Jennifer Golbeck: The curly fry conundrum: Why social media "likes" say more than you might think
Summary
TLDRThe speaker explores how social media transformed the web from a static space to an interactive platform driven by user-generated content. They discuss the vast amount of personal data shared online, often unknowingly, and how predictive models can reveal hidden traits. Using examples like Target's pregnancy score and Facebook 'likes,' the speaker highlights the power of data analysis and the lack of user control. They propose future solutions, including science-driven tools for user empowerment, while acknowledging the challenges of balancing privacy with data usage for online interactions.
Takeaways
- 🌐 The early web was static, with most content created by organizations or tech-savvy individuals.
- 📱 The rise of social media has transformed the web into an interactive platform where average users generate most of the content.
- 👥 Social media has enabled users to create online personas easily, leading to the widespread sharing of personal data.
- 🔍 Large companies, like Target, use behavioral patterns from users' data to predict personal attributes and events, such as pregnancy.
- 📊 Machine learning models can predict hidden user traits, like political preferences, intelligence, and even personality, based on subtle patterns in behavior and online actions.
- 🤖 Likes on platforms like Facebook, such as the example of 'curly fries,' can predict personal attributes due to patterns in social networks.
- 🛑 Users often lack control or understanding of how their data is used and what it reveals about them.
- ⚖️ Solutions to regain user control include policy and law changes, but these are unlikely due to the business models of social media companies and the slow political process.
- 🔐 Technological solutions, such as data encryption and transparent risk warnings, could give users more control over their data sharing decisions.
- 🔬 Scientists aim to empower users with tools that help them make informed choices about their data, focusing on improving online interactions rather than exploiting personal information.
Q & A
How did the web evolve from the first decade to the era of social media?
-In the first decade, the web was mostly static, with content created by organizations or tech-savvy individuals. The rise of social media in the early 2000s transformed the web into an interactive space where average users contribute content through platforms like YouTube, blogs, and social media posts.
What is homophily, and how does it relate to predicting traits online?
-Homophily is a sociological theory that suggests people are friends with others who are like them. In the context of online behavior, it helps explain how people with similar attributes, like intelligence, cluster together, allowing models to predict traits based on patterns of behavior.
What example is given to illustrate how companies predict personal traits based on seemingly irrelevant data?
-An example is Target predicting a teenage girl's pregnancy before she informed her parents. By analyzing her purchase history, including buying more vitamins or a large handbag, Target computed a 'pregnancy score' and used it to predict her due date.
How does liking something like curly fries on Facebook relate to predicting intelligence?
-Liking the curly fries page is indicative of high intelligence, not because of the content itself, but due to homophily. A smart person may have initially liked the page, and through their network of similarly smart friends, this action propagated, making it a signal of intelligence.
Why do companies like Facebook hold so much data about users, and what are the concerns around this?
-Social media companies rely on user data as their primary asset for generating revenue. The concern is that users often don't understand how their data is used or shared, and they have little control over it. This leads to privacy risks and potential misuse of personal information.
What are the potential dangers of using predictive models on social media data?
-These models can predict sensitive personal attributes, such as political preferences, sexual orientation, and drug use, without users' knowledge or consent. Such information could be exploited for non-altruistic purposes, like by employers making hiring decisions based on these predictions.
What challenges exist in addressing user control over their personal data?
-There are two primary challenges: the slow political process in enacting laws to protect data rights and the reliance of social media companies on user data for revenue. This makes it unlikely for companies to voluntarily give users full control over their data.
How can science help users regain control over their online data?
-Research can develop tools that inform users about the risks of their actions, such as liking a page or sharing information. This could help users make informed decisions about what data they share. Scientists could also work on encrypting data to protect user privacy.
What is the speaker’s perspective on balancing data prediction models and user control?
-The speaker believes that users should have control over how their data is used. Although predictive models are useful for enhancing online interactions, users should have the option to prevent data collection if they wish, even if that diminishes the models' effectiveness.
Why does the speaker think law reform on user data control is unlikely in the near future?
-The speaker observes that the political process is slow and unlikely to prioritize or effectively address the complexities of data privacy laws. Additionally, social media companies have a vested interest in maintaining control over user data for profit.
Outlines
🌐 The Evolution of the Web: From Static Pages to Social Media
The early internet was a static space where content was mostly created by tech-savvy individuals or organizations. The rise of social media in the early 2000s transformed it into an interactive platform where average users now generate the majority of content, such as YouTube videos, blog posts, and social media updates. Facebook, with 1.2 billion monthly users, illustrates this change, allowing users to create online personas without much technical skill. However, the massive amounts of personal data shared have led to unprecedented data collection on behavior, preferences, and demographics. While this data allows computer scientists to build predictive models for various hidden attributes, it raises concerns about users' lack of understanding and control over the information they unknowingly share.
🎯 Target's Predictive Data Insights and the Power of Subtle Behavior Patterns
The famous Target anecdote illustrates the predictive power of subtle behavior patterns. Target predicted a teenage girl's pregnancy using her purchase history to calculate a 'pregnancy score,' not by tracking obvious purchases like baby products but by analyzing minor changes in behavior, such as increased vitamin purchases or buying a larger handbag. This incident highlights how seemingly insignificant behaviors, when viewed in large datasets, can reveal hidden attributes. Similarly, social media platforms predict personal details like political preferences, personality traits, and even intelligence by analyzing patterns in user behavior, such as Facebook 'likes.' These predictive models demonstrate the growing capability of data analytics to infer sensitive personal information from seemingly trivial actions.
🍟 Curly Fries and the Science of Homophily: How Social Networks Reveal Hidden Traits
A study on Facebook 'likes' shows that seemingly irrelevant content, like liking a page for curly fries, can reveal surprising insights about users' intelligence. This phenomenon is explained by 'homophily,' the sociological theory that people tend to associate with others like themselves—smart people have smart friends. Over time, behaviors like liking a page can propagate through a network of similar individuals, creating patterns that reflect back shared traits, even if the content itself seems unrelated. This illustrates the complexity of understanding how online actions reflect personal characteristics, and the difficulty for users to grasp the hidden meaning behind their digital footprints.
🔐 The Problem of Data Control: Users' Limited Power Over Their Personal Data
Despite the complex nature of online data prediction, users have little control over how their data is used. The speaker presents the ethical dilemma of how data could be exploited by companies to predict traits such as drug use or team-working ability, and sell this information to HR departments without user consent. While policy and legal reform could help, the speaker is skeptical that political systems will enact necessary changes to give users control over their data. Moreover, many social media platforms rely on user data for revenue, making it unlikely that companies will willingly cede control to users.
🧪 Science as a Solution: Empowering Users Through Better Data Awareness
The speaker advocates for scientific research to empower users by giving them better control over their data. Science has developed the methods for predicting user traits, and similar research could create tools to inform users of the risks associated with sharing certain data online. By understanding how their actions contribute to data collection, users can make more informed decisions about what to share. The speaker also suggests research into encrypting user data, allowing people to control who can access their information. This approach offers a promising path toward balancing data collection with user autonomy, creating a more educated and empowered user base in the digital world.
Mindmap
Keywords
💡Static Web
💡Social Media
💡User-Generated Content
💡Data Privacy
💡Behavioral Data
💡Homophily
💡Predictive Models
💡Big Data
💡Online Persona
💡Control over Data
Highlights
The web in its early days was mostly static, with content put up by tech-savvy individuals or organizations.
Social media revolutionized the web by allowing average users to create most of the content, making it much more interactive.
Facebook has 1.2 billion monthly users, representing half of the Earth's internet population.
Massive amounts of personal data are now available online due to social media, leading to unprecedented levels of behavioral and demographic insights.
Computer scientists can now build models that predict hidden user attributes, such as political preference, personality, and even intelligence.
Companies like Target use predictive algorithms, such as pregnancy scores, based on seemingly unrelated purchases, to make accurate inferences about customers.
Small, seemingly insignificant behaviors on social media, like Facebook likes, can reveal hidden traits due to patterns in behavior across large groups.
A study found that liking a Facebook page for curly fries was one of the strongest indicators of high intelligence, due to the sociological concept of homophily.
The spread of likes or viral content follows similar patterns to how diseases spread through social networks.
Users currently lack control over how their data is used, and it can be exploited in ways they may not be aware of.
There is a potential threat of data being used for purposes like employment screenings, where attributes like drug use or team compatibility could be predicted.
Legal and policy changes are unlikely to happen quickly due to the complexity and the revenue models of social media companies relying on user data.
There is a need for scientific research to develop tools that give users better control over the data they share online.
One potential solution is encryption, where data is made invisible to third parties but accessible to chosen users.
The speaker advocates for informed consent in online data usage, ensuring users have control over how their data is utilized in the future.
Transcripts
If you remember that first decade of the web,
it was really a static place.
You could go online, you could look at pages,
and they were put up either by organizations
who had teams to do it
or by individuals who were really tech-savvy
for the time.
And with the rise of social media
and social networks in the early 2000s,
the web was completely changed
to a place where now the vast majority of content
we interact with is put up by average users,
either in YouTube videos or blog posts
or product reviews or social media postings.
And it's also become a much more interactive place,
where people are interacting with others,
they're commenting, they're sharing,
they're not just reading.
So Facebook is not the only place you can do this,
but it's the biggest,
and it serves to illustrate the numbers.
Facebook has 1.2 billion users per month.
So half the Earth's Internet population
is using Facebook.
They are a site, along with others,
that has allowed people to create an online persona
with very little technical skill,
and people responded by putting huge amounts
of personal data online.
So the result is that we have behavioral,
preference, demographic data
for hundreds of millions of people,
which is unprecedented in history.
And as a computer scientist, what this means is that
I've been able to build models
that can predict all sorts of hidden attributes
for all of you that you don't even know
you're sharing information about.
As scientists, we use that to help
the way people interact online,
but there's less altruistic applications,
and there's a problem in that users don't really
understand these techniques and how they work,
and even if they did, they don't have a lot of control over it.
So what I want to talk to you about today
is some of these things that we're able to do,
and then give us some ideas of how we might go forward
to move some control back into the hands of users.
So this is Target, the company.
I didn't just put that logo
on this poor, pregnant woman's belly.
You may have seen this anecdote that was printed
in Forbes magazine where Target
sent a flyer to this 15-year-old girl
with advertisements and coupons
for baby bottles and diapers and cribs
two weeks before she told her parents
that she was pregnant.
Yeah, the dad was really upset.
He said, "How did Target figure out
that this high school girl was pregnant
before she told her parents?"
It turns out that they have the purchase history
for hundreds of thousands of customers
and they compute what they call a pregnancy score,
which is not just whether or not a woman's pregnant,
but what her due date is.
And they compute that
not by looking at the obvious things,
like, she's buying a crib or baby clothes,
but things like, she bought more vitamins
than she normally had,
or she bought a handbag
that's big enough to hold diapers.
And by themselves, those purchases don't seem
like they might reveal a lot,
but it's a pattern of behavior that,
when you take it in the context of thousands of other people,
starts to actually reveal some insights.
So that's the kind of thing that we do
when we're predicting stuff about you on social media.
We're looking for little patterns of behavior that,
when you detect them among millions of people,
lets us find out all kinds of things.
So in my lab and with colleagues,
we've developed mechanisms where we can
quite accurately predict things
like your political preference,
your personality score, gender, sexual orientation,
religion, age, intelligence,
along with things like
how much you trust the people you know
and how strong those relationships are.
We can do all of this really well.
And again, it doesn't come from what you might
think of as obvious information.
So my favorite example is from this study
that was published this year
in the Proceedings of the National Academies.
If you Google this, you'll find it.
It's four pages, easy to read.
And they looked at just people's Facebook likes,
so just the things you like on Facebook,
and used that to predict all these attributes,
along with some other ones.
And in their paper they listed the five likes
that were most indicative of high intelligence.
And among those was liking a page
for curly fries. (Laughter)
Curly fries are delicious,
but liking them does not necessarily mean
that you're smarter than the average person.
So how is it that one of the strongest indicators
of your intelligence
is liking this page
when the content is totally irrelevant
to the attribute that's being predicted?
And it turns out that we have to look at
a whole bunch of underlying theories
to see why we're able to do this.
One of them is a sociological theory called homophily,
which basically says people are friends with people like them.
So if you're smart, you tend to be friends with smart people,
and if you're young, you tend to be friends with young people,
and this is well established
for hundreds of years.
We also know a lot
about how information spreads through networks.
It turns out things like viral videos
or Facebook likes or other information
spreads in exactly the same way
that diseases spread through social networks.
So this is something we've studied for a long time.
We have good models of it.
And so you can put those things together
and start seeing why things like this happen.
So if I were to give you a hypothesis,
it would be that a smart guy started this page,
or maybe one of the first people who liked it
would have scored high on that test.
And they liked it, and their friends saw it,
and by homophily, we know that he probably had smart friends,
and so it spread to them, and some of them liked it,
and they had smart friends,
and so it spread to them,
and so it propagated through the network
to a host of smart people,
so that by the end, the action
of liking the curly fries page
is indicative of high intelligence,
not because of the content,
but because the actual action of liking
reflects back the common attributes
of other people who have done it.
So this is pretty complicated stuff, right?
It's a hard thing to sit down and explain
to an average user, and even if you do,
what can the average user do about it?
How do you know that you've liked something
that indicates a trait for you
that's totally irrelevant to the content of what you've liked?
There's a lot of power that users don't have
to control how this data is used.
And I see that as a real problem going forward.
So I think there's a couple paths
that we want to look at
if we want to give users some control
over how this data is used,
because it's not always going to be used
for their benefit.
An example I often give is that,
if I ever get bored being a professor,
I'm going to go start a company
that predicts all of these attributes
and things like how well you work in teams
and if you're a drug user, if you're an alcoholic.
We know how to predict all that.
And I'm going to sell reports
to H.R. companies and big businesses
that want to hire you.
We totally can do that now.
I could start that business tomorrow,
and you would have absolutely no control
over me using your data like that.
That seems to me to be a problem.
So one of the paths we can go down
is the policy and law path.
And in some respects, I think that that would be most effective,
but the problem is we'd actually have to do it.
Observing our political process in action
makes me think it's highly unlikely
that we're going to get a bunch of representatives
to sit down, learn about this,
and then enact sweeping changes
to intellectual property law in the U.S.
so users control their data.
We could go the policy route,
where social media companies say,
you know what? You own your data.
You have total control over how it's used.
The problem is that the revenue models
for most social media companies
rely on sharing or exploiting users' data in some way.
It's sometimes said of Facebook that the users
aren't the customer, they're the product.
And so how do you get a company
to cede control of their main asset
back to the users?
It's possible, but I don't think it's something
that we're going to see change quickly.
So I think the other path
that we can go down that's going to be more effective
is one of more science.
It's doing science that allowed us to develop
all these mechanisms for computing
this personal data in the first place.
And it's actually very similar research
that we'd have to do
if we want to develop mechanisms
that can say to a user,
"Here's the risk of that action you just took."
By liking that Facebook page,
or by sharing this piece of personal information,
you've now improved my ability
to predict whether or not you're using drugs
or whether or not you get along well in the workplace.
And that, I think, can affect whether or not
people want to share something,
keep it private, or just keep it offline altogether.
We can also look at things like
allowing people to encrypt data that they upload,
so it's kind of invisible and worthless
to sites like Facebook
or third party services that access it,
but that select users who the person who posted it
want to see it have access to see it.
This is all super exciting research
from an intellectual perspective,
and so scientists are going to be willing to do it.
So that gives us an advantage over the law side.
One of the problems that people bring up
when I talk about this is, they say,
you know, if people start keeping all this data private,
all those methods that you've been developing
to predict their traits are going to fail.
And I say, absolutely, and for me, that's success,
because as a scientist,
my goal is not to infer information about users,
it's to improve the way people interact online.
And sometimes that involves inferring things about them,
but if users don't want me to use that data,
I think they should have the right to do that.
I want users to be informed and consenting
users of the tools that we develop.
And so I think encouraging this kind of science
and supporting researchers
who want to cede some of that control back to users
and away from the social media companies
means that going forward, as these tools evolve
and advance,
means that we're going to have an educated
and empowered user base,
and I think all of us can agree
that that's a pretty ideal way to go forward.
Thank you.
(Applause)
Ver Más Videos Relacionados
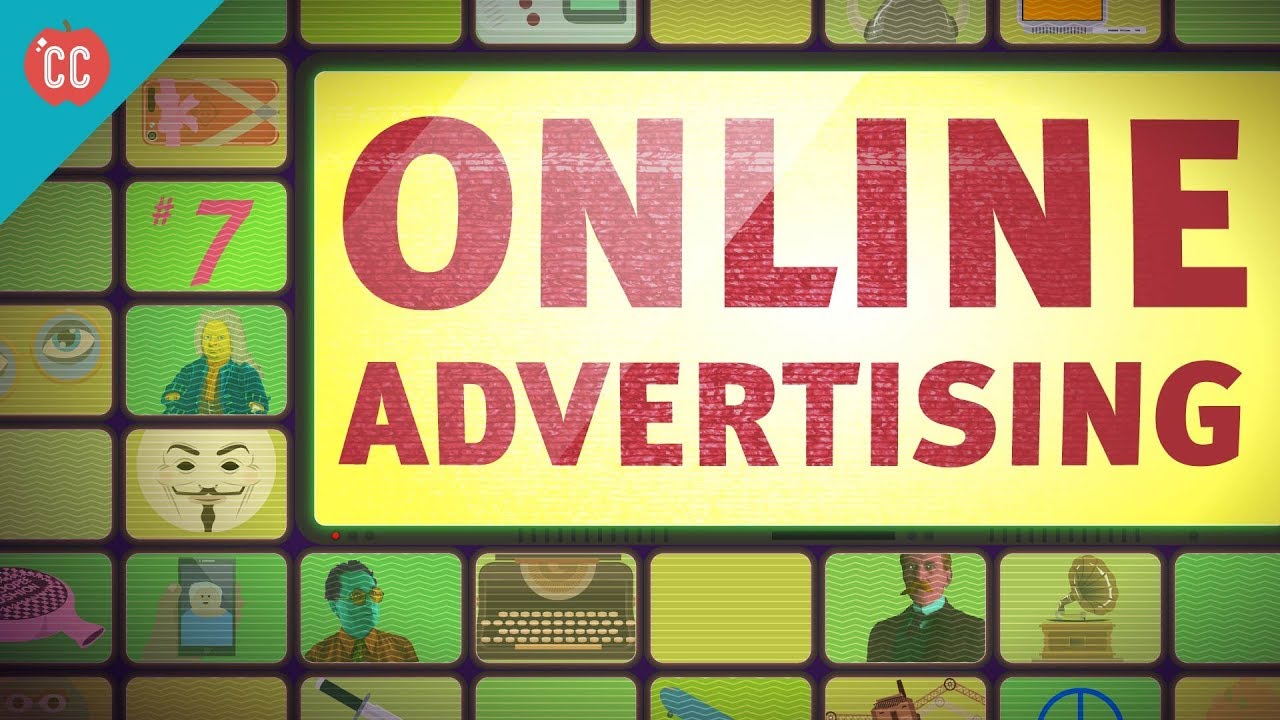
Online Advertising: Crash Course Media Literacy #7

How Social Media Uses Your Data
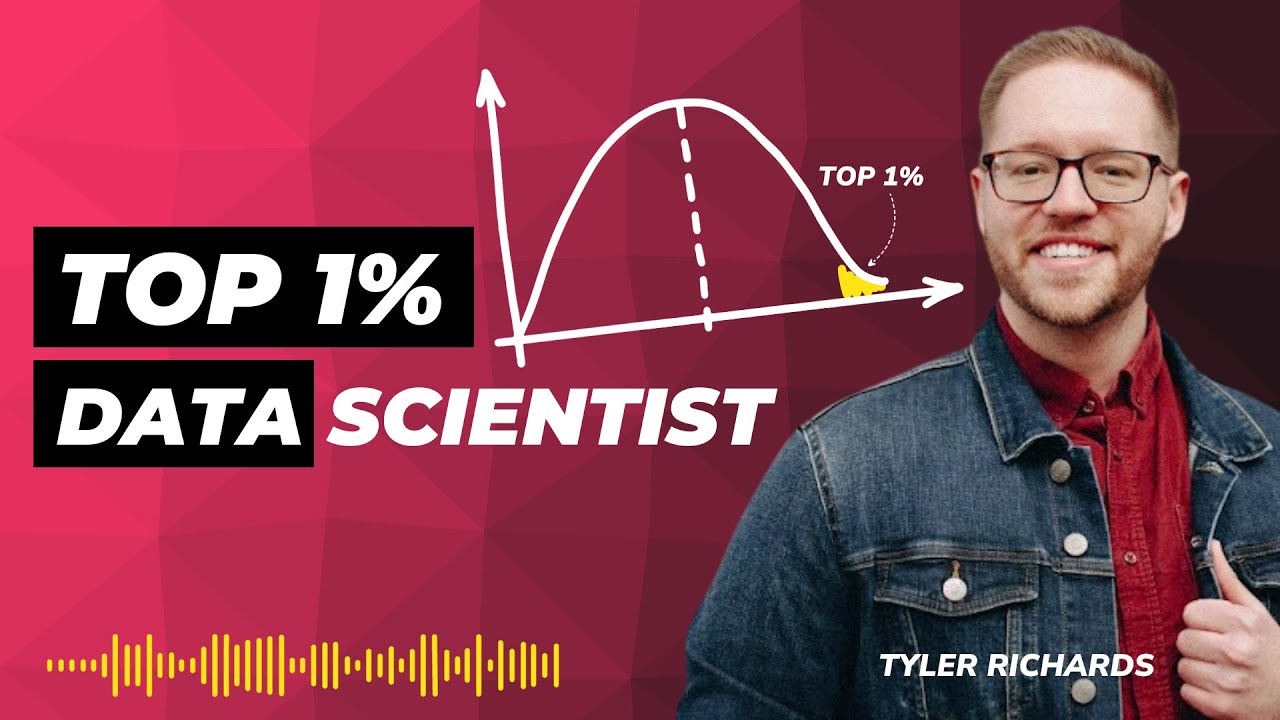
How to Get Ahead of 99% of Data Scientists with Streamlit (Tips from Tyler Richards)
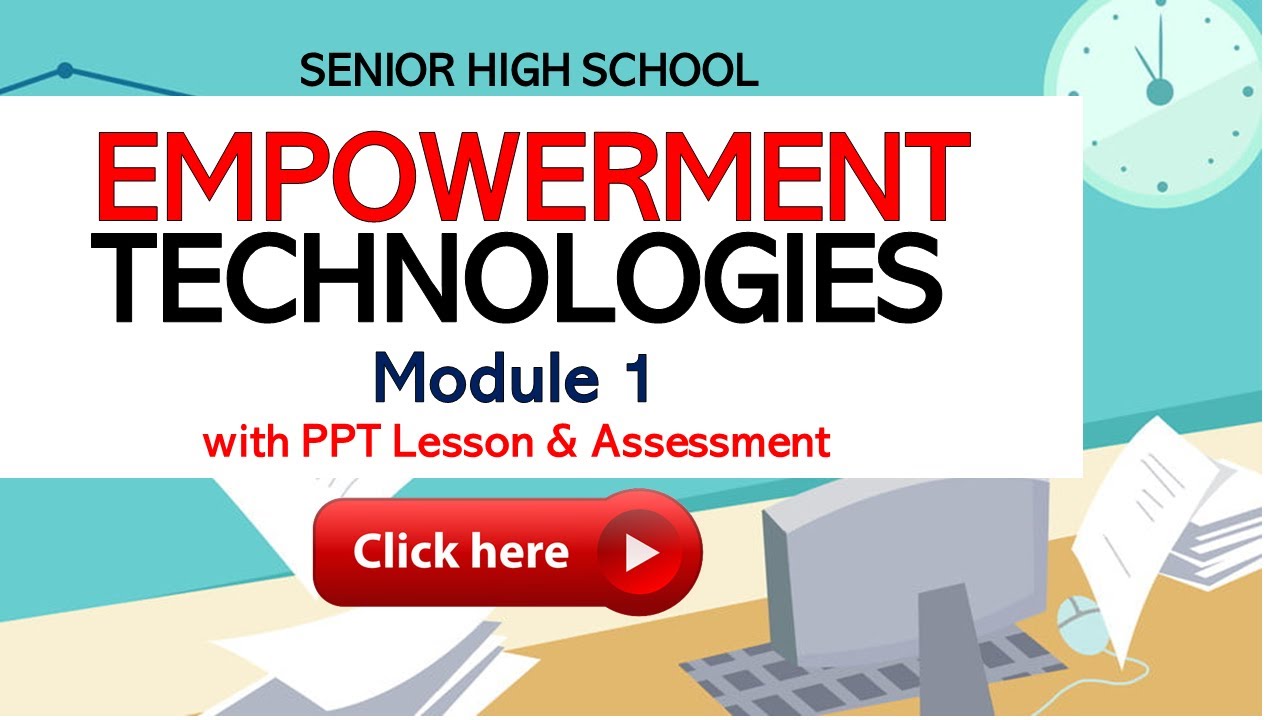
EMPOWERMENT TECHNOLOGIES - Module 1

TEDxMidAtlantic 2011 - Duncan Watts - The Myth of Common Sense
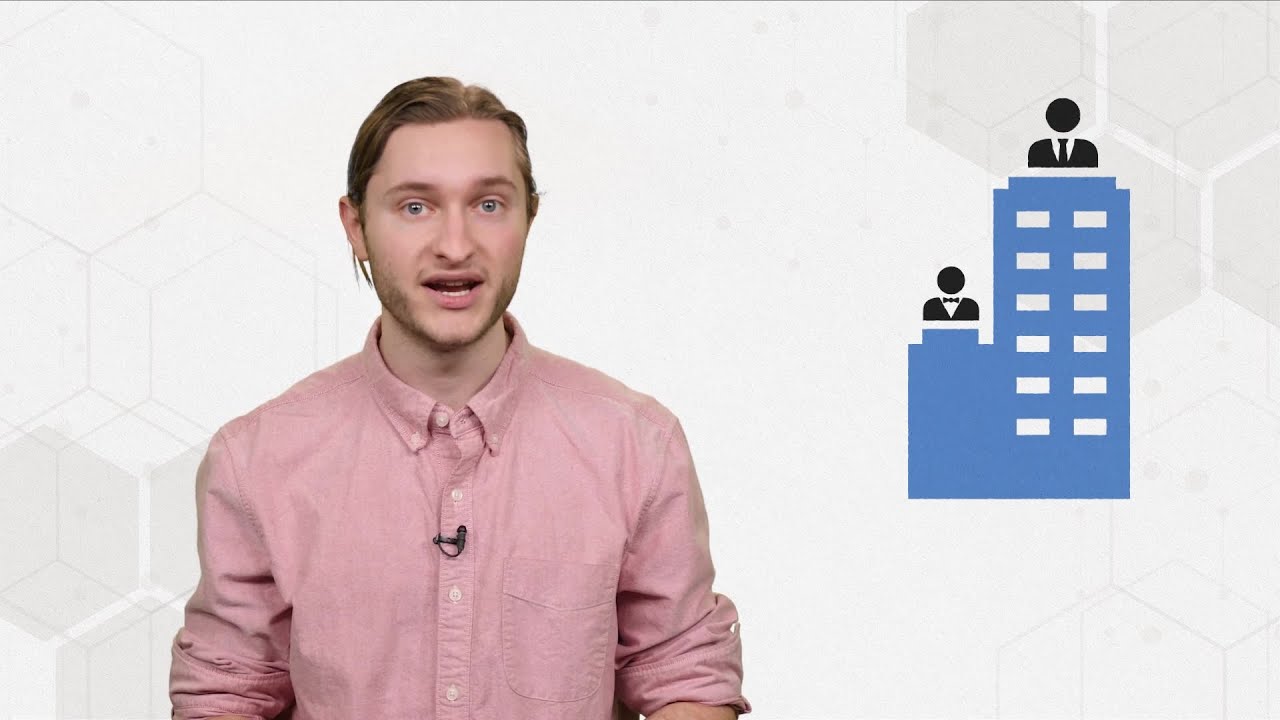
What is Business Intelligence (BI) and Why is it Important? Updated for 2024
5.0 / 5 (0 votes)