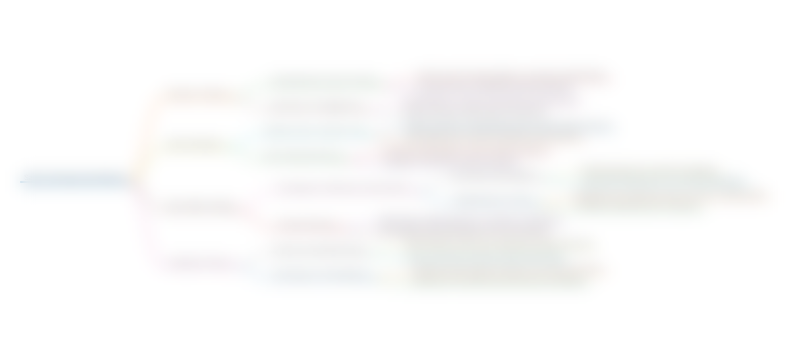
このセクションは有料ユーザー限定です。 アクセスするには、アップグレードをお願いします。
今すぐアップグレードKeywords
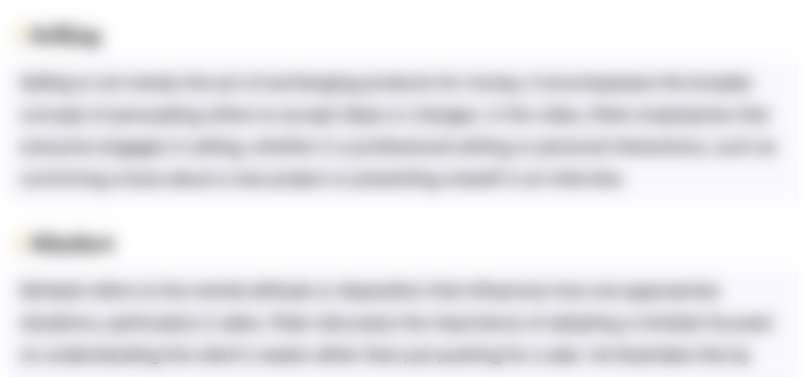
このセクションは有料ユーザー限定です。 アクセスするには、アップグレードをお願いします。
今すぐアップグレードHighlights
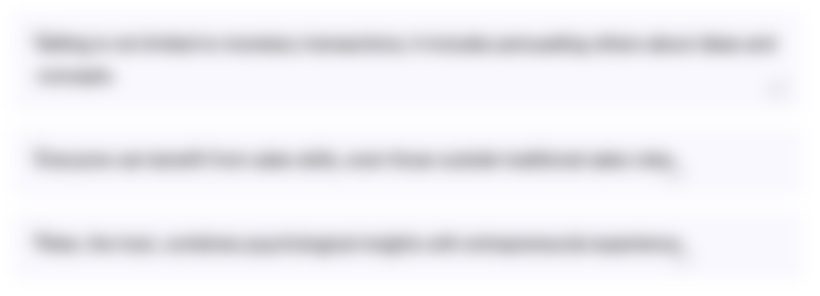
このセクションは有料ユーザー限定です。 アクセスするには、アップグレードをお願いします。
今すぐアップグレードTranscripts
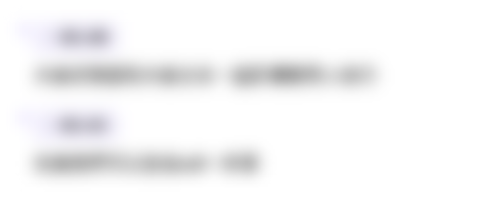
このセクションは有料ユーザー限定です。 アクセスするには、アップグレードをお願いします。
今すぐアップグレード関連動画をさらに表示
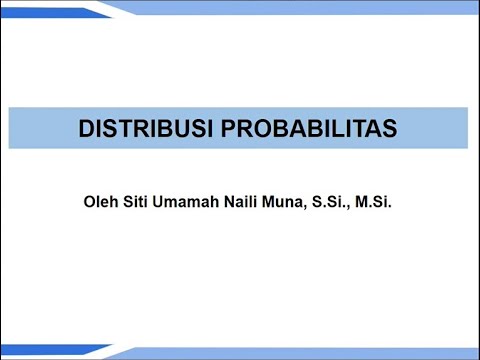
Pertemuan 1 - Distribusi Probabilitas (Part 2)
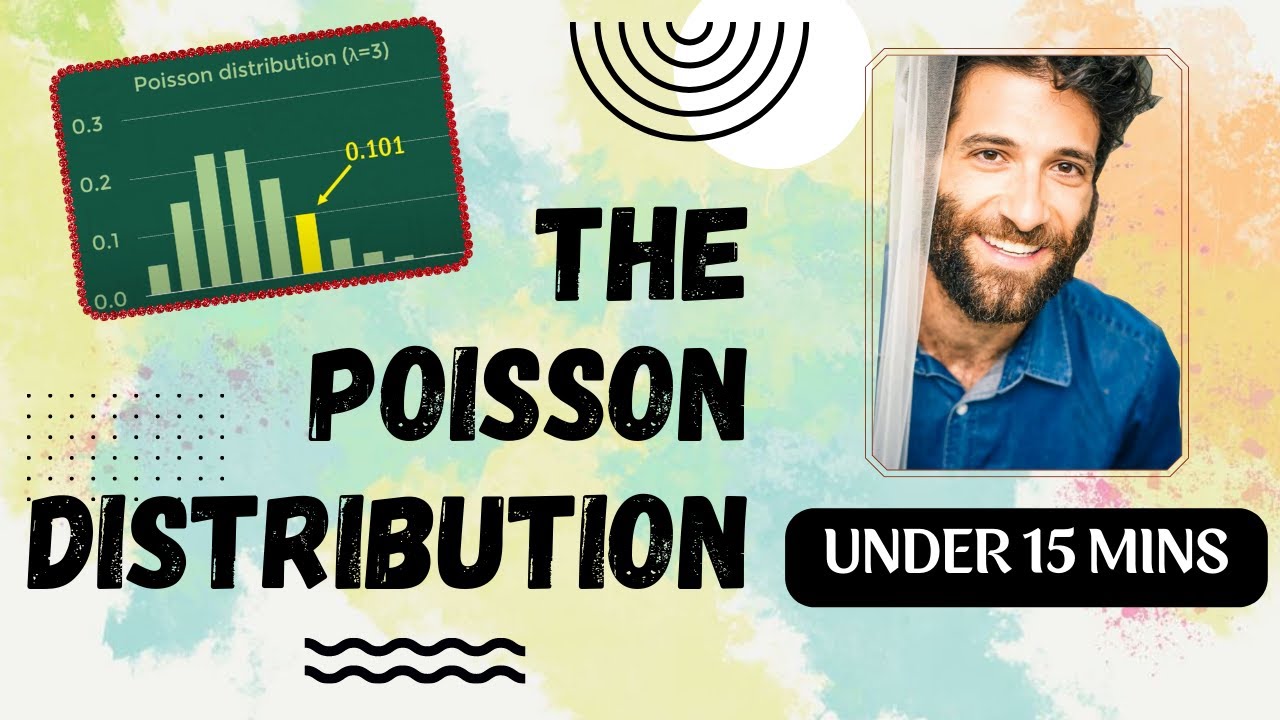
Poisson Distribution EXPLAINED in UNDER 15 MINUTES!
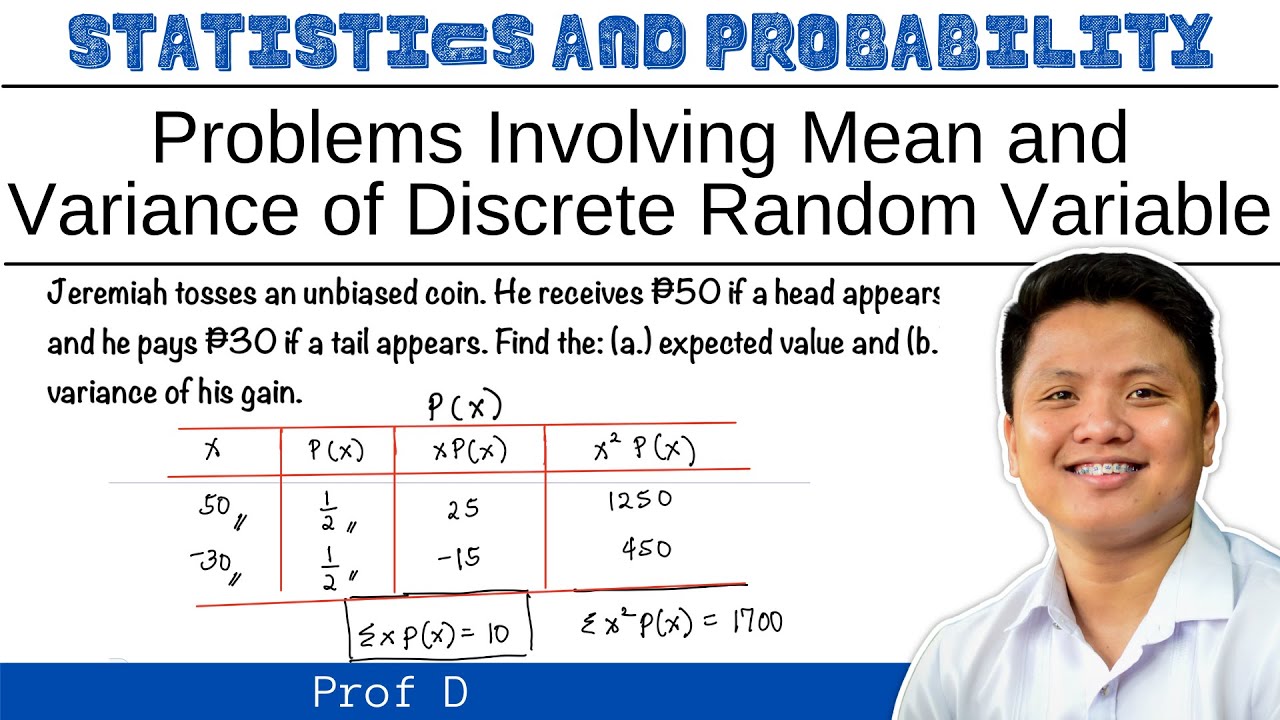
Problems Involving Mean and Variance of Discrete Random Variable | @ProfD

Repeated independent trial and Bernoulli distribution

Lecture 10.1 - Binomial distribution - Bernoulli distribution

Confidence Interval [Simply explained]
5.0 / 5 (0 votes)