Expectation Maximization | EM Algorithm Solved Example | Coin Flipping Problem | EM by Mahesh Huddar
Summary
TLDRThis video introduces the Expectation-Maximization (EM) algorithm, a crucial method in machine learning for estimating parameters in probabilistic models, particularly with incomplete data. Using a coin-flipping problem, the speaker explains how to calculate the probabilities of obtaining heads with two coins when the labels are unknown. The process involves initializing random values, iteratively applying the E-step and M-step, and converging on final estimates for the biases of each coin. The video emphasizes the importance of EM in handling missing data and provides a clear, step-by-step approach to understanding its application.
Takeaways
- 😀 The Expectation Maximization (EM) algorithm is widely used for estimating parameters in probabilistic models, especially when dealing with incomplete or missing data.
- 😀 Common models that utilize the EM algorithm include hidden Markov models, Gaussian mixtures, and Kalman filters.
- 😀 The EM algorithm involves multiple steps, starting with the assignment of initial random values for the parameters being estimated.
- 😀 A practical example using the EM algorithm involves a coin-flipping problem, where two coins with different biases are considered.
- 😀 In the first part of the example, the biases of the coins are calculated based on the number of heads and tails observed in a series of experiments.
- 😀 If the labels (which coin was chosen) are unknown, the EM algorithm can help in estimating the parameters by iteratively refining the guesses.
- 😀 The algorithm consists of two main steps: the Expectation step (calculating the probabilities of the data given the current parameters) and the Maximization step (updating the parameters based on these probabilities).
- 😀 The likelihood of each experiment belonging to a specific coin is calculated using the binomial distribution.
- 😀 The process continues iteratively until the change in parameter values between iterations is minimal, indicating convergence.
- 😀 The final estimated probabilities indicate the likelihood of getting heads when tossing each coin, providing insights into their respective biases.
Q & A
What is the Expectation Maximization (EM) algorithm?
-The EM algorithm is a popular technique in machine learning used for estimating parameters in probabilistic models, especially when dealing with incomplete data.
In which types of models is the EM algorithm commonly used?
-The EM algorithm is commonly used in models such as hidden Markov models, Gaussian mixtures, and Kalman filters.
Why is the EM algorithm beneficial?
-The EM algorithm is beneficial for handling data that is incomplete or has missing data points, allowing for accurate parameter estimation.
What example does the speaker use to explain the EM algorithm?
-The speaker uses a coin flipping problem, involving two coins with unknown biases, to illustrate how the EM algorithm works.
How are the probabilities (theta values) of the coins calculated in the example?
-The probabilities are calculated by dividing the number of heads obtained from each coin by the total number of flips for that coin.
What challenges arise when the labels for the coin flips are unknown?
-When the labels are unknown, it becomes difficult to assign heads and tails to the correct coin, making it impossible to calculate the probabilities directly.
What are the main steps involved in the EM algorithm?
-The main steps of the EM algorithm include initialization, the expectation step (calculating probabilities), the maximization step (updating parameters), and convergence checking.
How does the speaker determine the likelihood that a trial belongs to a specific coin?
-The speaker uses the binomial distribution to calculate the likelihood of each trial belonging to Coin A or Coin B based on the observed heads and tails.
What does the final result of the EM algorithm indicate in this example?
-The final result indicates that the estimated probabilities of getting heads for Coin A and Coin B are approximately 80% and 52%, respectively.
What should viewers do if they want to learn more about the EM algorithm?
-Viewers are encouraged to check out the previous detailed video on the EM algorithm for a deeper understanding and to share the information with others.
Outlines
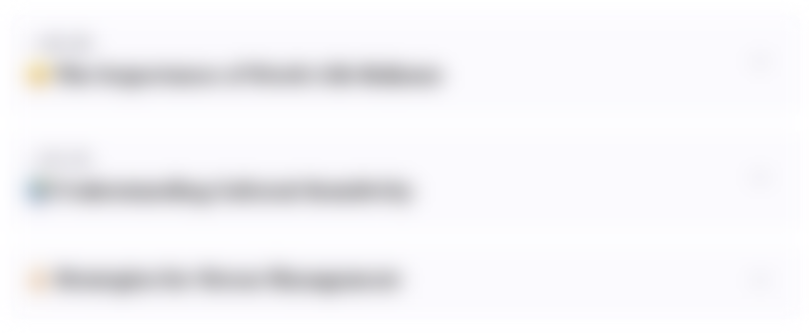
Cette section est réservée aux utilisateurs payants. Améliorez votre compte pour accéder à cette section.
Améliorer maintenantMindmap
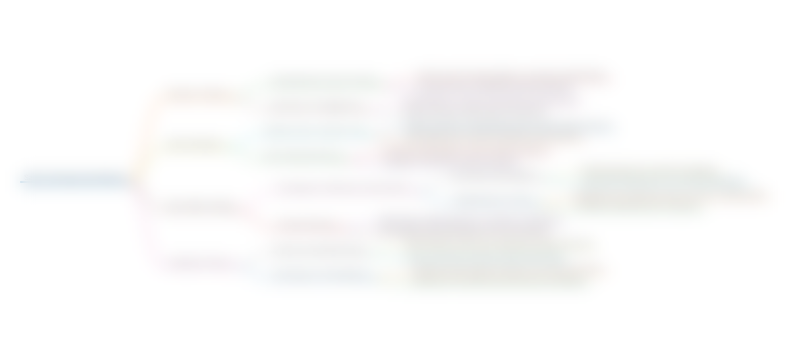
Cette section est réservée aux utilisateurs payants. Améliorez votre compte pour accéder à cette section.
Améliorer maintenantKeywords
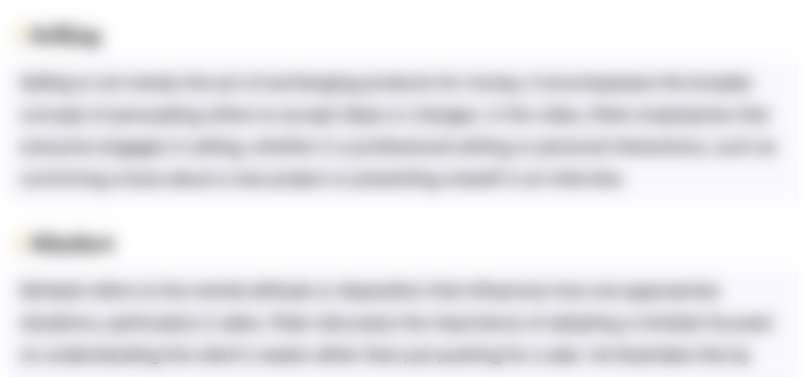
Cette section est réservée aux utilisateurs payants. Améliorez votre compte pour accéder à cette section.
Améliorer maintenantHighlights
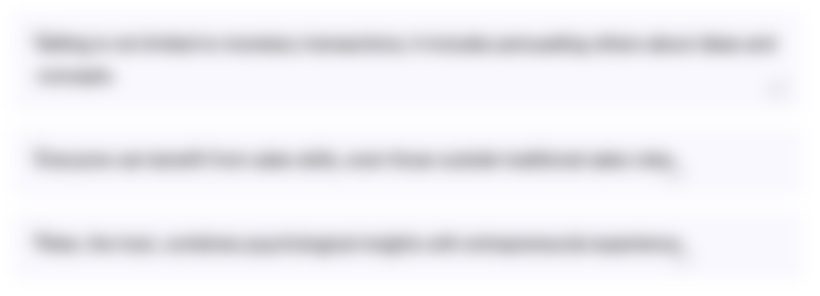
Cette section est réservée aux utilisateurs payants. Améliorez votre compte pour accéder à cette section.
Améliorer maintenantTranscripts
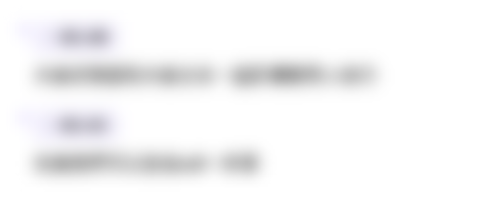
Cette section est réservée aux utilisateurs payants. Améliorez votre compte pour accéder à cette section.
Améliorer maintenantVoir Plus de Vidéos Connexes
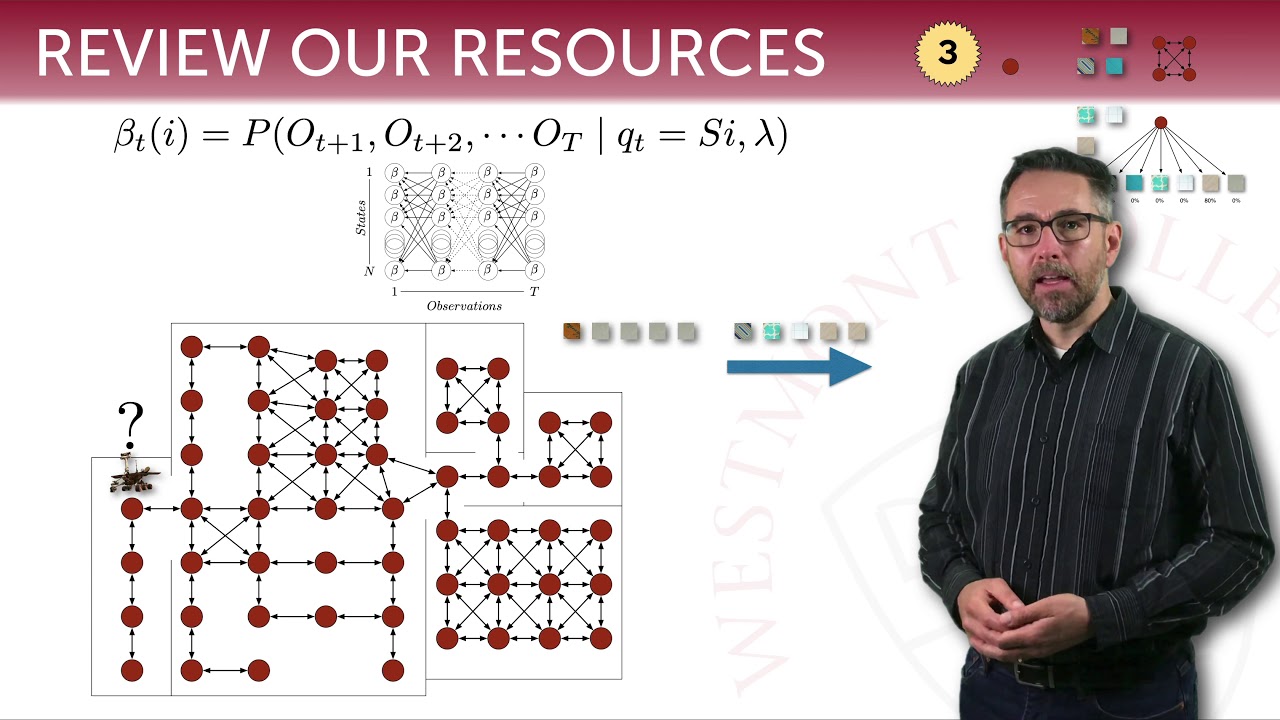
Hidden Markov Models 12: the Baum-Welch algorithm
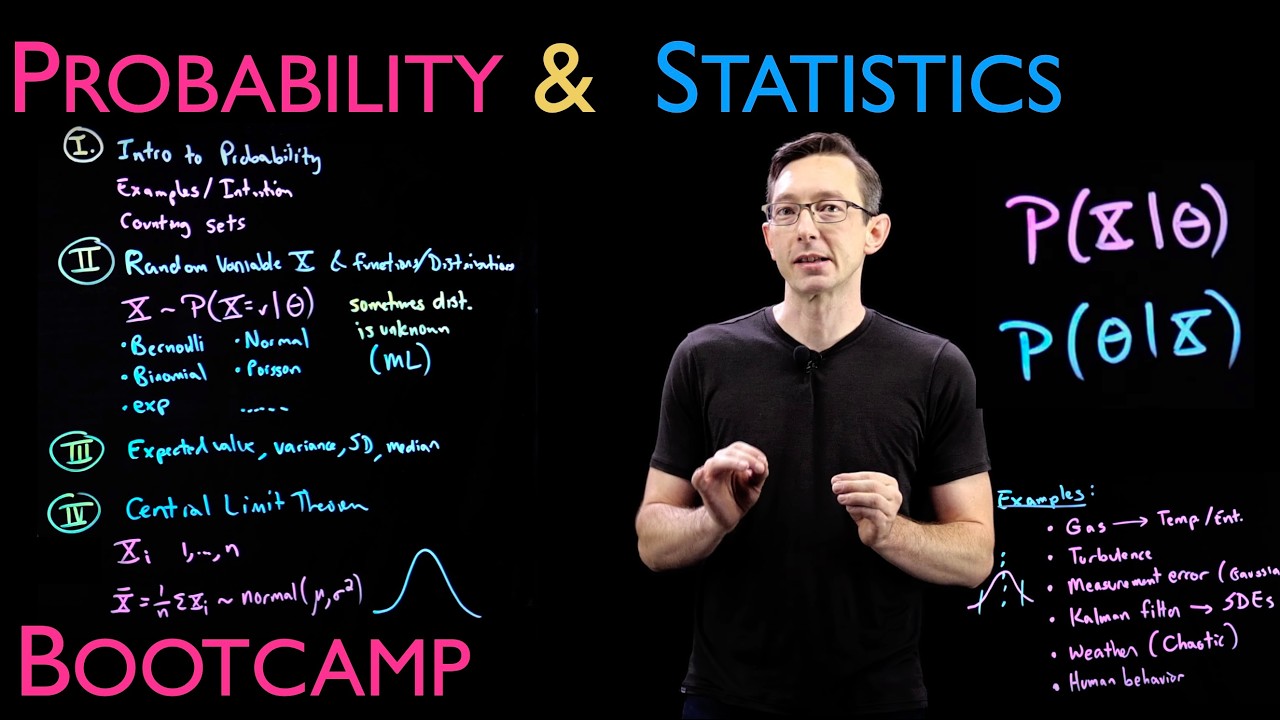
Probability and Statistics: Overview
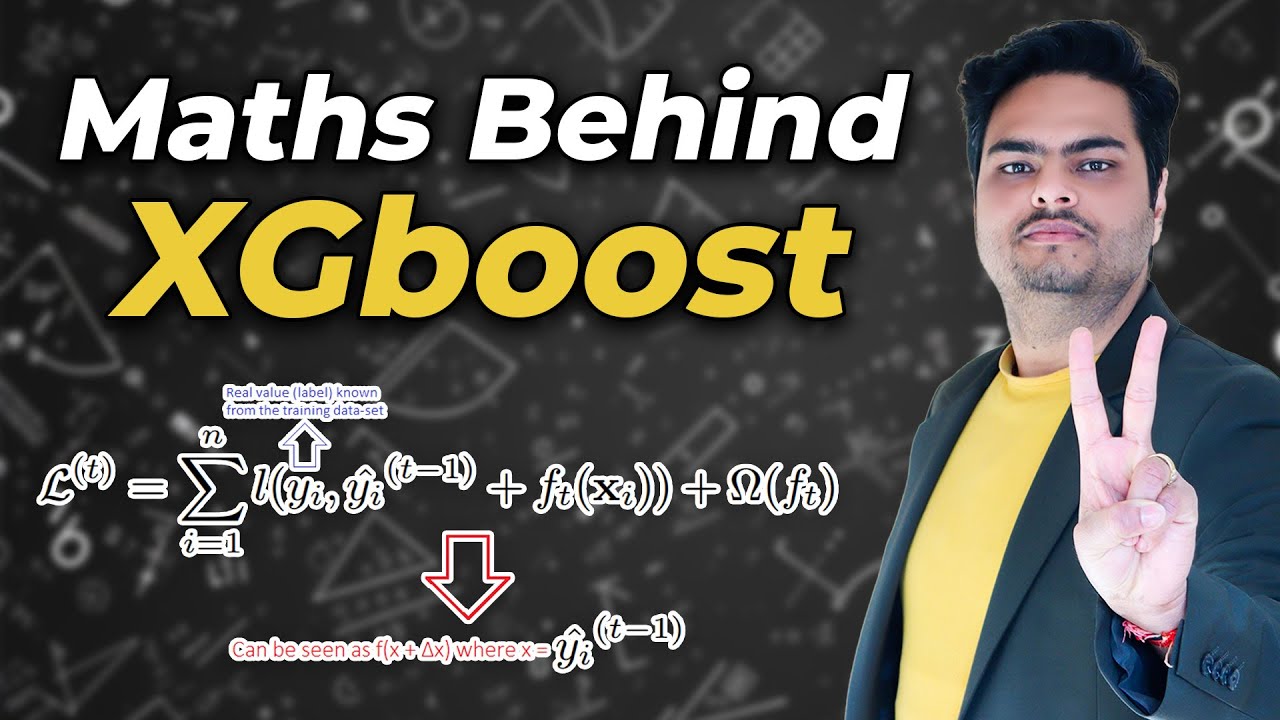
Maths behind XGBoost|XGBoost algorithm explained with Data Step by Step
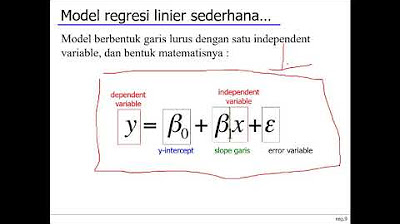
Week 6 Statistika Industri II - Analisis Regresi (part 1)
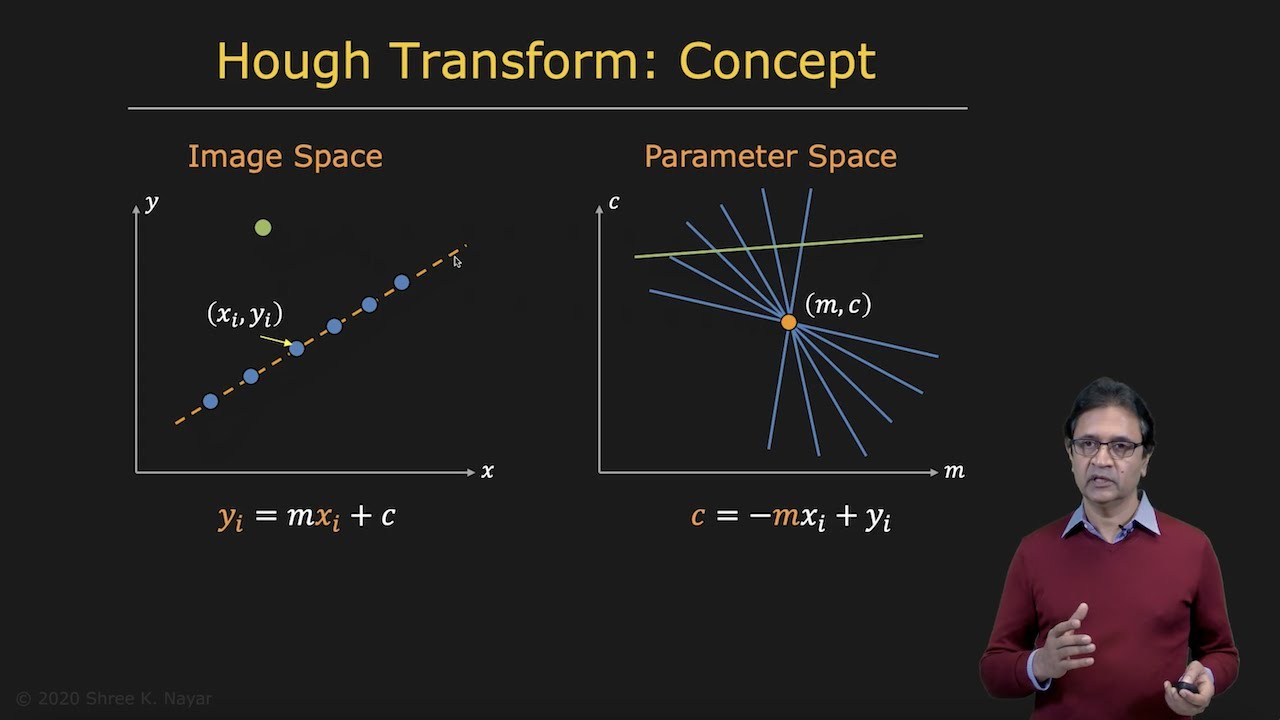
Hough Transform | Boundary Detection
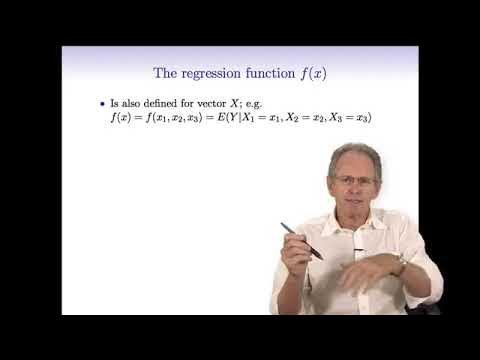
Statistical Learning: 2.1 Introduction to Regression Models
5.0 / 5 (0 votes)