Understanding Statistical Inference - statistics help
Summary
TLDRDr. Nic introduces statistical inference as a method to draw conclusions about a population from a sample. He differentiates it from descriptive statistics, emphasizing its ability to extend insights beyond the sample. The video covers the importance of sample representativeness, the inherent uncertainty in sampling, and the impact of sampling methods on inference accuracy. It also touches on the concepts of sampling error, confidence intervals, and hypothesis testing, all crucial for understanding statistical inference.
Takeaways
- 📊 Descriptive statistics summarize data using graphs and summary values like mean and interquartile range, helping identify relationships and patterns but not drawing conclusions beyond the data.
- 🔍 Inferential statistics allow making conclusions about the population from which a sample is drawn, extending insights beyond the sample itself.
- 🔑 The definition of inference is drawing conclusions about population parameters based on a sample, highlighting the importance of the sample as a representation of the population.
- 🍎 Examples of populations and samples include all apples in an orchard versus a sample of 100 apples, illustrating how samples are used to infer about the whole.
- 📈 There are three main ideas underlying inference: the sample as a good representation of the population, the uncertainty in how well the sample represents the population, and the importance of the way the sample is taken.
- 🤔 The expectation that a sample will represent the population is reasonable but not guaranteed due to sampling error and the fact that no sample is a perfect representation.
- 📊 Through simulation and probability theory, we can estimate what the population is likely to be like based on sample information, addressing the uncertainty of representation.
- 📉 A simulation can show the likelihood of certain outcomes in samples, such as the probability of a sample proportion deviating significantly from the population proportion.
- 📐 The margin of error, typically around 3% with a sample size of 1000, is used to create confidence intervals, providing a range where the population parameter is likely to fall.
- 🧐 In inferential statistics, hypothesis testing and p-values are used to evaluate claims about the population based on sample data.
- 🔄 The method of sampling is crucial for representativeness, with random sampling being ideal but often challenging and costly, especially with human populations.
Q & A
What are the two main focuses of statistical analysis?
-The two main focuses of statistical analysis are descriptive statistics and inferential statistics.
What do descriptive statistics do?
-Descriptive statistics summarize data using graphs and summary values, such as the mean and interquartile range. They help identify relationships and patterns but do not draw conclusions beyond the data.
How does inferential statistical analysis differ from descriptive statistics?
-Inferential statistical analysis allows us to make conclusions beyond the data we have to the population from which it was drawn, whereas descriptive statistics only summarize the existing data.
What is a definition of statistical inference?
-Statistical inference is the process of drawing conclusions about population parameters based on a sample taken from the population.
What are some examples of populations and samples?
-Examples include: the population of all apples in an orchard with a sample of 100 apples; the population of all chocolate bars produced on a machine with a sample of 30 bars; the population of all voters in New Zealand with a sample of 1000 people surveyed online.
What are the three main ideas underlying inference?
-The three main ideas are: 1) A sample is likely to be a good representation of the population. 2) There is an element of uncertainty in how well the sample represents the population. 3) The way the sample is taken matters.
Why is there uncertainty in how well a sample represents a population?
-There is uncertainty because a sample will never be a perfect representation of the population, leading to sampling error. Different samples from the same population can yield different results.
How can we estimate the likelihood of a sample proportion being close to the population proportion?
-We can estimate this using probability theory or simulations. For example, by simulating multiple samples from a population and analyzing the distribution of the sample proportions.
What is the margin of error, and how is it related to sample size?
-The margin of error indicates the range within which the sample proportion is expected to differ from the population proportion. For a sample size of 1000, the margin of error is typically about 3%.
Why does the way the sample is taken matter?
-The sample must be representative of the population, which is best achieved when each member of the population has an equal chance of being selected. This reduces non-sampling error.
Outlines
📊 Introduction to Statistical Inference
Dr. Nic introduces the concept of statistical inference, distinguishing it from descriptive statistics. Descriptive statistics summarize data through graphs and values like mean and interquartile range, helping to identify patterns but not making conclusions beyond the data. In contrast, inferential statistics use sample data to make conclusions about the larger population. The video defines inference as drawing conclusions about population parameters from a sample and provides examples of populations and samples, such as apples in an orchard, chocolate bars from a machine, and voters in New Zealand. It also discusses the three main ideas underlying inference: the representativeness of a sample, the inherent uncertainty in sampling, and the importance of the sampling method.
🔍 Sampling and Inference in Practice
This paragraph delves into the practical aspects of sampling and inference. It explains that a sample is expected to be a good representation of the population but acknowledges the uncertainty due to sampling error. The concept of margin of error and confidence intervals is introduced, which are used to estimate the range in which the population parameter is likely to fall. The video also touches on hypothesis testing and p-values, which are further explained in other videos. The importance of the sampling method is emphasized, stating that the sample must be representative and that each member of the population should have an equal chance of being selected. The challenges of obtaining random samples, especially with human populations, are discussed, and the video concludes by differentiating inference from causation, which is covered in separate videos. The video is produced by the Statistics Learning Centre, which offers additional resources on their website.
Mindmap
Keywords
💡Statistical Inference
💡Descriptive Statistics
💡Inferential Statistics
💡Population
💡Sample
💡Uncertainty
💡Sampling Error
💡Margin of Error
💡Confidence Intervals
💡Hypothesis Testing
💡Representative Sample
Highlights
Dr. Nic explains the concept of statistical inference in a video by the Statistics Learning Centre.
Statistical analysis is divided into descriptive and inferential statistics.
Descriptive statistics summarize data using graphs and summary values like mean and interquartile range.
Descriptive statistics help identify relationships and patterns but do not draw conclusions beyond the data.
Inferential statistics allow making conclusions about the population from which a sample is drawn.
Inference is defined as the process of drawing conclusions about population parameters based on a sample.
Examples of populations and samples include all apples in an orchard and a sample of 100 apples.
Inference can be used to draw conclusions about the weights of apples in an orchard from a sample.
Three main ideas underlie inference: sample representation, uncertainty, and the method of sampling.
A sample is expected to be a good representation of the population.
Uncertainty in sample representation is the reason for sampling error.
Simulation and probability theory help understand the population from sample information.
The probability of a sample proportion deviating significantly from the population proportion can be calculated.
Margin of error, derived from sample size, can be used to create confidence intervals.
Confidence intervals provide a range within which the population parameter is likely to be.
Inferential statistics also involve hypothesis testing, explained in other videos.
The method of sampling is crucial for the sample to be representative of the population.
Random sampling is ideal for ensuring representativeness, but can be difficult and costly with human populations.
The video concludes by emphasizing the focus on sample-to-population inference rather than causation.
The video was produced by the Statistics Learning Centre, offering more resources on their website.
Transcripts
Statistics Learning Centre presents
Understanding Statistical Inference.
Hi, I'm Dr Nic,
and in this video,
I'm going to explain what statistical inference is.
Statistical analysis has two main focuses:
1. Descriptive statistics, and
2. Inferential statistics.
Descriptive statistics summarise data
using graphs and summary values
such as the mean and interquartile range.
Descriptive statistics can help us identify
relationships and patterns.
Descriptive statistics do not draw conclusions
beyond the data we already have.
Inferential statistical analysis
does allow us to make conclusions
beyond the data we have
to the population
from which it was drawn.
A definition of inference is:
The process of drawing conclusions
about population parameters
based on a sample taken from the population.
The sample
is the data we collect from the population.
Here are some examples
of populations and samples.
The population is all the apples in an orchard.
A sample is 100 apples we pick from the orchard.
The population is all the chocolate bars we produce on a machine.
A sample is the 30 bars we choose to melt down
and weigh the nuts from.
The population is all the voters in New Zealand.
A sample is 1000 people who are asked their opinion
in an online survey.
In each of these examples,
we can use the data from our sample
to draw some conclusions about the objects or people
in the population.
For example,
we want to know about the weights of the apples in the orchard.
So we take a sample of apples and weigh them
and use that information
to draw conclusions
about the weights of apples
in the whole orchard.
There are 3 main ideas underlying inference:
1. A sample is likely to be a good representation of the population.
2. There is an element of uncertainty
as to how well the sample represents the population.
And,
3. The way the sample is taken matters.
First, it is reasonable to expect that a sample of objects
from a population
will represent the population.
If our orchard has only red apples
of a fairly consistent size,
then we would expect the sample to contain red apples
of a fairly consistent size.
If our machine is producing chocolate bars
with a percentage of nuts within a certain range,
then we would expect the sample to also have nuts within that range.
If 40% of the people in New Zealand believe that the economy is getting worse,
then about 40% of the sample will also believe that.
Second, there is an element of uncertainty
as to how well the sample represents the population.
A sample will never be a perfect representation
of the population from which it is drawn.
This is the reason for sampling error.
We have a video about sampling error.
Nor will all the samples drawn from the same population
be the same.
But through simulation and probability theory,
we can get an idea of what the population is likely to be like
from the information the sample gives us.
For example,
let us assume that 40% of the population of New Zealand
think the economy is getting worse.
If we took a sample of 1000 people
how likely is it that 50% or more of them
will say that they think the economy is getting worse?
We can work this out
using probability theory,
or we can run a simulation on the computer
to see what we would expect
from a whole lot of samples of size 1000
taken from a population with 40% thinking the economy is getting worse.
This graph is the result of a simulation
with 1000 samples of 1000 people each
drawn from a population with a proportion of success
of 0.4 or 40%.
We define "success" as being
that the person thinks the economy is getting worse.
It turns out
that if the true proportion in the population is 40%,
the probability of getting a sample with 50% or more people
saying that they think the economy is getting worse
is zero.
It won't happen.
Just about always,
the sample proportion
will be within about 3% of the population proportion
when we use a sample size of 1000.
That 3% is called the margin of error.
It can be used to create confidence intervals.
Confidence intervals give a range within which we think
the population parameter is likely to be.
You can see more about confidence intervals
in our video,
"Understanding confidence intervals".
In inferential statistics we can also test hypotheses.
Our videos, "Understanding the p-value" and "Hypothesis testing" explain these.
The third principle is,
the way the sample is taken matters.
This principle relates to non-sampling error,
which we also have a video about.
The sample must be representative of the population.
And this happens best when each person or thing in the population
has an equal chance
of being selected in the sample.
In natural or manufacturing processes we may be able to take random samples
reasonably easily.
However, when our population consists of people,
this can be more difficult and costly.
So we do the best we can.
We explain this further in our video on sampling methods.
This video has been about sample-to-population inference.
We are interested in what the sample tells us about the population.
It is not about causation
which is covered in other videos.
This video was brought to you
by Statistics Learning Centre.
Visit our website for more resources to help you learn.
Ver Más Videos Relacionados

Understanding Confidence Intervals: Statistics Help

Statistical Inference: Introduction and Terminology (in Hindi)
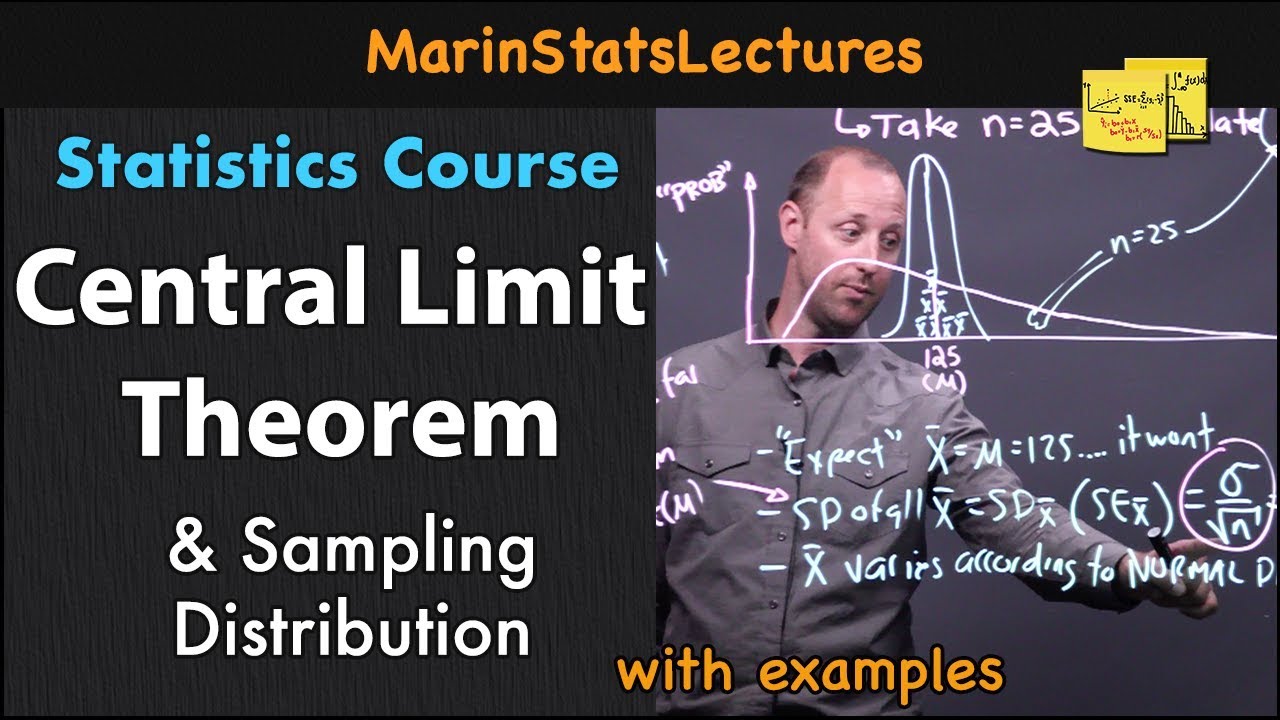
Central Limit Theorem & Sampling Distribution Concepts | Statistics Tutorial | MarinStatsLectures
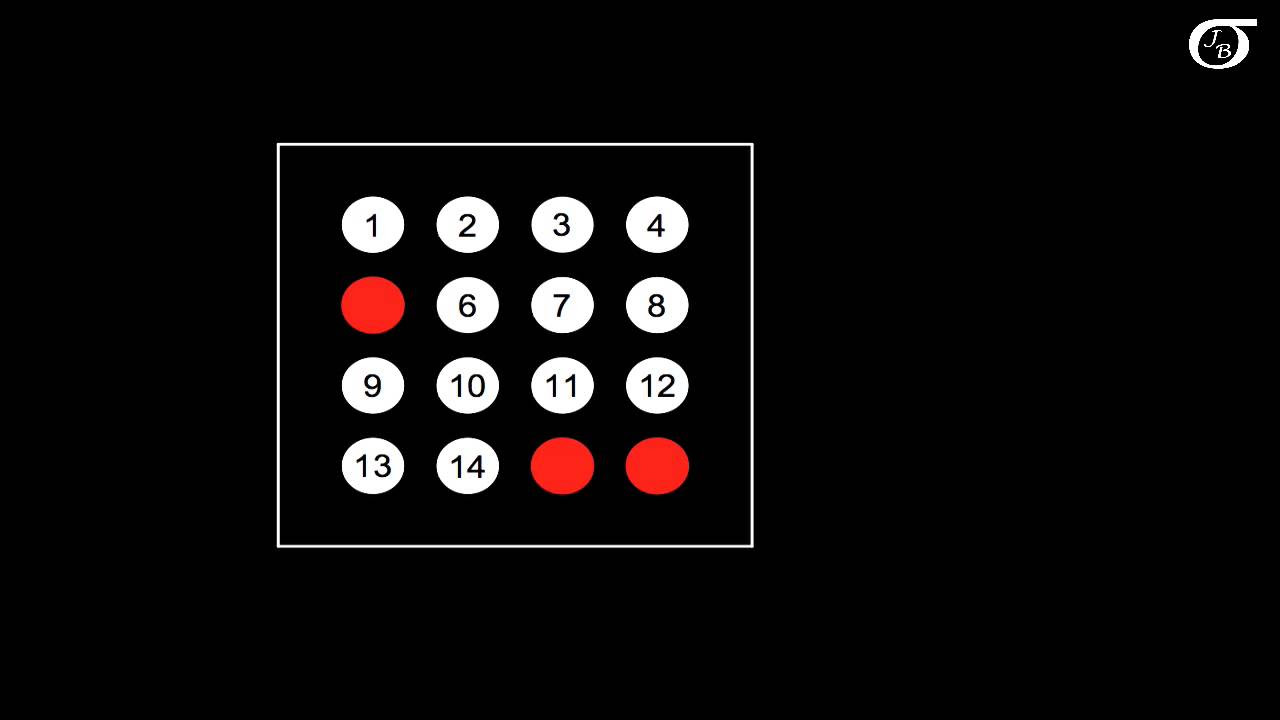
Sampling Distributions: Introduction to the Concept

100+ Statistics Concepts You Should Know
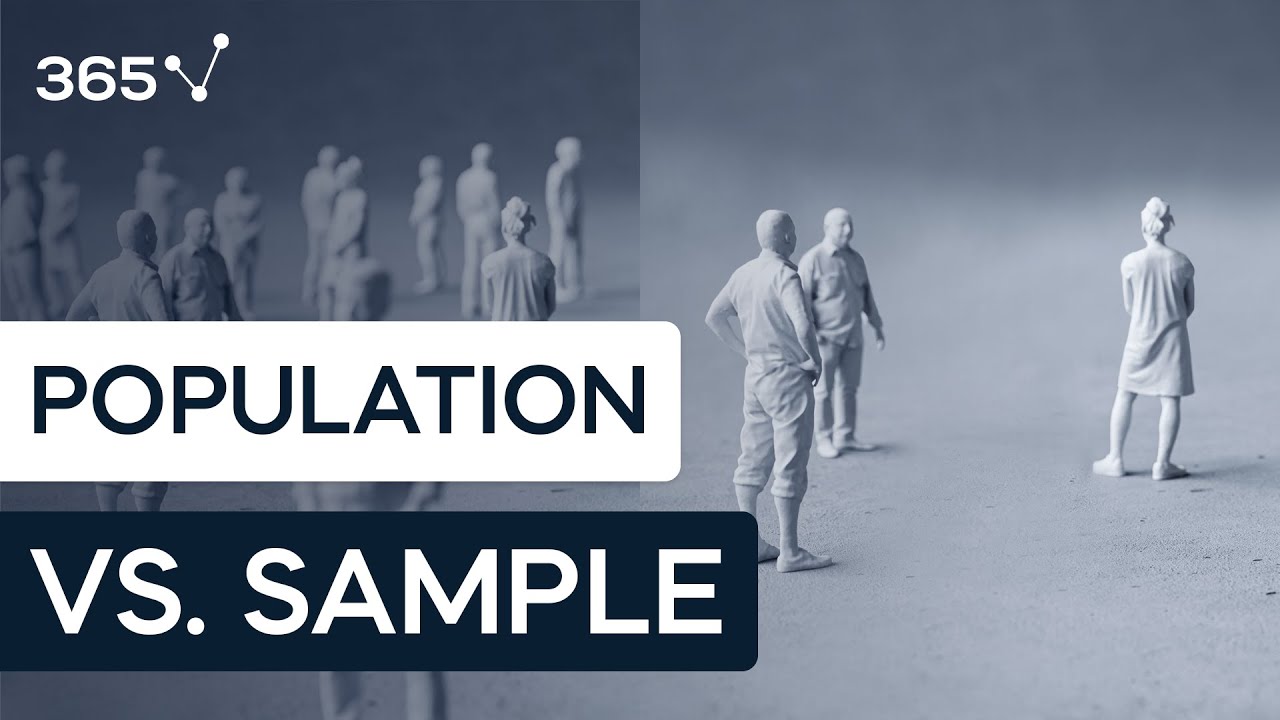
Population vs Sample
5.0 / 5 (0 votes)