Nassim Taleb on prediction, probability, and what Mandelbrot taught him about forecasting
Summary
TLDRThe speaker, an experienced option trader, critiques super forecasting, emphasizing the naivety of binary versus continuous payoffs. He explains the paradox that out-of-the-money options decrease in value as tail risks increase, contrary to common intuition. The speaker also discusses the impracticality of forecasting, highlighting the unpredictability of extreme events and the folly of point forecasts in fat-tailed distributions. He argues that forecasting should consider the payoff function and the real-world consequences of predictions, not just the probability of an event.
Takeaways
- 📊 **Binary vs. Continuous Payoffs**: The speaker explains the difference between binary and continuous payoffs, noting that binary options decrease in value as tails fatten due to their probability nature.
- 📉 **Impact of Fat Tails**: Discusses how fat tails affect the value of options, where the probability distribution is altered by extreme events, leading to a misunderstanding of risk by non-experts.
- 🔢 **Standard Deviation in Extreme Events**: Highlights the concept that there's no standard deviation in extreme events, emphasizing the unpredictability and non-linearity of outcomes in such cases.
- 🌐 **Scale-Free Distribution**: Explains the idea of scale-free distribution, where the ratio of events does not change significantly, but the consequences can vary greatly.
- 📈 **Exponential Growth and Error Margins**: Points out that small errors in growth rates can lead to vastly different outcomes, especially when considering exponential functions.
- 🚫 **Limitations of Point Forecasting**: Critiques the practice of point forecasting for fat-tailed variables, arguing it's inadequate due to the unpredictability of extreme outcomes.
- 💵 **Wealth and Forecasting Ability**: Suggests that those who are good at forecasting are not necessarily wealthy, implying that forecasting skill does not directly translate to financial success.
- 📝 **Insight from Mandelbrot**: References the influence of Benoit Mandelbrot on the understanding of fat tails and the importance of characteristic scales in forecasting.
- 🌐 **Pandemic Forecasting**: Discusses the challenges of forecasting pandemic-related deaths, illustrating the difficulty of estimating outcomes in fat-tailed distributions.
- 💭 **Conceptual Framework**: Emphasizes the need for a framework that understands the nature of probability and payoffs, rather than just focusing on binary outcomes.
Q & A
What is the main substantive disagreement between the speaker and the superforecasting project?
-The main disagreement revolves around binary versus continuous payoffs. The speaker argues that superforecasting relies too heavily on binary events (yes/no outcomes), whereas real-world outcomes, especially in complex systems, have continuous payoffs that can't be reduced to simple binary decisions.
How does the speaker use options trading to explain the difference between binary and continuous payoffs?
-The speaker, drawing from experience in options trading, explains that in naive thinking, one might believe that a binary option increases in value when the probability of a rare event (fat tail) increases. In reality, binary options may decrease in value as the likelihood of extreme outcomes shifts, showing the flaw in using binary approaches for complex events.
Why does the speaker criticize the use of standard deviation in extreme events?
-The speaker, citing the work of Benoit Mandelbrot, argues that there is no standard deviation in extreme events, or 'extremistan,' because large deviations do not behave predictably. This makes the concept of a typical event irrelevant in such scenarios, where tail risks dominate.
How does the speaker differentiate between making forecasts in binary versus real-world scenarios?
-In binary forecasting, the outcome is limited to yes/no or win/lose, but in real-world scenarios, especially in markets or events with fat tails, the payoff is continuous and potentially large. Therefore, forecasting in binary terms fails to capture the magnitude of outcomes in fat-tailed environments.
What does the speaker mean by 'payoff space' when discussing forecasting?
-Payoff space refers to the potential rewards or losses associated with an event, which often have a continuous range in real-world scenarios. The speaker contrasts this with binary outcomes, where the value of a prediction is either correct or incorrect, without considering the scale of consequences.
What is the speaker's view on the importance of rare, extreme events in forecasting?
-The speaker emphasizes that rare, extreme events—often ignored in traditional forecasting—are where the most significant impact lies. He argues that superforecasting tends to focus on smaller, more predictable events, missing the consequences of large-scale, fat-tailed phenomena.
Why does the speaker mention the example of a war that kills two people versus one that kills 600,000?
-The speaker uses this example to highlight the flaw in binary forecasting. A 'war' can vary dramatically in its impact, and forecasting whether an event will occur without considering the magnitude of its consequences misses the crucial part of what matters in real-world outcomes.
How does the speaker describe the limitations of traditional risk assessment methods like Value at Risk (VaR)?
-The speaker criticizes traditional risk assessment methods like VaR because they often fail to account for extreme outcomes. VaR may tell you that a loss of a certain magnitude is unlikely, but it doesn't account for the potentially catastrophic losses that can occur in the tail events, which is where most of the risk lies.
What is the speaker's criticism of point forecasting for fat-tailed variables?
-The speaker criticizes point forecasting (predicting a specific outcome) for fat-tailed variables because it fails to capture the unpredictable, extreme variations in these distributions. Point forecasts may miss the larger, more impactful outcomes that are crucial in fat-tailed environments.
Why does the speaker argue that superforecasters are not successful in making money from their forecasts?
-The speaker argues that superforecasters may be good at predicting outcomes but are not successful in monetizing those predictions. In financial markets or other real-world applications, the payoff is not just about being right but about being right in a way that generates substantial rewards, which superforecasters haven't demonstrated.
Outlines
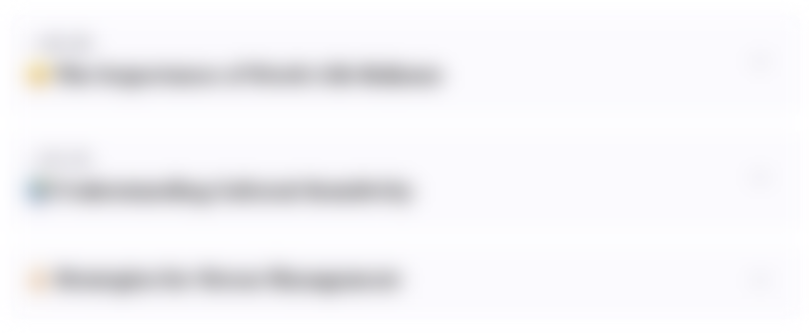
このセクションは有料ユーザー限定です。 アクセスするには、アップグレードをお願いします。
今すぐアップグレードMindmap
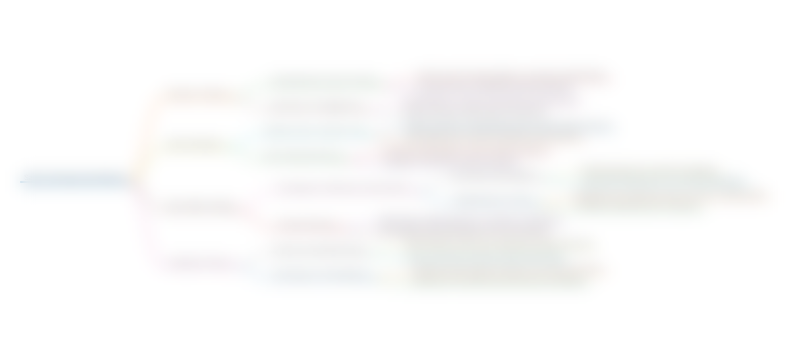
このセクションは有料ユーザー限定です。 アクセスするには、アップグレードをお願いします。
今すぐアップグレードKeywords
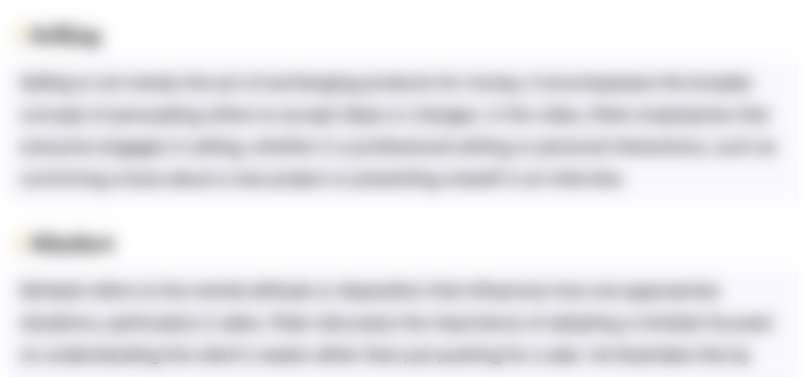
このセクションは有料ユーザー限定です。 アクセスするには、アップグレードをお願いします。
今すぐアップグレードHighlights
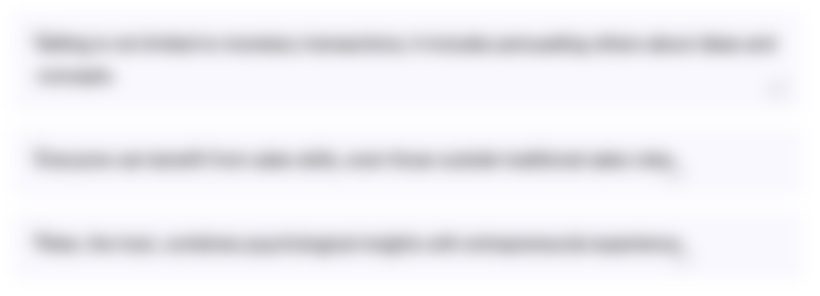
このセクションは有料ユーザー限定です。 アクセスするには、アップグレードをお願いします。
今すぐアップグレードTranscripts
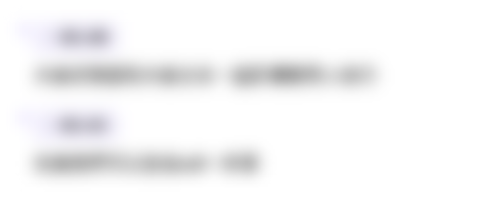
このセクションは有料ユーザー限定です。 アクセスするには、アップグレードをお願いします。
今すぐアップグレード関連動画をさらに表示
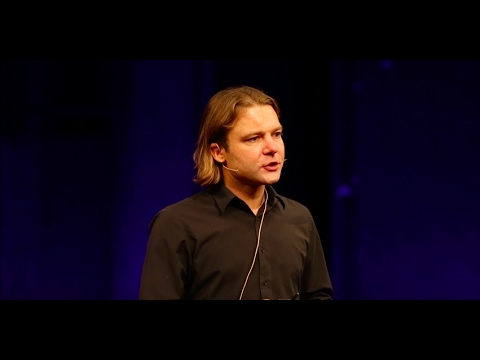
How to predict an election | Andreas Graefe | TEDxMünchen
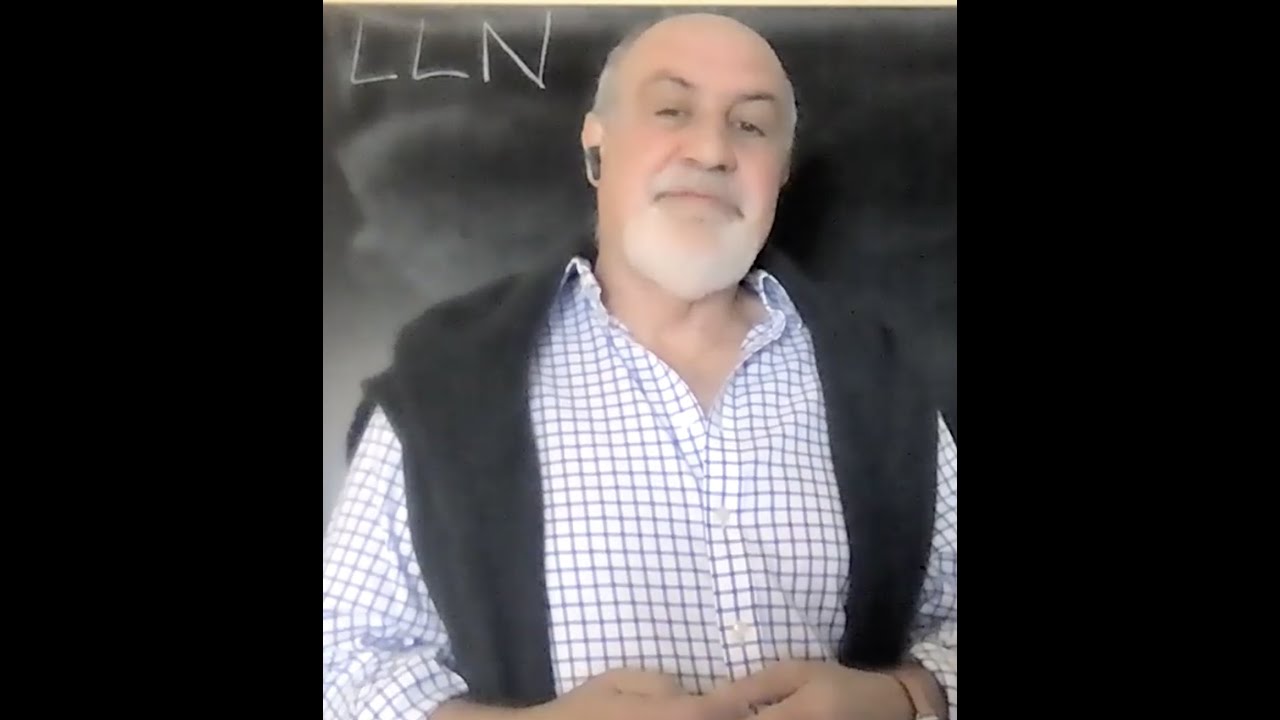
MINI-LESSON 3: The Law of Large Numbers. A very intuitive introduction.
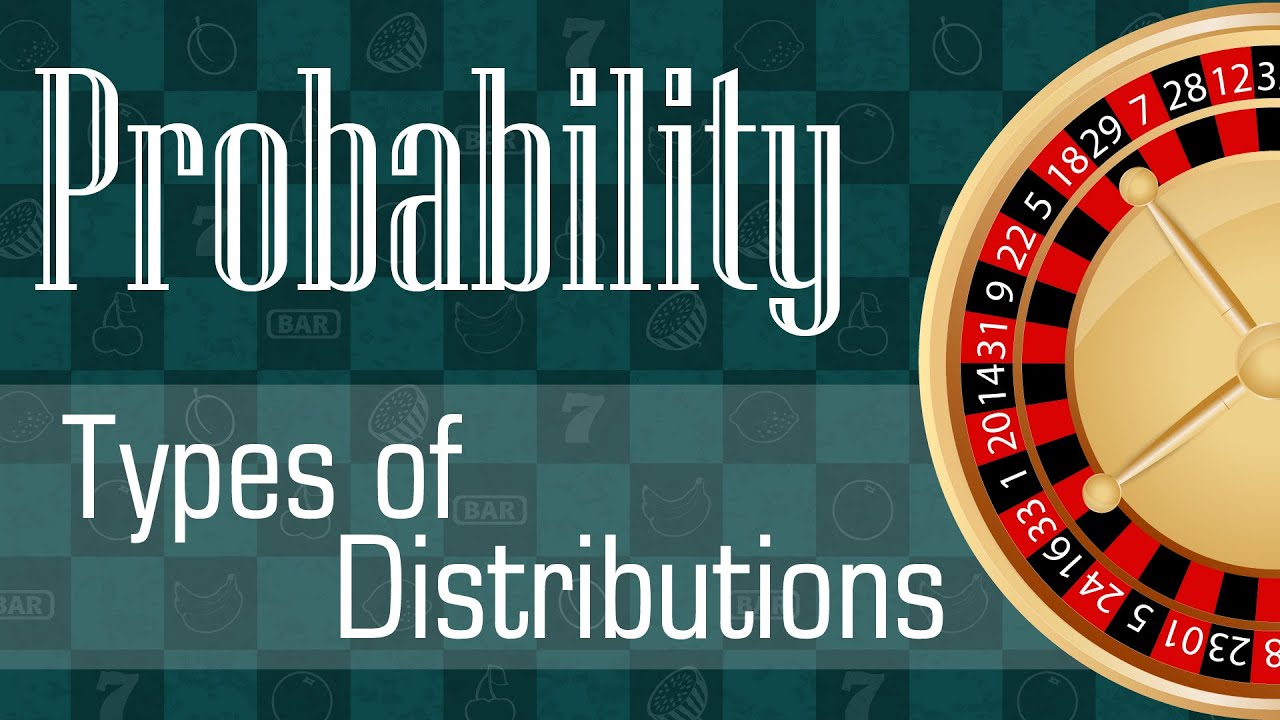
Probability: Types of Distributions
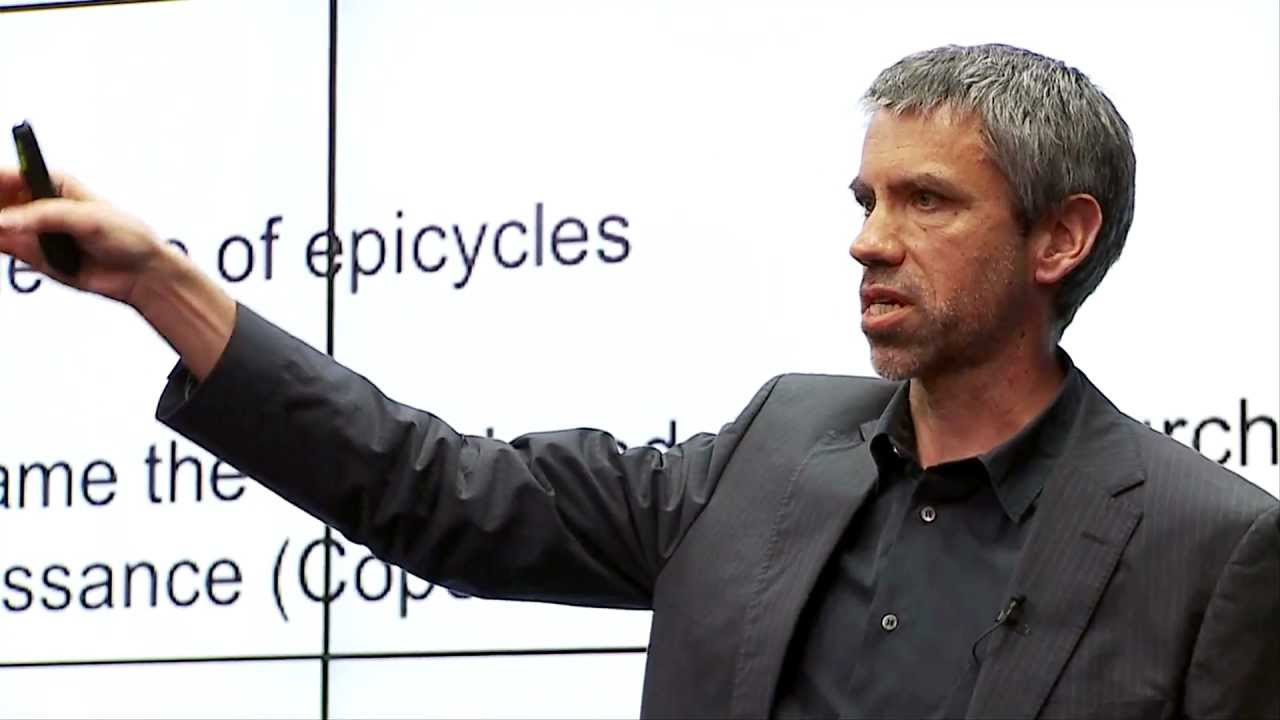
Forecasting in the past, present, and future: David Orrell at TEDxParkKultury
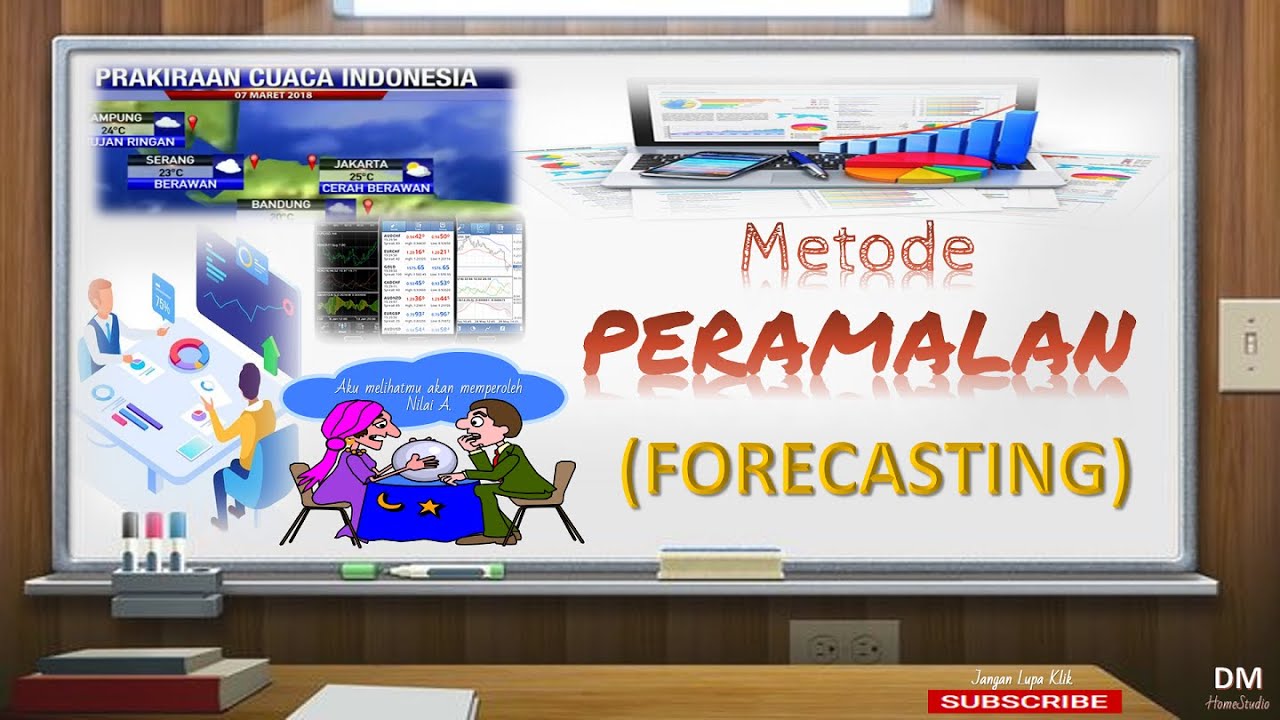
Metode Peramalan

Introduction to Forecasting in Machine Learning and Deep Learning
5.0 / 5 (0 votes)