Is the AI Boom Real?
Summary
TLDRThe author traveled to Japan and the U.S. to learn about the AI chip industry. Much buzz surrounds Sam Altman raising huge funds for a new AI chip venture. The concept of 'scaling laws' suggests that with more data and compute, AI models like GPT continue improving. This drives massive investment. Nvidia faces competition but responds aggressively. Giants like Microsoft vertically integrate to cut costs. OpenAI's ChatGPT generated $2B revenue, but it's unclear if consumer demand can sustain the AI boom. The industry hopes Microsoft's Copilot subscription catches on. If not, some assumptions need rethinking. AI's main impact could just be improving ad targeting for giants like Google and Meta.
Takeaways
- 😲 The chip venture Sam Altman is working on could involve up to $1-7 trillion in investments over several years, largely for data centers and manufacturing
- 📈OpenAI's 'scaling laws' show that combining more data and compute leads to better AI model performance
- 👀Nvidia is aggressively updating its AI chips to stay ahead of new competitors
- 🚨Tech giants like Microsoft and Google want to cut costs by making their own custom AI chips
- 🤔It's unclear if demand from mainstream consumers will sustain the massive investments expected in AI
- 🙌ChatGPT is one of the fastest growing consumer tech products ever at over $2 billion annual revenue
- 🔎All eyes are on Microsoft's Copilot subscription to indicate enterprise demand for AI
- 💰AI promises automation that could greatly boost advertising revenue
- 😕But AI may just make rich companies even richer, not necessarily transform industries
- ✈The author plans more trips to learn about and discuss the AI landscape
Q & A
What was the purpose of the author's recent travel to Japan and the United States?
-The purpose was part sight-seeing and part learning tour to understand more about the AI and AI chip boom landscape outside of Taiwan.
What figure is Sam Altman said to be seeking for investments related to AI chips?
-Initially it was reported to be billions, but more recently a figure of $1-7 trillion was reported, likely referring to total investments over multiple years related to manufacturing, real estate, power, etc.
What concept is driving the massive investments forecasted in AI chips?
-The 'scaling laws' concept that combining more data and compute leads to better AI model performance. This concept helped drive advances like GPT-3 and GPT-4.
How is Nvidia responding to increased competition in AI chips?
-By aggressively increasing the pace of updates to their AI accelerator lineup, releasing new products as often as every 6 months.
How might vertical integration by tech giants like Microsoft impact Nvidia?
-In the medium to long term, as tech giants develop more advanced custom AI chips, it could reduce Nvidia's market share. Nvidia aims to stay ahead by leading on performance and cost.
What evidence is there so far that AI products are driving real consumer demand?
-There are some signs of promise like ChatGPT's reported $2 billion annualized revenue run rate, but it's still early. Microsoft's Copilot subscription will be an important test case.
Where else might AI have a major financial impact?
-In advertising and automation, where AI could greatly boost revenues and efficiencies for major platforms like Google and Meta.
What does the author see as a disappointing possibility for AI?
-That its biggest impact is automating and optimizing existing industries rather than enabling wholly new products and companies, making the rich richer rather than producing new wealth.
What approach does the author recommend for embedding AI capabilities?
-Recommend embedding AI into existing successful products to maximize its impact, using AI as an enabling technology rather than a standalone consumer product.
What are the author's plans going forward regarding AI?
-The author plans to make more frequent trips to the US and other regions to have interesting conversations with people in the AI space and keep abreast of the latest developments.
Outlines
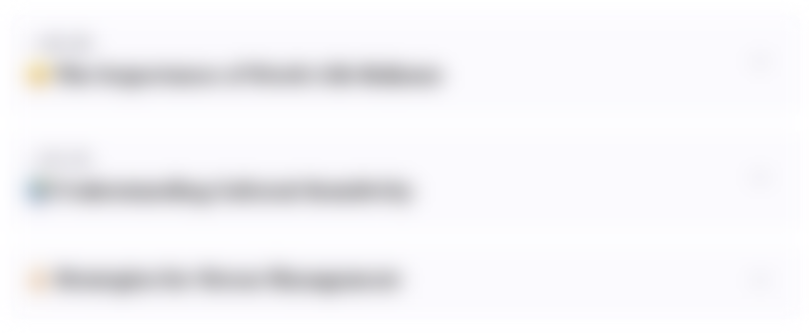
Dieser Bereich ist nur für Premium-Benutzer verfügbar. Bitte führen Sie ein Upgrade durch, um auf diesen Abschnitt zuzugreifen.
Upgrade durchführenMindmap
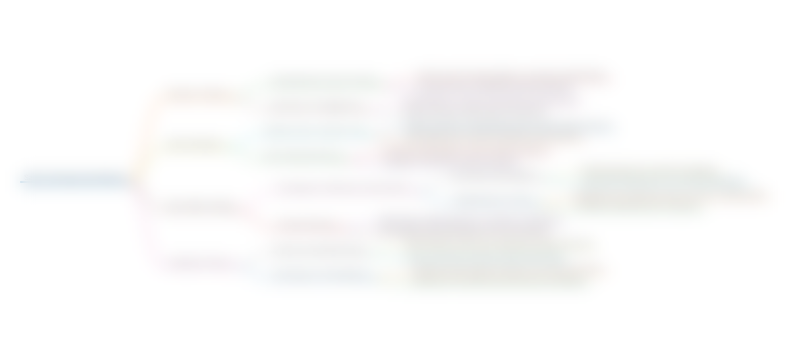
Dieser Bereich ist nur für Premium-Benutzer verfügbar. Bitte führen Sie ein Upgrade durch, um auf diesen Abschnitt zuzugreifen.
Upgrade durchführenKeywords
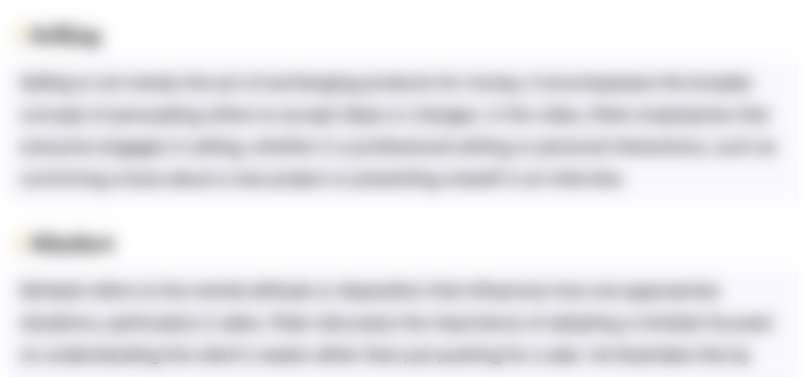
Dieser Bereich ist nur für Premium-Benutzer verfügbar. Bitte führen Sie ein Upgrade durch, um auf diesen Abschnitt zuzugreifen.
Upgrade durchführenHighlights
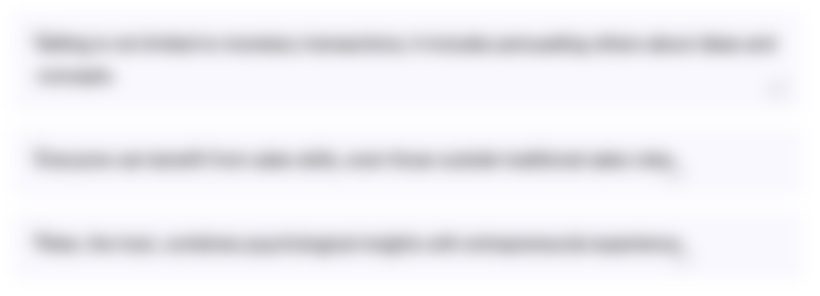
Dieser Bereich ist nur für Premium-Benutzer verfügbar. Bitte führen Sie ein Upgrade durch, um auf diesen Abschnitt zuzugreifen.
Upgrade durchführenTranscripts
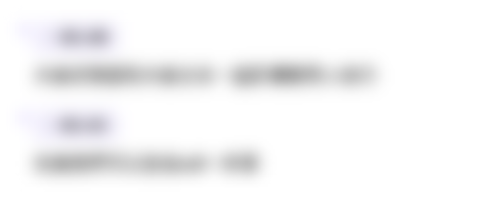
Dieser Bereich ist nur für Premium-Benutzer verfügbar. Bitte führen Sie ein Upgrade durch, um auf diesen Abschnitt zuzugreifen.
Upgrade durchführenWeitere ähnliche Videos ansehen
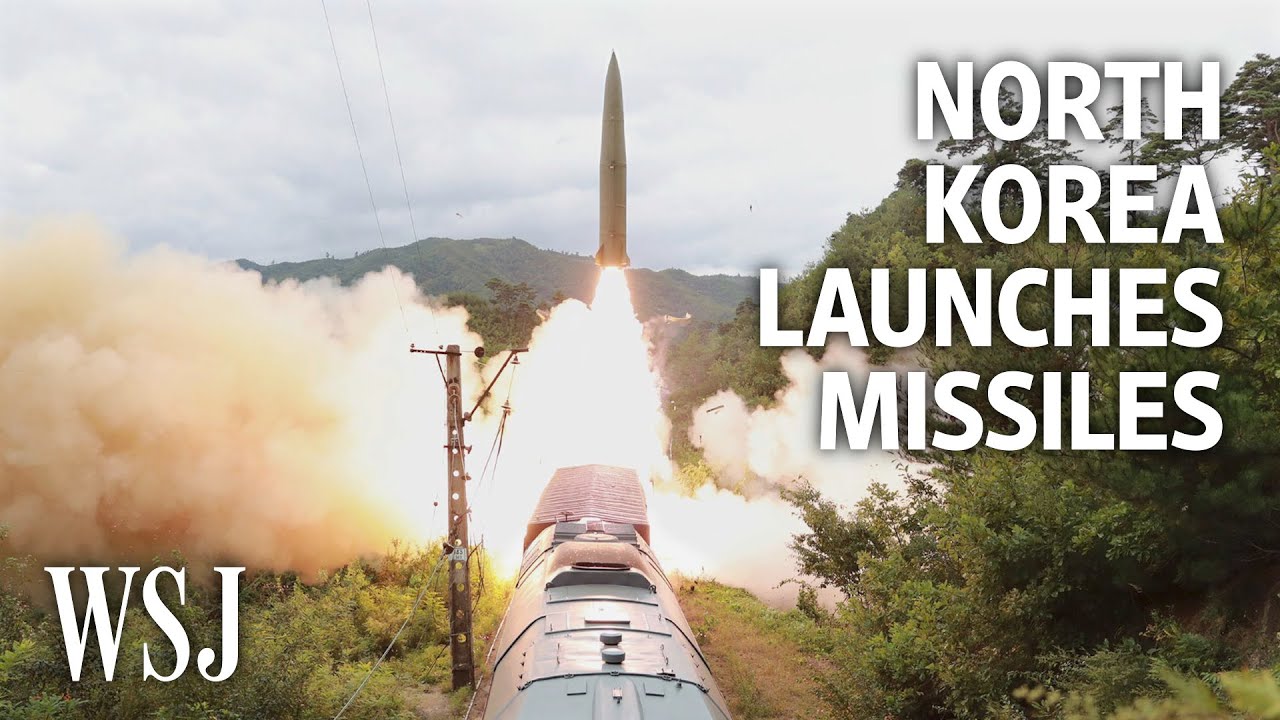
North Korea Launches Missiles From Trains, Seeking U.S. Attention | WSJ
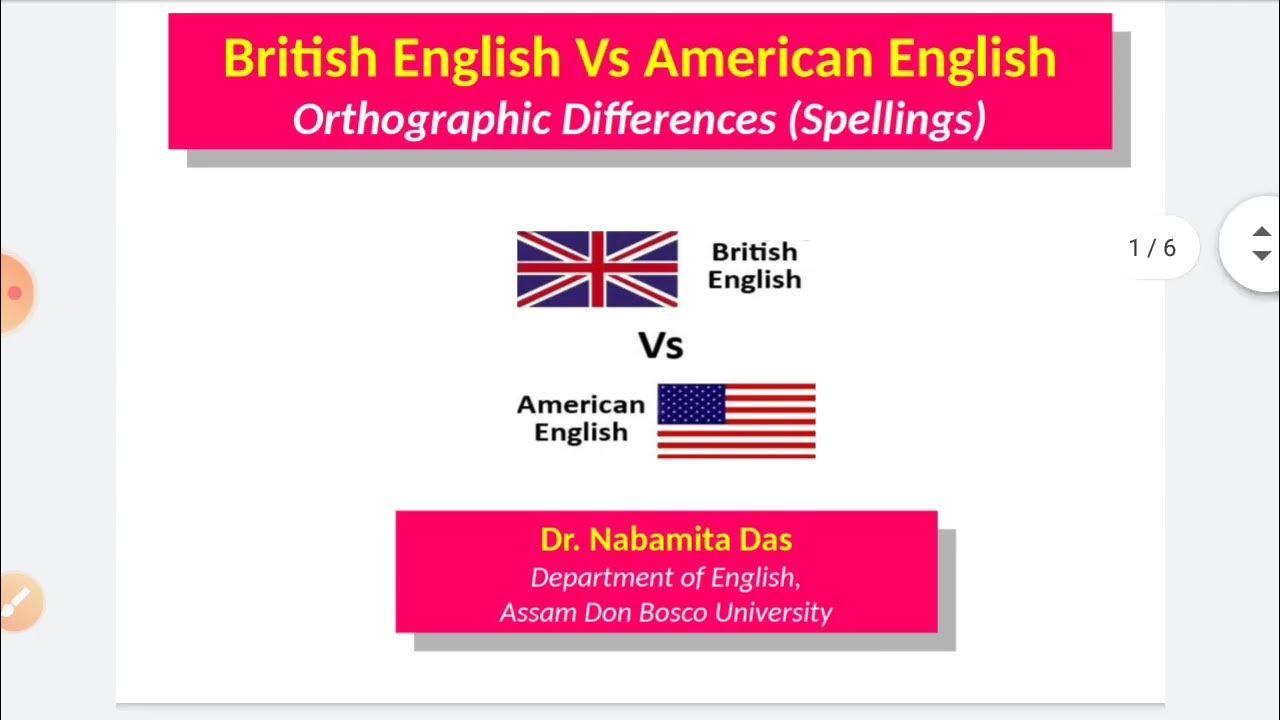
Orthographic Differences II American English Vs British English

Kasus Virus Corona Pertama di Indonesia
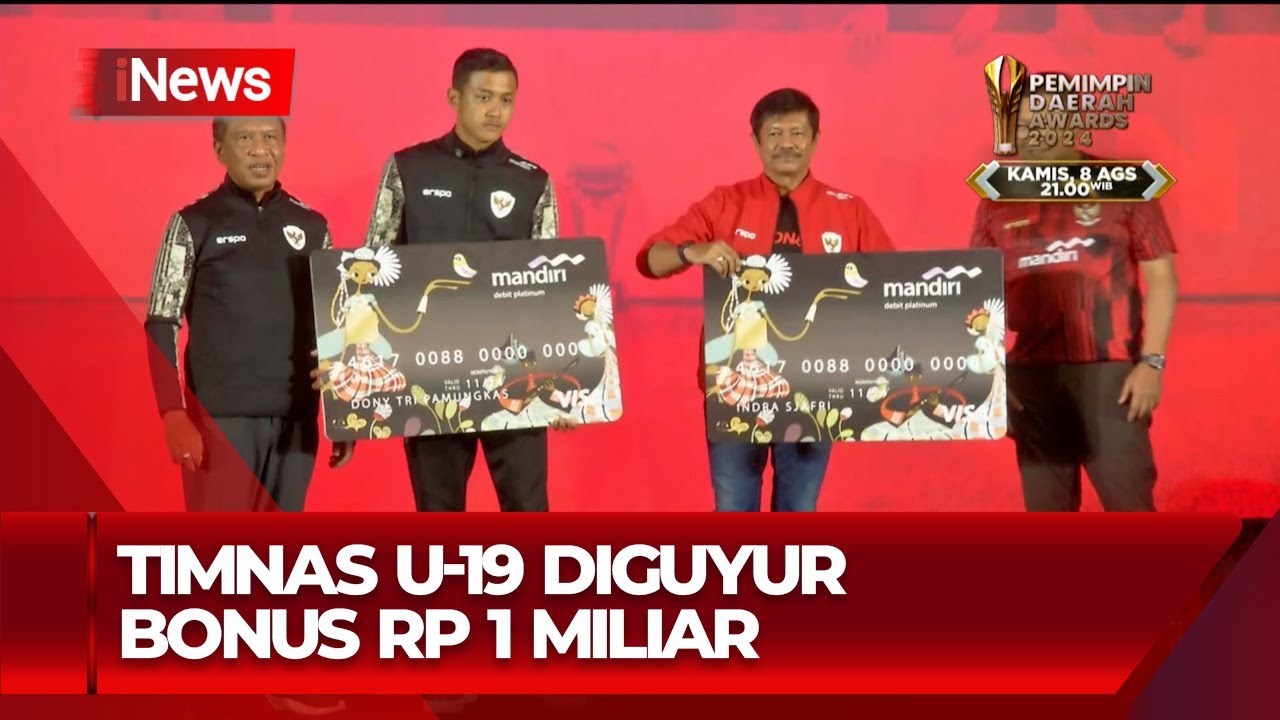
Apresiasi Usai Timnas Juara Piala AFF U-19 2024 - iNews Pagi 01/08
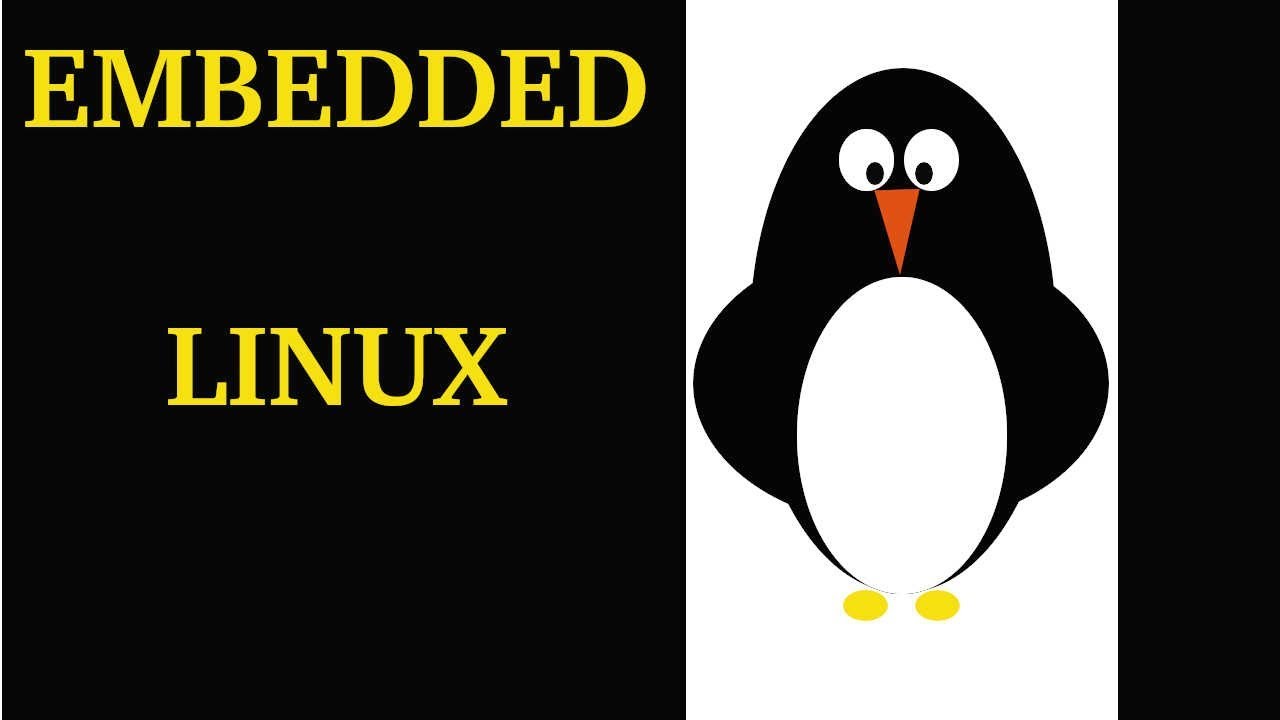
Embedded Linux | Introduction To U-Boot | Beginners
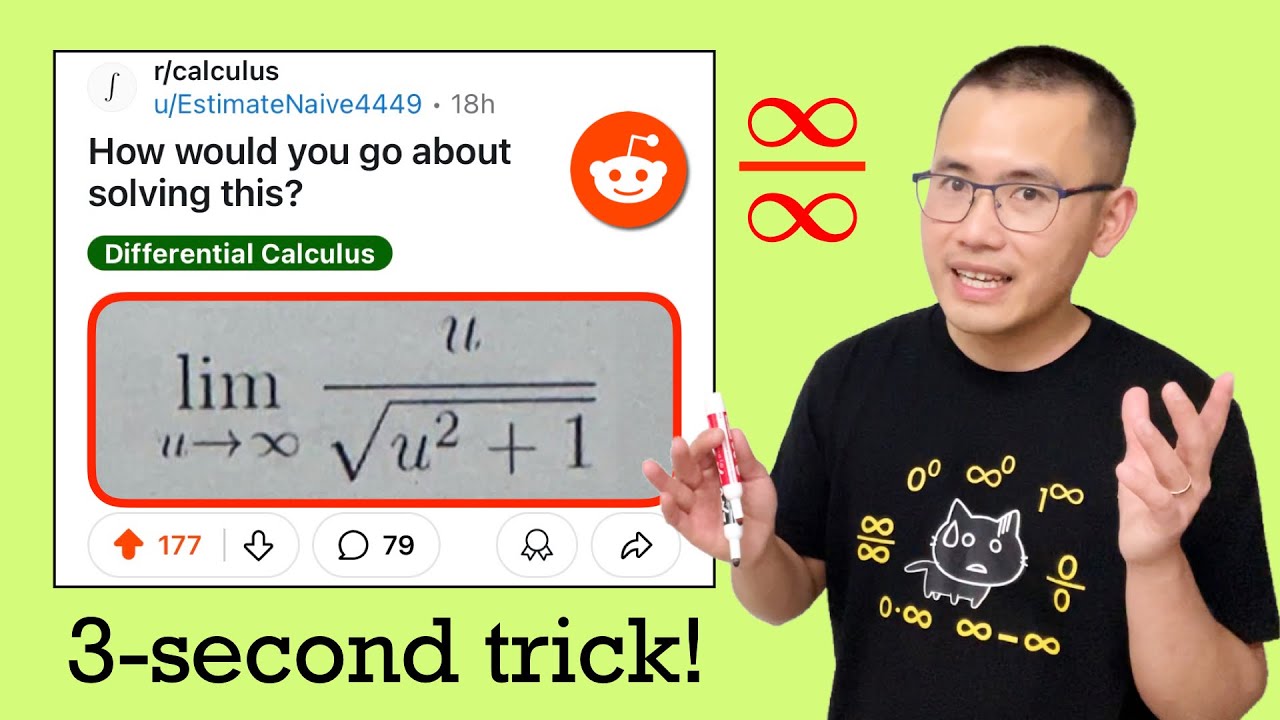
How would you go about solving this? Limit of x/sqrt(x^2+1) as x goes to infinity. Reddit inf/inf
5.0 / 5 (0 votes)