Why it's harder for AI to open doors than play chess | Pulkit Agrawal | TEDxMIT
Summary
TLDRIn this talk, the presenter explores the paradox of intelligence, highlighting that tasks humans find easy, like opening doors, are challenging for robots, while complex cognitive tasks like chess are relatively easy for AI. The speaker discusses the Model X paradox, where physical intelligence is undervalued compared to cognitive intelligence. They argue for the importance of developing physical intelligence in AI, showcasing simulations and real-world applications of robots learning to walk on various terrains and manipulate objects. The talk emphasizes the need for a holistic approach, including hardware and perception, to achieve true artificial intelligence.
Takeaways
- 🤖 The speaker discusses the paradox that tasks requiring physical intelligence, like opening a door, are more challenging for robots than intellectual tasks like playing chess, which AI has mastered.
- 🏆 AI systems have been able to surpass human capabilities in complex games like chess and Go, but physical tasks remain a significant challenge.
- 🧠 The 'Moravec's paradox' highlights the contrast between what humans perceive as hard (intellectual tasks) and what robots find hard (sensory-motor skills).
- 🕵️♂️ The speaker cites experts like Hans Moravec and Stephen Pinker, who argue that high-level reasoning requires less computation than sensory-motor skills.
- 🧬 The evolution of intelligence is discussed, with a focus on how evolution has spent much more time developing sensory-motor skills than language or reasoning.
- 🌐 The speaker emphasizes the importance of physical intelligence in AI, suggesting that it is a prerequisite for true artificial intelligence.
- 🤝 The use of simulation is highlighted as a key technique for generating data and training AI systems to perform complex physical tasks.
- 🤸♂️ Examples of robots learning to walk and manipulate objects in simulation and then transferring these skills to the real world are provided.
- 🤲 The importance of hardware design, such as hands with touch sensing, is discussed in the context of developing physical intelligence.
- 🔧 The speaker argues for a 'full stack' approach to physical intelligence, combining control algorithms, perception, and hardware design.
Q & A
What is the main paradox discussed in the script regarding AI capabilities?
-The main paradox discussed is the Moravec's paradox, which states that tasks requiring high-level reasoning that are considered difficult for humans are easy for AI, while tasks considered simple for humans, such as sensory motor skills, are actually difficult for AI.
Why do AI systems struggle with tasks like opening doors, despite their ability to play complex games like chess?
-AI systems struggle with tasks like opening doors because these tasks require a level of physical intelligence and sensory motor skills that are not as developed as their reasoning capabilities. These tasks involve understanding and interacting with the physical world in a complex way, which is still challenging for AI.
What is the 'Model X Paradox' mentioned in the script?
-The 'Model X Paradox' is a concept that emerged from observations that AI systems can solve complex cognitive tasks like chess relatively easily, but struggle with tasks involving physical intelligence, such as walking or opening a door, which humans find easy.
How does the script explain the difference in difficulty between chess and sensory motor skills for AI?
-The script explains that while chess, which requires reasoning, is considered a complex task for humans, it is relatively easy for AI systems. In contrast, sensory motor skills, which are second nature to humans, require enormous computational power and are much harder for AI to replicate accurately.
What role does human intuition play in the development of AI, according to the script?
-Human intuition plays a significant role in the development of AI by influencing what tasks are prioritized and how they are approached. The script suggests that human intuition often misjudges the difficulty of tasks for AI, leading to a focus on cognitive tasks at the expense of physical intelligence.
What is the significance of the timeline provided in the script regarding the evolution of intelligence?
-The timeline in the script is significant because it illustrates the vast amount of time evolution has spent developing sensory motor skills compared to language and reasoning. This highlights the complexity of physical intelligence and suggests that AI development should also prioritize physical capabilities.
How does the script suggest AI systems can improve their physical intelligence?
-The script suggests that AI systems can improve their physical intelligence through the use of simulation, where large amounts of data can be generated to train AI in various physical tasks. This data can then be transferred to real-world applications.
What is the importance of hardware in the development of AI physical intelligence as discussed in the script?
-The importance of hardware in the development of AI physical intelligence is highlighted by the need for hands with touch sensing and the development of tools to design better hands for specific tasks. The script emphasizes that hardware, perception, and control algorithms are all crucial for achieving physical intelligence.
How does the script relate the evolution of life on Earth to the development of AI?
-The script relates the evolution of life on Earth to the development of AI by drawing a parallel between the long evolutionary development of sensory motor skills and the relatively recent development of language and reasoning. It suggests that AI should also prioritize the development of physical intelligence before focusing on higher cognitive functions.
What is the speaker's stance on the future of AI and physical intelligence?
-The speaker believes that physical intelligence is a critical foundation for true artificial intelligence. They argue against the idea that AI can be fully realized without embodied intelligence and advocate for a 'full stack' approach that includes control, perception, and hardware in the development of AI.
Outlines
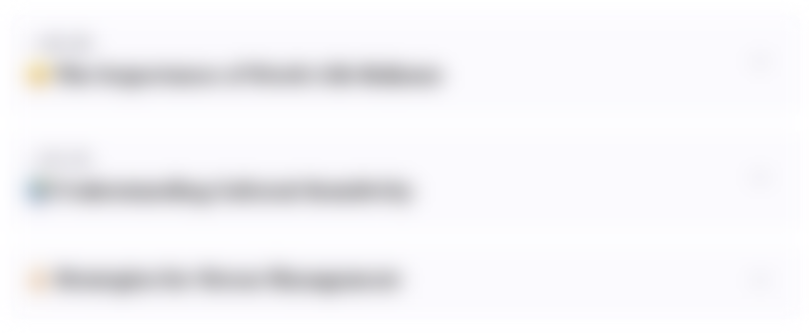
このセクションは有料ユーザー限定です。 アクセスするには、アップグレードをお願いします。
今すぐアップグレードMindmap
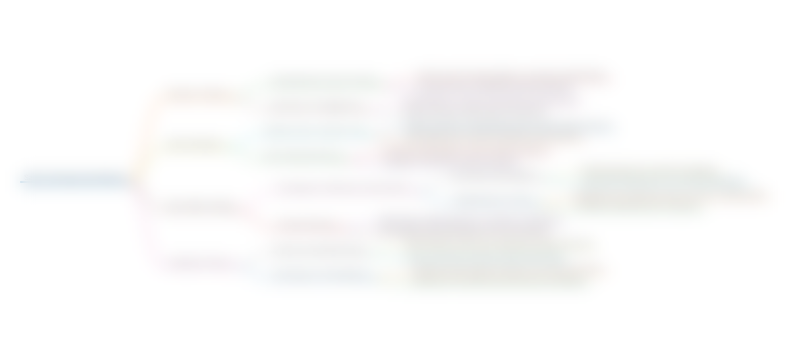
このセクションは有料ユーザー限定です。 アクセスするには、アップグレードをお願いします。
今すぐアップグレードKeywords
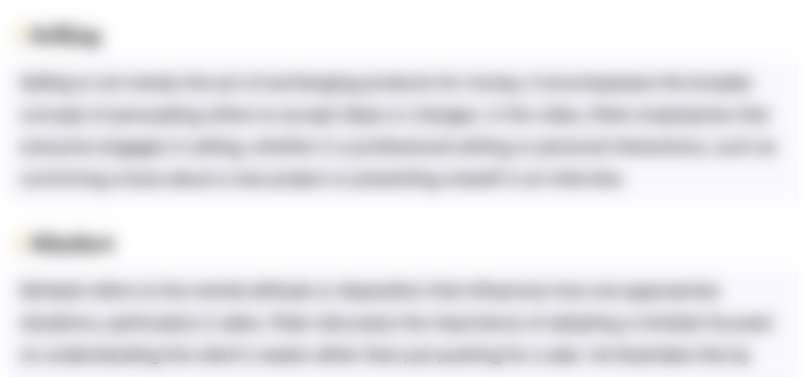
このセクションは有料ユーザー限定です。 アクセスするには、アップグレードをお願いします。
今すぐアップグレードHighlights
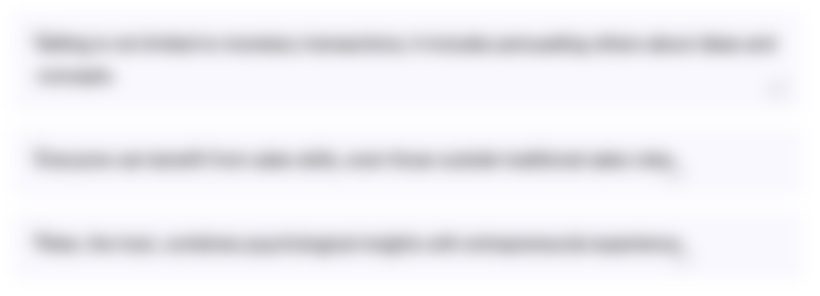
このセクションは有料ユーザー限定です。 アクセスするには、アップグレードをお願いします。
今すぐアップグレードTranscripts
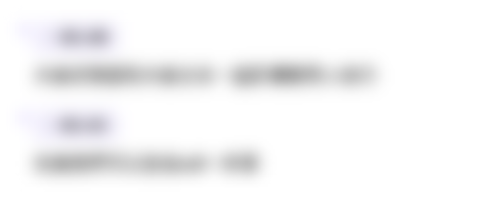
このセクションは有料ユーザー限定です。 アクセスするには、アップグレードをお願いします。
今すぐアップグレード関連動画をさらに表示
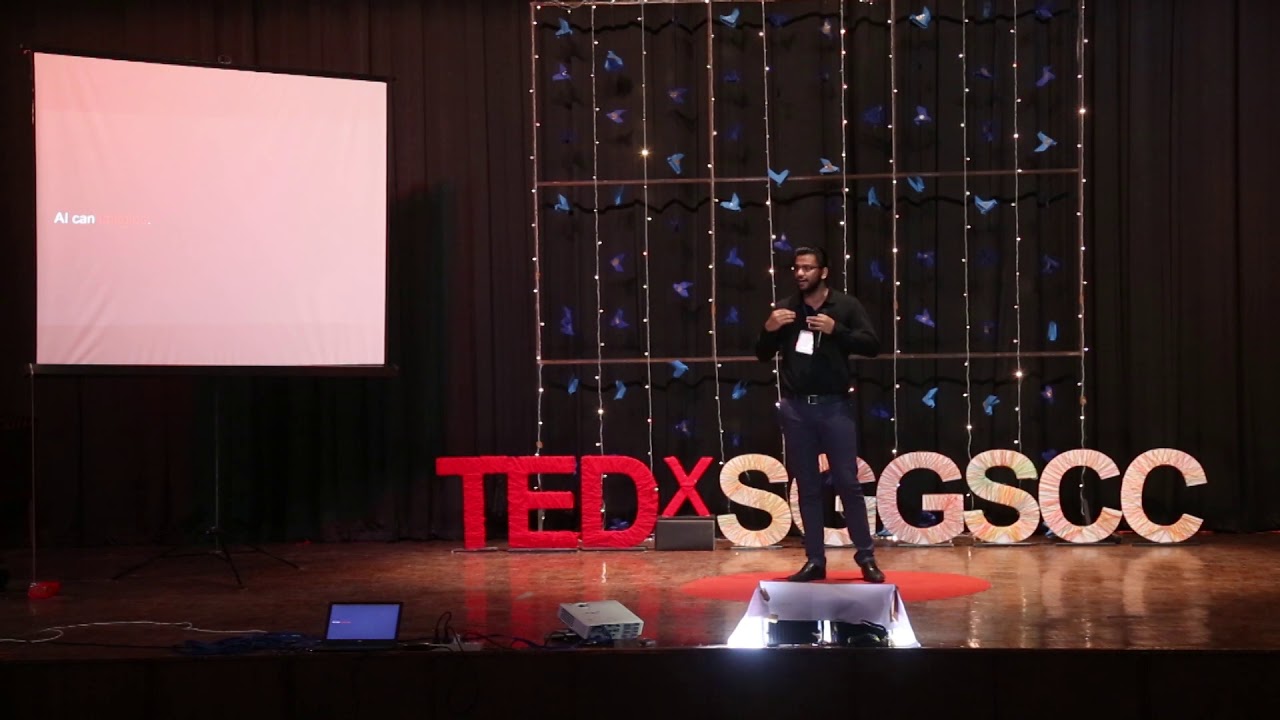
Artificial Intelligence Is No Longer The Future Of Technology | Prakhar Srivastava | TEDxSGGSCC
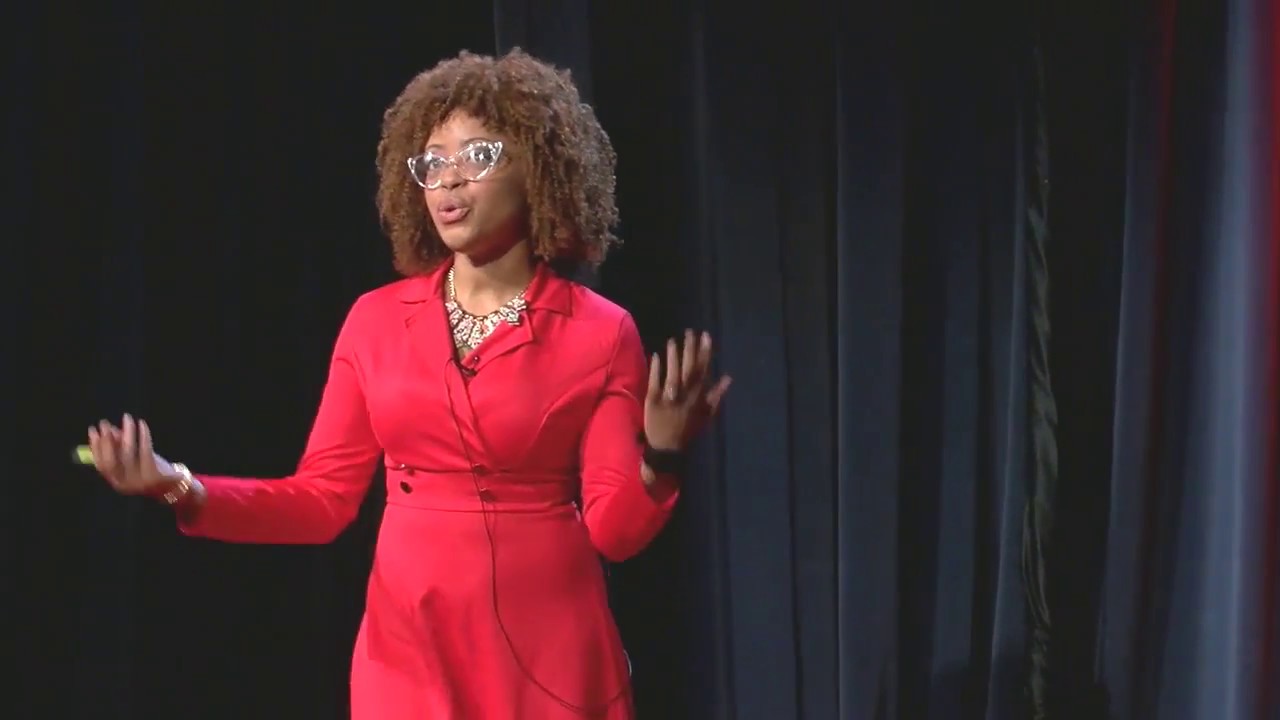
Make Emotional Intelligence Great Again | Janice Gassam | TEDxHofstraUniversity
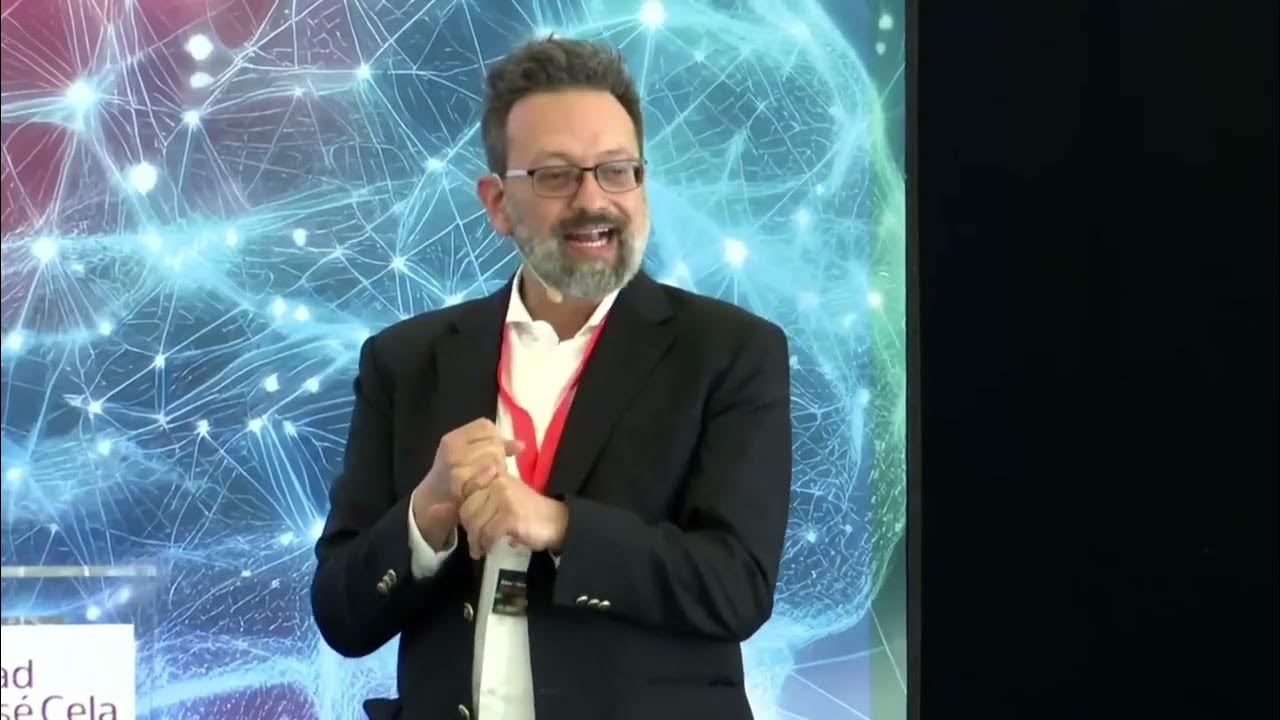
Summit Fernando Díaz Chief Learning and Technology Office Mentu GEF 2024
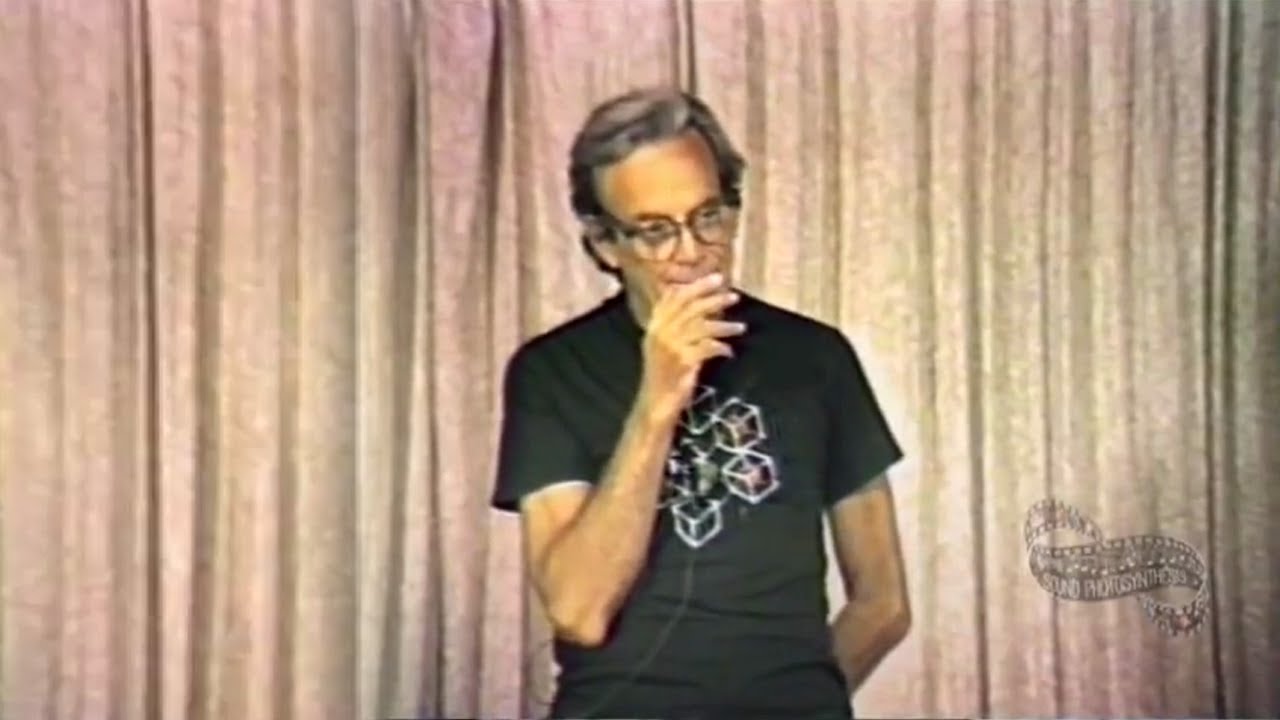
Richard Feynman: Can Machines Think?
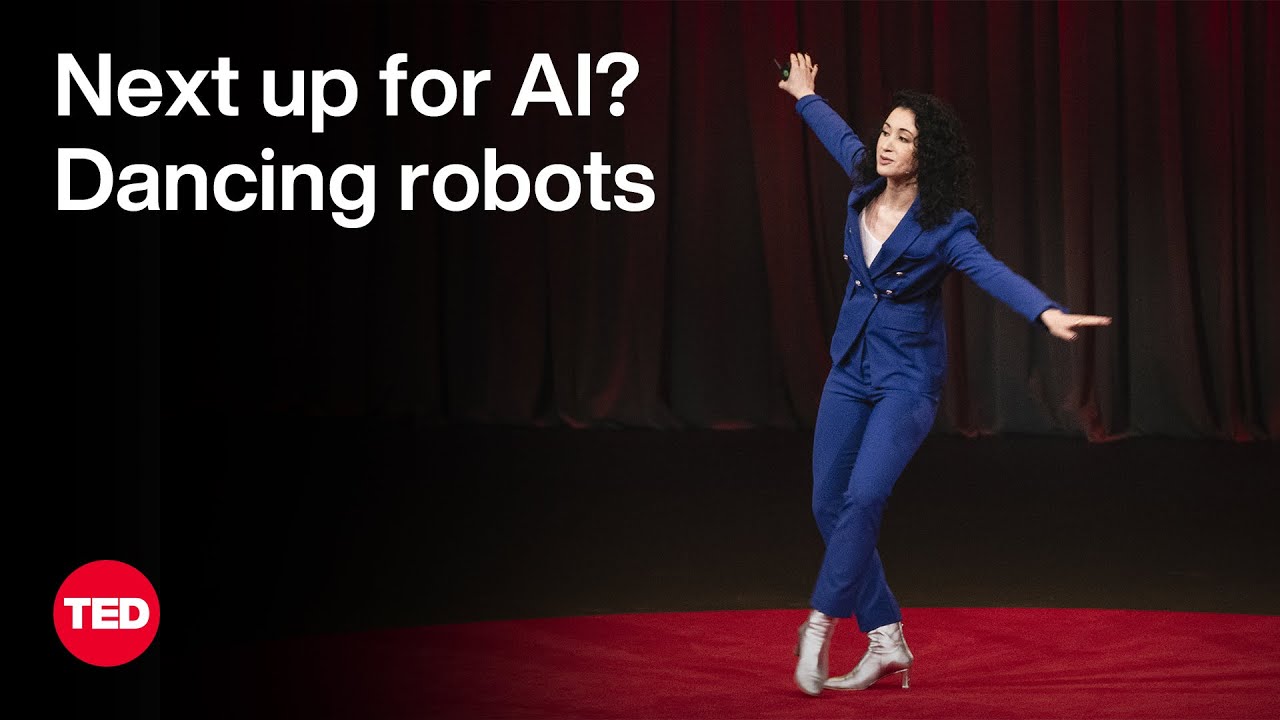
Next Up for AI? Dancing Robots | Catie Cuan | TED
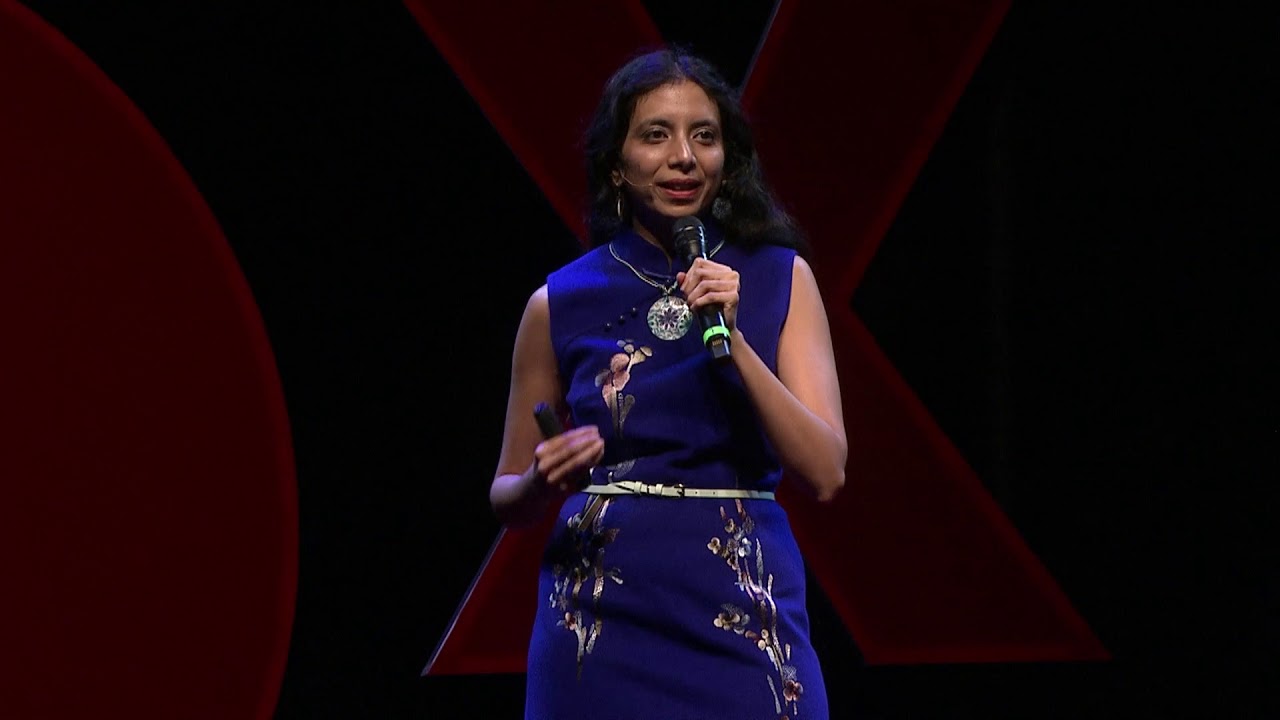
Trinity of Artificial Intelligence | Anima Anandkumar | TEDxIndianaUniversity
5.0 / 5 (0 votes)