Probability Distributions
Summary
TLDRThis business analytics lecture focuses on probability distributions, emphasizing fitting distributions to data. It explains discrete and continuous distributions, contrasting probability mass with density functions. The session explores three data analysis approaches: trace-driven simulation, theoretical distribution fitting, and empirical distribution creation. The advantages of theoretical distributions over empirical ones are discussed, noting the limitations of relying solely on observed data for predictive modeling.
Takeaways
- đ Probability distributions are statistical models that show possible outcomes for a given event or action.
- đ For discrete variables, distributions are represented by possible values with corresponding probabilities; for continuous variables, by a density function.
- đ The focus of the session is on fitting distributions to data rather than just describing them.
- đ Trace driven simulation uses actual collected data directly in simulations without fitting a theoretical distribution first.
- đ Fitting a theoretical distribution involves checking how well it represents the data, such as normal or uniform distributions.
- đ If theoretical distributions do not fit well, empirical distributions can be created from the collected data itself.
- đ Empirical distributions are built from the data collected and are not an attempt to fit a pre-existing model to the data.
- đ Building an empirical distribution involves arranging data in ascending order and defining a distribution function from rank order statistics.
- đ For grouped data, a piecewise linear function can represent the distribution function, estimating the proportion of observations in each interval.
- đ The building blocks of any distribution include density functions, distribution functions, and moments around the mean.
- đŹ Empirical distributions are useful when no theoretical distribution fits the data well, but they are limited by the range of the collected data.
Q & A
What is the primary focus of the second session of the business analytics course?
-The primary focus of the second session is to discuss probability distributions, specifically how to fit a distribution to a given set of data.
What are the two main types of probability distributions discussed in the script?
-The two main types of probability distributions discussed are discrete and continuous distributions.
How are discrete random variables represented in a probability distribution?
-For discrete random variables, the probability distribution is represented by all possible values of the random variable along with the corresponding probabilities for each value.
What is the difference between the representation of a discrete and a continuous probability distribution?
-A discrete probability distribution is represented by probability masses, while a continuous distribution is represented by a density function, where the y-axis represents the probability density instead of the probability itself.
What is the significance of the normal distribution in the context of grades of a course?
-The normal distribution signifies that grades are expected to follow a bell-shaped curve, with a few very high and very low marks, and a majority of students scoring in the middle range.
What is meant by 'trace driven simulation' in the context of using business data?
-Trace driven simulation refers to the direct use of collected data in simulations without fitting a theoretical distribution to the data first. It involves using the actual data points, such as monthly sales volumes, directly in the analysis.
What is a 'theoretical distribution' and how does it differ from an empirical distribution?
-A theoretical distribution is a pre-defined statistical distribution, such as the normal, uniform, binomial, Poisson, or exponential distribution. It differs from an empirical distribution, which is built from the actual data collected, rather than being a pre-defined model.
Why might one choose to create an empirical distribution instead of using a theoretical one?
-One might choose to create an empirical distribution if the collected data does not fit well with any of the available theoretical distributions, allowing for a custom distribution that better represents the data.
What are the building blocks needed to characterize a normal distribution?
-The building blocks needed to characterize a normal distribution include the density function and distribution function, from which parameters like mean, standard deviation, and moments around the mean can be estimated.
How can one build an empirical distribution from ungrouped data?
-To build an empirical distribution from ungrouped data, one can arrange the data in ascending order, calculate rank order statistics, and then define a distribution function based on these ordered values.
What are the limitations of using empirical distributions compared to theoretical distributions?
-Empirical distributions are limited by the range of data used to create them and may not accurately represent values outside of this range. They can also be biased towards the pattern of the collected data and are not as versatile as theoretical distributions for generating new values for simulations.
Outlines
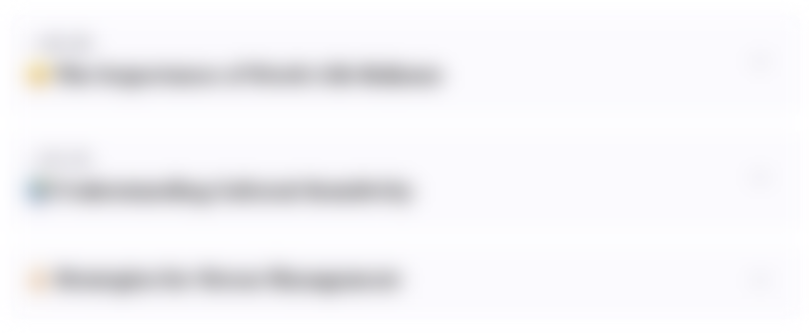
Cette section est réservée aux utilisateurs payants. Améliorez votre compte pour accéder à cette section.
Améliorer maintenantMindmap
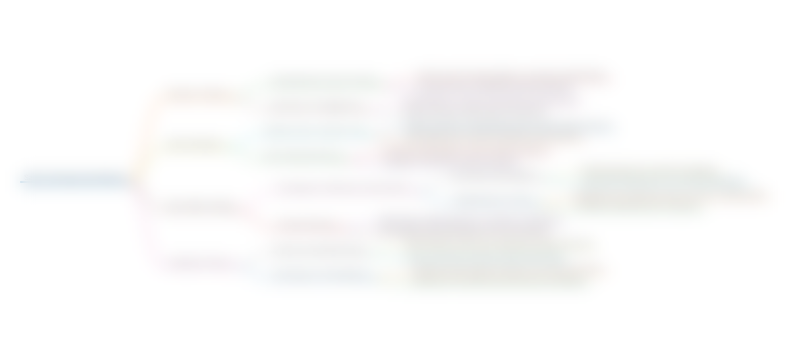
Cette section est réservée aux utilisateurs payants. Améliorez votre compte pour accéder à cette section.
Améliorer maintenantKeywords
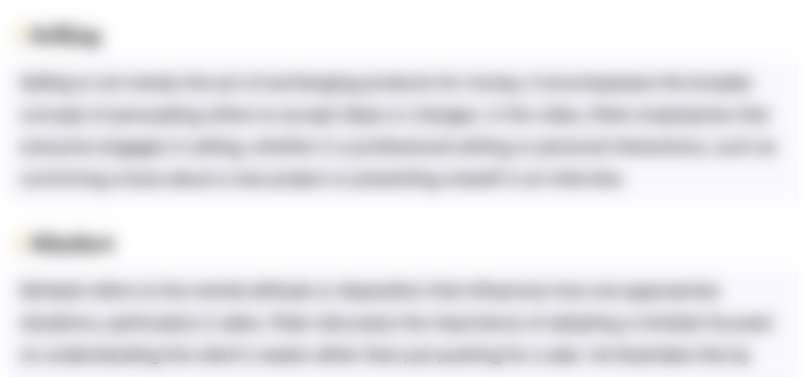
Cette section est réservée aux utilisateurs payants. Améliorez votre compte pour accéder à cette section.
Améliorer maintenantHighlights
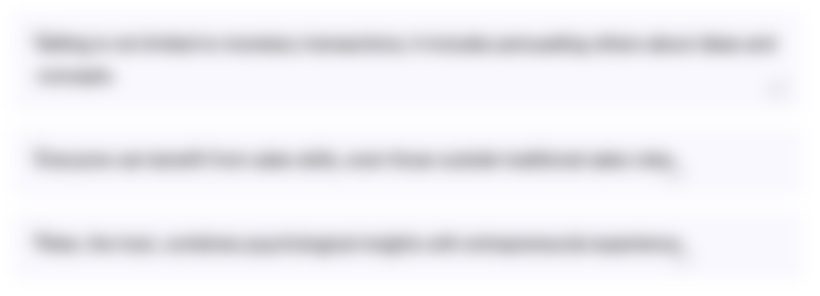
Cette section est réservée aux utilisateurs payants. Améliorez votre compte pour accéder à cette section.
Améliorer maintenantTranscripts
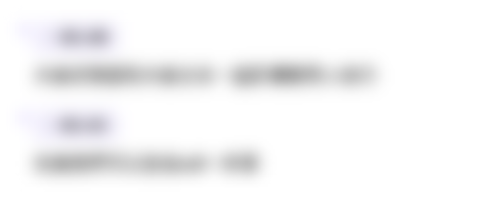
Cette section est réservée aux utilisateurs payants. Améliorez votre compte pour accéder à cette section.
Améliorer maintenantVoir Plus de Vidéos Connexes
5.0 / 5 (0 votes)