Are We Automating Racism?
Summary
TLDRThe video explores the issue of algorithmic bias in AI systems, particularly focusing on racial bias in image cropping algorithms. It illustrates how AI, despite being seen as neutral, can exhibit discriminatory outcomes due to biased training data and design processes. Examples include Twitter's photo cropping favoring white faces and biased healthcare algorithms. Experts like Ruha Benjamin and Deborah Raji discuss the importance of scrutinizing and improving AI systems to prevent such biases, emphasizing the need for ethical considerations and accountability in AI development and deployment.
Takeaways
- 🤖 The script discusses the issue of bias in AI systems, highlighting that even with good intentions, the outcomes can still be discriminatory.
- 📸 It describes an experiment with an image cropping algorithm on Twitter that consistently chose to display white faces over black faces, suggesting racial bias.
- 🔍 The script mentions the importance of testing AI systems publicly to uncover potential biases, as was done with the Twitter image cropping feature.
- 👥 The conversation includes the perspectives of various individuals, including Ruha Benjamin, a professor at Princeton University, on the implications of AI bias.
- 📈 The script points out that AI systems learn from data that is influenced by human decisions, which can perpetuate existing biases in society.
- 🧐 It emphasizes the difficulty in understanding why a machine learning model makes certain predictions, especially when those predictions are biased.
- 👁 The concept of 'saliency' in image recognition is explored, explaining how AI determines what is important in an image, which can be influenced by the data it was trained on.
- 📊 The script discusses the use of data sets in training AI and how the lack of diversity in these sets can lead to biased outcomes.
- 🏥 An example of a healthcare algorithm is given to illustrate how biased algorithms can have real-world consequences, such as unequal healthcare provision.
- 🛡 The need for better vetting and regulation of AI systems is highlighted, with suggestions like Model Cards for transparency and ethical considerations.
- 🔧 The script concludes with the idea that while AI bias is a complex issue, it is not insurmountable, and awareness and enforcement of solutions are key steps forward.
Q & A
What issue is highlighted in the script regarding data-driven systems?
-The script highlights the issue of algorithmic bias in data-driven systems, showing that even with good intentions, the outcomes can still be discriminatory, affecting different groups of people unequally.
What was the public test of algorithmic bias involving Mitch McConnell and Barack Obama?
-The public test involved uploading extreme vertical images of Mitch McConnell and Barack Obama to force an image cropping algorithm to choose one of the faces, revealing an alleged racial bias as the algorithm consistently chose McConnell's face over Obama's.
What is a Saliency Prediction Model, and how is it related to the Twitter image cropping controversy?
-A Saliency Prediction Model is a type of software that guesses what's important in an image based on what humans typically look at. It is related to the Twitter controversy because it is the kind of technology Twitter uses to crop images, which was tested and found to potentially display bias in choosing which face to prioritize in a cropped image.
What role do human decisions play in the development of machine learning algorithms?
-Human decisions play a crucial role in the development of machine learning algorithms by labeling examples, selecting data, and determining the design of the technology. These decisions can inadvertently introduce biases into the system.
How did the script demonstrate the potential bias in face-tracking software?
-The script demonstrated potential bias by showing that the face-tracking software did not follow a person of color as expected, suggesting that the algorithm might not perform equally well for all skin complexions.
What is the significance of the quote read in the script about robots and racism?
-The quote emphasizes that racism can exist beyond individual malice and can be embedded in systems and structures. It challenges the narrow definition of racism that requires intent, suggesting that even without hate-filled hearts, systems can perpetuate racial disparities.
What is the role of data representation in creating bias in AI systems?
-Data representation is crucial because if the data set used to train AI systems lacks diversity or is not representative of various demographics, the AI system can develop biases that reflect the imbalance in the data.
What is the concept of 'Model Cards' and how do they contribute to addressing bias in AI?
-Model Cards are a documentation effort that provides a simple one-page summary of how a model works, including its intended use, data source details, data labeling, and instructions for evaluating system performance across different demographic subgroups. They contribute to addressing bias by promoting transparency and ethical considerations in AI development.
What ethical considerations should be taken into account when deploying machine learning systems?
-Ethical considerations include evaluating and assessing the system's impact on vulnerable or marginalized groups, ensuring fairness in outcomes, and considering whether machine learning should be used at all in certain situations where it may cause harm.
What is the importance of understanding the power dynamics in the development and deployment of AI technologies?
-Understanding power dynamics is important because it determines whose interests are served by the predictive model and which questions get asked. It influences the trajectory of technology development and its impact on society, especially in terms of resource allocation and decision-making authority.
How can the problem of bias in AI be addressed, and what steps are being taken in the industry?
-Bias in AI can be addressed by becoming aware of the problem, enforcing solutions, and implementing measures like Model Cards for transparency. The industry is beginning to see efforts to enforce these solutions and to question which algorithms should be used and how they are deployed.
Outlines
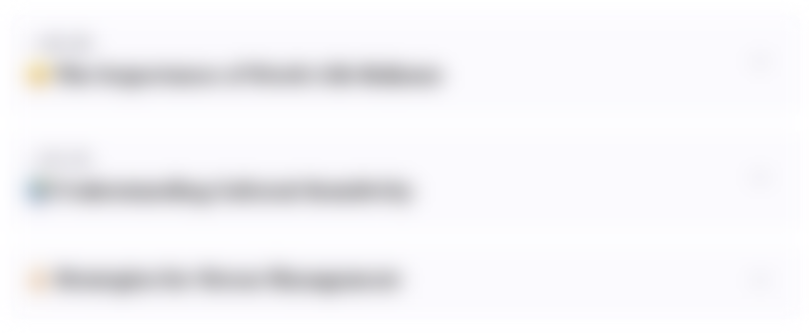
Cette section est réservée aux utilisateurs payants. Améliorez votre compte pour accéder à cette section.
Améliorer maintenantMindmap
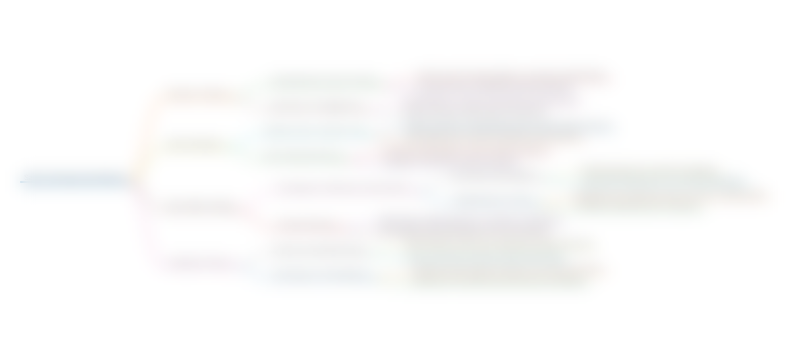
Cette section est réservée aux utilisateurs payants. Améliorez votre compte pour accéder à cette section.
Améliorer maintenantKeywords
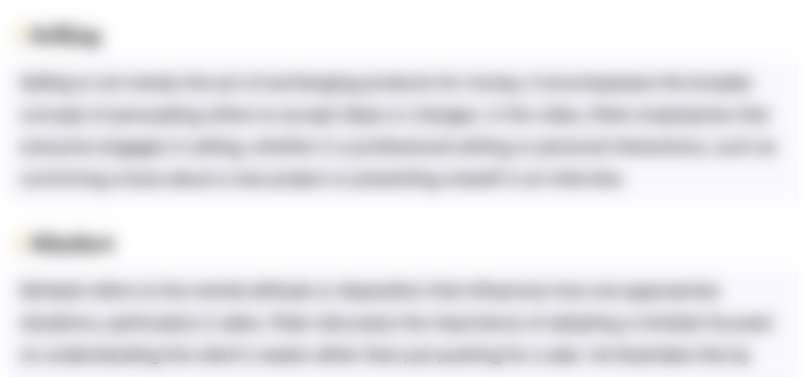
Cette section est réservée aux utilisateurs payants. Améliorez votre compte pour accéder à cette section.
Améliorer maintenantHighlights
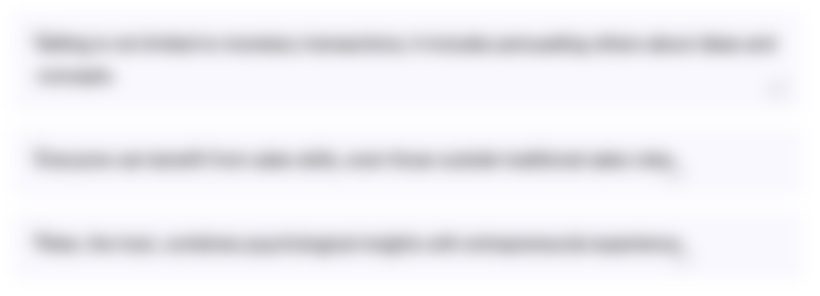
Cette section est réservée aux utilisateurs payants. Améliorez votre compte pour accéder à cette section.
Améliorer maintenantTranscripts
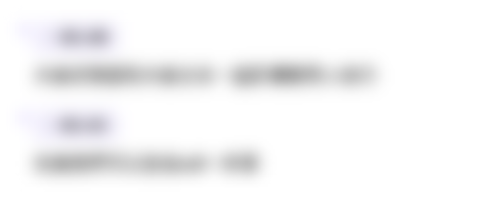
Cette section est réservée aux utilisateurs payants. Améliorez votre compte pour accéder à cette section.
Améliorer maintenant5.0 / 5 (0 votes)