AI Hype is completely out of control - especially since ChatGPT-4o
Summary
TLDRThe video script discusses skepticism around large-language model AIs' impact on software development. It critiques the hype following the release of ChatGPT-4o, questioning job market disruption and AI's actual capabilities. The speaker, Carl, uses evidence-based reasoning to argue that despite speed improvements, there's mixed evidence on AI's accuracy. He highlights the history of companies overstating AI capabilities and the psychological tendency of humans to anthropomorphize AI, suggesting a 'toxic culture of lying' around AI demonstrations.
Takeaways
- 🤖 The video discusses the impact of large-language model AIs on the software development industry and the mixed reactions to the release of ChatGPT-4o.
- 📈 There is a debate on whether AIs will replace programmers significantly or if the current AI advancements are a passing trend.
- 🔍 Companies like BP are reportedly using fewer programmers due to AI, but the overall industry impact is still unclear.
- 👨💼 The speaker, Carl, emphasizes the importance of evidence-based analysis in understanding AI's role in the job market and its potential future.
- 🔬 Carl's background in physics has shaped his approach to evaluating technology trends through experimentation and evidence gathering.
- 📊 The script mentions various benchmarks that show mixed improvements in AI capabilities, suggesting that AI's ability to perform tasks correctly is not consistently better.
- 🗣️ Voice interfaces are highlighted as a feature of ChatGPT-4o, but Carl argues that this is not a new advancement and does not significantly impact AI's capabilities.
- 🤔 The video raises questions about the trustworthiness of AI demonstrations and the history of companies overstating AI capabilities.
- 🕊️ The 'Eliza Effect' and 'dark patterns' in AI chatbots are discussed as psychological tricks that make humans more likely to believe in AI sentience.
- 📉 Carl points out a trend of companies being caught lying about AI capabilities, which undermines confidence in current and future AI advancements.
- 🧐 The video concludes by urging viewers to critically assess the evidence and be wary of narratives promoted by those with a history of dishonesty.
Q & A
What is the main topic of discussion in the video script?
-The main topic of the video script is the impact of large-language model AIs, particularly ChatGPT-4o, on the software development industry and the validity of the hype surrounding AI capabilities.
What is the current trend in the job market regarding AI and programmers?
-The current trend indicates that AI is causing some job disruptions, with companies like BP reporting a significant reduction in the number of programmers needed, possibly due to AI advancements.
What does the speaker suggest about the hype around AI and its potential impact on society?
-The speaker suggests that the hype around AI might be exaggerated and that the truth likely lies somewhere in between the extreme views of AI replacing human jobs entirely or being as short-lived as NFTs.
What evidence does the speaker consider reliable for evaluating AI capabilities?
-The speaker considers peer-reviewed papers, benchmarks, firsthand observations from unbiased sources, and trends under similar circumstances as reliable evidence for evaluating AI capabilities.
What is the 'Eliza Effect' mentioned in the script?
-The 'Eliza Effect' refers to the phenomenon where humans are predisposed to believe that AI chatbots have thoughts and feelings, leading to 'powerful delusional thinking' akin to a 'slow-acting poison'.
What is the speaker's opinion on the voice interface feature of ChatGPT-4o?
-The speaker is not impressed by the voice interface feature of ChatGPT-4o, stating that it is not new and has been available for some time, and that it does not necessarily represent an advancement in AI.
What is the term used to describe user interfaces that trick people into certain behaviors?
-The term used to describe such user interfaces is 'dark patterns'.
What are some examples of companies that have been caught exaggerating or lying about their AI capabilities?
-Examples include Tesla with its self-driving demo, Google with Duplex and Gemini AI demos, and OpenAI with the GPT-4 bar exam performance claims.
What is the speaker's stance on the future of AI and its potential to achieve human-level intelligence?
-The speaker is skeptical about the near-future prospects of achieving human-level AI, citing a lack of clear evidence and a history of companies exaggerating AI capabilities.
What advice does the speaker give to those trying to understand the impact of AI on their careers or industries?
-The speaker advises individuals to make up their own minds, follow the evidence, and be cautious of narratives promoted by those with a history of dishonesty.
Outlines
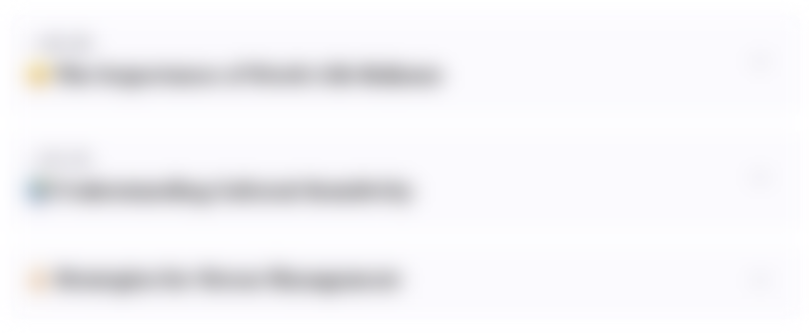
Cette section est réservée aux utilisateurs payants. Améliorez votre compte pour accéder à cette section.
Améliorer maintenantMindmap
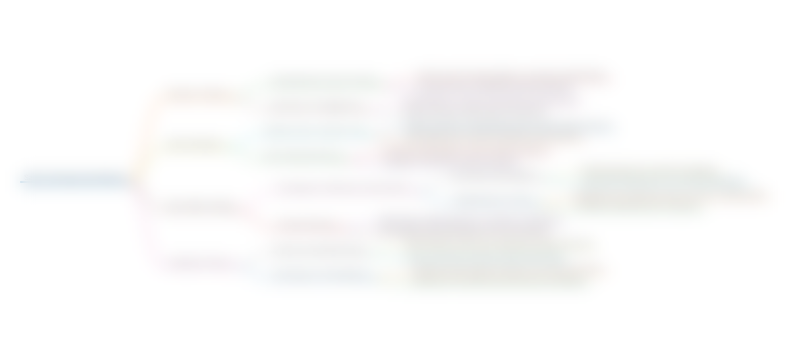
Cette section est réservée aux utilisateurs payants. Améliorez votre compte pour accéder à cette section.
Améliorer maintenantKeywords
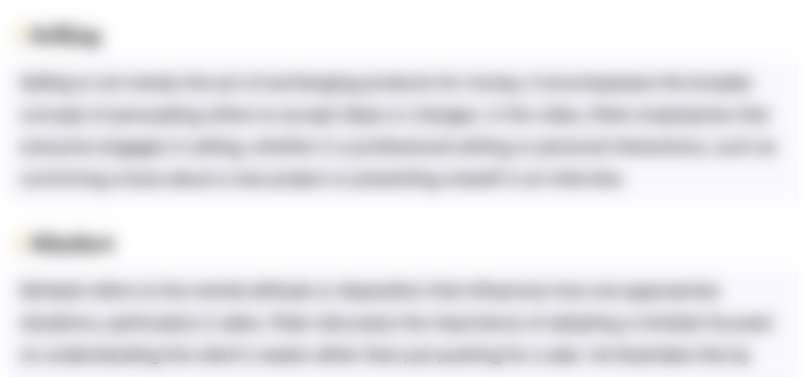
Cette section est réservée aux utilisateurs payants. Améliorez votre compte pour accéder à cette section.
Améliorer maintenantHighlights
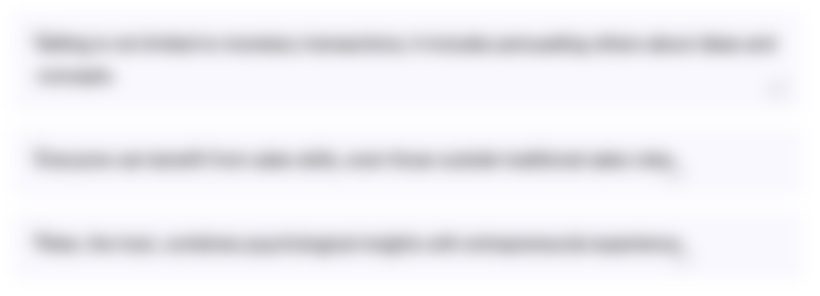
Cette section est réservée aux utilisateurs payants. Améliorez votre compte pour accéder à cette section.
Améliorer maintenantTranscripts
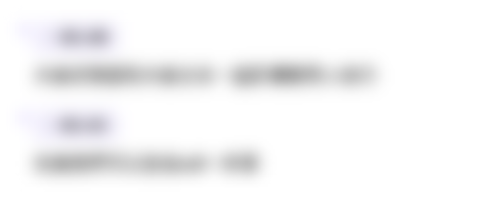
Cette section est réservée aux utilisateurs payants. Améliorez votre compte pour accéder à cette section.
Améliorer maintenant5.0 / 5 (0 votes)