XAI-SA 2024, Opening talk
Summary
TLDRこのビデオスクリプトは、説明可能な機械学習(AI)に関するワークショップの概要を提供しています。ディープラーニングの進展により、ブラックボックスモデルが増え、その解釈性が失われています。ワークショップの目的は、これらのモデルを透明化し、ホストウェア解釈方法や解釈可能なモデルの設計など、様々な解決策を探求することです。20の論文発表、4つの口頭発表、ポスターセッション、および招待されたスピーカーのトークが予定されており、音楽、音声、音響の分野における解釈可能なAIの進歩を探求します。
Takeaways
- 😀 イベントは説明可能な機械学習(説明可能なAI)についての議論を中心としています。
- 🕵️♂️ 説明可能な機械学習は、ディープラーニングの黒箱モデルを透明化する試みです。
- 🛠️ 説明可能性の向上には、モデルの解釈方法やモデルの設計方法など、いくつかの解決策があります。
- 📈 今日のワークショップでは、招待されたスピーカーのプレゼンテーションや多数の論文発表が行われます。
- 🎓 招待されたスピーカーには、音楽機械学習の解釈可能性、解釈可能なモデルとポスト解釈、ディープラーニングのオーディオ処理、AIモデルのデータ記憶の調査、解釈可能なスピーチモデル、オーディオ分類のためのNMFの使用などのトピックが含まれています。
- 🗓️ 20件のポスター発表と4つの口頭発表が予定されており、参加者は全てポスターセッションに参加する予定です。
- 📊 提出した論文は、解釈可能なモデル、ポスト解釈方法の適用、音楽や音声の分析、メソッド論的なAIの分野にわたります。
- 🤝 ワークショップの目標は、この分野で働く人々を集め、協力を促進し、新しいアイデアを出そうとしています。
- 📹 イベントはYouTubeチャンネルで録画され、招待されたトークも同様にアップロードされる予定です。
- 📢 参加者は、パネルディスカッションでインタラクティブな議論に参加し、質問をすることができます。
Q & A
このワークショップの主なテーマは何ですか?
-このワークショップの主なテーマは、説明可能な機械学習(説明可能なAI)です。ディープラーニングの進展により、ブラックボックスモデルが増えたことで、それらを透明化しようとする取り組みが議論されています。
ブラックボックスモデルとは何を指し、なぜ問題になるのですか?
-ブラックボックスモデルとは、内部のメカニズムや動作が理解できないモデルを指します。問題になるのは、そのモデルの判断プロセスが透明でなく、検証や信頼性が確保できないためです。
説明可能な機械学習のアプローチにはどのようなものがありますか?
-説明可能な機械学習のアプローチには、元のモデルを変更せずにトレーニング後に何が起こっているか理解するためのホストウェア解釈方法、または最初から解釈可能なモデルを設計する方法があります。
ワークショップの目標は何ですか?
-ワークショップの目標は、この分野で活動している人々を集め、協力を促進し、良いアイデアを出すことです。
ワークショップで何人のポスター発表が行われますか?
-ワークショップで20人のポスター発表が行われます。
ワークショップの日程にどのようなセッションがありますか?
-ワークショップの日程には、4つの口頭発表、ポスターセッション、および特別な招待講演が含まれています。
招待されたスピーカーの分野は何ですか?
-招待されたスピーカーは、音声や音響の専門家、メソッドロジーの機械学習研究者など、説明可能なAI分野に貢献する専門家です。
ポスター発表の最初のセッションは何時に始まりますか?
-ポスター発表の最初のセッションは午前10時に始まります。
ワークショップのビデオはどこで見ることができますか?
-ワークショップのビデオは、ワークショップのYouTubeチャンネルで見ることができます。
このワークショップで取り上げられる音楽の分野における説明可能性のスペクトルとは何を指しますか?
-音楽の分野における説明可能性のスペクトルは、音楽の機械学習モデルを理解するための解釈可能性の範囲を指しており、Ethanの講演で詳しく説明される予定です。
ワークショップの最終日に何が行われますか?
-ワークショップの最終日にパネルディスカッションが行われ、参加者は質問を投げかけ、アイデアを出し合うことが期待されています。
Outlines
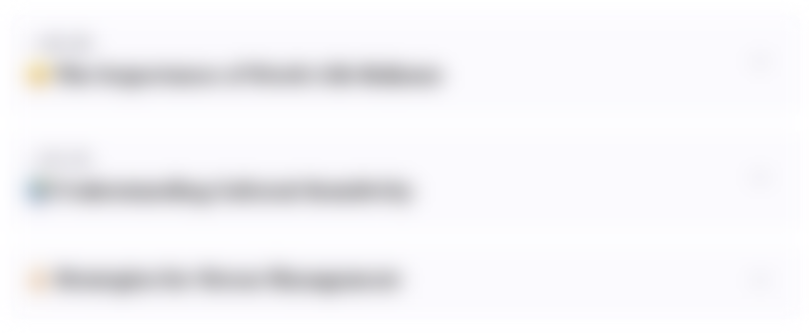
このセクションは有料ユーザー限定です。 アクセスするには、アップグレードをお願いします。
今すぐアップグレードMindmap
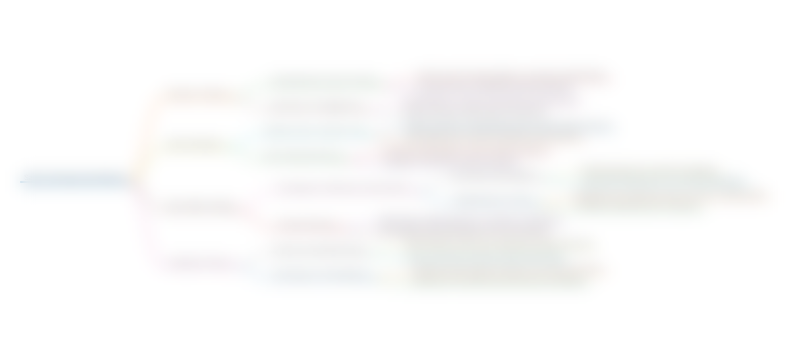
このセクションは有料ユーザー限定です。 アクセスするには、アップグレードをお願いします。
今すぐアップグレードKeywords
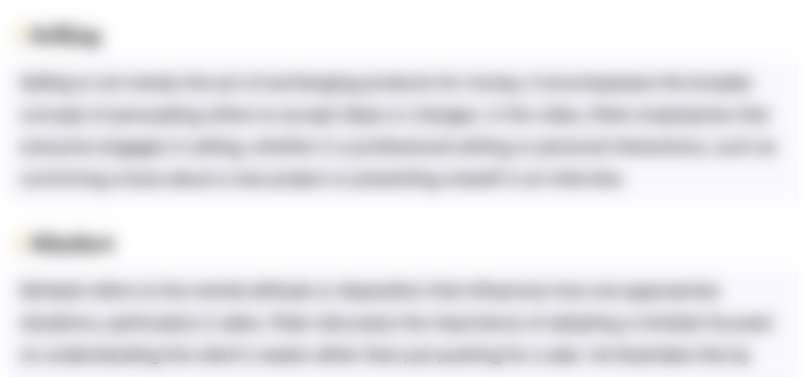
このセクションは有料ユーザー限定です。 アクセスするには、アップグレードをお願いします。
今すぐアップグレードHighlights
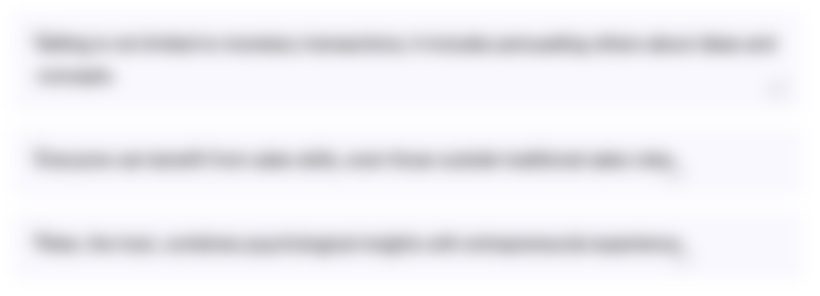
このセクションは有料ユーザー限定です。 アクセスするには、アップグレードをお願いします。
今すぐアップグレードTranscripts
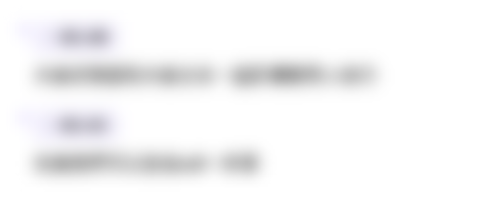
このセクションは有料ユーザー限定です。 アクセスするには、アップグレードをお願いします。
今すぐアップグレード5.0 / 5 (0 votes)