Snowflake Summit 2023 Keynote: Generative AI’s Impact on Data Innovation in the Enterprise
Summary
TLDR在Snowflake Summit上,前Greylock合伙人、Conviction AI投资公司的创始人Sarah Guo欢迎了Snowflake和Nvidia的CEO Frank Slootman和Jensen Huang。他们讨论了生成式AI的革命性影响,强调了数据在其中的重要性,并宣布了两家公司合作扩展,旨在为客户提供加速创建定制生成式AI应用的途径。该合作利用Nvidia的AI平台和Snowflake的数据能力,推动企业使用自有数据开发智能应用,预示着企业将转变为AI工厂,不断生产智能。
Takeaways
- 🌟 Snowflake和Nvidia宣布扩大合作伙伴关系,允许客户在Snowflake平台上利用Nvidia AI合作平台开发自定义生成AI模型。
- 🤖 强调生成AI(Generative AI)的重要性,它正在改变世界,需要数据来实现其功能。
- 🔍 讨论了AI如何帮助企业,特别是通过自然语言处理和增强查询来简化与数据的交互。
- 🛠️ 强调了Nvidia在计算革命中的作用,特别是在加速计算和AI应用开发方面。
- 💡 讨论了AI如何帮助企业解决复杂问题,例如消费者流失、供应链管理和定价优化。
- 📈 强调了AI在提高企业效率和降低成本方面的潜力,特别是在处理大型数据集和复杂计算任务时。
- 🛑 讨论了AI应用开发中的挑战,包括数据安全、合规性和版权问题。
- 🚀 提到了AI如何加速产品开发,例如Nvidia使用AI来设计下一代GPU。
- 💼 讨论了企业如何通过AI改变其业务模型,特别是在金融服务、零售和制造业中。
- 🔑 强调了数据作为企业最宝贵的资产,以及如何通过AI将其转化为智能和洞察力。
Q & A
什么是生成性人工智能(Generative AI)?
-生成性人工智能是一种能够创建新内容而不仅仅是识别或标记数据的人工智能。它能够生成文本、音乐、图像等内容,代表了人工智能领域的一个重大进步。
为什么数据对于生成性AI至关重要?
-数据是生成性AI的基石,因为AI模型需要大量的数据来训练和学习,以便生成准确和有用的输出。没有数据,生成性AI就无法理解和创造与人类需求相关的新内容。
Snowflake和Nvidia宣布了什么样的合作?
-Snowflake和Nvidia宣布了一个大规模的合作扩展,该合作使客户能够在Snowflake上利用Nvidia AI平台开发定制的生成性AI模型,并使用他们自己的专有数据。
Nvidia在AI领域扮演了什么角色?
-Nvidia是AI领域的关键参与者,提供了强大的计算平台和加速技术,使得AI模型能够更快速、更高效地进行训练和推理。
Snowflake作为数据平台,它的核心优势是什么?
-Snowflake是一个云数据平台,它提供了一个安全、可扩展且易于使用的环境来存储、处理和分析大量数据。Snowflake的优势在于其能够处理各种规模的数据,并支持实时分析。
为什么Snowflake和Nvidia的合作对于企业来说很重要?
-Snowflake和Nvidia的合作为企业提供了一个集成的解决方案,使他们能够在自己的专有数据上快速开发和部署定制的生成性AI应用,这有助于企业提高效率、降低成本并创造新的商业机会。
什么是软件1.0、软件2.0和软件3.0?
-软件1.0指的是传统的、由工程师一行一行编写代码的软件。软件2.0涉及到通过大量标记好的训练数据来优化神经网络。而软件3.0则是与基础模型一起工作,这些模型本身就非常强大,但仍然需要与企业数据和自定义数据集一起工作。
为什么说Snowflake是存储和处理数据的最安全、最好的地方?
-Snowflake提供了一个高度安全的环境,具有强大的数据治理和合规性功能。此外,它的架构设计允许高效的数据处理和分析,使其成为企业存储和处理数据的理想选择。
Nvidia如何帮助企业加速其AI应用的开发?
-Nvidia通过提供预训练的基础模型和专业知识,帮助企业在Snowflake平台上快速构建和部署AI应用。这些预训练模型已经在Nvidia的AI工厂中进行了优化,可以显著减少企业开发AI应用的时间和成本。
企业如何从AI中获得最大的价值?
-企业可以通过利用AI来优化运营、改善客户体验、开发新产品和服务以及提高决策效率来获得最大的价值。关键是要找到正确的数据集,并与像Snowflake和Nvidia这样的合作伙伴合作,以确保AI应用的成功部署。
Outlines
🌟 雪湖峰会开幕与生成式AI的前景
Sarah Guo,前Greylock合伙人及Conviction创始人兼CEO,在雪湖峰会上致开幕词。她强调了生成式AI在过去一年中的快速发展,并介绍了Frank Slootman和Jensen Huang,分别作为Snowflake和Nvidia的CEO,讨论了数据和生成式AI如何为企业带来变革。两位CEO讨论了他们对AI的看法,Jensen Huang认为这是前所未有的技术革命,而Frank Slootman则强调了与数据打交道的重要性。他们还宣布了Snowflake和Nvidia之间新的合作伙伴关系,旨在帮助企业利用自己的专有数据创建定制的生成式AI应用程序。
🤝 Snowflake与Nvidia的合作及其对企业的意义
Snowflake和Nvidia宣布扩大合作伙伴关系,允许客户在Snowflake平台上使用Nvidia AI合作平台开发自定义的生成式AI模型。Frank Slootman提到,Snowflake与Nvidia的合作是天作之合,双方在提供计算能力、服务和数据方面有着天然的一致性。Jensen Huang强调了将计算引擎带到数据所在的地方的重要性,并解释了如何通过结合数据、AI算法和计算引擎来帮助客户使用他们的专有数据开发AI应用程序。
🛠️ 利用生成式AI模型和企业数据
Jensen Huang和Frank Slootman讨论了如何将预训练的基础模型与企业数据结合使用。Jensen Huang提到,预训练模型需要根据特定行业进行微调和专业化,以适应企业的具体需求。他们讨论了如何通过监督学习和增强学习来训练模型,并强调了在Snowflake平台上创建AI应用的目标,即帮助企业解决特定问题。此外,他们还讨论了如何通过使用大型语言模型将任何数据库转换为应用程序。
💡 企业如何从AI中快速获得价值
讨论集中在企业如何快速从AI中获得价值。Frank Slootman和Jensen Huang认为,最直接的应用将是增强查询,因为这是一种相对容易实现的方式,可以让更多人以更自然的方式与数据互动。他们还提到,一旦解决了这些初步问题,企业将开始关注更复杂的问题,如消费者流失、供应链管理和定价优化等,这些问题需要更深入地挖掘企业数据。
🚀 Snowflake和Nvidia如何革新数据处理
Jensen Huang强调了Snowflake和Nvidia合作的目标,即通过加速计算来革新数据处理。他们计划将Nvidia的GPU计算能力整合到Snowflake中,以提高数据处理、训练、推理和部署的效率。这种整合将使企业能够在Snowflake中快速开发AI应用,而不需要传统的编程技能。
💼 企业如何利用AI改变业务模式
讨论转向企业如何利用AI改变业务模式。Frank Slootman提到,企业将能够通过AI更深入地了解数据,从而改变利润结构并扩大对客户的服务范围。Jensen Huang分享了Nvidia如何在自己的数据上应用AI来解决复杂的工程问题,例如GPU设计和软件编译优化。
🏆 为什么选择Snowflake和Nvidia进行AI和ML工作负载
最后,讨论了为什么企业应该选择Snowflake和Nvidia来处理他们的AI和机器学习工作负载。Jensen Huang和Frank Slootman强调了他们提供的服务的独特价值,包括安全的数据存储、处理和AI应用开发。他们承诺将帮助企业将数据转化为智能,创造出前所未有的价值。
Mindmap
Keywords
💡生成式AI
💡数据
💡Nvidia
💡Snowflake
💡合作伙伴关系
💡大语言模型
💡人工智能应用
💡定制模型
💡查询引擎
💡数据治理
Highlights
生成性人工智能(AI)在过去一年席卷全球,成为人们关注的焦点。
生成性AI需要数据的支持,Snowflake和Nvidia的CEO讨论了数据与生成性AI的结合如何为企业带来变革。
Snowflake和Nvidia宣布扩大合作伙伴关系,共同提供加速创建定制生成性AI应用程序的途径。
Nvidia的Jensen Huang认为,当前的计算革命是前所未有的,AI能够自我编写软件,对行业产生深远影响。
Snowflake的Frank Slootman强调了与数据的自然和富有成效的关系的重要性,以及AI如何帮助实现这一点。
通过结合Snowflake的数据和Nvidia的计算能力,企业可以在自己的专有数据上开发定制的生成性AI模型。
AI技术的发展使得人们可以用自然语言与数据库交互,就像与人交谈一样。
Nvidia和Snowflake的合作将推动企业利用AI优化成本结构,例如通过减少呼叫中心的规模。
投资者认为,软件3.0时代的到来意味着企业将能够以更低的成本开发AI应用程序。
定制的AI模型与通用模型相比,可以更专注于特定领域的复杂数据集,从而降低计算成本。
Nvidia将提供预训练的基础模型和专业知识,以帮助企业在Snowflake平台上与企业数据互动。
企业将能够快速在Snowflake上开发AI应用程序,而不需要从头开始构建大型语言模型。
Nvidia和Snowflake的合作将使企业能够在Snowflake上训练、开发模型,并运营他们的AI,实现数据到智能的转化。
Snowflake和Nvidia的合作是关于将世界上最强大的计算引擎带到世界上最有价值的数据旁边。
通过Nvidia的AI基础服务,企业可以在Snowflake上以更经济高效的方式微调和增强预训练模型。
Nvidia和Snowflake的合作将加速AI在企业中的应用,使企业能够快速构建和部署AI应用程序。
Snowflake Summit上,Nvidia和Snowflake展示了他们的合作成果,包括Nvidia AI Enterprise在Snowflake上的运行。
Nvidia和Snowflake的合作将帮助企业将数据转化为智能,这是最有价值的商品,为企业带来前所未有的变革。
Transcripts
please welcome former Greylock General
partner and founder and CEO of
conviction Sarah Gore
hello Las Vegas
I have the pleasure to officially kick
off snowflake Summit I'm Sarah Gua
former Greylock General partner and
founder of conviction a venture
investing firm purpose-built for
partnering with AI entrepreneurs from
idea to IPO I also host no priors a Tech
podcast focused on the AI opportunity
ahead of all of us
generative AI has taken the World by
storm over the past year and is likely
top of mind for everyone in this room
but what do you need to enable
generative AI That's Right data
there are no two people better on the
entire planet to talk about what
generative Ai and data can do for you
than Frank slitman and Jensen Huang the
legendary CEOs of snowflake and Nvidia
we'll also learn more about their new
partnership to provide businesses with
an accelerated path to creating custom
generative AI applications built with
their own proprietary data please
welcome to the stage Jensen Huang and
Frank slootman sir
[Applause]
[Applause]
[Music]
here come here Frank hugs hugs all
around yeah
he never once get left out
okay
um we will start with a question about
what you two are seeing
um the hype and excitement around AI is
extraordinary this past year uh is it
real is it oriented Jensen let's start
with you
well this is the biggest Computing
Revolution we have ever seen
um no one has ever seen a technology
like this
a computer that writes software
itself
and now the computer can write software
for other computers by itself
when you take a step back and think
about the implications it's really
really profound
and and all of our experiences every
single one of ours I'm sure
has proven that this is the easiest
application to use in the history of
computing because it's so smart
it understands what you mean even when
you say what you mean poorly
and it does things that you tell it to
do that exceeds your expectations
and you can connect it to almost
anything it's a universal translator
and so when you when you take a step
back and think about its implications
I think we can all we can all uh be
fairly excited about the all of us in
the computer industry and what we've
done
Frank is It Something That Matters to
Enterprises and and should they be
paying attention
well yes
um you know we we have a long strenuous
uh relationship with data you know we're
old enough actually Jensen and I might
be you will not be you know where I was
learning COBOL and COBOL stood for
common business oriented language and
there was nothing common or business
oriented about it
um you know in the 80s you know we had
SQL structured data that helped a lot
because data now kind of made sense rows
and columns
um but still it's it's it's been hard to
have a relationship with data that's
natural and productive and insightful
all these things and all of a sudden you
know we have this huge step function
from where we've been all this time and
it's like it's just an absolute wow
which is why we're all so infatuated
with the experiences that we're seeing
it's a perfect segue to um news hot off
the presses in the last two minutes
Nvidia and snowflake just announced a
massive expansion to their partnership
where you know customers can Leverage
The Nvidia AI partnership platform on
snowflake for developing custom
generative AI models on their own
proprietary data can you tell us more
about this partnership
yeah you know you know snowflake
disorder things that we do there's a lot
of people trying to kill us so uh
finding partners that have natural
alignment with us and have extraordinary
capabilities
um that doesn't come along you know
every day and uh you know obviously
Nvidia is an extraordinary place you
know in time and in history in terms of
the role they play in the entire history
you know for us you know to be able to
bring data and relationships with large
Enterprises everybody that you see here
in the room if we combine that you know
with the Computing fabric that that we
need to enable this technology as well
as the entire stack of services to use
it productively yeah that's you know I
don't want to use the say it's a match
made in heaven but it's about as close
as you as you're going to get we have
full alignment there's no conflict and
uh that's that's that's a heck of an
opportunity for for all of us in the
room here so we're lovers not Fighters
so
I woke up this morning without a voice I
have no idea where it went I'm going to
find it here somewhere and so but the
the big deal here Sarah is that we're
we're going to bring the world's best
compute engine
to the world's most valuable data you
know back in the old days when we first
started our career and and uh Frank
Frank mentioned how long he's been
working I've been doing my job a long
time I'm not that old you're old
they think that's funny well they know
it's true and and
so
so back in the old days we used to bring
data to the computer
and the reason for that is because
there's so little data to move but these
days for all the reasons that we know
well the data is gigantic the data is
valuable it has to be secure
it's regulated
governance has to be incredible and so
it's tough to move data around the data
gravity is real and so it's a lot easier
for us to bring our compute engine to
Snowflake and our partnership is about
about accelerating snowflake but it's
also about bringing AI to snowflake you
know that at the core
the big revolution is about the
combination of data plus AI algorithms
plus compute engine
our combination our collaboration here
our partnership brings all of those
three things together
incredibly valuable data incredibly
great AI incredibly great compute engine
and the thing that we could do together
is to help customers use their
proprietary data and write AI
applications with it you know the big
breakthrough here and and this is I'm
sure you guys are going to learn a lot
about this during this week is that for
the very first time
you could develop a large language model
you stick it in front of your data and
you talk to your data
you talk to your data like you talk to a
person
and that data will be augmented with the
would augment the large language model
and you'll ask it all kinds of questions
about what and how and when and why all
of the things that you might query the
database in the future you'll talk to
the database in the future the
combination of a large language model
plus knowledge base equals an AI
application
it's as simple as that
a large language model turns any data
knowledge base into an application and
just think about all of the amazing
applications that people have written
it's always at the core of it some
valuable data now you have a query
engine Universal query engine in front
of it that's super intelligent and you
can get it to of course respond to you
but you can also connect it to an agent
as you know and this is uh the
Breakthrough of of Lang chain plus
Vector databases Plus data
from large language models
groundbreaking stuff is happening
everywhere now everybody's going to do
it and Frank and I are going to help
everybody do that yeah we we've seen
um you know in our investing a huge
explosion of chat Bots search
summarization and Frank you and I were
talking a few weeks ago about how much
the interface to business data can
change and what a big opportunity that
is for customers is that um something
you expect to show up in the snowflake
product or or P or applications that
people build themselves
well we we expect to be you know
visiting with our customers at some
point you know relatively soon you know
I would say I said look you know we're
we're going to push a boundary in terms
of what kind of questions you know
you'll be able to ask of your data I
mean just just connecting natural
language I think it's amazing and I I
love it right because many more people
can be productive and all this kind of
stuff but asking really hard questions
of your business is a whole you know
different matter and I I hope you know
we're all right here that the
intelligence is in the data and the
models are able to extract the the
reasoning and intelligence from that
data and that will lead to those you
know incredibly insightful predictive
relationships with data but if not you
know we're going to sort of unpack and
break it down and understand it so we
can keep pushing those boundaries people
ask very very hard questions of their
data sometimes they have to you know
launch whole teams for weeks on end to
come back with answers that we even have
difficult be trusting there's no Frank
it's like this this is I mean there's
there are tons of customers in the
audience who have large customer support
organizations yeah and all that data is
going into snowflake
could you imagine if you had hundreds of
your customer support agents sitting in
front of you and you just asked them a
whole bunch of questions
that's the future you're going to put it
you're going to put a large language
model in front of that customer service
database and you're going to talk to it
yeah you're going to ask you know what
are the most frequent things that are
happening to our customers that we could
improve on what are the things that they
seem to find really delightful about
products you could ask those questions
in normal human language and it will
talk back to you that's the miracle
you're going to turn customer
service data into a customer service
application
that's the that's the incredible
opportunity a lot of the uh you know the
originals of large telcos and Banks I
mean they're looking to redefine the
economics of their businesses which is
really something they have not been able
to do and they're thinking you know that
that opportunity is coming large call
centers that's the examples you you just
used right sales organizations that feel
incredibly unwieldy and expensive you
know to them
um so redefining cost structures
um I mean people get heady just thinking
about the uh the possibility and
opportunity yeah we're we're going to be
part of this of this journey and I it's
a good time to be alive in this business
you know one of the ways that we um you
know as investors think about this
transition is going from software uh 1.0
which is uh you know very deterministic
code written function by function by
Engineers to software 2.0 which is you
know you're optimizing a neural network
with um you know carefully collected
labeled training data and I think the
opportunity that you guys are really
helping people Leverage is what I think
of as software 3.0 which is we are
working with this set of foundation
models that are incredibly capable on
their own but they still need to work
with Enterprise data and custom data
sets it's just much cheaper to go
develop those applications against them
right you can think of them as the
world's best query engine on top of your
most important data so I think that's
like a really attractive opportunity to
how much the cost comes down for
customers so actually this is a this
raises an interesting question for
people who are paying deep attention to
this area
um you know one question is foundation
models are very general uh
can they just do everything like why do
we need custom models and Enterprise
data anyway
well I mean there's there's a school of
thought in there it's like maybe but it
will be incredibly expensive right so we
we have you know very broad General
models that can do poetry and process
the Great Gatsby and summarize it and
all these and math problems broken down
how I wish I had that as a kid right but
you know in business we don't need that
right we're looking for a co-pilot that
has
extraordinary Insight in a very narrow
set of data but a very complex Sarah
data we need to understand business
models and business Dynamics now that's
that is computationally not as expensive
because you just don't need to be
trained on a million things you need to
be trained on you know very few and very
very deep subject matter so if we think
of these models as
the general knowledge of the
from these AI applications that is not
in the general knowledge of the internet
yeah well I'll give you an example
um you know one of my uh I'm on the
board of instacart and you know one of
the topics a great customer of ours as
well and you know one of the topics that
comes up in businesses like doordash and
all the others is you know they drive
Top Line with huge amount of marketing
expense and it's very very hard to do
and then they onboard a customer and
then the customer places an order and
then the customer either doesn't come
back or he comes back 90 days later it's
very erratic and they call that churn
churn is the most you know most
incredibly complex problem to analyze
because you may not come back for one
reason I may not come back for another
reason right so people want to find
answers to these questions it is in the
data it is right but but try to reason
your way you know to that right that's
that that's an example that is worth an
enormous amount of money you know right
and the answer to you know why is this
instacart customer churning is not in
the general knowledge of the internet no
somewhere in the instacart data we hope
yes it is and we we see other software
companies and within in product
marketing and so on they try to you know
understand behaviors to the point where
they can then because if you understand
the problem you can do something about
it and be productive in changing the
turn ratios if you're wrong everything
you're doing is is ineffective and that
that is literally what's going on you
know
Jensen part of the partnership is you
guys bringing your pre-trained
foundation models and expertise
to uh you know the snowflake platform
right so how do you think about how
those models should interact with
Enterprise data
uh first of all I've never met
um an employee that was too smart
you know just it embarrassed me how
smart they were
I've never hired a new college grad I
just got too smart too smart
so we can make the pre-trained models as
smart as we can and then we still have
to onboard them we still have to
fine-tune them we still have to you know
we have to still specialize them into
our particular craft and so our strategy
and our offering is state-of-the-art
pre-trained models of a variety of sizes
and sometimes you need to create a very
large pre-trained model so that it can
generate prompts so that you could teach
the smaller models which generates
prompts to teach even smaller Pro Models
and the smaller models could run almost
almost anything and maybe the latency is
really really low however it doesn't
generalize as well it's you know zero
shot capability is probably a lot more
limited and so you might have several
different types of different different
sizes of models but in every single case
you have to do supervised fine-tuning
you have to do reinforcement learning
human feedback so that you could keep it
and keep it aligned to your your um are
your goals and principle and you need to
guard rail it you need to augment it
with Vector databases and things like
that and and so all of that comes
together in a platform and we have the
skills and the knowledge and the basic
platform to help them create their own
AI
and um and then connected to the
incredible data that Frank has in uh
snowflake now ultimately what every
Enterprise customer will do is not
ultimate their goal shouldn't be to
think about how do I build a large
language model their goal should be how
do I build an AI application that solves
this particular problem and it might
that application might require 17
questions in previous prompts that it
finally came to the right answer and
then you might say now I would like to
take these I would like you to to to
write me a program and make it could be
a SQL program it could be a Python
program so that I could do this
automatically in the future and so you
still have to guide this AI through as
you know so that it could ultimately
give you the right answer but then after
that you can create an application that
could run as an agent 24 7. Looking for
circumstances like this and revealing it
to you in advance and so our job is to
help customers build these AI
applications that are guard railed and
specific and customized to them and then
lastly we're all going to be
intelligence manufacturers
in the future we'll hire or hire
employees of course and um but we're
going to create a whole bunch of agents
and these agents could be created with
Lang chain and or something like that
that
connects models and knowledge bases and
other apis and you deploy in the cloud
and connected to all the snowflake data
and and you'll operate these AIS operate
these AIS at scale and you'll
continuously refine these AIS and so
every one of us are going to be
manufacturing AIS and we're going to be
running AI factories
we're going to put that infrastructure
in Snowflake and so customers could work
with their data there
train and develop their models there and
then operate their AIS there and so
snowflake will will be your data
repository and your bank
and Frank is sitting on a gold mine of
data as you guys know your gold mine of
data and all and and snowflake is going
to help you turn that gold mine of data
into incredible intelligence and all of
you will be running AI factories all of
it in Snowflake that's the goal
one of
yeah
there's a lot of gold mines out there
I I actually saw the the Nvidia Nemo
stack you know running inside a
snowflake on the park near pavilion
floor at three o'clock this afternoon so
this is not just Pie in the Sky I mean
this is literally happening as we sit
here so that's what's so exciting I mean
the deployability of these Services is
quite High yeah
one of the things that res
ident is talking to some of the
technical
working on this and I said you know
where did the idea come from
came from these two leaders but also
came from seeing you know large
snowflake customers struggled to get
data out to GPU Computing to do machine
learning and then get it back into
Snowflake and you know seeing that you
have the most sophisticated customers
already going down that path and just
making it possible to consolidate in one
place with one stack that's obvious to
operate on seems like a huge win
you should be able to run on Nvidia
everywhere and anywhere you know like I
said we're lovers and so so if
if you if you need if you need Nvidia
GPU Computing we'll come find you
and all of you are in snowflakes so we
found you
but I do have one important question on
this
um if we pay attention to these
companies that are you know training
this generation of llms it is very
expensive to do so on huge numbers of
gpus is you know what should customers
expect about
um you know GPU Computing spend
it's not free uh and and uh that's the
wrong answer yeah I I know and by the
way everybody in this room uh is is
running consumption models uh it's very
different from a capacity model
um you have to really Master the model
you have to have levels of discipline
around it it's hard to come to terms
with so you know when you when you're
running workloads queries or AI
factories whatever you want to call it
there's a cost so in other words it's
not just fun and games you can ask the
question what are we having for dinner
tonight but is that a question worth
asking just because a generation
interesting answer right so there
there's the consumption model is already
you know an enormous evolution in in the
world of software this is going to you
know take that to another level I mean
Nvidia are wonderful products but
they're not free last time I checked you
know
I think Frank better let me let me
answer this one
sympathetic CEO so so for
for first of all he's announcing they're
all free actually first of all first of
all there's a coupon coming
first of all
as
as you know
um we we built we we have five AI
Factories at Nvidia and uh four of them
are in the world's uh top 500
supercomputers and another one is coming
online as we speak we use those super
computers to do pre-trained models and
so when you use our Nemo AI Foundation
service inside snowflake the first thing
you're going to get
is a state-of-the-art pre-trained model
that tens of millions of dollars of
expense has already gone into it not to
mention all the r d that went into it
and so it's pre-trained and it's like a
new college grad pre-trained super smart
and and not so not so smart that you're
scared not so smart well not so smart as
me but not I'm just
smart super smart
and so so if they're pre-trained and
then there's a whole bunch of other
models around it and those models for
fine-tuning for uh you know human human
feedback reinforcement learning human
feedback for augmentation all of those
models are a lot more cost effective
to train and so now you've adapted that
pre-trained model to your functionality
to your guard rails to be able to
optimize for the type of skills or
functionality you would like it to have
and and augment it with your data and so
that is going to be a lot more cost
effective and the the important thing is
here what I'm what I hope what I hope
and I believe this this will happen is
that you'll be you'll be able to develop
AI applications connected to your data
at snowflake in a matter of days not
months
you should be able to build AI
applications quickly in the future and
the reason for that is because we're
seeing it happening in real time right
now as we speak isn't that right
communities are
um you know almost enthusiasts and
developers are creating all kinds of
applications with Lang chain and uh
Pinecone and uh and whatever data that
they have uh chat chat PDF is pretty
pretty terrific you load a bunch of PDFs
into it you talk to your PDF and uh
instead of reading a research paper this
morning I was asking this chat PDF about
this particular piece of research which
is a lot more interesting having a
conversation about it than having to
read the whole thing and it happens to
be like 49 pages and so being able to
ask a few questions about it is really a
lot more productive you're going to be
able to do this almost everywhere yeah I
think one of the things that's
encouraging if you just think about
software 2.0 versus software 3.0 is one
way to think about the foundation models
is
you know 95 of the training costs has
been born already by somebody else
that's right right you know as early
stage investors did you hear that
thank you thank you Jensen another
incredible Frank Jensen yeah incredible
it's 95 95 off yeah
can't imagine a better deal slightly
different than what I said but
but I think it's I think it's a real
Dynamic right because you know we're
investors in companies like seek in the
analytics automation space Harvey in the
legal space where uh you know these are
very young companies but they're
applications that have gone to real
business value in six months or less
yeah and part of it is because they're
starting with these pre-trained models
and I imagine that's a massive
opportunity for the Enterprise yeah
every single company will have hundreds
if not thousands of AI applications and
you're just connected to all kinds of
data in your company yeah so all of us
have to get good at building these
things
one of the questions I've been hearing
from you know large Enterprise
practitioners is
we have to go invest in this AI thing do
we need a new stack like how should we
think about this in relation to our
existing data stack how do you how do
you think about that you know I think
the uh it's it's evolving actually
something that we're going to talk about
you know tomorrow is to sort of you know
show all the models that are becoming
possible some are already possible and
already being used are in production and
then you know they're getting
progressively you know tighter more
secure more governed
um and and and and all of that so you
know we don't have a real clear view of
this is the reference architecture that
everybody's going to use you know some
people are going to have some set Of
Central Services you know Microsoft has
its you know open AI you know version
running in Azure and a lot of their
customers uh you know are interacting
it's Azure to Azure it's not exactly you
know uh you know where where we want it
to be but we're not clear on what model
is going to be sort of the dominance you
know form that this is going to take
which is why we will be traversing many
paths because I think the market is
going to sort itself on what is the
trade-off between how hard is it and and
and how easy is it to use and what does
it cost and all these kinds of things so
we're we're speculating a little bit we
see people rigging things up like that
right now and asking cute little
questions why is my portfolio down today
you know all this kind of stuff that
that's all fine right but that's not
that's the beginning that's not going to
be the end State you know in terms of
the sort of production Deployable
applications because the whole the whole
security side of the house is going to
weigh in and and Enterprises like are we
violating any copyrights here when we
use this this kind of all these
questions
um you know we're very enthralled right
now with the technology and and and for
good reason but there's going to be a
level of reality that's going to start
to settle in as well you know well Sarah
you're at the core of your question and
and I agree with everything that Frank
said
um but you know that that we're living
through right now the First Fundamental
Computing platform change in 60 years
if you just read the press release of
the IBM system 360 you will just you
will hear about central processing units
i o subsystems dma controllers virtual
memory multitasking it's scalable
Computing forward and backwards
compatibility you're going to hear about
all those literally written in 1964 and
those words have carried us with CPU
scaling right for
60 years but that has run its course we
can't scale CPUs anymore and that is now
well understood at exactly the same time
that all of a sudden software is
different the way that software is
written the way that software is
operated and what software can do is
profoundly different than it was before
isn't that right you call it software
2.0 and now we're talking about software
3.0 the fact of the matter is Computing
has fundamentally changed and so we're
seeing two fundamental Dynamics
happening at the same time that's the
reason why things are shaken up so hard
on the one hand you can't just keep on
buying CPUs you know that and the reason
for that is because if you bought a
whole bunch more CPUs next year your
Computing throughput would be no more
than if you didn't touch it at all
because it's the end of CPU scaling you
would spend a whole bunch more money you
wouldn't get a lot more throughput and
so the answer is you have to go
accelerate it the touring Award winners
spoke about acceleration Nvidia has
pioneered acceleration and and so
accelerated Computing is now here the
second part of it of course is the whole
operating system on top of that computer
is profoundly different we have a layer
called Nvidia AI Enterprise Nvidia AI
Enterprise data processing training
inference deployment that entire
end-to-end is now integrated into or in
the path of being integrated into
snowflake so that the compute engine
underneath from the beginning of data
processing all the way to the large
language models are now going to be
completely accelerating we're going to
turbocharge The Living Daylights out of
snowflake that's our that's our mission
and and you'll be able to do more and
you'll be able to do more with less and
so so the the um and it's it's it's uh
very very evident if you go to any of
the clouds you will see that Nvidia gpus
are the most expensive compute instances
but if you put a workload on it you will
find
that we do it so fast it's as if you've
got a 90 discount you said 90 earlier is
in fact ten to one and so what's what's
interesting is that we're the most
expensive instance but we're the most
cost effective TCO
so if your job is to run workloads then
the best way to do it is acceleration an
example workload is training large
language models an example workload is
fine-tuning large language models if you
want to do that
absolutely do not
do not not accelerate it please do
accelerate it so accelerate every
workload you can and that's that's the
reinvention of the whole stack of the
processor is different the operating
system is different the large language
model is different and now the way you
write AI applications is different isn't
that right software 3.0 you don't have
to write it at all
AI applications we're all going to write
applications we're all going to connect
our prompts and our contacts and our few
python commands right we're going to
connect it to a large language model
we're going to connect it to our own
database or the corporate database and
we'll develop our own application
everybody's going to be an application
developer
but the constant
is still your data and you still need to
fine tune it yeah you know I I think you
know what in the early days of stuff
like we really because it's a cloud
computing fabric faster is cheaper and
faster has always been more expensive
and all of a sudden faster is cheaper
right it's an inverted
mentality so there's sometimes people
want to under provision thinking it's
cheaper ends up being more expensive
right so this this counter
counterintuitive the other thing you
mentioned in terms of compute paradigms
I mean the idea of
uh the data the work going to the data
instead of the data going to the work we
are completely retracing ourselves and
you know for
60 years pick a number we've been
pushing the data to the work and it has
led to massive siloing and segmentation
that's going to be really difficult if
you want to have ai factories right
that's right so we we have to bring that
work to the data and you know we're
doing that now together and I think
that's we're doing it the right way you
know so yeah that's right yeah
uh uh maybe just as advice for the
audience about what you're seeing from
your own customers or your predictions
where do you expect that customers will
get the most value from AI most quickly
well most quickly it's not the most
value but the most quickly yeah two
separate tanks I mean most quickly is
that
um we're going to be you're going to see
augmented query all over the place
because that's just relatively easy to
add you don't even have to be literate
to get value out of data now which is
quite an amazing uh thing so the
ultimate democratization of your
relationship and interaction so that's
that's all great right it's like search
on steroids right and chat and stuff you
think that's more people interacting
with the data yeah but but you know it's
it's like you're at these cute little uh
you know questions to your dashboards
you know I mean dashboards they are
relatively static and the data is one of
this but then I can bring these
questions to that data and that's that's
low-hanging fruit and then people are
going to go to that board meeting and
say look at what we've got you know
everybody will be applauding and this is
really exciting
um but then I'll be like phase one and
then then it really starts you know once
we sort of get over to low hanging fruit
we start really focusing on a much
harder type questions I'm talking about
proprietary Enterprise data and
combinational structured unstructured
all of it right how do we mobilize that
what's an example of a hard question in
phase two in whatever industry well I I
mentioned the problem of churning
consumer Industries the the problems
that we have in Supply Chain management
because they they when Supply chains can
be extraordinarily complex and if there
is an event then you know how do we you
know re-configure rejigger a supply
chain they're running these extremely
intense models you have to ask these
questions what do I do now and that does
the event does happen and bioid Supply
chains are comprised of many many
different entities it's not a single
Enterprise right so by the way this is a
problem that has never been solved in
the in the history of computing Supply
Chain management has never been platform
it's pretty much an email you know
spreadsheet you know type of a business
you know with small exceptions here and
there so so it's exciting you know
collapsing these these enormous call
center Investments that we we have
pricing optimizations in the world of
retail right like I said it's a
redefinition of business models that
people are going to see and it's it's
exciting but that's that's the real
potential that I think you know CEOs of
large institutions are after you know
Jensen where do you where do you see the
most impact coming in the next five
years across the Enterprise uh I would
ask myself number one what is my single
most valuable database
and the second thing I would ask myself
is
if I had a super super super smart
person in front of it
and everything goes through that super
intelligence
scary scary smart person what would I
ask that person
and and um uh it is can I guess is it
the customer database customer database
in the case because Frank has a lot of
customers I don't have that many
customers
um but but the asset database if you're
manufacturing supply chain yeah so my
supply chain is super complicated and
and my design database is super
complicated
uh it's impossible for NVIDIA to build
our gpus without AI anymore
because none of our Engineers can go
through the number of iterations and
exploration that AIS could do for us and
so when we were coming up with AI our
first application was ourselves
and and so
um uh you know Hopper couldn't have been
designed without Ai and our next
Generation cannot be designed without AI
none of our optimizing compilers could
be done without AI just optimizing the
graph is so complicated because there
are so many targets uh so many gpus so
many different neural network models uh
so many different constraints that
you're optimizing for no human could
possibly compile for that optimize for
that and so we have ai just run
continuously looking for the best
Optimal Solutions and so so we we apply
our our AIS to our own data we would do
we would do that of course our bugs
database is a perfect place because we
have so much code we've got to go and
figure out where
um how to how to how to as quickly as
possible enable our Engineers we have um
you know we're a full stack company if
you look at the amount of code that goes
through Nvidia AI is some several
hundred packages of software that comes
together so that an application can sit
up here be accelerated by a factor of 50
on top of a GPU and then scale across a
full data center that piece of software
called Nvidia AI Enterprise is insanely
complicated
we need in the future and this is these
are some of the things that we're
working on now is how do we use an AI to
go figure out how to security patch it
how to best how to best maintain it so
that we can keep compatibility so that
we don't have to disturb the entire
upper layer on the in the meantime
support backwards compatibility maybe
support a particular security hole
etc etc and these are questions that AI
is capable of answering for you that's
right exactly we can use a large
language model to go answer those
questions and then find the answer for
us or reveal the exposure to us and then
the engineer will be able to go in and
fix it
yeah or recommend a fix and then we can
be the human in the loop to go
acknowledge whether this is a good fix
or there's a better fix yeah yeah when
we talk to practitioners we hear
um understand our data better across the
organization so that's kind of phase one
that you talked about like how can we
just ask more questions and let more
people ask those questions we hear
change the margin structure of our
business right and then expand the scope
of what we are doing for our customers
um I think that what you're talking
about with Nvidia actually using it
within engineering across problems that
are too complex for humans to handle
today I think that's actually new but it
makes sense to be honest it makes a lot
of sense because because you have to
Traverse across so many layers
no person could see all those layers and
and so wherever we can when the data is
so so valuable so complex and so I just
asked myself what's my most valuable
data
oh you have we have great customers and
you know for example auto loans you know
um every time they they price alone you
know the amount of computation and data
that gets involved in pricing that loan
I mean you're a market of one to them
right and the one basis point here or
there at the scale that they operate you
know becomes real money and it's only
computationally understandable you look
at auto insurance right they use
Telemetry data from the devices that are
in your car to price risk because that's
the data that determines you know
whether you're going to have claims or
not in other words that business can't
be run without Telemetry data anymore
because you know the competition drives
the prices down but how do you need to
drive profits up at the same time so you
need to really really understand the the
cost of the risks you're taking so we
can already not live with data uh in
many many businesses that we're in you
know impossible
I can't let you guys get away without
asking at least like one more
challenging question here so uh you know
we have a lot of portfolio companies
they need uh you know they have ML and
AI workloads there's also a series of
cloud providers out there like why
Snowflake and Nvidia for their ml
workloads
what was the question hang on a second
her mind's I think cocktail you want
this one too no no yours is water I
think mine's not yeah
I was just saying I'm the guest Frank
was I also wanted a cocktail what did
you say what was the last part the
question is like there's a bunch of
cloud providers out there who are vying
for your ml or your AI workload business
like why why Nvidia and snowflake
well we're the best
that's a good answer yes
and and we're gonna we're gonna come to
a theater near you and prove it of
course you know and uh and and prove Us
in the putting you know we we always aim
for outcomes your outcomes not ours
yours right and uh you know that we're
gonna leave it all in the field I mean
this is this is I find it's the most
interesting time to be alive you know in
this business because the ability to aim
for superlative outcomes is is there now
that's for a long time you know um I I
we talk about data warehousing it used
to be begging for 2 30 a.m time slot
three months from now that was the state
of the business and look at where we are
now it's just unbelievable right you
know the types of problems that we're
talking about now so it's it's shipping
an intelligent application in days and
weeks yeah I I think not not everybody
realizes how much intelligence is
embedded in the data that they look at
every day how much there is to be
unlocked you know in insights and and
impact so that's where we all are going
to come in and make that happen yeah
you know Sarah there's a reason
that everybody is in Snowflake
there's a reason for that because this
is the safest best place to store your
data
to process your data and and so for
everybody who's in Snowflake
we are your best answer
and for the very first time if you date
a warehouse we're going to connect the
AI Factory next to it
and you're going to be producing
intelligence the most valuable commodity
the world's ever produced
you are sitting on a gold mine of
Natural Resources your company's data
proprietary data we're now going to
connect it to an AI engine
and you're gonna on the other end of
that is just intelligent spewing out
every single day
unbelievable amounts of Intelligence
coming out the other end even while you
sleep
this is the best thing ever so if you're
if you're a snowflake customer as many
many are
Frank and I are going to help you
turn your data into intelligence
that's a great way to summarize I can't
improve on that
it was a great conversation guys welcome
to snowflake Summit congratulations on
your partnership thank you so much thank
you so much
right here let's do it thank you great
job oh one more thing
oh
Jensen we're not going to let you go
home empty-handed
I mean this is one of the most valuable
wow wow it's snowflake Nvidia Brandon
look at this wow
[Applause]
this is awesome thanks awesome let it go
everybody thank you for your office okay
you can get on it you have to stand on
it actually I don't want to ruin it
what's up
Ver más vídeos relacionados

NVIDIA黃仁勳給年輕人的忠告!你要面對的世界將是這樣子的!不看真的會後悔!
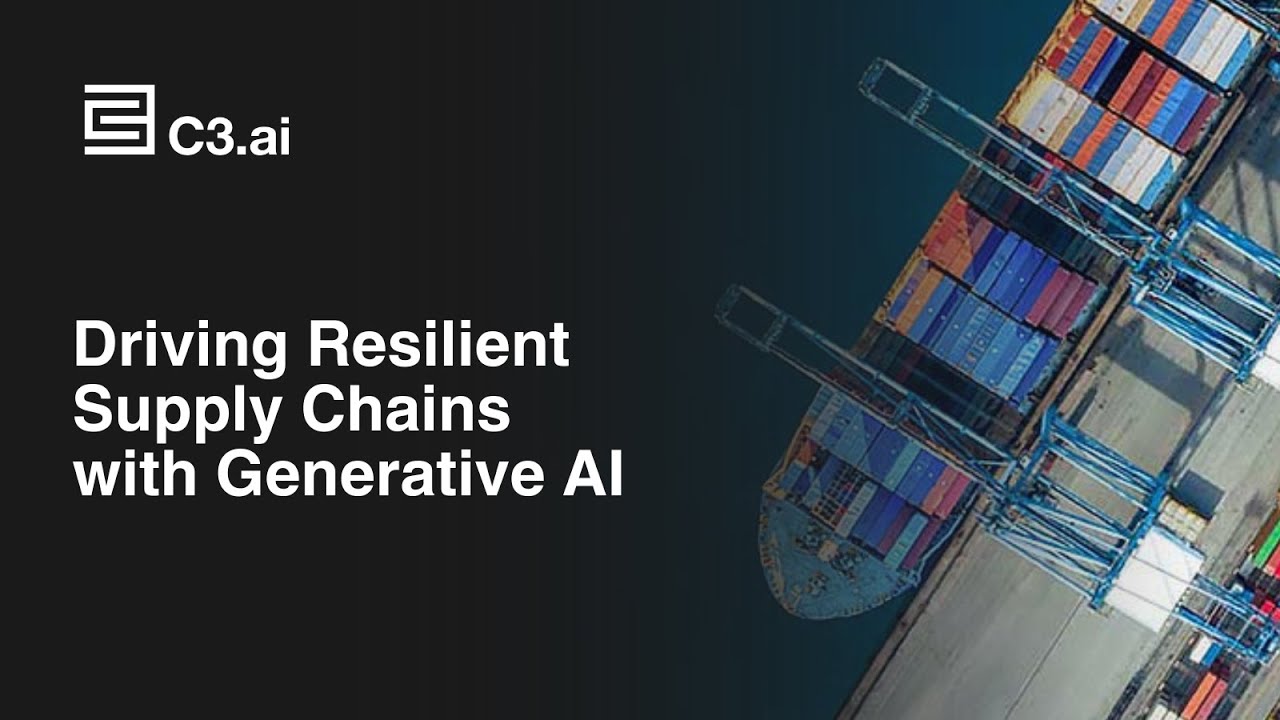
Generative AI - Driving Resilient Supply Chains

"Generación de Resiliencia en la Cadena de Suministro"
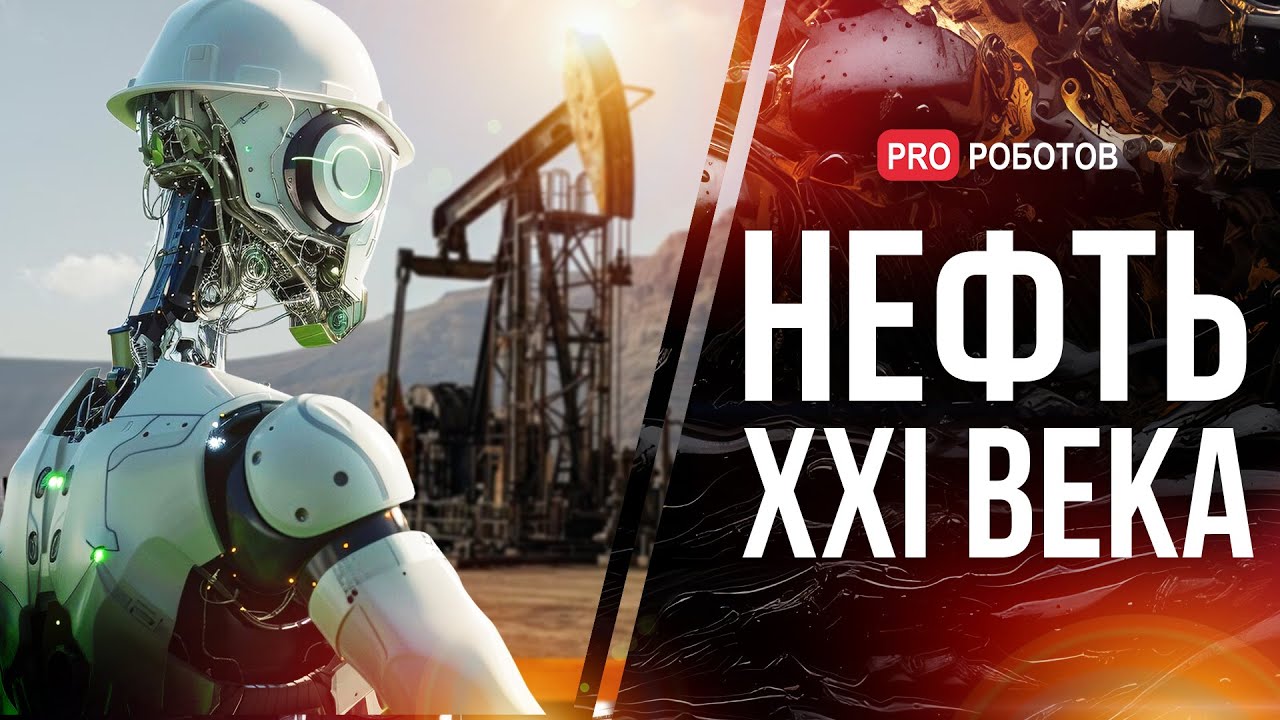
Как ИИ-компании победили Google, Apple и Amazon | Искусственный интеллект: путь к завоеванию мира

"Introducing the AI Robot Billionaire: Pioneering the Future of Wealth and nnovation
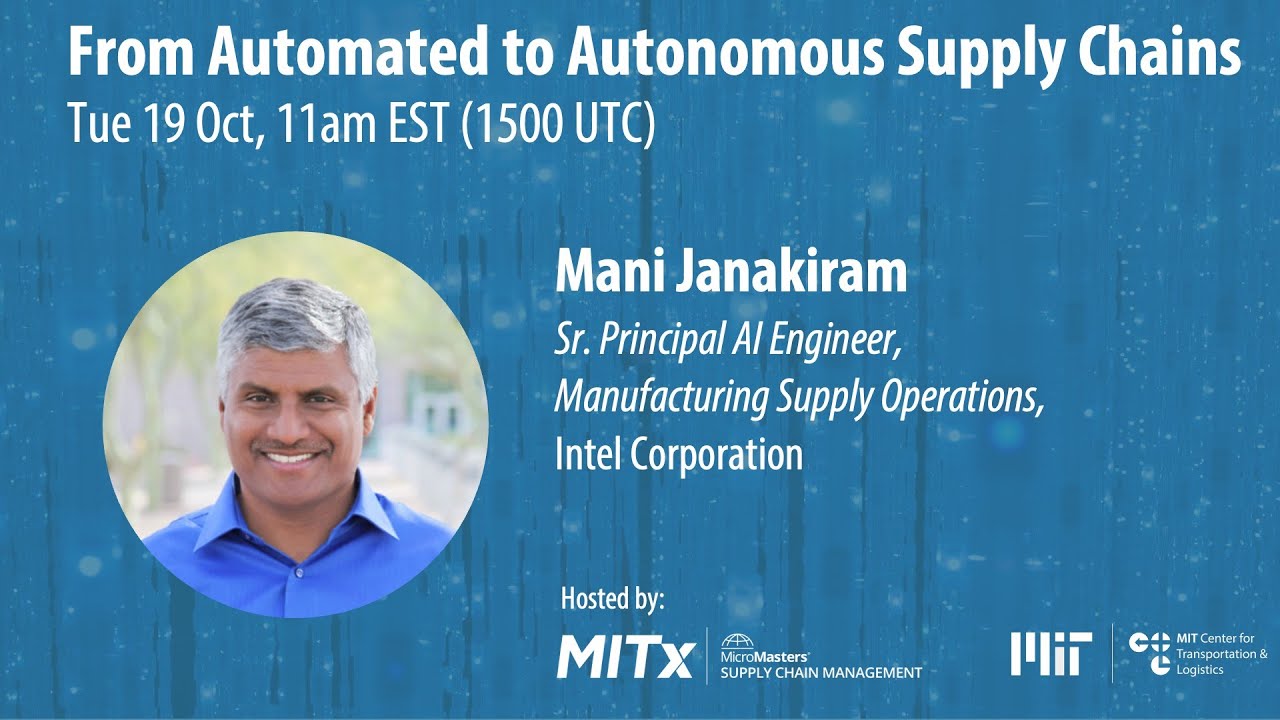
From Automated to Autonomous Supply Chains
5.0 / 5 (0 votes)