Trip based fuel consumption modelling using Machine Learning Project built a website from scratch 😁👍
Summary
TLDRThe project FP presents a fuel consumption prediction model using machine learning. It utilizes various regression methods, with the decision tree model achieving 98% accuracy. The model considers parameters like distance, speed, temperature, and weather conditions to predict fuel consumption. The project includes a user-friendly website with a predictor feature, providing drivers with cost-efficient and environmentally sustainable fuel usage insights.
Takeaways
- 📊 **Data-Driven Model**: The project utilizes a dataset named 'measurements.csv' for fuel consumption prediction.
- 🌐 **Web Integration**: A web application is developed to interface with the prediction model using Python.
- 🔍 **Data Visualization**: The team performed data visualization as part of their analysis process.
- 🤖 **Machine Learning Models**: Various regression models were tested including Linear Regression, Lasso Regression, Decision Tree, Random Forest, and SVM.
- 🏆 **Best Model Chosen**: The Decision Tree model achieved the highest accuracy at 98% and was selected for deployment.
- 📝 **Model Persistence**: The trained Decision Tree model is saved using a 'pickle' file for later use.
- 💻 **Web Development**: The project features a web application with HTML pages connected via Python.
- 🔗 **Live Website**: A live website is demonstrated, accessible through a provided link.
- 📈 **Input Parameters**: Users can input parameters such as distance, speed, temperature, and weather conditions to predict fuel consumption.
- 🌍 **Global Impact**: The project aids drivers and travel managers in planning fuel needs and promoting cost efficiency and environmental sustainability.
- 📦 **Deployment**: The model is deployed through a command-line interface, showcasing its practical application.
Q & A
What is the main focus of the project FP?
-The main focus of the project FP is to develop a fuel consumption prediction model using machine learning.
What type of data file is used for the project?
-The project uses a CSV file named 'measurements' for the dataset.
What are the different machine learning models explored in the project?
-The project explored various regression models including linear regression, Lasso regression, decision tree model, random forest model, and SVM.
Which evaluation metric was used to compare the models?
-The R-squared score was used as the evaluation metric to compare the models.
Which model provided the best accuracy according to the R-squared score?
-The decision tree model provided the best accuracy with an R-squared score of 98%.
How is the decision tree model's performance utilized in the project?
-The decision tree model is used to predict fuel consumption, and the model is dumped into a pickle file for later use.
What is the programming language used to connect the web pages with the Python language?
-The project uses Flask, a micro web framework written in Python, to connect the web pages.
How is the project deployed and accessed?
-The project is deployed by running a Python script that starts a web server, and it can be accessed through a provided link in a web browser like Microsoft Edge.
What are the parameters required for the fuel consumption prediction model?
-The parameters required include distance, speed, temperature inside and outside the vehicle, and whether the weather is rainy or sunny.
What is the unit of fuel consumption predicted by the model?
-The model predicts fuel consumption in liters per 100 kilometers.
How does the project help drivers or travel managers?
-The project helps drivers or travel managers by predicting the fuel needed for a trip, suggesting cost-efficient methods, and promoting environmental sustainability.
What additional features are available on the project's website?
-The website includes pages for the home, predictor, about us, team details, and a contact us section.
Outlines
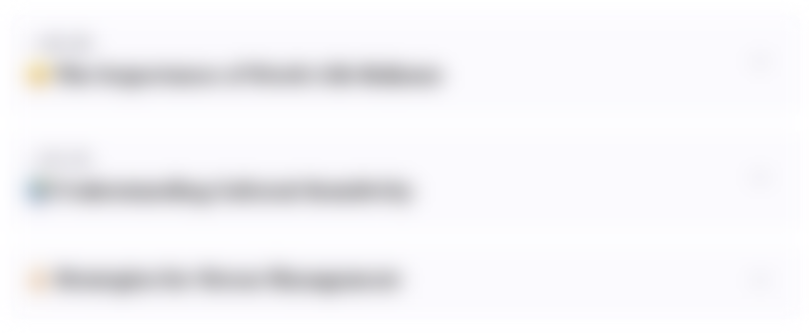
Esta sección está disponible solo para usuarios con suscripción. Por favor, mejora tu plan para acceder a esta parte.
Mejorar ahoraMindmap
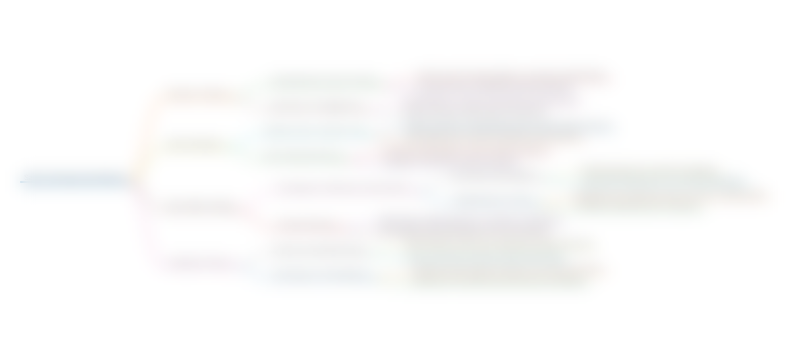
Esta sección está disponible solo para usuarios con suscripción. Por favor, mejora tu plan para acceder a esta parte.
Mejorar ahoraKeywords
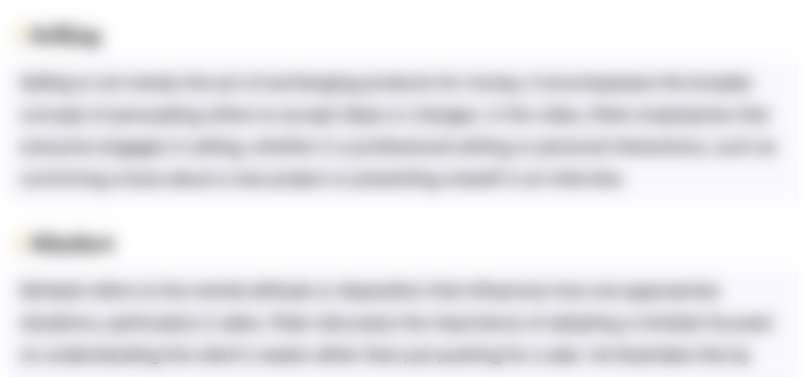
Esta sección está disponible solo para usuarios con suscripción. Por favor, mejora tu plan para acceder a esta parte.
Mejorar ahoraHighlights
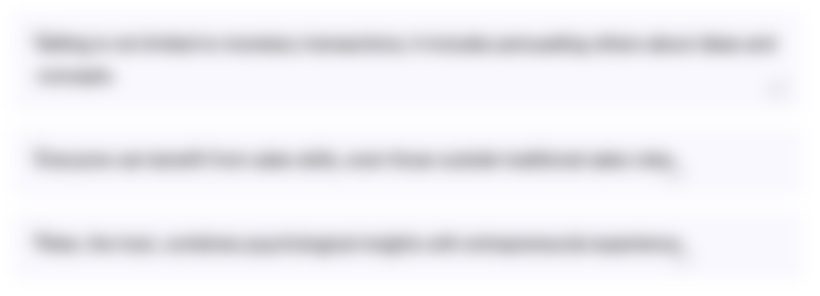
Esta sección está disponible solo para usuarios con suscripción. Por favor, mejora tu plan para acceder a esta parte.
Mejorar ahoraTranscripts
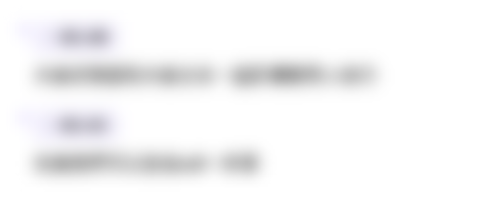
Esta sección está disponible solo para usuarios con suscripción. Por favor, mejora tu plan para acceder a esta parte.
Mejorar ahoraVer Más Videos Relacionados
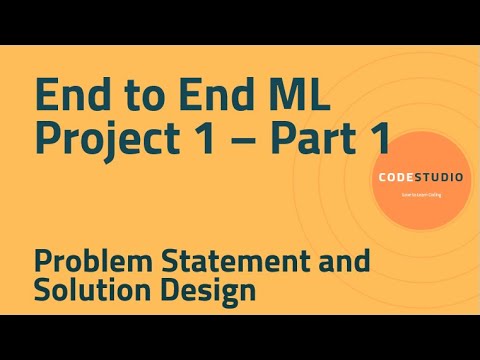
End to End ML Project 1 - P1 - Problem Statement and Solution Design

Fake Profile Detection on Social Networking Websites using Machine Learning | Python IEEE Project

Prediksi Penyakit Serangan Jantung | Machine Learning Project 11

ML.NP1.1 Diabetes Prediction Part - 1
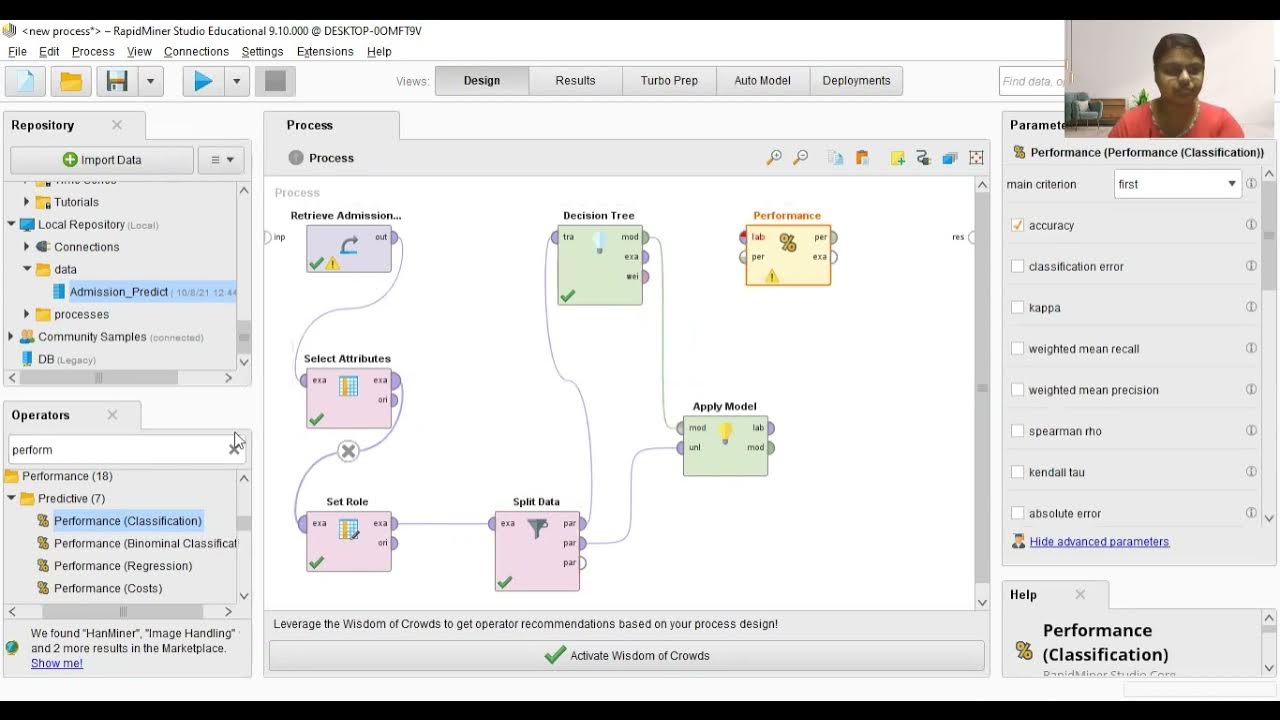
Decision Tree and Logistic Regression using RapidMiner Studio ( Gyanadipta Mohanty 19BCE1224)

AI Vs Kebakaran Hutan: Teknologi Cerdas Hadang Bencana Alam
5.0 / 5 (0 votes)