NERFs (No, not that kind) - Computerphile
Summary
TLDRThis video explores Neural Radiance Fields (NeRF), a cutting-edge AI technique for generating 3D reconstructions from 2D images. The host, with the help of a PhD student, explains how NeRF uses RGB images to create detailed 3D scenes, contrasting it with traditional methods like point clouds and meshes. They discuss the process of training a neural network to 'see' and render 3D objects, emphasizing the importance of multiple viewpoints for accurate reconstruction. The video also touches on the limitations, such as the need for a large dataset and the challenges of capturing dynamic scenes, while highlighting NeRF's potential in revolutionizing 3D rendering and asset creation.
Takeaways
- 🌐 The script introduces Neural Radiance Fields (NeRF), a technology for generating new views of scenes from a series of RGB images.
- 🎓 Lewis, a PhD student, explains NeRF, which he works with as part of his PhD, to the audience.
- 🌳 NeRF reconstructs 3D scenes with high detail from simple RGB images, unlike traditional 3D reconstruction methods like point clouds or meshes.
- 🖼️ Rendering with NeRF involves shooting rays into the environment and querying a neural network for color and density at various points along the ray.
- 🔍 NeRF's 3D representation allows it to understand the environment, unlike other generative models like diffusion which lack a 3D context.
- 📸 For effective training, NeRF requires a substantial number of images, ideally 250 or more, to achieve a good reconstruction.
- 📹 The script discusses the challenges of capturing a dynamic scene like a Christmas tree with NeRF, including issues like motion blur and overfitting.
- 🚫 Changes in the scene or photobombing can lead to artifacts like 'floaters' where the model struggles to place certain pixels accurately.
- 📈 Despite its limitations, NeRF can be used to quickly generate 3D representations from images, which can then be converted into meshes for use in games or other applications.
- 🔮 The future of 3D rendering may involve technologies like NeRF, but there are also emerging rivals like GAN splatting that show promise.
Q & A
What are Neural Radiance Fields (NeRF)?
-Neural Radiance Fields, or NeRF, is a method used in AI literature for generating new views of scenes from a series of RGB images. It reconstructs 3D scenes using a neural network, unlike traditional 3D reconstruction methods such as point clouds, voxel grids, or meshes.
How does NeRF differ from traditional 3D reconstruction methods?
-Traditional 3D reconstruction methods like point clouds, voxel grids, and meshes are discrete and can become complicated with many points or faces. NeRF, on the other hand, reconstructs scenes in high-quality detail using only RGB images, encoding the 3D scene within the parameters and weights of a neural network.
How does the rendering process in NeRF work?
-In NeRF, rendering involves shooting rays into the environment and querying a series of points along these rays. The neural network then provides color and density for each point, which is different from traditional rendering methods that might use rasterization or ray tracing.
What is the significance of density in the context of NeRF?
-Density in NeRF indicates whether a point along a ray is within an object or in empty space. A density of zero corresponds to empty space, while a density of one or more signifies that the point is inside an object, which is crucial for 3D representation.
Why is capturing multiple images from different angles important for NeRF?
-Capturing multiple images from various angles is essential for NeRF to properly reconstruct a 3D scene. It allows the neural network to understand the environment from different perspectives, which is necessary for accurate rendering when the camera viewpoint changes.
What are the downsides of using NeRF for 3D reconstruction?
-One downside of NeRF is the need for a large number of images for good reconstruction quality. Additionally, the method can struggle with scenes where objects change position or are not fully captured, leading to artifacts or noise in the rendered image.
How many images are typically needed for a good NeRF reconstruction?
-For a good reconstruction, at least 250 images are recommended. However, the quality can vary depending on the technique used and the complexity of the scene.
What is the role of the camera positions in NeRF?
-Camera positions are crucial in NeRF as they provide the spatial context for the images captured. They help the neural network understand where each image was taken from, which is necessary for reconstructing the 3D scene accurately.
Can NeRF be used to create 3D assets for games or other applications?
-Yes, NeRF can be used to extract the 3D volume of objects, which can then be converted into meshes. This can speed up the creation of 3D assets for use in games or other applications, as it requires fewer manual design and modeling steps.
What is the current state of NeRF in terms of real-time rendering?
-NeRF is not suitable for real-time rendering due to its computational intensity. It can take several seconds to render a single image, making it more suitable for pre-rendered scenes rather than real-time applications.
Are there any alternatives to NeRF for 3D reconstruction?
-Yes, there are alternatives such as GAN splatting, which is a newer method also providing impressive results for 3D reconstruction and is worth exploring for potential advantages over NeRF.
Outlines
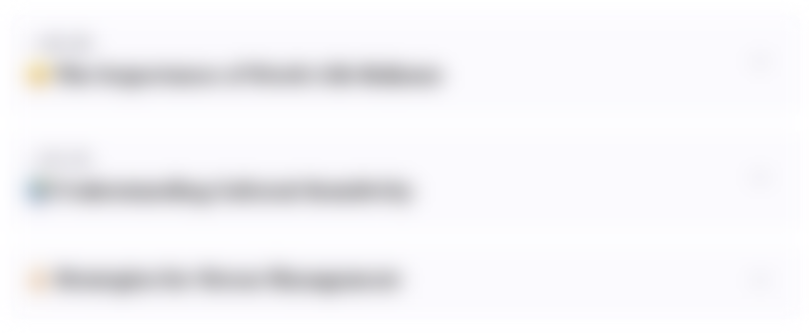
Esta sección está disponible solo para usuarios con suscripción. Por favor, mejora tu plan para acceder a esta parte.
Mejorar ahoraMindmap
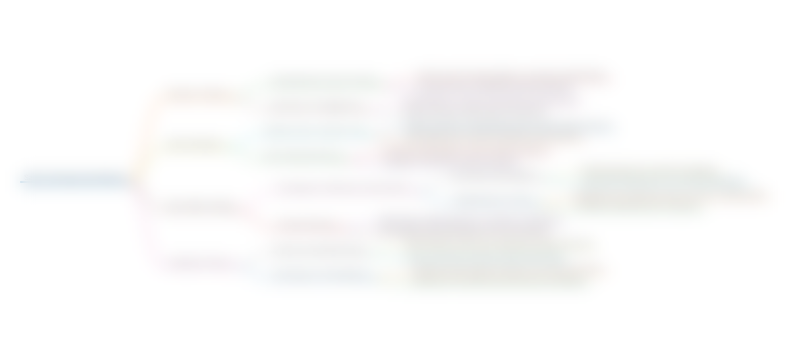
Esta sección está disponible solo para usuarios con suscripción. Por favor, mejora tu plan para acceder a esta parte.
Mejorar ahoraKeywords
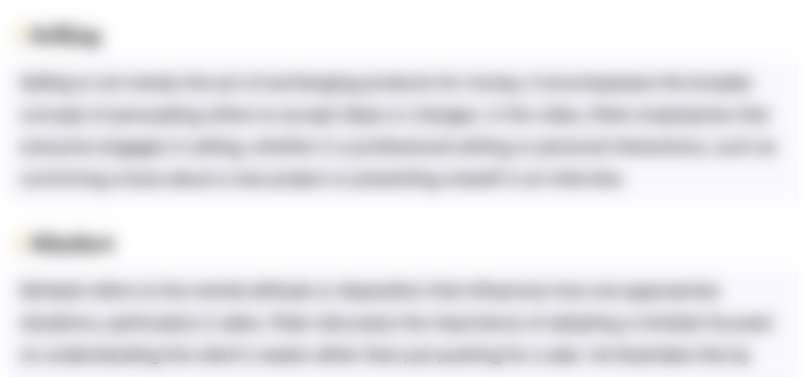
Esta sección está disponible solo para usuarios con suscripción. Por favor, mejora tu plan para acceder a esta parte.
Mejorar ahoraHighlights
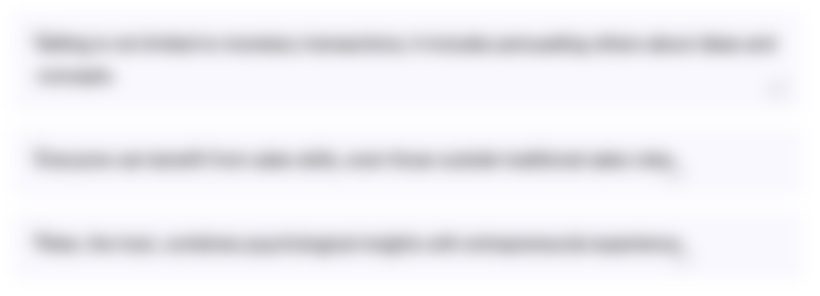
Esta sección está disponible solo para usuarios con suscripción. Por favor, mejora tu plan para acceder a esta parte.
Mejorar ahoraTranscripts
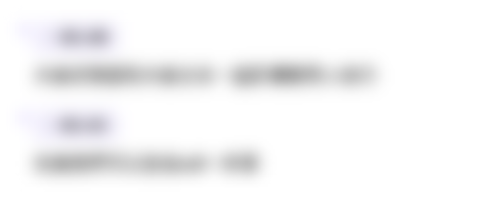
Esta sección está disponible solo para usuarios con suscripción. Por favor, mejora tu plan para acceder a esta parte.
Mejorar ahoraVer Más Videos Relacionados
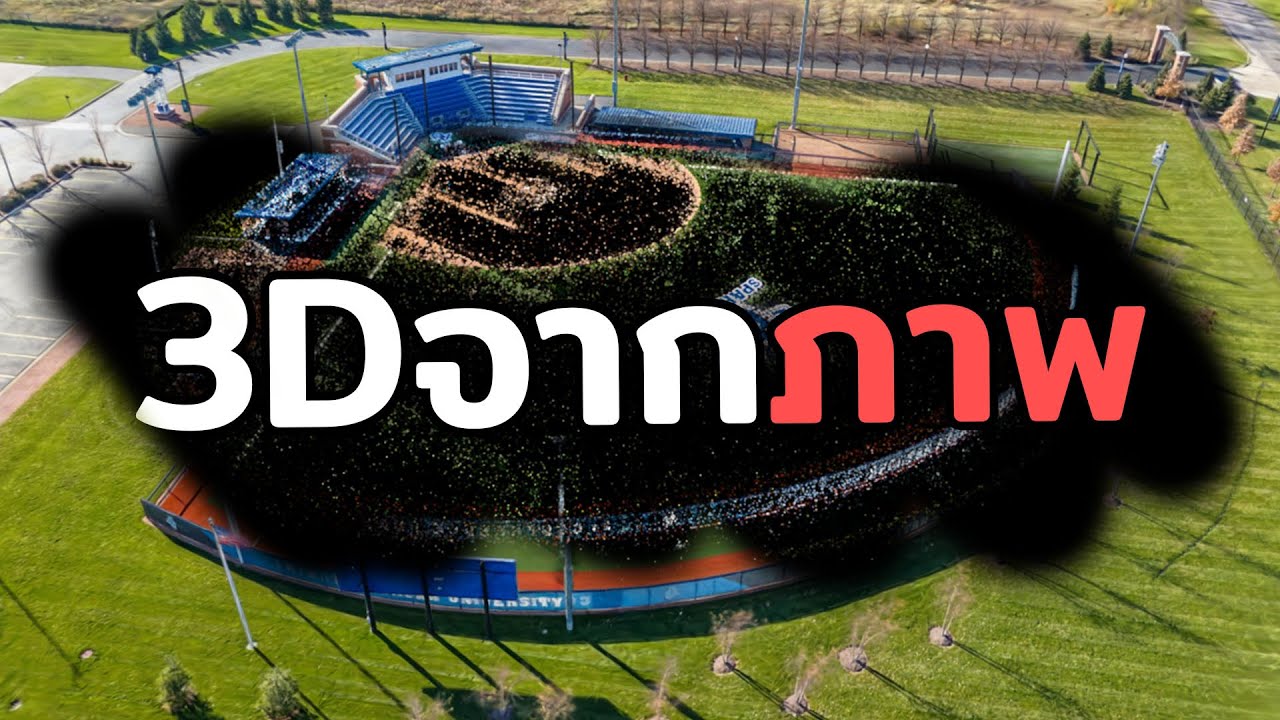
สร้าง 3D Model จากรูปภาพ
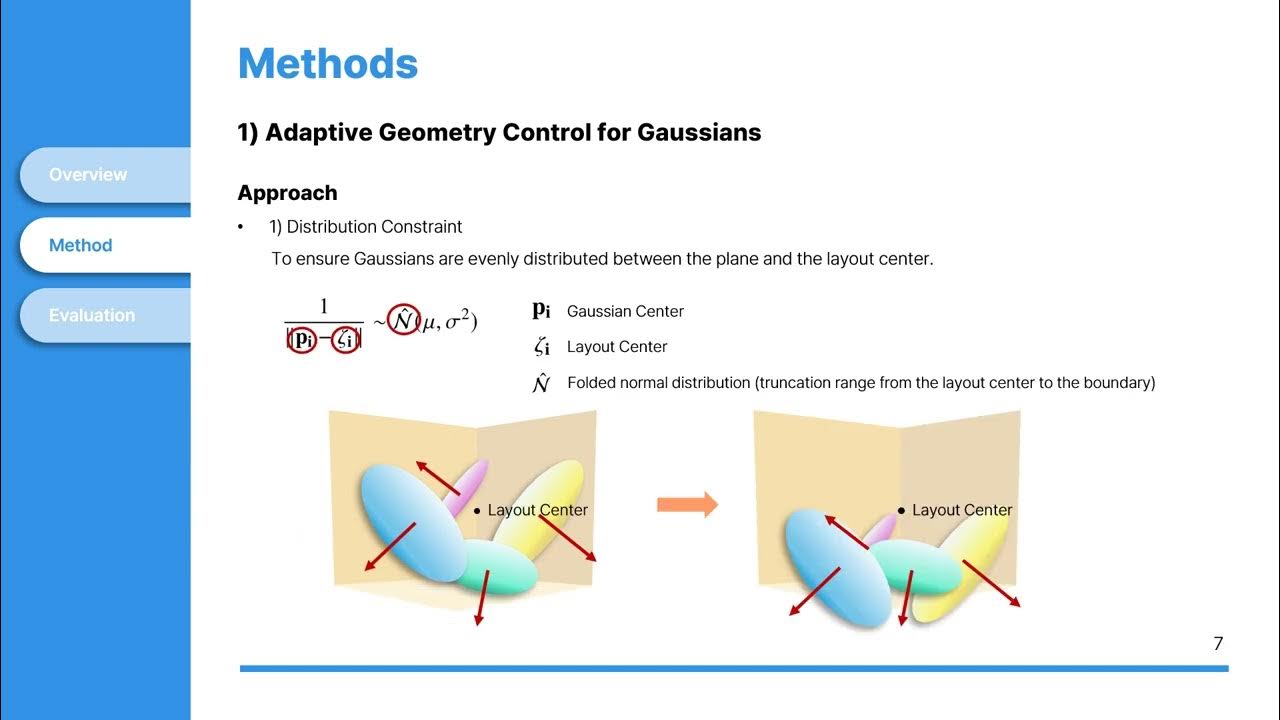
[Seminar] Text-to-3D Complex Scene Generation via Layout-guided Generative Gaussian Splatting
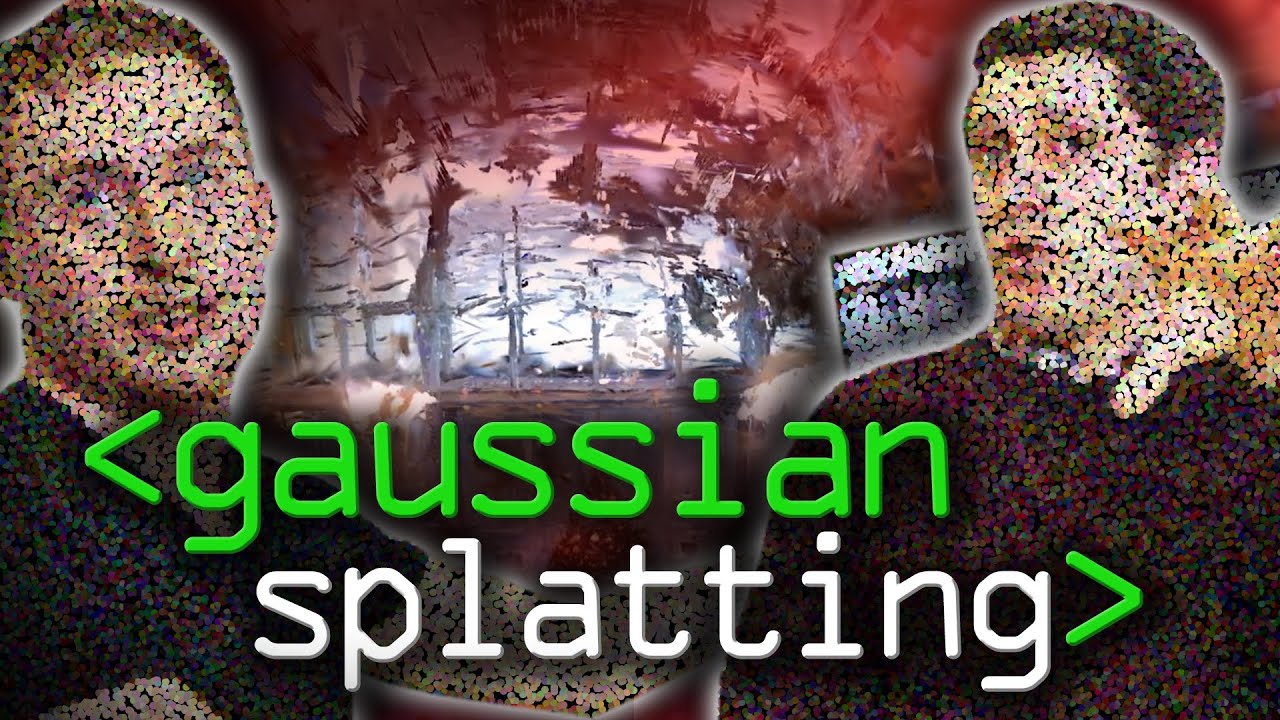
3D Gaussian Splatting! - Computerphile
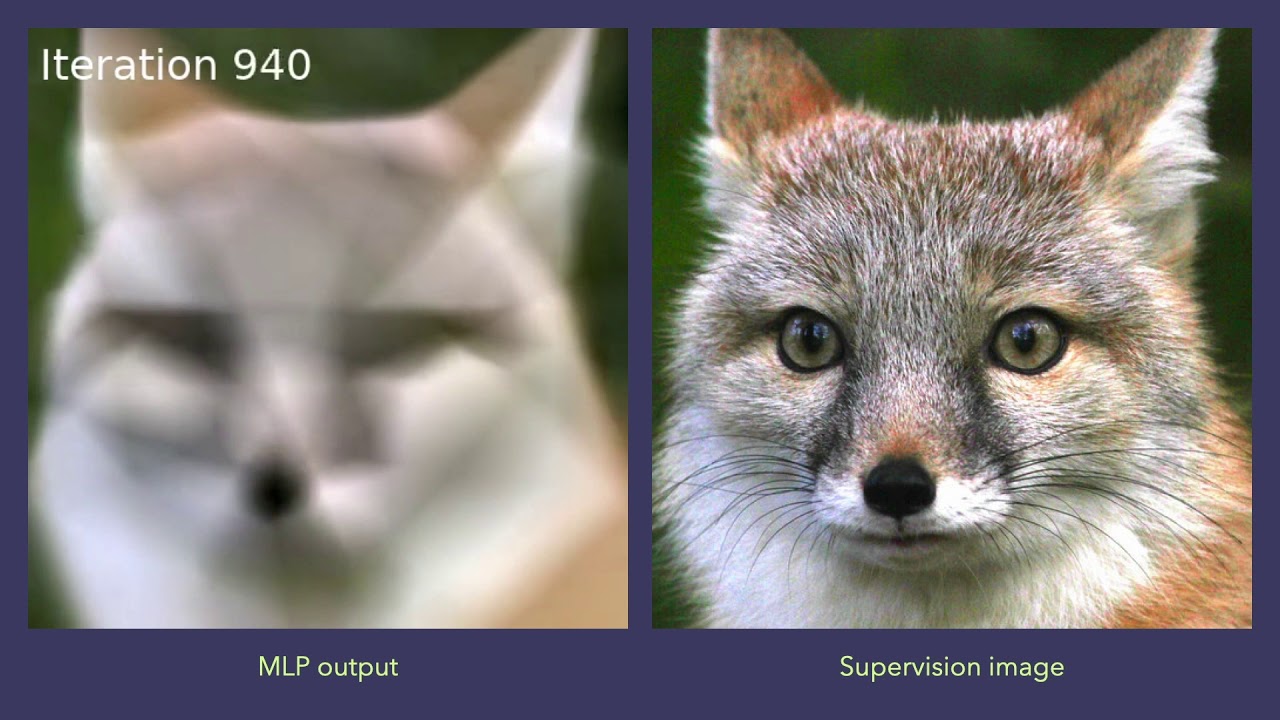
Fourier Features Let Networks Learn High Frequency Functions in Low Dimensional Domains

NVIDIA’s New AI: 50x Smaller Virtual Worlds!
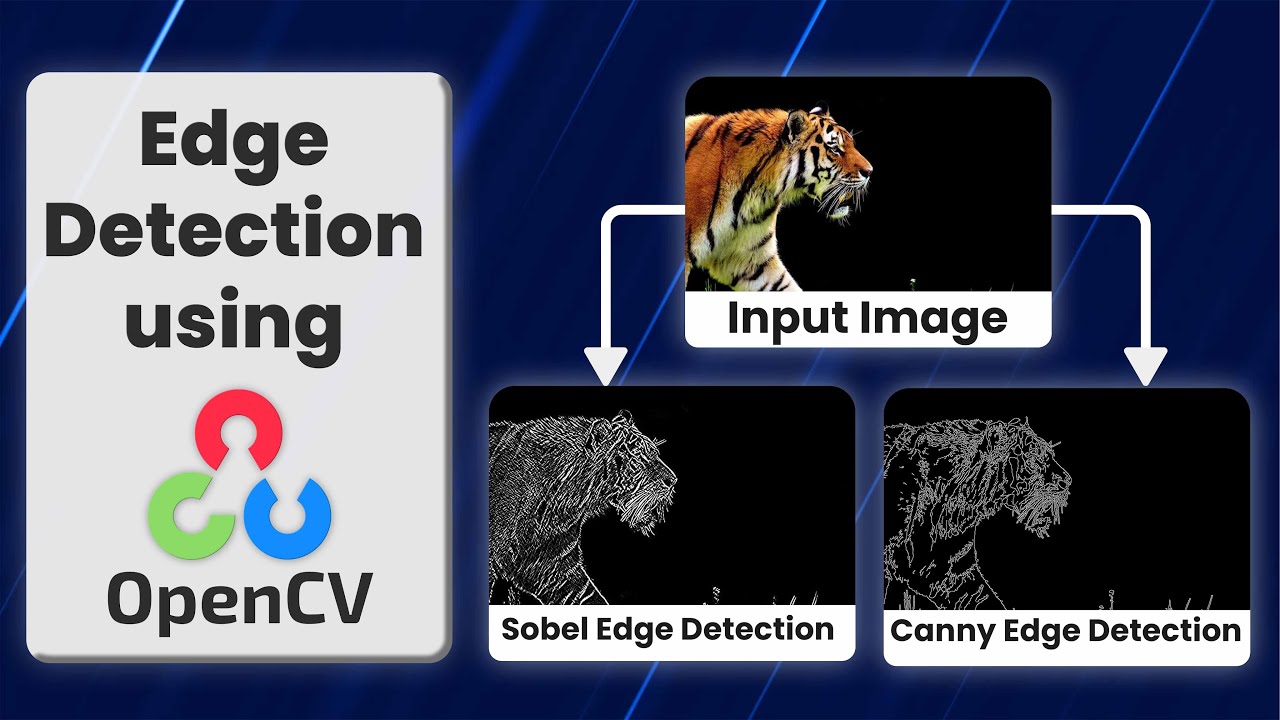
Edge Detection Using OpenCV Explained.
5.0 / 5 (0 votes)