Image classification + feature extraction with Python and Scikit learn | Computer vision tutorial
Summary
TLDRIn this advanced computer vision tutorial, Felipe demonstrates building an image classifier using feature extraction with the 'image-to-vec with pytorch' repository. The tutorial utilizes a weather dataset categorized into cloudy, rain, shine, and sunrise images. Felipe guides viewers through data preparation, model training with a RandomForestClassifier from scikit-learn, performance testing, and model saving. The video concludes with a demonstration of model inference, achieving a high accuracy of 94.4%, and encourages viewers to compare this method with previous tutorials using YOLO v8 and Teachable Machine.
Takeaways
- 😀 Felipe introduces an advanced computer vision tutorial focused on image classification and feature extraction.
- 🌐 The tutorial uses a weather dataset with four categories: cloudy, rain, shine, and sunrise, which has been used in previous videos.
- 📁 The dataset is organized into 'train' and 'val' directories for training and validation data respectively.
- 🛠 Felipe demonstrates using a GitHub repository called 'image-to-vec with pytorch' for feature extraction from images.
- 💻 The process begins in PyCharm by creating a new Python project and setting up a virtual environment with Python 3.8.
- 📦 Necessary Python packages are installed, including 'image-to-vec/pytorch', 'scikit-learn', and 'pillow'.
- 🔍 The script iterates over images in the dataset, using 'os' and 'PIL' to open and process images, computing features with 'Image2Vec'.
- 📝 Features and labels are stored in lists and then saved in a dictionary with keys for training and validation data and labels.
- 🌳 A 'RandomForestClassifier' from 'scikit-learn' is chosen for the machine learning model, which is trained using the training data and labels.
- 📊 The model's performance is tested using the validation data, achieving a 94.4% accuracy score.
- 🔒 The trained model is saved using 'pickle' for future use, and a new script 'infer.py' demonstrates how to load and make predictions with the model.
Q & A
What is the main topic of the video?
-The main topic of the video is building an image classifier using feature extraction for image classification in the field of computer vision.
What dataset is used in the tutorial?
-The tutorial uses a weather dataset that includes four categories: cloudy, rain, shine, and sunrise.
What are the two main directories in the dataset?
-The two main directories in the dataset are 'train' for training data and 'val' for validation data.
What is the GitHub repository mentioned for feature extraction?
-The GitHub repository mentioned for feature extraction is called 'image-to-vec-with-pytorch', which is used to extract features from images.
Which Python packages are installed for this tutorial?
-The Python packages installed for this tutorial are 'image-to-vec-pytorch', 'scikit-learn', and 'pillow'.
What is the first step in the process described in the video?
-The first step in the process is to prepare the data for training the image classifier.
How are the features extracted from the images?
-Features are extracted from the images using the 'Image2Vec' object from the 'image-to-vec-pytorch' package by calling the 'get_vec' method on the image.
What classifier from scikit-learn is used in the tutorial?
-The tutorial uses the 'RandomForestClassifier' from scikit-learn for the image classification model.
How is the model's performance evaluated?
-The model's performance is evaluated using the accuracy score computed with the validation data, which is unseen data for the model.
What is the final accuracy achieved by the model in the video?
-The final accuracy achieved by the model in the video is 94.4 percent.
How is the trained model saved for later use?
-The trained model is saved using the 'pickle' library by opening a file named 'model.p' in write-binary mode and calling 'pickle.dump' with the model object.
How can the saved model be used for making predictions?
-The saved model can be loaded using 'pickle.load' by opening the 'model.p' file in read-binary mode and then used to make predictions by calling the 'predict' method on new feature data.
Outlines
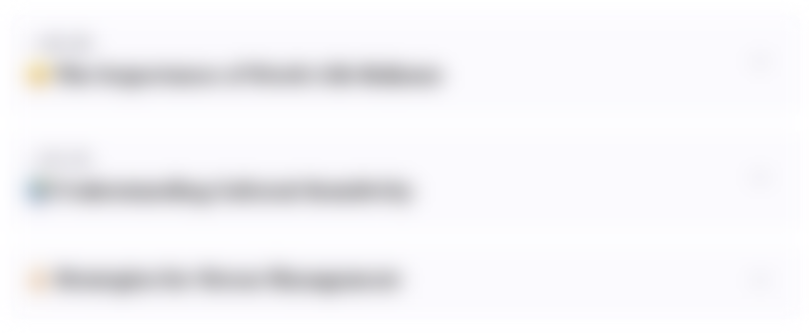
Dieser Bereich ist nur für Premium-Benutzer verfügbar. Bitte führen Sie ein Upgrade durch, um auf diesen Abschnitt zuzugreifen.
Upgrade durchführenMindmap
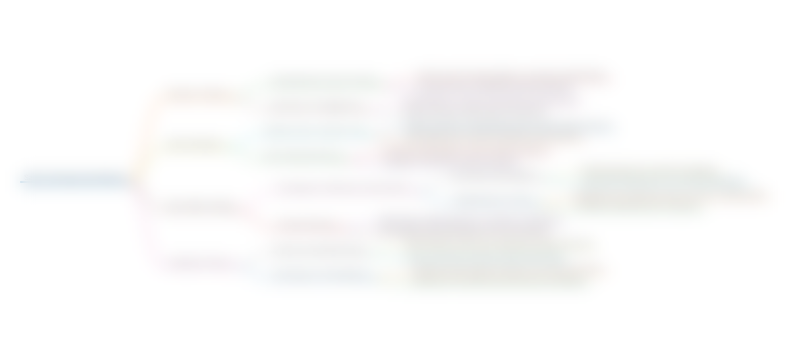
Dieser Bereich ist nur für Premium-Benutzer verfügbar. Bitte führen Sie ein Upgrade durch, um auf diesen Abschnitt zuzugreifen.
Upgrade durchführenKeywords
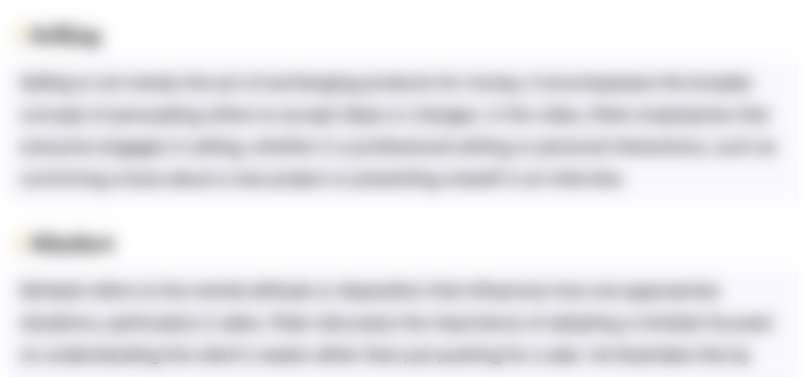
Dieser Bereich ist nur für Premium-Benutzer verfügbar. Bitte führen Sie ein Upgrade durch, um auf diesen Abschnitt zuzugreifen.
Upgrade durchführenHighlights
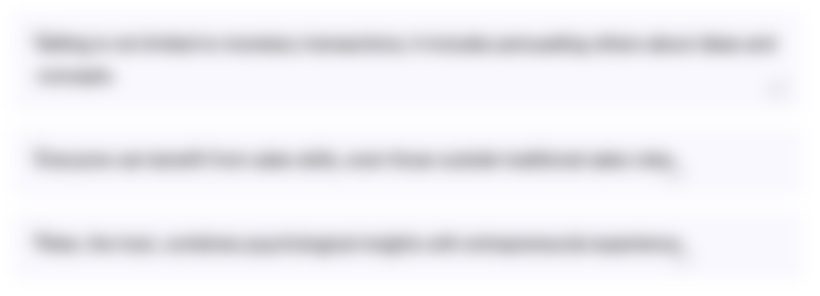
Dieser Bereich ist nur für Premium-Benutzer verfügbar. Bitte führen Sie ein Upgrade durch, um auf diesen Abschnitt zuzugreifen.
Upgrade durchführenTranscripts
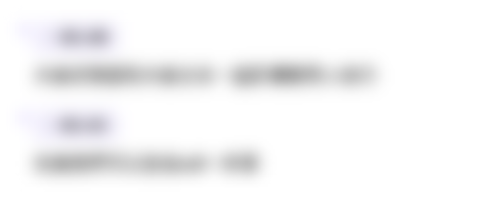
Dieser Bereich ist nur für Premium-Benutzer verfügbar. Bitte führen Sie ein Upgrade durch, um auf diesen Abschnitt zuzugreifen.
Upgrade durchführenWeitere ähnliche Videos ansehen
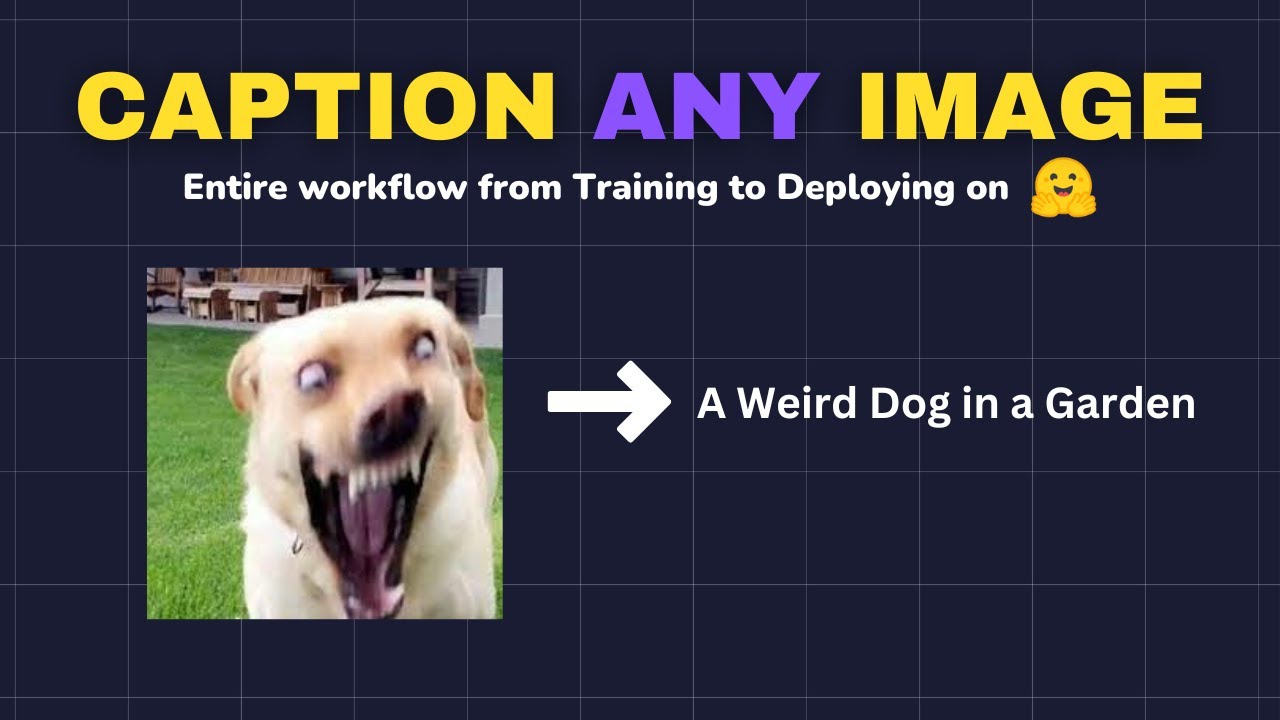
How to Make Your Images Talk: The AI that Captions Any Image
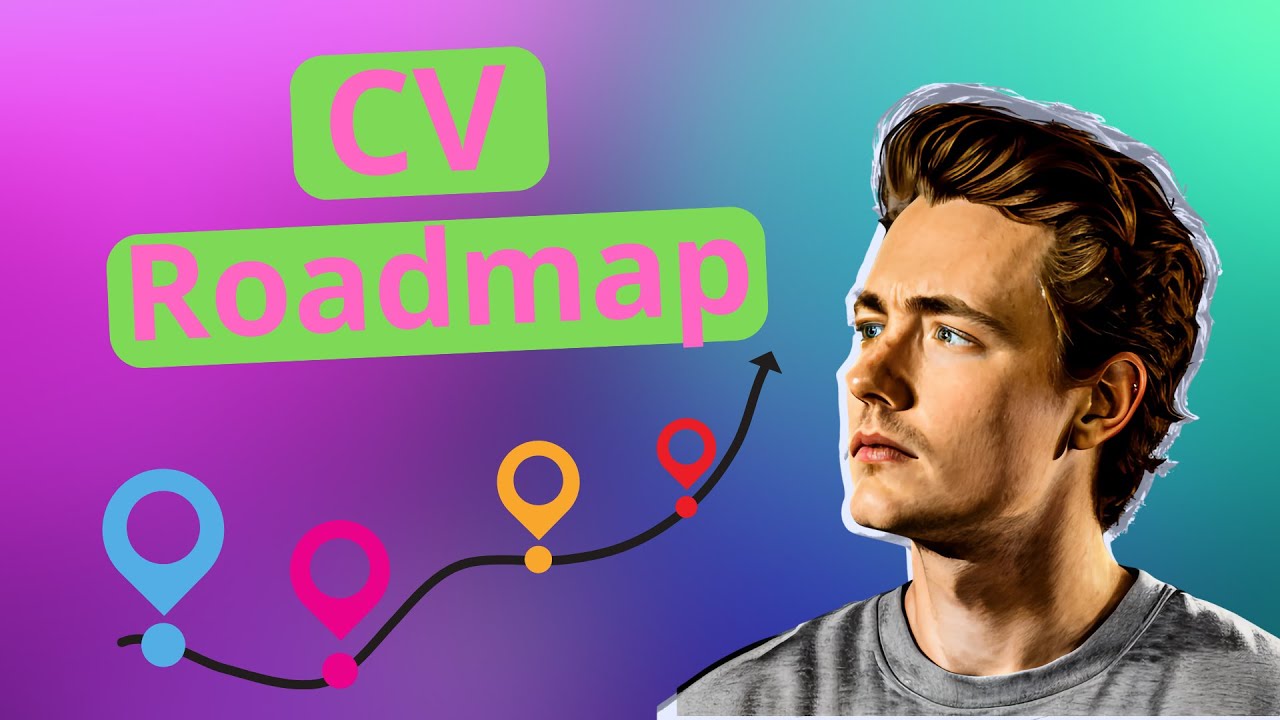
How to Get Started with Computer Vision - Beginner to Advanced Roadmap
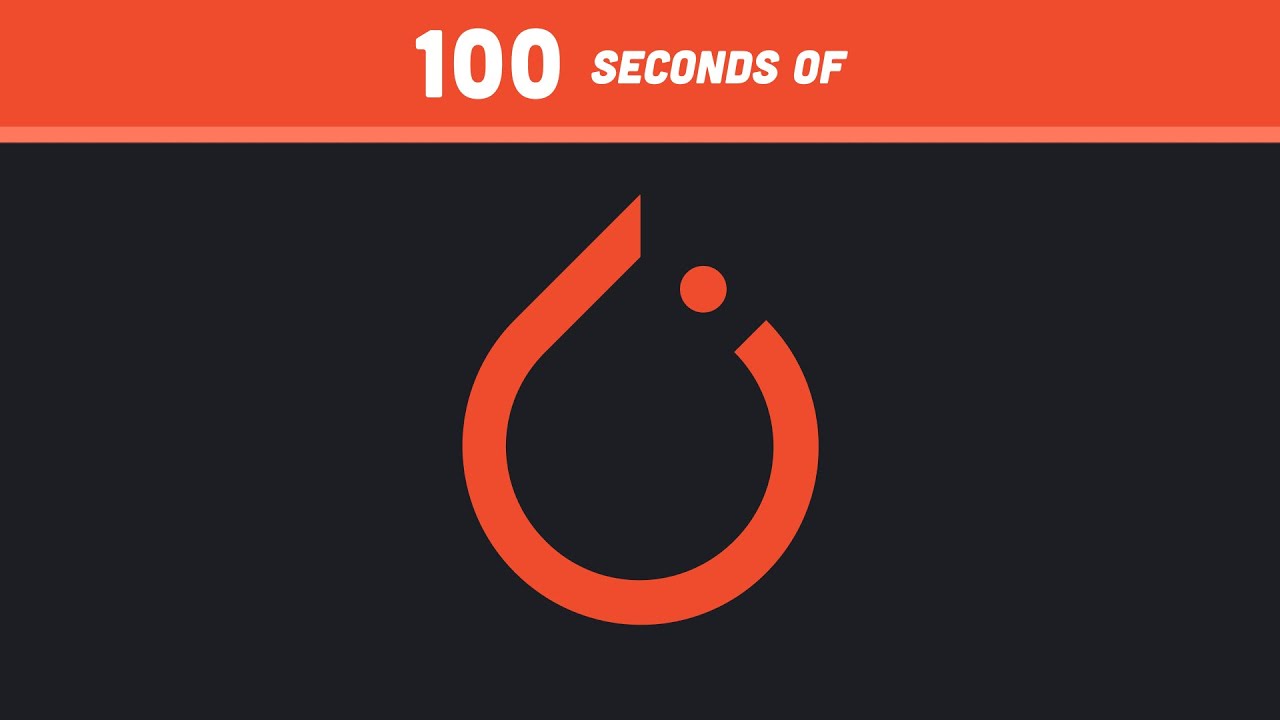
PyTorch in 100 Seconds

Connecting Raspberry Pi w/ Pixhawk and Communicating via MAVLink Protocol
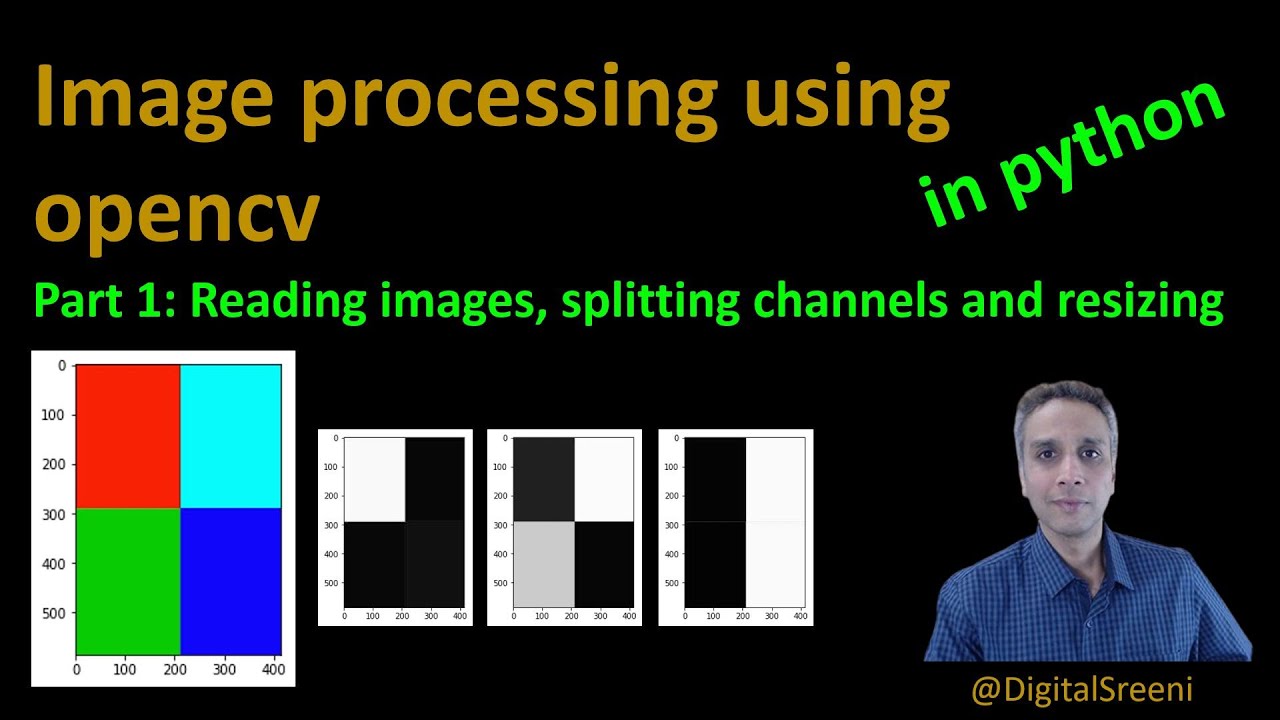
25 - Reading Images, Splitting Channels, Resizing using openCV in Python

Auto Image Segmentation using YOLO11 and SAM2
5.0 / 5 (0 votes)