Introduction to Generative AI (Day 10/20) What are vector databases?
Summary
TLDRThe script delves into the workings of the Retrieval-Augmented Generation (RAG) model, highlighting its efficiency in extracting pertinent information from a knowledge source. By breaking down the source into segments, computing their vector representations, and storing them in a vector or embedding database, RAG expedites the process of finding relevant data. When a new question is posed, the model computes its vector and swiftly searches the database for the most pertinent vectors, using the corresponding text as context to formulate an accurate response. This method ensures a faster and more precise retrieval of information, akin to efficiently navigating through pages during an open-book exam.
Takeaways
- 📚 The script discusses the importance of using a Retrieval-Augmented Generation (RAG) model to make language models more effective.
- 🔍 RAG retrieves the most relevant information from a knowledge source to enhance the language model's response generation.
- 📈 The process involves breaking down the knowledge source into smaller chunks to facilitate efficient retrieval.
- 📝 These chunks are then converted into vector representations and stored in a vector or embedding database.
- 🔎 When a new question is asked, the RAG model computes the question's vector and searches the database for the most relevant vectors.
- 📑 The corresponding text chunks from the knowledge source are used as context to help the language model generate a better answer.
- 🚀 Vector databases are crucial for speeding up the process of finding relevant information due to their optimization for vector operations.
- 🧭 They allow for quick searches and are essential for the RAG model to function effectively.
- 🔑 The method used to identify useful parts of the knowledge source is akin to finding the right pages or lines in a book during an open book exam.
- 💡 The script emphasizes the efficiency and effectiveness of using vector databases in conjunction with RAG for improved language model performance.
- 🌐 The process described highlights the integration of retrieval mechanisms with language models to enhance their ability to provide contextually relevant answers.
Q & A
What is the primary function of RAG in the context of the script?
-RAG, or Retrieval-Augmented Generation, is designed to retrieve the most relevant information from a knowledge source and append it as context to assist a language model in generating the best possible answer.
Why is it necessary to break down the knowledge source into smaller chunks?
-Breaking down the knowledge source into smaller chunks allows for more efficient computation of their vector representations, which is essential for identifying the most relevant parts of the knowledge source in response to a query.
What is a vector database or an embedding database in the context of RAG?
-A vector database or an embedding database is a system used to store the vector representations of the smaller chunks of the knowledge source, facilitating quick searches and retrieval of the most relevant information.
How does the RAG system respond to a new question?
-When the RAG system receives a new question, it computes the question's vector representation and searches the vector database to find the most relevant vectors from the knowledge source.
What is the significance of computing the question's vector representation in RAG?
-Computing the question's vector representation is crucial for the RAG system to effectively search the vector database and retrieve the most relevant information chunks that can be used as context for the language model.
How do vector databases optimize the process of finding relevant information?
-Vector databases are optimized for working with vectors, allowing for quick searches and efficient retrieval of the most relevant information, which speeds up the process of answering queries.
What is the role of the language model (LM) in the RAG process?
-The language model (LM) uses the retrieved, contextually relevant information to generate the best possible answer to the given question.
How does the RAG system compare to an open book exam scenario?
-The RAG system is similar to finding the right pages or lines in a book during an open book exam, where the goal is to quickly identify and utilize the most relevant information.
What is the importance of identifying useful parts of the knowledge source in RAG?
-Identifying the useful parts of the knowledge source is key to providing accurate and relevant answers, as it ensures that the language model is provided with the most pertinent information to generate its response.
How does the RAG system ensure the relevance of the retrieved information?
-The RAG system ensures the relevance of the retrieved information by using vector representations and searching the vector database for the most closely matching vectors to the question's vector representation.
What are the advantages of using a vector database in the RAG system?
-The advantages of using a vector database in the RAG system include faster retrieval of information, optimization for vector-based searches, and the ability to handle large volumes of data efficiently.
Outlines
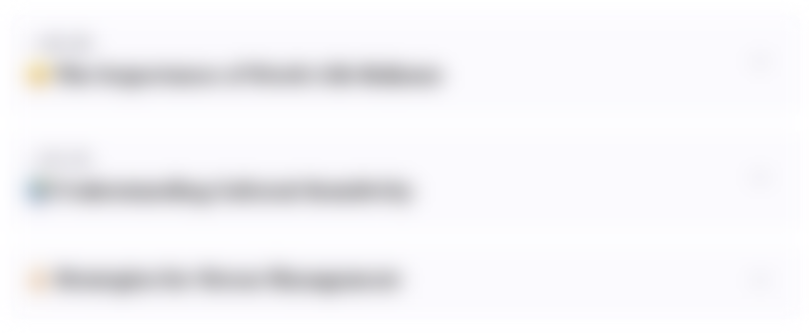
Esta sección está disponible solo para usuarios con suscripción. Por favor, mejora tu plan para acceder a esta parte.
Mejorar ahoraMindmap
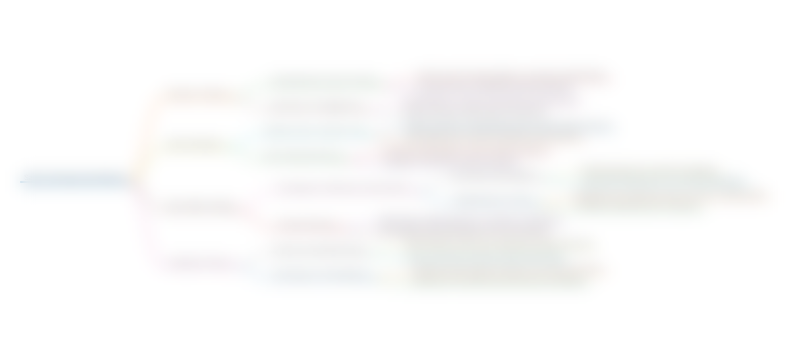
Esta sección está disponible solo para usuarios con suscripción. Por favor, mejora tu plan para acceder a esta parte.
Mejorar ahoraKeywords
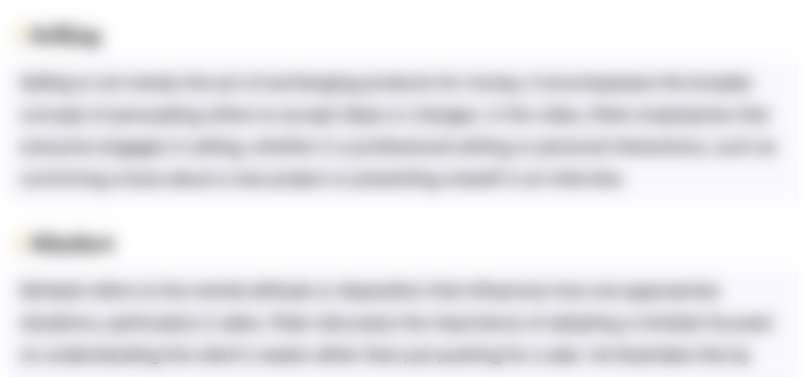
Esta sección está disponible solo para usuarios con suscripción. Por favor, mejora tu plan para acceder a esta parte.
Mejorar ahoraHighlights
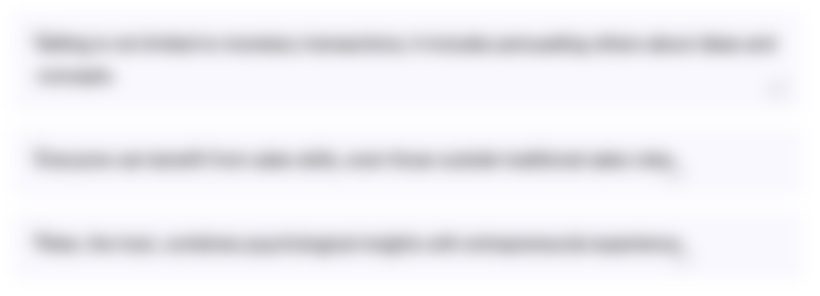
Esta sección está disponible solo para usuarios con suscripción. Por favor, mejora tu plan para acceder a esta parte.
Mejorar ahoraTranscripts
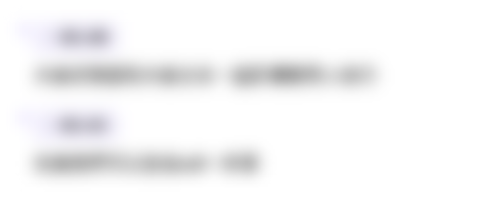
Esta sección está disponible solo para usuarios con suscripción. Por favor, mejora tu plan para acceder a esta parte.
Mejorar ahoraVer Más Videos Relacionados
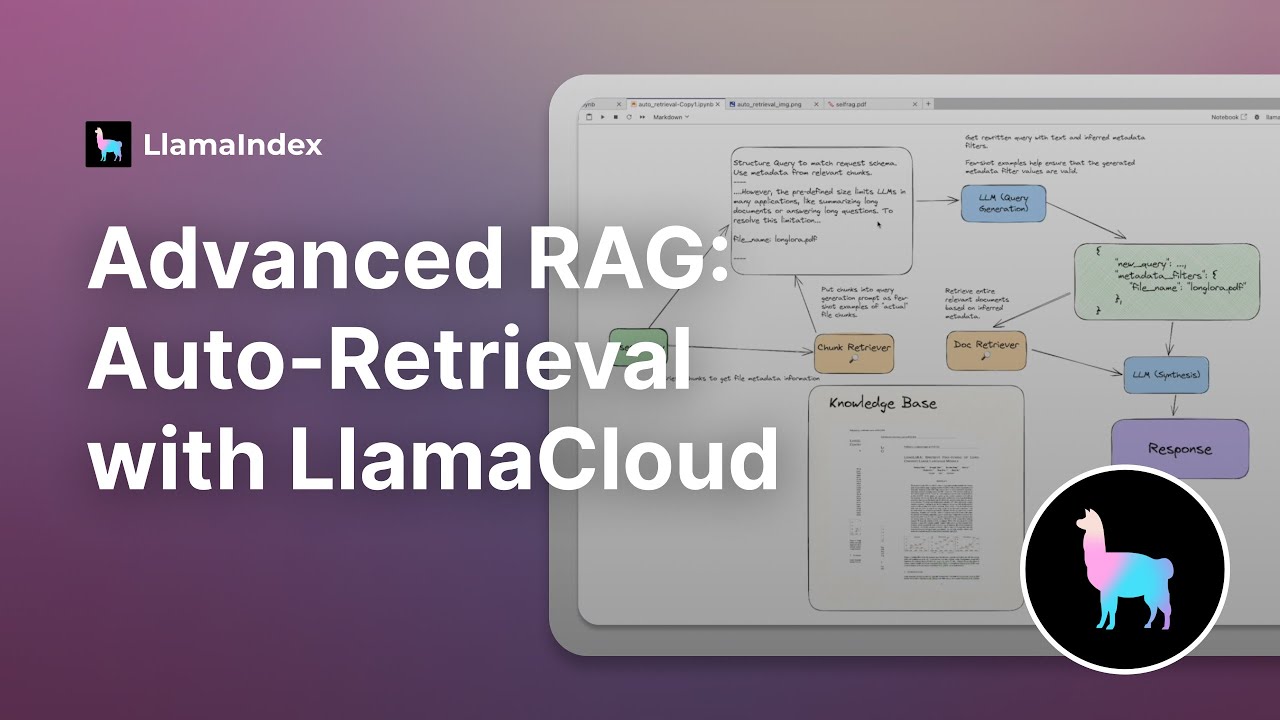
Advanced RAG: Auto-Retrieval (with LlamaCloud)
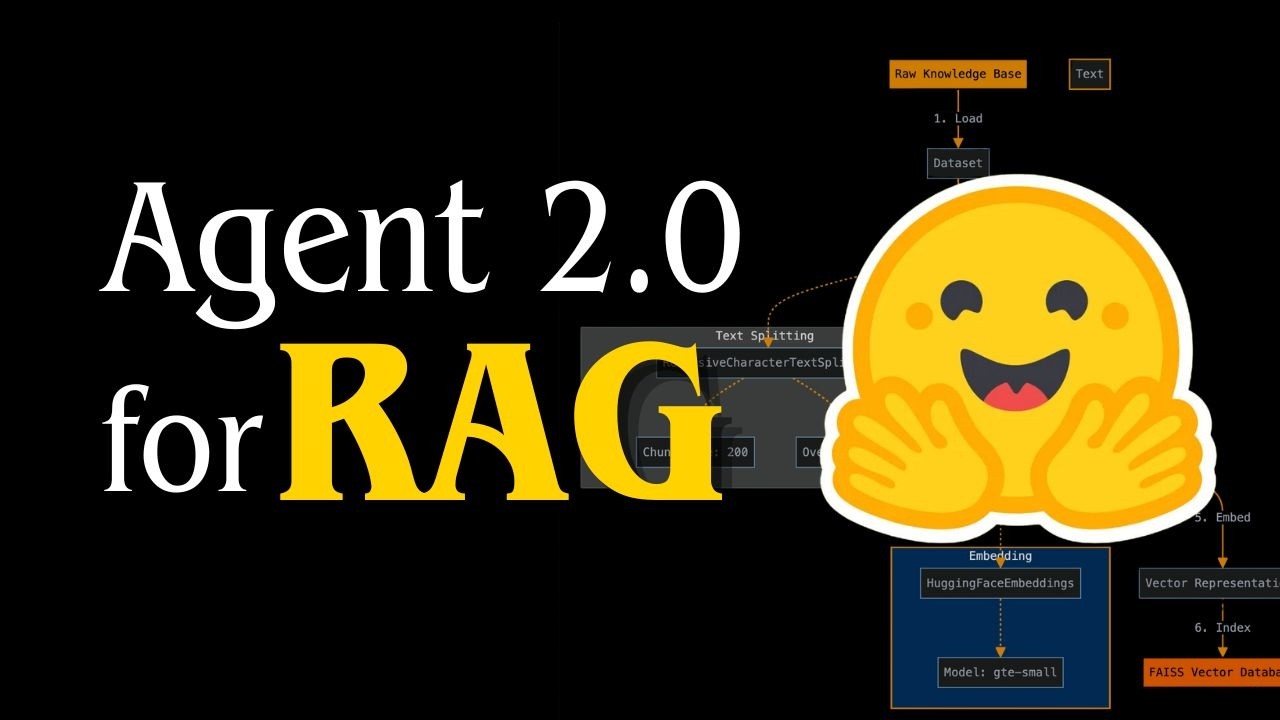
Agentic RAG: Make Chatting with Docs Smarter

Feed Your OWN Documents to a Local Large Language Model!

Build your own RAG (retrieval augmented generation) AI Chatbot using Python | Simple walkthrough
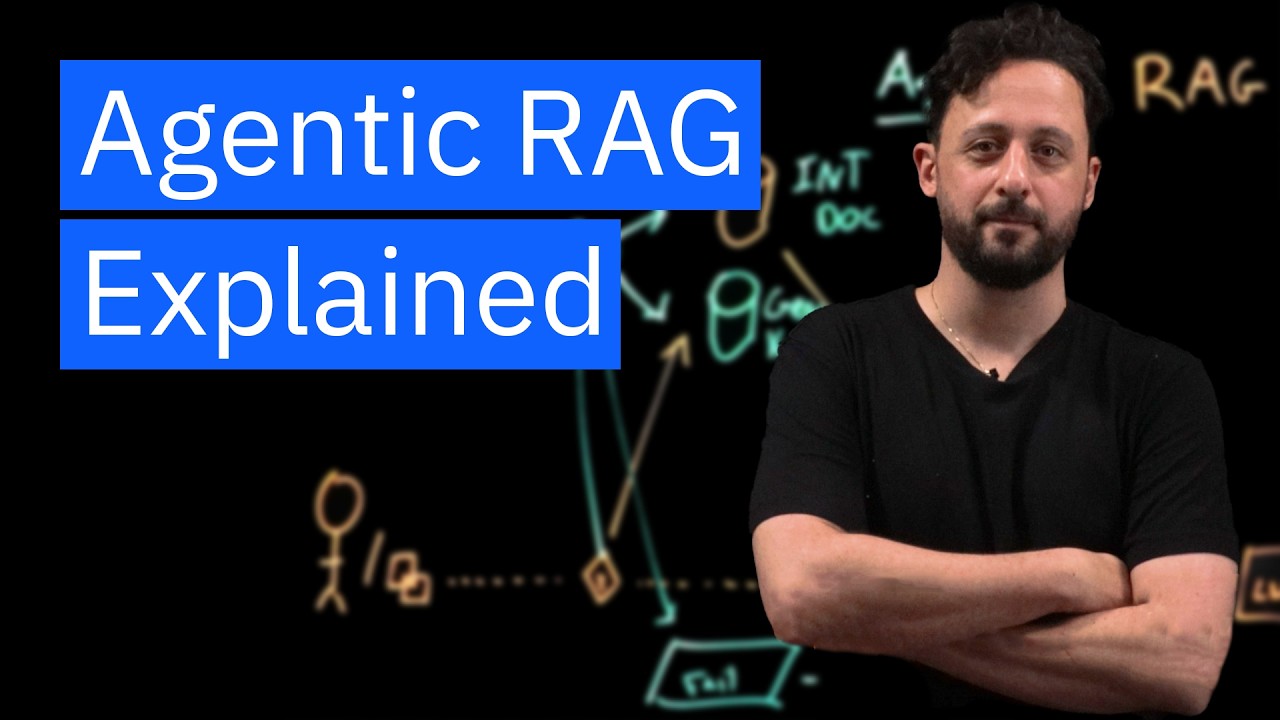
What is Agentic RAG?
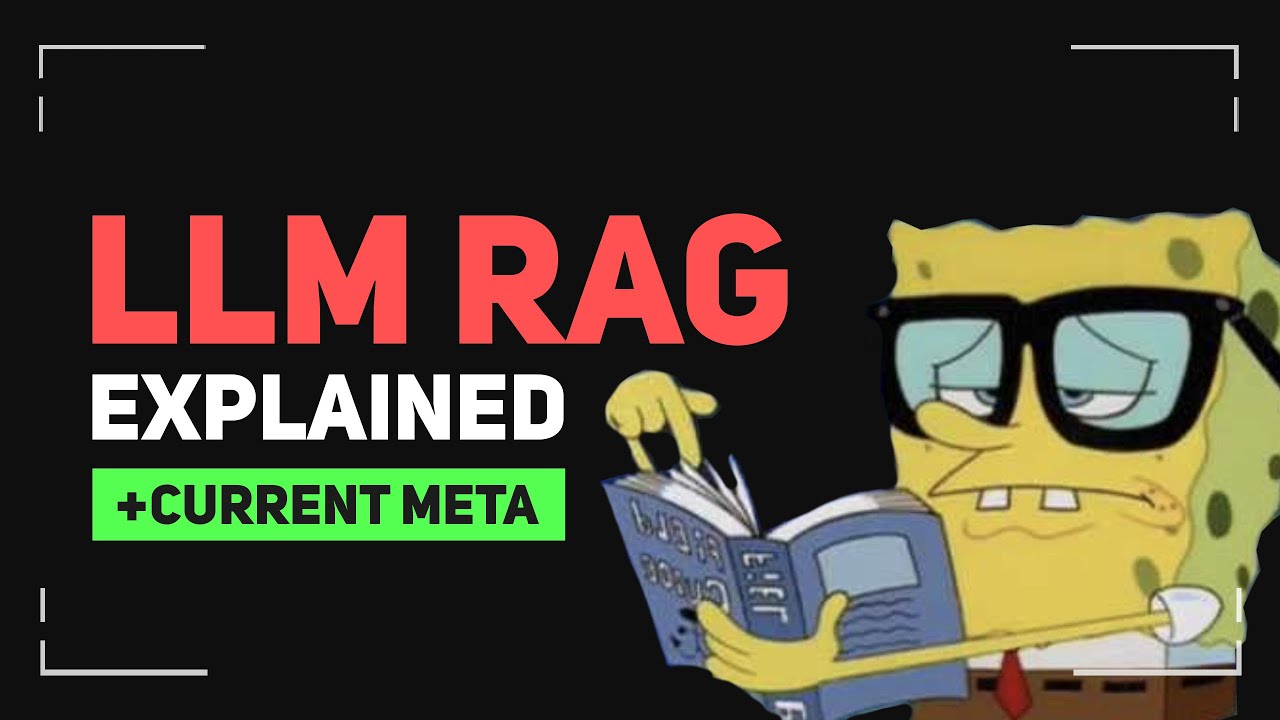
How RAG Turns AI Chatbots Into Something Practical
5.0 / 5 (0 votes)