Bernardo Lares & Igor Skokan - Min. Human Bias in Marketing Mix Models using Meta Open Source Robyn
Summary
TLDRBernardo and Igor from Meta discuss the impact of privacy regulations on digital advertising and introduce Project Robin, an open-source tool designed to modernize Marketing Mix Modeling (MMM). They highlight its use of advanced techniques like multi-objective hyperparameter optimization and randomized control trials to minimize bias and improve marketing strategy. The tool aims to democratize MMM, making it more accessible and transparent for businesses to optimize their marketing investments.
Takeaways
- 😀 The speakers are Bernardo Lares and Igor Or, both from Meta, with Bernardo being a Marketing Science Partner and Igor a mountain climber and recent participant in a five-day run in the Sahara.
- 🌐 Bernardo has lived in three countries and is colorblind, which he humorously suggests might be a slight disadvantage in data science.
- 🔍 They work in digital advertising at Meta, focusing on the changes in the advertising ecosystem due to new regulations like GDPR and CCPA, which empower people to control their data.
- 📉 These regulations and browser changes have led to challenges in targeting, optimization, and measurement in digital advertising.
- 📈 They introduced econometrics and marketing mix modeling (MMM) as statistical tools to understand marketing's impact, which have become more prominent due to the limitations of traditional digital measurement techniques.
- 🛠️ Project Robin is an open-source initiative by Meta that aims to modernize MMM by reducing bias and leveraging machine learning to create a continuous, granular analysis tool.
- 📊 Project Robin uses advanced techniques like multi-objective hyperparameter optimization, regularization, and trend and seasonality decompositions to improve model accuracy and reduce human bias.
- 🔧 The project encourages community involvement, with a GitHub repository, a Facebook group for discussions, and a website with success stories and resources.
- 📈 Igor emphasized the importance of randomized control trials (RCTs) for providing causal information and selecting the best models, which is a core feature of Project Robin.
- 🌟 Companies like Resident and Wheely have successfully used Project Robin to quickly implement and optimize their marketing mix models, demonstrating the tool's value for both digital disruptors and established companies.
Q & A
What are the challenges faced by the advertising industry due to recent changes in regulations and browser policies?
-The advertising industry is facing challenges due to new regulations like GDPR, CCPA, and LGPD, which empower individuals to control their data. Additionally, changes in browser policies, such as the deprecation of third-party cookies, have impacted traditional methods of measuring, targeting, and optimizing digital advertising campaigns.
What is the role of the Meta Marketing Science team in the context of these changes?
-The Meta Marketing Science team works with advertisers and agencies to understand the effectiveness of campaign elements and devise strategies to increase the return on marketing investment. They focus on adapting to the changes in the advertising ecosystem by exploring new tools and techniques in response to the challenges posed by regulatory changes and browser policies.
What is Econometrics and how does it relate to Marketing Mix Modeling (MMM)?
-Econometrics is a statistical tool that uses regression analysis to understand the impact of marketing and non-marketing activities over time. It is used in Marketing Mix Modeling (MMM) to build regression models that help understand the proportion of media driven by different channels and to analyze business performance.
How does the traditional MMM approach differ from the contemporary approach promoted by Meta?
-Traditional MMM is manually built by experts, slow, heavily biased, and time-consuming, allowing for limited model iterations per year. Meta's contemporary approach, using Project Robin, enables more dynamic, frequent model updates, minimizes bias through advanced techniques, and incorporates experimental data for more accurate results.
What is Project Robin and how does it aim to improve MMM?
-Project Robin is an open-source package developed by Meta to enable semi-automatic Marketing Mix Models. It aims to minimize bias and improve the MMM process by using advanced techniques like hyperparameter optimization, regularization, and experimental data to provide more accurate and actionable insights.
What are the four pillars of contemporary methods implemented in Project Robin?
-The four pillars of contemporary methods in Project Robin are mitigating bias in model training, selection, and decision-making. This includes using multi-objective hyperparameter optimization, automated trend and seasonality decomposition, regularization to handle multicollinearity, and clustering to select the best models.
How does Project Robin utilize experimental data to enhance MMM?
-Project Robin incorporates experimental data from randomized control trials (RCTs) to provide causal information and measure incrementality, moving beyond traditional MMM's reliance on statistical correlations. This helps in selecting models that more accurately reflect business performance.
What are the benefits of using Project Robin for advertisers?
-Using Project Robin offers benefits such as faster implementation of MMM models, more dynamic and frequent model updates, reduced bias, and the ability to optimize budget allocation across media channels based on experimental data and advanced analytics.
What future developments are planned for Project Robin?
-Future developments for Project Robin include a Python wrapper for easier use by Python users, nested modeling for advanced users, forecasting and prediction capabilities, and a user interface (UI) through a Shiny app for non-coders to interact with the tool.
How can interested parties get involved with Project Robin and contribute to its community?
-Interested parties can join the Project Robin community through the Facebook group for discussions, contribute to the GitHub repository where the code lives, and visit the official website for more information and success cases. They can also provide feedback and feature requests to help drive the project forward.
Outlines
🌐 Introduction to Meta's Marketing Science Speakers
The script introduces two speakers from Meta, Bernardo and Igor, who work in digital advertising. Bernardo is a Marketing Science Partner from Venezuela living in Colombia, while Igor is based in London and has a background in mountain climbing. They discuss the significant changes in the advertising ecosystem due to regulations like GDPR and CCPA, which empower individuals to control their data. These changes have affected targeting, optimization, and measurement in digital advertising. Bernardo and Igor are part of Meta's Marketing Science team, which collaborates with advertisers and agencies to enhance marketing investment returns amidst these challenges.
📊 Project Robin: Advancing Marketing Mix Modeling with Machine Learning
The speakers delve into Project Robin, an open-source initiative aimed at improving Marketing Mix Modeling (MMM) with machine learning. Traditional MMM is criticized for being slow, biased, and less actionable due to its manual construction by experts. Project Robin seeks to automate and modernize MMM by minimizing bias and leveraging advanced techniques. It incorporates hyperparameter optimization, regularization to address multicollinearity, and experimental data for causal insights. The project also aims to foster a community of MMM experts, promote transparency, and make sophisticated marketing analysis more accessible.
🛠️ Methodologies and Features of Project Robin
The paragraph discusses the methodologies and features of Project Robin in detail. It includes multi-objective hyperparameter optimization using evolutionary algorithms to minimize model errors and bias. The use of the Profit package for trend and seasonality decomposition is highlighted, alongside rich regression and regularization techniques to prevent overfitting. The importance of randomized control trials for model validation is emphasized, with incremental results used to discard inaccurate models. The paragraph also outlines the project's outputs, such as one-pagers with performance metrics and visual decompositions, and the ability to run budget allocation scenarios using a non-linear solver.
🚀 Project Robin's Evolution and Future Directions
The final paragraph covers the evolution of Project Robin from a collection of scripts to a more robust and user-friendly tool. It mentions upcoming features like a Python wrapper, nested modeling for advanced users, forecasting capabilities, and a user interface (UI) for non-coders. The speakers invite the audience to join their community, contribute to the project, and use the tool to optimize marketing budgets. They also highlight the successful implementation of Robin by various companies, emphasizing its value for both digital disruptors and established enterprises. The paragraph concludes with an invitation to visit their website and resources for more information.
Mindmap
Keywords
💡Colorblind
💡Data Science
💡Marketing Science Partner
💡Digital Advertising
💡GDPR
💡Multi-touch Attribution
💡Econometrics
💡Project Robin
💡Randomized Control Trials (RCTs)
💡Nevergrad
💡Profit
Highlights
Two speakers from Meta discuss the challenges in digital advertising due to recent privacy regulations and browser changes.
The first speaker, Bernardo Lares, is a Marketing Science Partner from Venezuela, currently living in Colombia.
The second speaker, Igor, is based in London and has a background in mountain climbing and photography.
They work in the Meta Marketing Science team, focusing on digital advertising and its evolving landscape.
Recent regulations like GDPR and CCPA empower users to control their data, affecting digital advertising strategies.
The speakers discuss the impact of these changes on targeting, optimization, and measurement in advertising.
Multi-touch attribution, which relies on personal data, is becoming less viable due to privacy regulations.
Econometrics and geo experiments are gaining prominence as alternative tools in the advertising ecosystem.
Marketing Mix Modeling (MMM) is an old statistical tool being revisited for its ability to understand marketing's impact without personal data.
Project Robin is introduced as an open-source project to modernize MMM with machine learning and reduce analyst bias.
Robin aims to build a community of MMM experts and leverage open-source code for a more robust and transparent methodology.
Traditional MMM models are slow and biased, while Robin enables more dynamic and frequent model updates.
Robin uses advanced techniques like Nevergrad for hyperparameter optimization and Profit for data enrichment.
Randomized Control Trials (RCTs) are emphasized as the best way to validate MMM models in Robin.
Robin provides outputs that help businesses optimize their media budget allocation across different channels.
The project has evolved with new features like a Python wrapper, nested modeling, forecasting, and a user-friendly UI.
Robin is now available on CRAN, and the team invites the community to contribute, provide feedback, and use the tool.
Case studies are shared, showcasing how companies like Resident and Unilever have benefited from using Robin for faster and more accurate MMM.
The speakers conclude by encouraging the audience to join their community, use Robin, and contribute to its development.
Transcripts
we have two speakers coming up together
at the same time um
the first one is colorblind which
probably hurts a little bit during data
science
has lived in three countries in latdam
and is a retired
dj other speaker
is a mountain climber and just recently
this photo this might not be the photo
you got to tell me this one he took this
photo he did take this photo because he
climbs mountains but he also just did a
five-day run in the
sahara five days
please everyone welcome burr and igor
i guess you'll have to guess who's who's
hi everybody we're really really excited
to to be here and to be here in person
specifically
saying hello to everyone who is joining
us virtually but
for now at least we don't have to say
can you see my screen
to give quick intro to us we both work
at meta and this is bernardo and i'm
igor
hi my name is bernardo lares i'm a
marketing science partner i'm venezuelan
but i'm living in colombia
and
and my name is igor or as some people
call me
i'm based in in london in the uk and um
i'm the one who actually climbed that uh
climbed that mountain so that was that
was a picture that i took uh back in
november in nepal so but anyway
unrelated to the talk we have the the
talk we have is about uh advertising we
both work at meta we work both work in
digital advertising uh and as you
probably know
there is a huge amount of change going
on in the advertising uh ecosystem in
the industry so since about last three
years there have been maybe 50 different
regulations starting in you know in
europe in the eu with gdpr then there
was ccpa in california lpg lgpd in
brazil and another plenty of of
regulations giving more power to the
people to decide what they do with their
data
along with browser browser changes with
duplications of cookies and applications
of ways of how
digital industry used to measure target
and optimize optimize campaigns
and that itself has led into
challenges when it comes to
all kinds of things starting from
targeting to optimization but also
measurements so we are in the meta
marketing science team and we work with
advertisers and agencies try to
understand what kind of elements of
campaigns are working and then try to
devise uh
strategies to increase the return on the
on the marketing investment
as such what we have seen that we
because of these changes and because of
uh
some of the measurement techniques and
tools that have been trusted and used
for many many years
are are you know impacted this impact is
not binary it's not you know on and off
and there there is a spectrum and on one
side of the spectrum we have tools such
as multi-touch attribution uh and that
requires person you know log-level data
or or some kind of pii to be used this
is completely now impacted and almost uh
not viable at all but on the other side
we we see a emergence of tools and
techniques that have existed since even
before the internet uh have existed so
econometrics or geo experiments uh
econometrics we we refer to as often as
mmm uh has is now raised into
into much more prominence uh so just to
sort of put put a little definition of
what do we mean by econometrics and mmm
so mmm is a statistical tool that uses
regression analysis to understand the
components of marketing and
non-marketing activity try to cover it's
a correlation method in a way try to
bring uh
try to understand a trend over time by
building a regression by regression by
building regression models uh so this is
how it looks it's totally aggregated
time series there is uh
that uh the analyst would would uh take
the data over the last two to three
years and try to build a built-in model
from it uh using this this kind of
technique we can understand uh what is
the proportion of media that is driven
by the different channels and here on
the right side you can see that you can
actually go into quite a granularity uh
of
of a sort of like how how did the
business perform and you can understand
now this approach has been around since
maybe 50s or really into 70s but it has
a lot of challenges and the fact is that
it's built manually by hand by analysts
presents a huge challenge because it's
slow because it and it has a lot of bias
so a lot of analyst buyers a lot of
human bias is sort of projected into
into create creation of this of this
formula we think that is not a challenge
as such or it's an opportunity and uh
bernardo will talk about like how have
we approached this in in our open source
project uh that is um there's currently
available but it's fair to say we think
that this uh method that is old and
handmade can be can be dramatically
improved uh and it can actually become a
machine learning supported
continuous uh granular analysis that can
bring even aspects of
of uh experiments and and ground truth
measurement to improve the to improve
the overall delivery so this is so
seriously how it looks uh so taking
taking uh the best of the of the
classical approaches uh the strategic
the the
uh the tactical uh and and creating a
new contemporary version and this conte
actualization of this contemporary
version of uh of marketing miss models
or econometrics is what we call project
robin and bernie will take us through
what it is thanks iggy
so to have a better understanding of uh
of what motivates us to promote this
project
uh let's quickly check what is uh
robin's vision what is our goal in
marketing science and
finally what is robin
so
we are looking forward to build a
community of mmm experts
uh to leverage open source code and
techniques to create and build a more
robust and transparent methodology
especially for marketing
in marketing science our team
is to help all businesses grow based in
best practices using data science and
privacy
and robin what is robin robin is an our
open source package we developed to
enable semi automatic marketing mix
models in a way that we are minimizing
bias using advanced and modern
techniques
so what do we mean by contemporary
methods
so
traditional mms are usually
built by experts in the matter there are
manually built they are slow they are
heavily biased
and they take a lot of time to train
so that makes them like less actionable
given that you can
maybe if you are a large advertiser and
you have budget you can buy you can
have one or two
models per year
uh but with robin we enable more dynamic
and you can refresh as fast as you
can gather your data
another common problem is that uh
traditional mmms have
like the same parameters and same ad sub
transformations
which we customize using
never grad that it's a a library that
helps us optimize the hyper parameters
and pick which of these
values
better transform the data to reflect
your true values
we also use rich regression and
regularization to to take
to deal with multiculinarity and
penalize it
and avoid overfitting
we used a profit which is another our
package open source as well by meta to
enrich our data set with trend and
seasonality decompositions
and finally and one of the most
important features is that we push
forward to provide experimental results
experimental data so we run experiments
to
measure
incrementality and provide causal
information instead of pure correlations
which
traditional mmms only validate with
statistical
correlations and data
so now that i've spoken about all of
these
interesting techniques i'd like to show
you that there's a bunch of other
techniques and
that are already implemented they are
all trying to minimize a bias since the
moment we ingest the data until we
provide the the results
but we don't have more than 20 minutes
today to talk about it so feel free to
reach later to speak about any of these
we're going to focus on these four
pillars they are
mitigating bias in mm training selection
and decision
making so
as i've mentioned a couple of times mmm
it relies heavily on the analyst that is
training the model and selecting the
variables so we use a multi-objective
hyper parameter optimization with
evolutionary algorithm that means that
we use nevergrad which is another open
source um
library for python but we've enabled it
with reticulate so we're in safe zones
and so
what it does is it try to minimize the
errors so the model error the
decomposition distance that
reflects how different
it is to the your model and if you are
providing the calibration data it also
uses to minimize the error
and we use pareto front optimal results
given that we run all these simulations
all these iterations we can have maybe
ten thousand twenty thousand models and
we pick those that are closer to
the
to the edges which are the ones that
have the lower the lowest errors
we also use
profit to run at the composition out in
an automated way so we minimize the bias
and
like automatically
leave that information to the algorithm
and not to the analyst to enrich our
data and uh make it make a better fit
and reach regression with lambda as
hyperparameter to
to deal with
overfitting and multicollinearity and
lambda as a hyperparameter given it's a
parameter we can optimize we pro we give
this variable to never grad so it's
going to
play with the values and see
which lamp that will minimize the three
errors we're optimizing towards
uh we also have uh
some criteria to let the user know if it
if the
the algorithm has converged or if we
think that you will have further
possible better solution based on
standard deviation and means comparing
the initial iterations with the last
ones
and we have a super interesting
clustering ka with k-means so once we
have the 10 000 models we reduced to
maybe 100 using the pareto front
then with those 100 we run clustering
k-means with the roi results and we
group those models that are similar and
pick those that have the minimum
combined error so that way from 100 we
maybe select six seven eight that are
the ones that the analysts will inspect
and compare one by one
and
we also have
as i mentioned one of the most important
and relevant
things we have here with robin is that
we believe that randomized control
trials or rct experiments are
our key and the best way to pick which
are the best models so when we provide
this uh calibration information we use
uh incremental results that are
basically what we know is true because
we were we measured it and we discard
those models that are away from the
truth
when we do this we add this third
objective
objective function to minimize towards
to the never algorithm
so once you run robin then you have a
list of outputs that will make them a
pretty useful to be able to pick which
of these models are the ones that better
reflect the the business and that you
will continue towards to optimize your
budget allocation across all media
channels so we have one of the main
outputs is this one pager that it
contains like the unique id that for
that model the performance metrics and
some visuals with um the decomposition
the weights for each parameter the
sheriff effect versus share of spend so
you know which ones are more effective
uh the results for the ad stock
transformation that means they carry
over for each of your media
and
uh
and the regression
itself the last methodology i would like
to speak today is one of the most
valuable functionalities with how we
have with robin once you selected the
model then you can run several scenarios
based on the budget you
currently run or a
hypothetical
um budget
and the constraints so we use a
non-linear solver
on the back end to
search for that combination
based on the saturation curves uh to
optimize your
your portfolio and the allocation across
your media paid channels
so
as you might expect this is a
and a project that has evolved and has
changed naturally
we spoke last year so the ones that
heard about this project it was like a
bunch of scripts and it was
a good idea but not readily implemented
but we still have a list of things we
want to do a feature requests we've
received
the first one is a python wrapper so we
can enable two python users to use robin
and
we are not developing in python first
because we are not python coders and
secondly because it is still changing
and evolving and we are still having a
new feature request and things
implemented so
we have we'd have to wait
to have
a fully developed python version
we will enable nestle nested modeling
for more advanced users if you want to
disaggregate information based on on
brands on geographical data
forecasting and prediction for that
we'll have to enable time series
validation as well
and
it's there's a ui coming up with a shiny
app so in case you are not quite
friendly with coding then you can run
the app play with the the demo uh with
the dummy data we have and you'll get an
idea of what the outputs and uh how to
deal with with your mms
so um i'm really happy to be here again
with all of you sharing about this
hopefully you can join us to be part of
the community maybe as intellectual
curious people or as active users we
have the facebook group where a lot of
people and i've met some of you asking
questions around
we try to make it as
active as possible we have great people
collaborating and of course we have the
github repository where the code lives
and since like three weeks ago we've
enabled a robin in cran so we're really
proud to be the second
r package that comes from meta in chron
and
you can install the the the most stable
version from there
and also we have our website we have a
lot of great content where you can read
more about about us we have some success
cases of actual advertisers that have
used robin to optimize their
budget and they have measured it its
impact
and
what else uh
yeah um
visit us and join us and thank you all
for being here we have a bit more
we have some of the cases
[Applause]
so
some of the cases we do have because we
have about
because we have about three minutes um
they wanted to clap
well done
um
yeah we have some of the cases i mean in
the last
two minutes or so we do have oops where
is it
ah yeah it's come up so some of the
cases that we have so for example
resident is a is an israeli uh ecom they
have uh they have used robin they have
been with us since like very early
versions uh as our valued beta testers
they
really valued the speed so they tried
for about five months to implement this
inside the organization but using robin
they were able to get meaningful models
in about five days uh this sort of speed
and this sort of logic is sort of
carrying over through of uh of the many
cases that we have as bernardo said on
the on the website the other one is um
i think they are polish
uh wheely back
wheel tanks uh company the very similar
story they they they really valued robin
for its speed of implementation and
therefore the quality of the of the
models so these are let's say digital
disruptors or like smaller companies
that have maybe uh didn't have it before
but from the other cases that we have
seen even companies like unilever who
you know arguably may be invented and
the marketing mix modelling it's sort of
present form in in sometimes in the 50s
and 60s
have found value in it
so that
for example koppel who is a leading
mexican department store and and so many
others so really we
we think that this this tool being it
open source enables people to
transparently understand and what's
going on and and democratize a technique
that was typically restricted
um and we really invite everyone to help
us
drive it forward give us feedback use it
and let us know what you think so i
think we are just bank on time so we
really thank you thank you for your
attention
and yeah please visit us on the website
or any of the any of the resources so
thank you again and have a really lovely
day
you
Voir Plus de Vidéos Connexes

What is Vocable.ai and how can businesses use it to simplify and automate their content marketing.
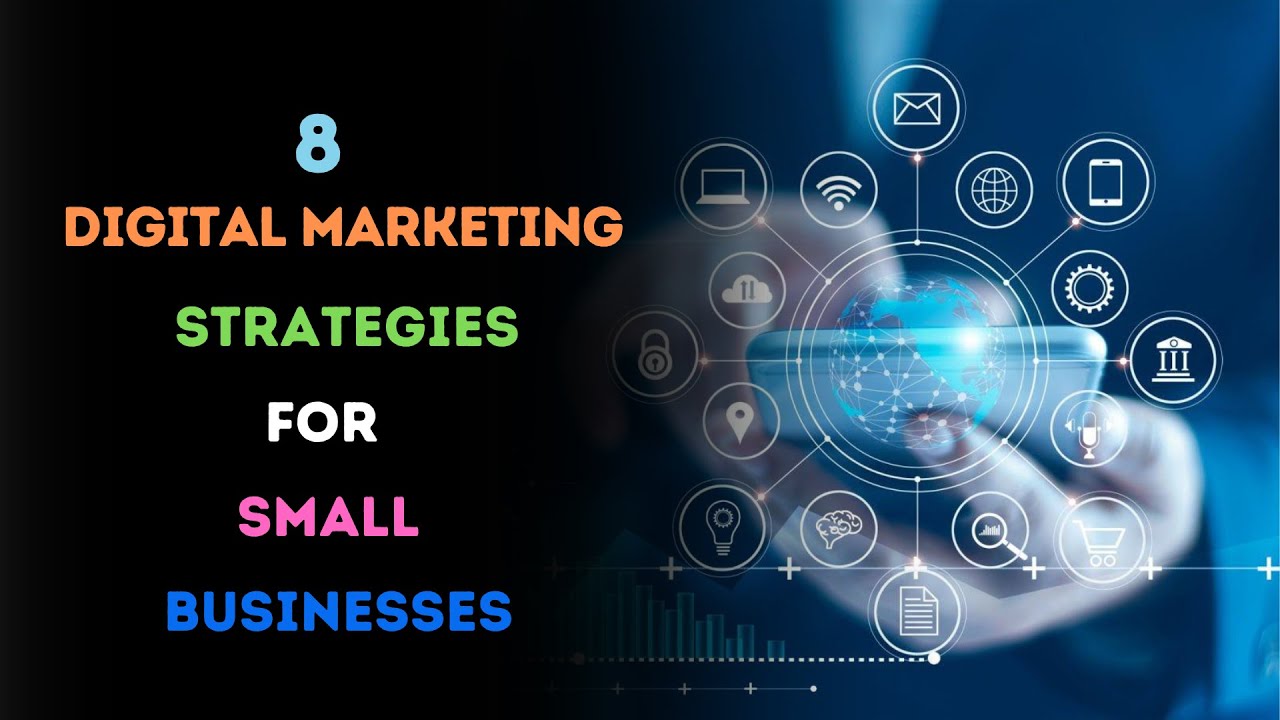
8 Digital Marketing Strategies for Small Businesses – [Hindi]- Quick Support

Ep294. Chat Gpt And The Future Of Ai In Chiropractic. Opportunities And Challenges. Angus Pyke
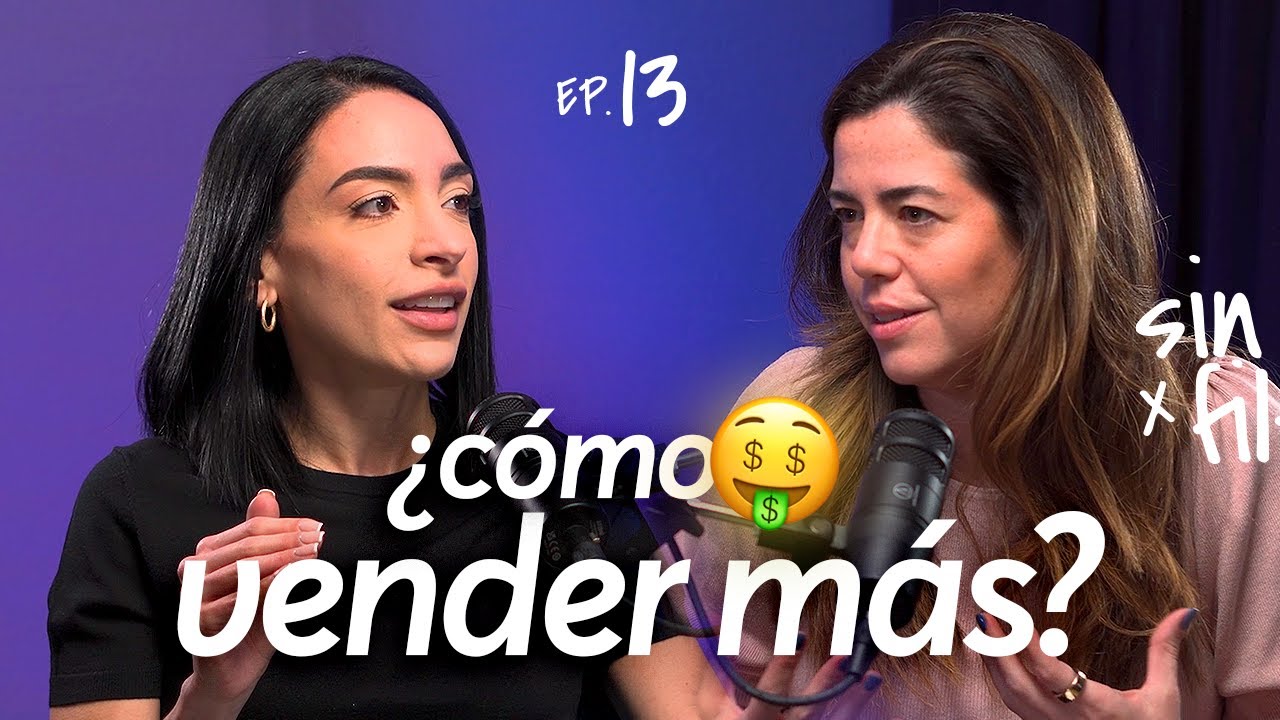
¿Cómo VENDER cualquier cosa? 💸💸 Técnicas efectivas de marketing ⎪EP13
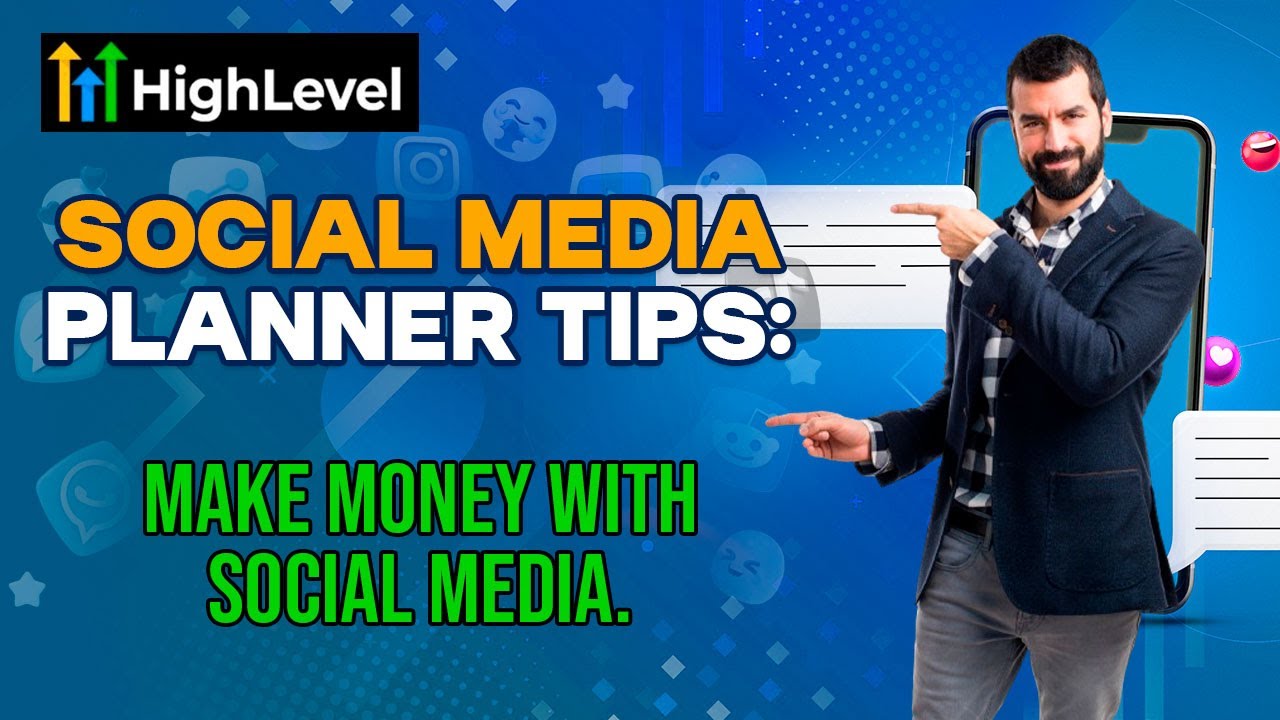
GoHighLevel Social Media Planner: How to Post Videos to Social Media Effectively
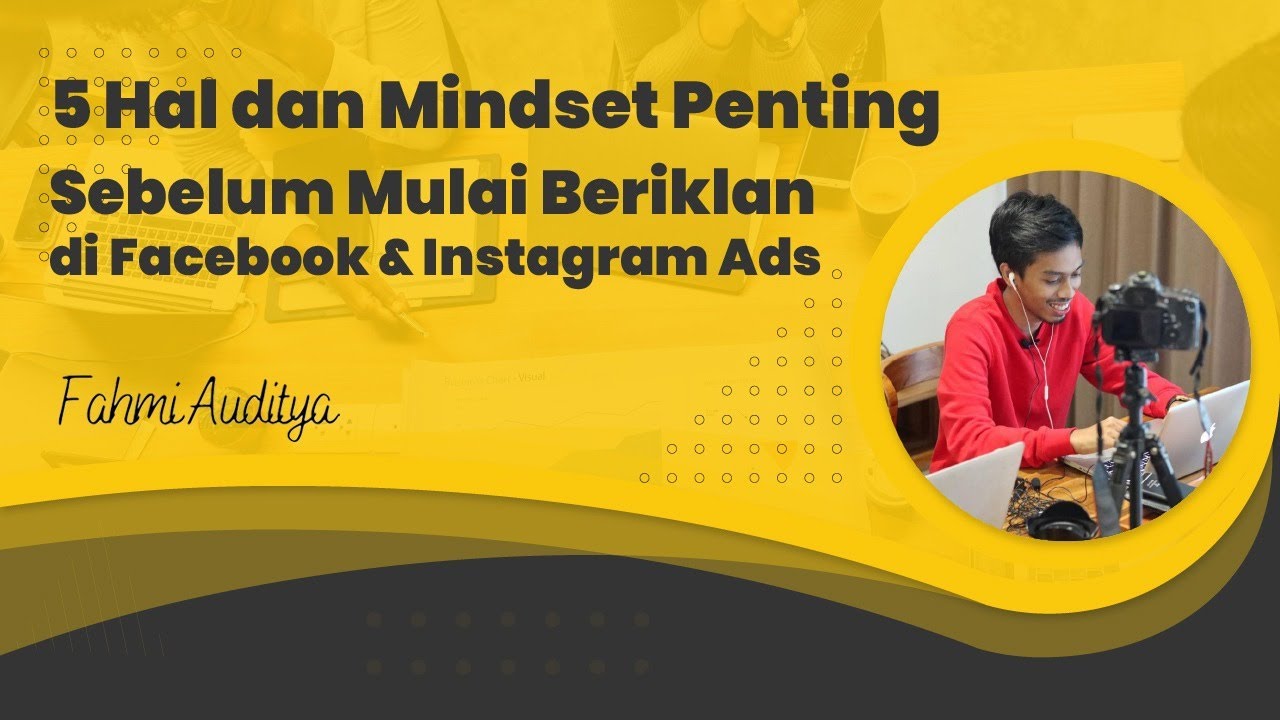
5 Hal & Mindset Penting Sebelum Beriklan di Facebook & Instagram Ads
5.0 / 5 (0 votes)