AI Portfolio Project | I built a MACHINE LEARNING MODEL using AI in 10 MINUTES
Summary
TLDREn este video, el creador demuestra cómo construir un poderoso modelo de aprendizaje automático utilizando una asistente de IA sin necesidad de codificar. Utilizando la herramienta pcan, el creador guía a los espectadores a través del proceso de generar un modelo de pronóstico de ingresos, simplemente interactuando con el asistente de IA y respondiendo a preguntas orientadoras. El asistente construye automáticamente las consultas SQL necesarias y entrena el modelo. El video destaca lo accesible que se ha vuelto el aprendizaje automático gracias a las últimas tecnologías de IA, aunque enfatiza que las habilidades técnicas sólidas siguen siendo esenciales para optimizar aún más los modelos.
Takeaways
- 🤖 La inteligencia artificial ha simplificado enormemente la creación de modelos de aprendizaje automático, permitiendo a los usuarios construir modelos potentes sin necesidad de codificación.
- 🚀 Herramientas como pcan democratizan el acceso al aprendizaje automático, haciendo posible que tanto principiantes como expertos construyan modelos de predicción con facilidad.
- 📊 El usuario creó un modelo de pronóstico de ingresos a nivel de cliente utilizando pcan, destacando cómo la IA puede guiar a los usuarios a través del proceso con preguntas orientativas.
- 🔗 Pcan facilita la conexión con diversas fuentes de datos, permitiendo a los usuarios cargar y analizar grandes conjuntos de datos eficientemente.
- 🛠️ Aunque la plataforma permite construir modelos sin codificar, ofrece la opción de ajustar y personalizar el código, lo que brinda flexibilidad a los usuarios con habilidades técnicas.
- 📈 El modelo generado proporciona métricas y visualizaciones para evaluar su precisión, mostrando la efectividad de las herramientas de IA en la generación de modelos confiables.
- 💡 La importancia de la columna en los modelos predice qué tan significativas son las diferentes variables para los resultados del modelo, ofreciendo insights valiosos para los usuarios.
- 🎓 Mientras que las herramientas de IA simplifican la creación de modelos, el conocimiento técnico y la comprensión de los fundamentos matemáticos y estadísticos siguen siendo cruciales para optimizar los modelos.
- 🔄 El usuario puede elegir la frecuencia de las predicciones, lo que muestra la adaptabilidad de pcan a diferentes necesidades de pronóstico.
- 🌐 La facilidad de uso de pcan y la capacidad de procesar grandes volúmenes de datos destacan su potencial para democratizar el aprendizaje automático, haciéndolo accesible para un público más amplio.
Q & A
¿Qué herramienta utiliza el autor del video para construir un modelo de machine learning sin necesidad de codificar?
-El autor utiliza pcan para construir un modelo de machine learning sin necesidad de codificar.
¿Cuál es el objetivo principal del modelo de machine learning construido en el video?
-El objetivo principal del modelo es realizar una predicción de ingresos a nivel de cliente.
¿Cómo interactúa el autor con la herramienta pcan para construir su modelo?
-El autor interactúa con la herramienta pcan a través de un asistente de IA que le hace preguntas guía para ayudarle a construir su modelo.
¿Se necesita experiencia en machine learning para utilizar la herramienta mencionada en el video?
-No, la herramienta está diseñada para ser utilizada tanto por principiantes como por expertos en machine learning, permitiendo construir modelos precisos sin necesidad de codificación.
¿Qué tipo de datos utiliza el autor para construir su modelo y dónde se pueden encontrar?
-El autor utiliza un archivo CSV de una competencia de Kaggle que fue limpiado previamente. El enlace para descargar el conjunto de datos se proporciona en la descripción del video.
¿Es posible conectar la herramienta pcan a diferentes fuentes de datos?
-Sí, pcan tiene varios conectores disponibles para conectar con conjuntos de datos que estén en servidores SQL o con proveedores de nube populares.
¿Qué hace el 'notebook predictivo' que genera pcan?
-El 'notebook predictivo' actúa como el cerebro o sala de control del proceso de construcción del modelo, conteniendo las consultas SQL y los pasos necesarios para entrenar el modelo.
¿Qué son los atributos en el contexto del modelo de machine learning mencionado?
-Los atributos son los datos que el modelo utiliza para identificar y aprender los patrones que luego le permitirán hacer predicciones precisas.
¿Cuánto tiempo tardó en entrenarse el modelo en el video y qué factores influyen en este tiempo?
-El modelo se entrenó en aproximadamente una hora, pero el tiempo puede variar dependiendo de la complejidad del modelo de machine learning.
¿El autor cree que las habilidades de codificación son irrelevantes debido a la existencia de herramientas como pcan?
-No, el autor enfatiza que la capacidad de leer, escribir, entender e interpretar código sigue siendo muy importante, ya que complementa el uso de herramientas de IA como pcan.
Outlines
🤖 Construyendo Modelos de Aprendizaje Automático sin Código
El video comienza destacando la facilidad con la que ahora se pueden construir modelos de aprendizaje automático poderosos gracias a las herramientas de inteligencia artificial, como pcan. El narrador comparte su experiencia en análisis predictivo y cómo ha cambiado con el advenimiento de estas herramientas, facilitando el aprendizaje y la ejecución de tareas complejas sin necesidad de conocimientos de codificación profundos. A través de un tutorial patrocinado por pcan, demuestra cómo construir un modelo de pronóstico de ingresos a nivel de cliente sin escribir código, utilizando solo la asistencia de IA de pcan para guiarlo a través del proceso. El video invita tanto a principiantes como a expertos en aprendizaje automático a explorar la simplicidad y eficacia de utilizar tecnologías avanzadas para construir modelos, enfatizando que la accesibilidad y la rapidez en la construcción de modelos precisos son posibles con las herramientas adecuadas.
📊 Profundizando en la Construcción de Modelos con pcan
El narrador continúa explicando el proceso de construcción del modelo utilizando pcan, detallando cómo la asistencia de IA formula preguntas guía para facilitar la creación del modelo y cómo se pueden utilizar consultas SQL y notebooks generados automáticamente para personalizar el modelo sin necesidad de codificación manual. Se describe la importancia de los atributos y cómo influencian en las predicciones del modelo, así como la flexibilidad para añadir más datos si se desea. Finalmente, se resalta la facilidad y rapidez con la que se pueden entrenar modelos de alta calidad en pcan, y se discute la relevancia de las habilidades técnicas y el conocimiento estadístico en la mejora y afinación de los modelos de aprendizaje automático, concluyendo que la capacidad de utilizar herramientas de IA de última generación complementa pero no reemplaza la necesidad de competencias técnicas sólidas.
Mindmap
Keywords
💡Predicción de ingresos
💡Aprendizaje automático
💡Sin codificación
💡Asistente de IA
💡pcan
💡Conectores de datos
💡DataBricks
💡Columna de importancia
💡Notebook predictivo
💡Evaluación de modelos
Highlights
I can build a powerful machine learning model nowadays just by chatting to an AI assistant.
With AI tools and services on the rise, the barrier to learning anything really has been lowered.
I'll show you the simplest way I know to build a strong machine learning model with no actual coding involved.
I used pcan, who have kindly also sponsored this video, but as always, all opinions, thoughts and reflections on the tool will of course be absolutely of my own.
You'll get to see how simple and effective it can be to utilize the latest technologies to build your own machine learning models regardless of your experience.
Even though you can build precise models with zero coding involved, you can of course adjust your code within pcan.
I walked through how I built a revenue forecasting model, but you could obviously predict other things like LTV, customer turn or winback, marketing mix or how to up and cross sell.
I got to interact with the pcan AI assistant, which asked me some guiding questions to help me build my machine learning model.
The assistant recommended the subject of my prediction, the target value, and all I had to do was to confirm that all was good so far.
The tool asked me how far into the future I'd like to predict the revenue, and I went with the most popular future time horizon - a month.
I chose to go with predicting on a monthly basis, but could have triggered predictions based on specific events.
Pcan generated the Predictive Analytics question for me, and I moved on to connecting to my data by uploading a CSV file.
The AI assistant looked at the schema of the file and recommended the column mappings based on the schema for each column type.
Pcan did all the heavy lifting for me in the background, so all I had to do was to click on generate notebook and go to it.
The predictive notebook acted as the brains or the control room of the entire model building process, with SQL queries and explanations built by the AI assistant.
Transcripts
I truly think it's insane that I can
build a powerful machine learning model
nowadays just by chatting to an AI
assistant I remember when I first
started my career doing Predictive
Analytics was hard really hard with AI
tools and services on the rise the
barrier to learning anything really has
been lowered or at the very least you
can learn whatever you want quicker if
you know what tools to use and how to
use them in the most efficient way in
today's video I'll show you the simplest
way I know to build a strong machine
learning model with no actual coding
involved I used pcan who have kindly
also sponsored this video but as always
all opinions thoughts and Reflections on
the tool will of course be absolutely of
my own whether you're a machine learning
newbie or have some or maybe lots and
lots of experience training your own
models I'd encourage you to stick around
as you'll get to see how simple and
effective it can be to utilize the
latest Technologies to build build your
own machine learning models regardless
of your experience and I say regardless
of experience because even though you
can build precise models with zero
coding involved you can of course adjust
your code within pcan I'll show you
later how so I'll walk you through how I
built a revenue forecasting model but
you could obviously predict other things
like LTV customer turn or winback
marketing mix or how to up and cross
sell you can try and build your own
model the same way by the way ass
signing up to pcan is completely free
I'll put the link in the description
below along with the link to the exact
data set that I used so after I logged
in straight away I got to interact with
the pcan AI assistant the way the
assistant works is that it asked me some
guiding questions to help me build my
machine learning model so I answered the
first question and told the AI assistant
that I would like a revenue forecast
model at the customer level this simply
means that I want to predict what the
revenue is for each and every customer
the assistant is pretty smart as it
recommended the subject of my prediction
which was the customer of course and my
target value which was the revenue and
all I had to do was to confirm that all
was good so far then I got another
guiding question asking me how far into
the future I'd like to predict the
revenue for each customer you can choose
whatever you want really next week month
quarter or even year but I went with
probably the most popular future time
Horizon a month year then the tool asked
me on what recurring basis I'd like to
make these Revenue predictions again I
went with something simplistic here so I
said that I'd like the frequency of the
predictions to be monthly I also had the
options to trigger my predictions based
on specific events for example when a
customer makes a purchase but for this
exercise for this model I chose to go
with predicting on a monthly basis then
pcan generated the Predictive Analytics
question for me all I had to do was to
confirm that everything still looked
good and I moved on to connecting to my
data I uploaded my CSV file which is
actually a kaggle competition file that
was cleaned up a bit and as I said
before the link is in the description
below so you can go ahead and download
it and build your own machine learning
model now of course pcon has a bunch of
connectors when it comes to connecting
to your data sets so if you have
something sitting on SQL servers or with
popular Cloud providers that's not a
problem at all pcan is um capable of
processing lots and lots of information
as it uses data bricks so you should
feel confident about loading massive
data sets after the file upload was
complete I simply sent it to the chat
and let the tool do its work with
analyzing the data the AI assistant
looked at the schema of the file and
recommended the column mappings of the
data set based on the schema for each
column type I quickly saw what the data
type was I confirmed that everything
looked good and moved on this time the
tool actually figured it out that the
user ID column was the customer ID the
amount represented the revenue for each
transaction and the event time was the
date or timestamp that I needed again I
confirmed that everything looked good
and then I got a summary with my
predictive question the schema my Target
table Target value column Target table
date column and the target entity pcan
did all the heavy lifting for me in the
background so now all I had to do was to
click on generate notebook and then go
to it to see what's actually in there
think of the predictive notebook that I
just generated as the brains or the
control room of the entire model
building process I had my SQL queries in
here that the AI assistant built for me
you can also see that all of the steps
were clearly explained each query had a
name and was actually saved kind of like
a view so then the next query or sell
could utilize the data from the query
above for example the sampled customers
table could utilize the information from
the monthly sampling table so Pan's AI
assistant constructed the core set for
me by using the answers I provided to
the guided questions now what do I mean
by a coret it is the data that allowed
my model to to know what it needs to
learn and where it needs to learn from
so the coret was a final table that
consisted of all users sample dates and
the target value which in my case was
the revenue within 1 month after the
sample date now the last thing in my
notebook was the attribute section and
if you have no idea what this is don't
worry as I'm going to explain this in a
very simple way and attribute is the
data that my model will use to identify
and find the patterns that will then
tell my my model what the predictions
will be so when the model is training
it's learning the patterns to produce
the correct Target values long story
short use the training set to predict
your Target on an ongoing basis then use
your model in real life pcan also has
the option to add more attributes if I
wanted to so I could have easily added
more by adding more data whether it was
just me uploading some files or
connecting to one of the many databases
that are supported now of of course if I
added more data I could have just used
the AI assistant to replace my already
existing query so that it runs on my new
data set again no coding involved just
pure AI magic I wanted to keep my model
nice and light so I chose not to add any
more attributes I just went with the one
I already had my notebook had everything
I needed to train my model using the
data set I uploaded into the chat
earlier I hit run all which ran all of
my SQL queries
then I trained my model now I had two
options fastest which is by no surprise
fast as it only takes about 10 to 30
minutes to train a model and production
quality which provides better
performance but takes a little longer to
train I know it says several hours here
but my model was actually built in about
an hour or so the time it takes will of
course depend on how complex your
machine learning model is so once I hit
train model P can quickly rans some data
valid ations to check that my data was
actually fit for building a predictive
model and that was it I sat back and
waited for about an hour and I had my
Model results with some cool metrics and
visualizations that helped me to gauge
how good my model actually was I could
see on the model evaluation tab under
model performance that my model was very
precise meaning the model was very close
to the actual values the pan platform
has a really powerful engine behind it
hence the really good result results
clearly their data teams know what
they're doing now the model evaluation
tab has a bunch of other metrics as well
and I won't go through all of them but I
will highlight column importance which
was very useful to know in my model I
could see that the amount column was by
far the top contributing column to the
model predictions which makes sense
another helpful tab I used for
interpreting my Model results was the
model output tab I could quickly see
what the prediction was for each user
for each month I'm getting more and more
used to how much and how fast AI can do
things but at times it is still
mindboggling to me that it allows me to
do such difficult tasks like building a
strong machine learning model just by
interacting with an AI chat assistant
now does this mean that coding is
useless absolutely not the ability to
read write understand and interpret code
is still very very important in my
opinion just think of this who can
develop the better machine learning
model in a shorter time frame the person
who knows how to use the latest AI tools
but has no real technical expertise or
the person who also knows how to use the
latest AI tools and has extremely strong
technical skills surely the person with
the strong technical skills will be able
to fine-tune the model better and faster
using the same AI tools compared to the
person with little technical expertise
not to mention all of the underlying
math and statistical knowledge that is
of course also essential to building
good machine learning models anyway I'm
going to end this video here if you
enjoy content like this make sure to
check out some of my other videos right
here thank you so much for watching and
I shall see you in the next
[Music]
one
Ver Más Videos Relacionados
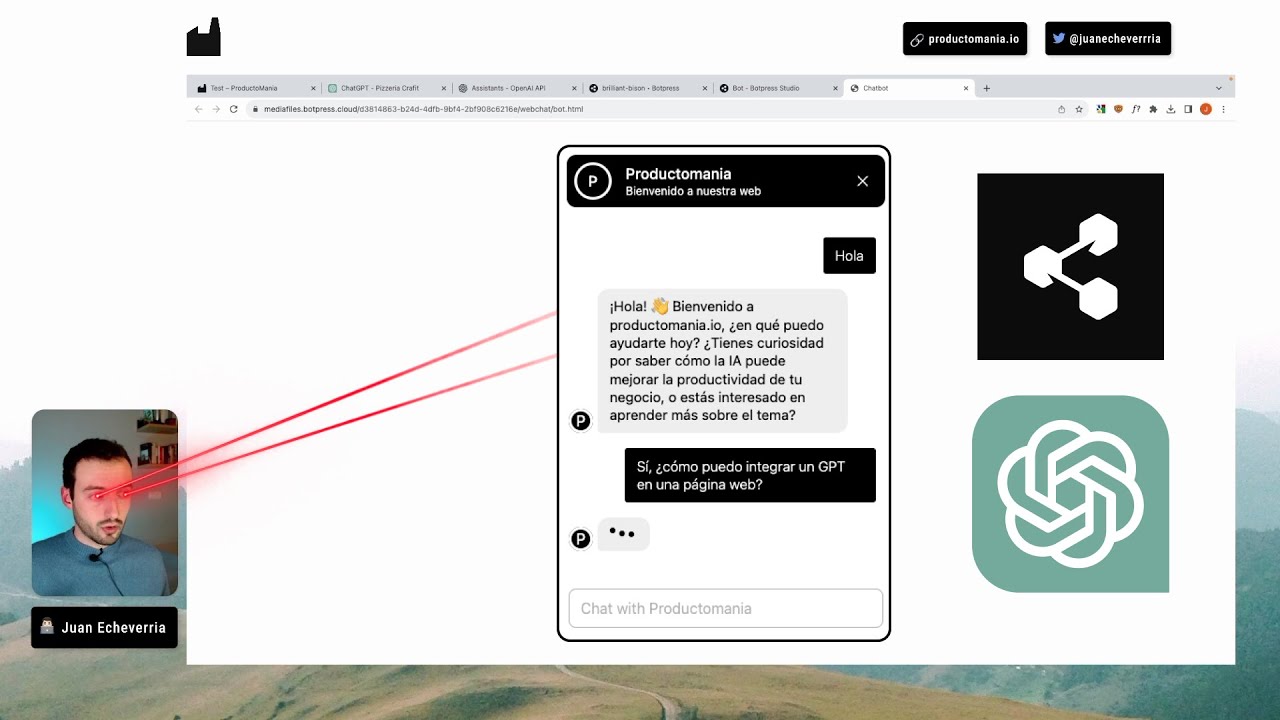
Cómo EMBEBER UN GPT en una página WEB [Tutorial paso a paso]
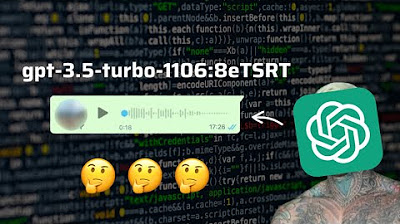
Cómo ENTRENAR tu PROPIO MODELO de OPENAI para que HABLE COMO UN FAMOSO
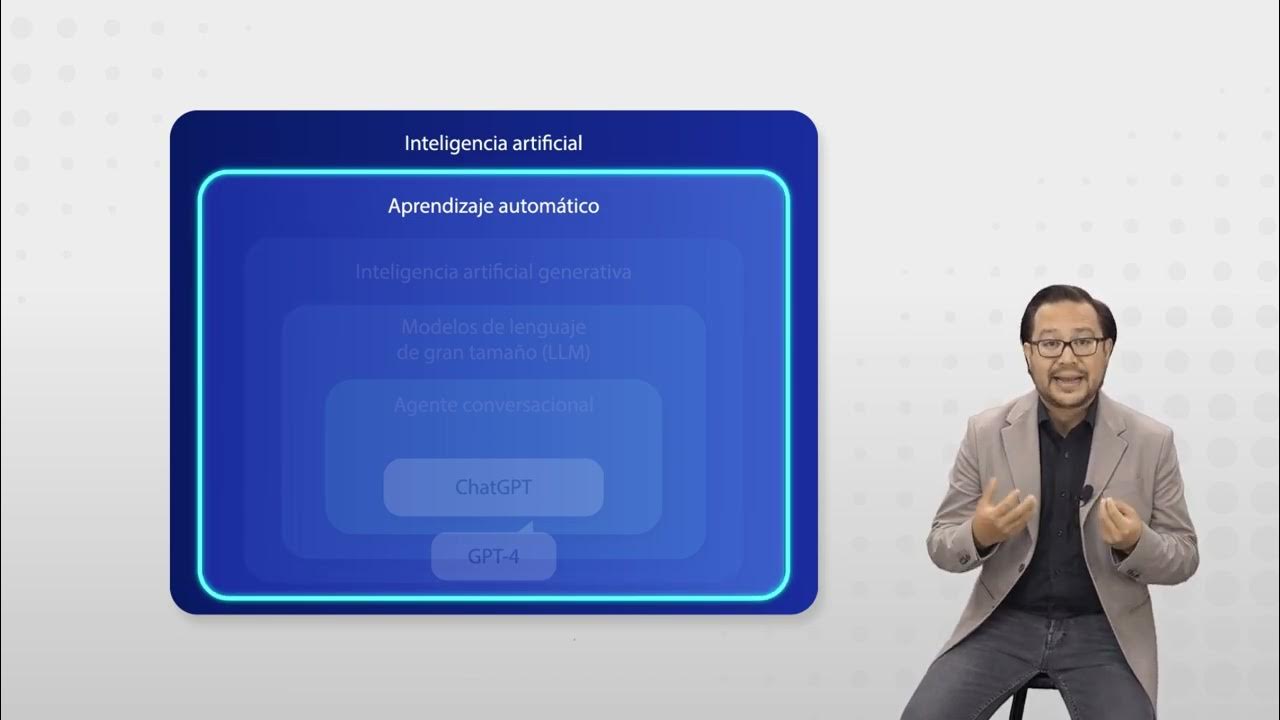
Qué es Inteligencia Artificial Generativa?
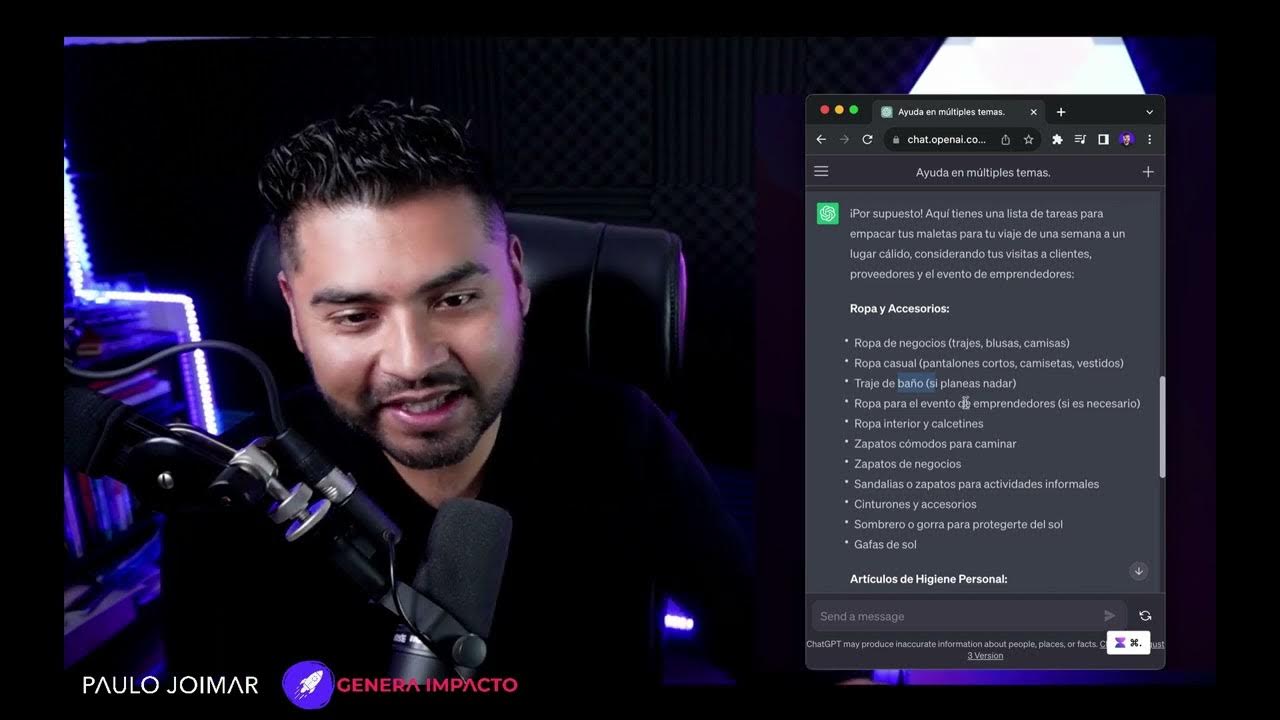
1 5
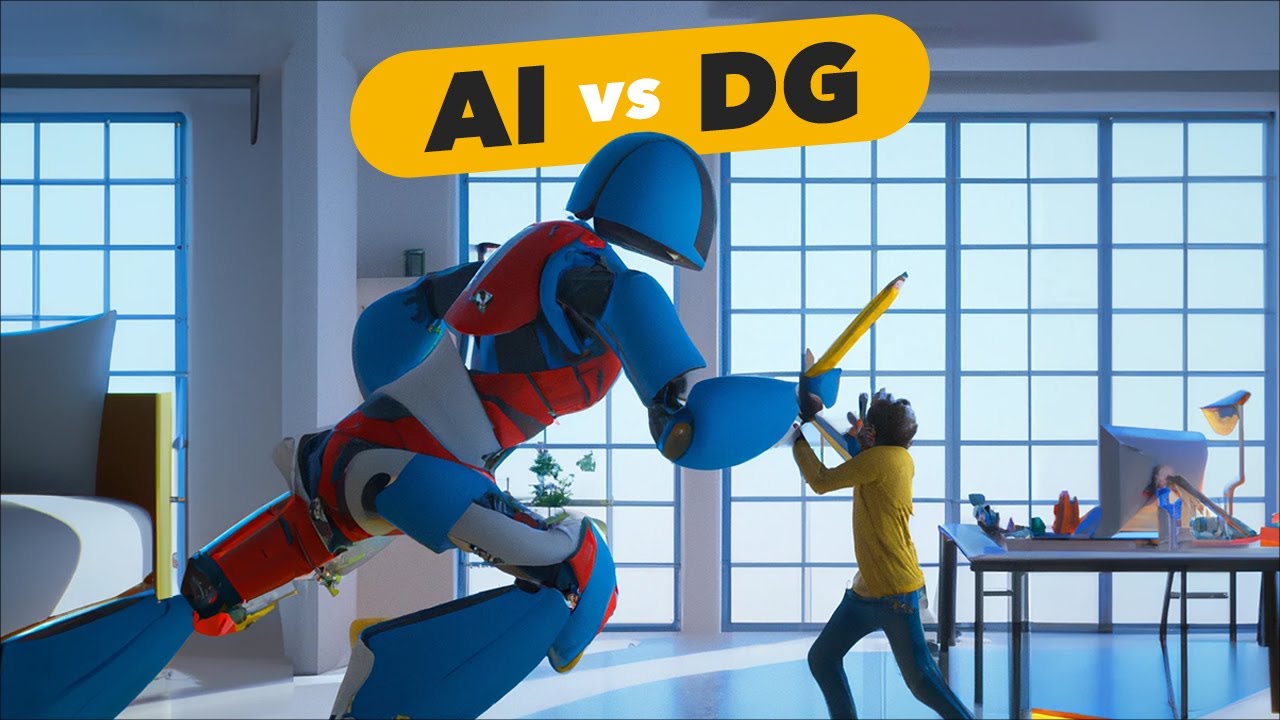
Inteligencia Artificial VS Diseñador Gráfico (QUÉDATE AL FINAL) | Geekformante
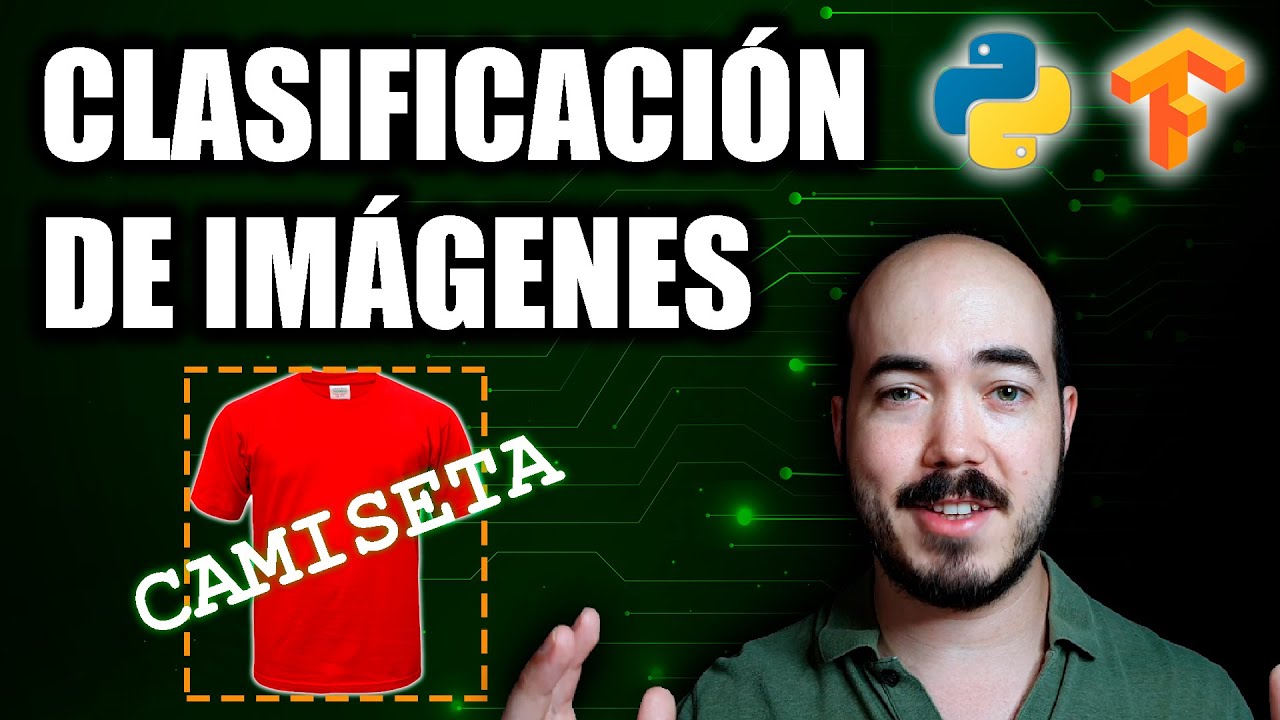
Tu primer clasificador de imágenes con Python y Tensorflow
5.0 / 5 (0 votes)