Mixture-of-Agents Enhances Large Language Model Capabilities
Summary
TLDRThe video script explores the collaborative potential of Large Language Models (LLMs), highlighting their enhanced performance when referencing outputs from other models. It introduces the Mixture of Agents (MOA) methodology, which iteratively refines responses through multiple LLMs, outperforming single models. The script discusses the significant improvements achieved by MOA on benchmarks like Alpaca, AVAL 2.0, and FLASK, showcasing its effectiveness in reasoning and language generation without fine-tuning.
Takeaways
- 🧠 Large Language Models (LLMs) have transformed natural language understanding and generation through vast data training aligned with human preferences.
- 📈 Despite remarkable capabilities, LLMs face limitations in size and training data, with scaling up being costly and each model having unique strengths.
- 🤝 The concept of 'collaborativeness of LLMs' is introduced, where models perform better when they can reference outputs from other models, even if individually less capable.
- 🔑 A new methodology called Mixture of Agents (MOA) is proposed, which leverages multiple LLMs to iteratively enhance response quality.
- 🛠️ MOA uses a layered structure of agents that generate and refine responses, aiming to overcome individual model limitations through collaborative synthesis.
- 🏆 The MOA framework achieves state-of-the-art performance on benchmarks like Alpaca, AVAL 2.0, demonstrating significant improvements over single LLMs.
- 🔍 The script highlights the importance of model diversity in MOA, showing that a variety of LLMs in each layer can improve overall performance.
- 🌟 Models like GPT-4, Quen 1.5, and Wizard LM are identified as excelling in both proposing and aggregating roles within the MOA framework.
- 💡 The MOA framework is inspired by the Mixture of Experts (MoE) technique in machine learning, extending the concept to operate at the model level through the prompt interface.
- 🚀 MOA variants like MOA with GPT-40 and MOA Light are developed, focusing on high-quality outputs and cost-effectiveness, respectively.
- 📊 The script discusses the impact of model diversity and the number of proposers on output quality, showing that more diverse and numerous agents enhance performance.
Q & A
What is the main focus of the section on Large Language Models (LLMs)?
-The section focuses on how Large Language Models (LLMs) have revolutionized natural language understanding and generation, their capabilities, limitations, and the concept of combining multiple LLMs to create a more powerful model.
What is meant by the 'collaborativeness of LLMs'?
-The 'collaborativeness of LLMs' refers to the phenomenon where models perform better when they can refer to outputs from other models, even if those models are not as capable individually.
What is the Mixture of Agents (MOA) methodology?
-The Mixture of Agents (MOA) methodology is a framework that leverages multiple LLMs to enhance response quality iteratively. It involves layers of agents that generate and refine responses until a robust and comprehensive output is achieved.
How does the MOA structure work in practice?
-In practice, the MOA structure uses layers of LLMs that generate and refine responses. Each LLM processes an input text and generates its continuation without needing fine-tuning. The final output is obtained by concatenating texts from all LLMs and applying an aggregation and synthesis prompt.
What is the significance of the evaluation of MOA using various benchmarks?
-The evaluation using various benchmarks demonstrates significant improvements with MOA, achieving a state-of-the-art win rate on benchmarks like Alpaca, AVAL 2.0, showing the effectiveness of the collaborative approach in enhancing reasoning and language generation.
What roles do proposers and aggregators play in the MOA framework?
-In the MOA framework, proposers provide diverse perspectives, while aggregators synthesize responses into high-quality outputs. This categorization helps in leveraging the strengths of different models for better collaboration.
How does the MOA framework differ from traditional mixture of experts techniques?
-The MOA framework extends the mixture of experts technique to operate at the model level using LLMs entirely through the prompt interface, without modifying internal activations or weights, thus eliminating the need for fine-tuning and offering flexibility.
What are the variants of the MOA model mentioned in the script?
-The script mentions two variants of the MOA model: MOA with GPT-40, which focuses on high-quality outputs, and MOA Light, which prioritizes cost-effectiveness by using only two MOA layers and a different aggregator.
How does the number of proposers impact the final output quality in the MOA framework?
-The output quality improves as the number of proposers increases, indicating the advantages of having more auxiliary information and a greater variety of LLM agents in each MOA layer.
What insights were gained from the experiments exploring the internal mechanism of MOA?
-The experiments showed that MOA significantly outperforms LLM rankers, indicating that the aggregator likely performs sophisticated aggregation over all proposed outputs, and MOA tends to incorporate the best proposed answers as shown by positive correlations between similarity and preference scores.
How does the script address the optimization of LLMs for various tasks?
-The script discusses recent advancements in optimizing LLMs for various tasks through prompt engineering techniques like Chain of Thought (CoT) and natural program prompting, as well as exploring model ensembles and collaboration strategies to improve response quality.
Outlines
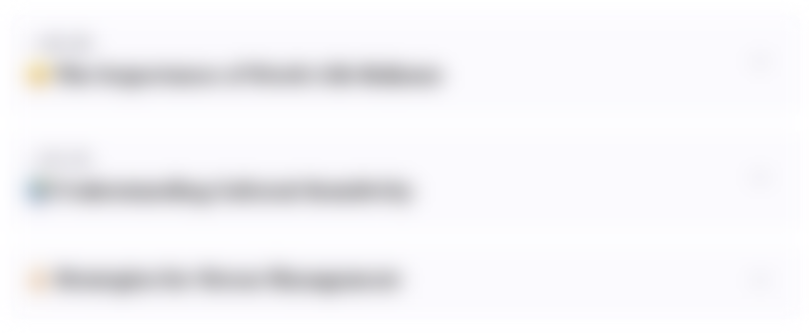
Dieser Bereich ist nur für Premium-Benutzer verfügbar. Bitte führen Sie ein Upgrade durch, um auf diesen Abschnitt zuzugreifen.
Upgrade durchführenMindmap
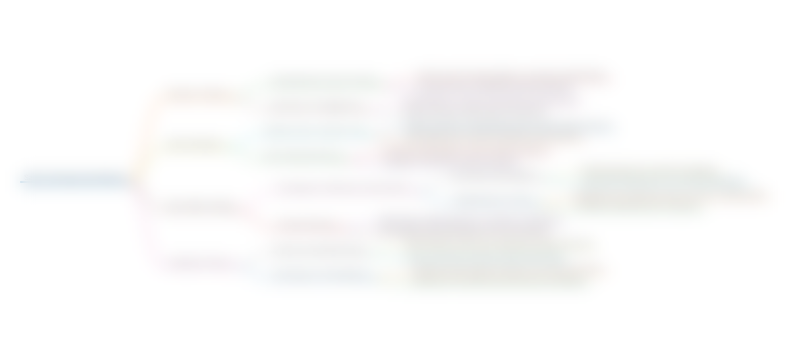
Dieser Bereich ist nur für Premium-Benutzer verfügbar. Bitte führen Sie ein Upgrade durch, um auf diesen Abschnitt zuzugreifen.
Upgrade durchführenKeywords
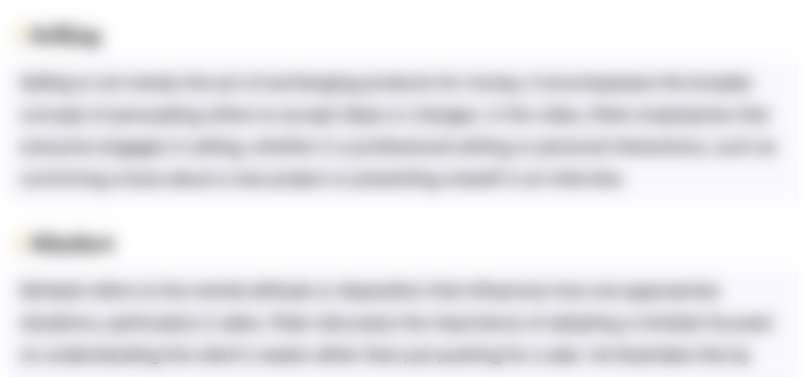
Dieser Bereich ist nur für Premium-Benutzer verfügbar. Bitte führen Sie ein Upgrade durch, um auf diesen Abschnitt zuzugreifen.
Upgrade durchführenHighlights
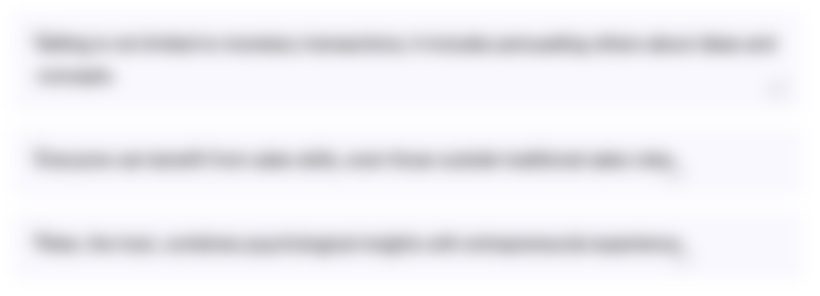
Dieser Bereich ist nur für Premium-Benutzer verfügbar. Bitte führen Sie ein Upgrade durch, um auf diesen Abschnitt zuzugreifen.
Upgrade durchführenTranscripts
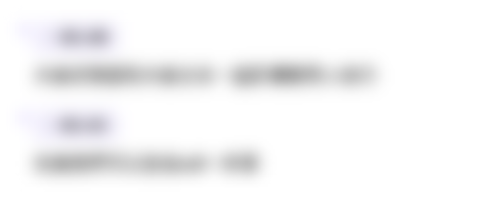
Dieser Bereich ist nur für Premium-Benutzer verfügbar. Bitte führen Sie ein Upgrade durch, um auf diesen Abschnitt zuzugreifen.
Upgrade durchführenWeitere ähnliche Videos ansehen
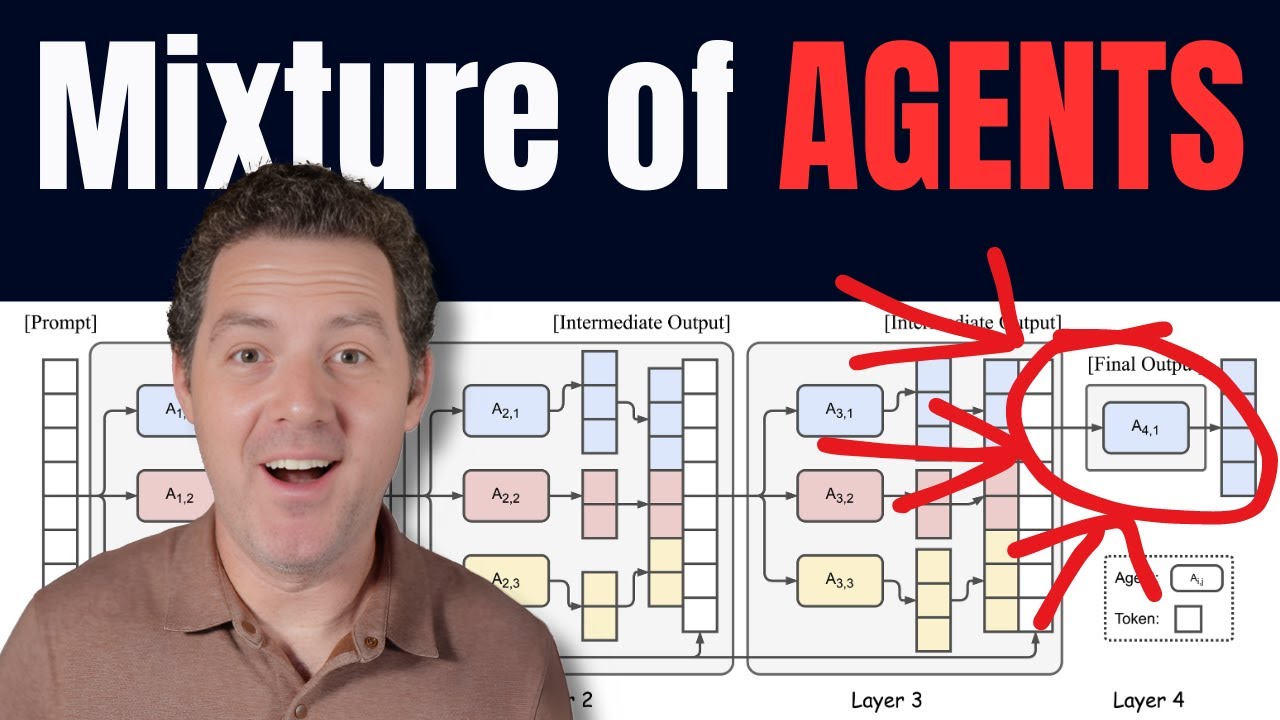
MoA BEATS GPT4o With Open-Source Models!! (With Code!)
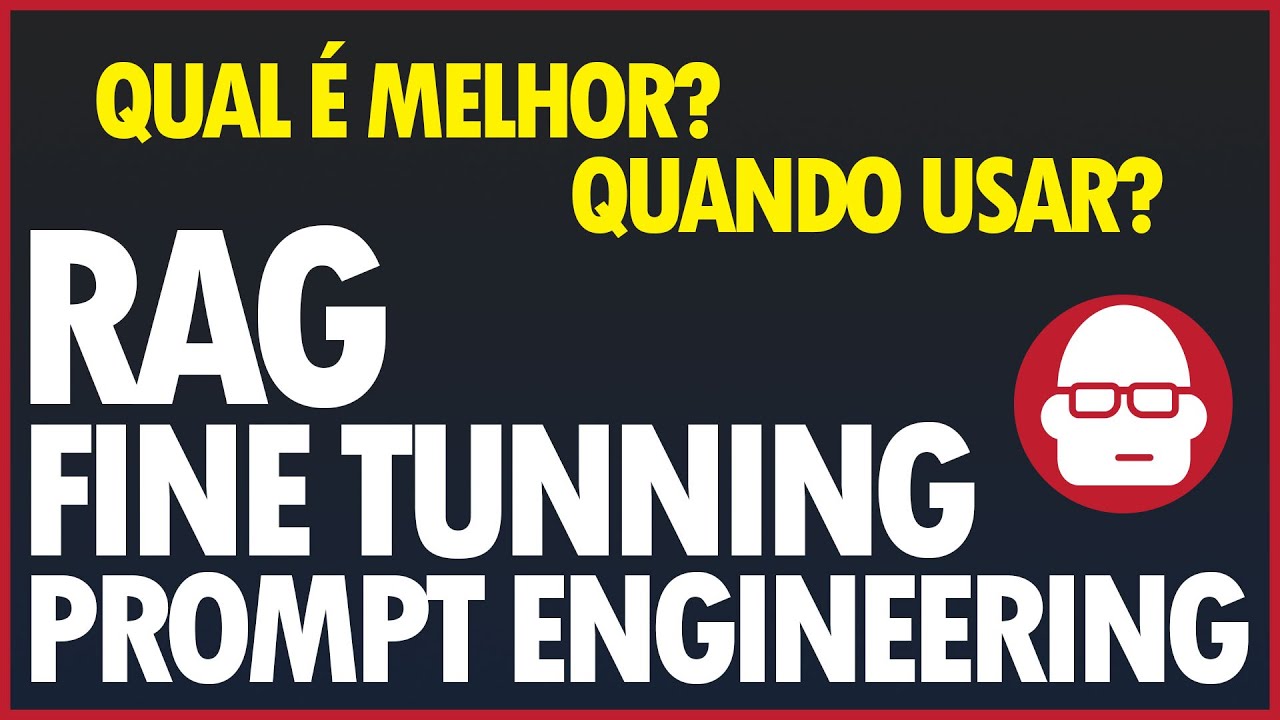
Fine Tuning, RAG e Prompt Engineering: Qual é melhor? e Quando Usar?
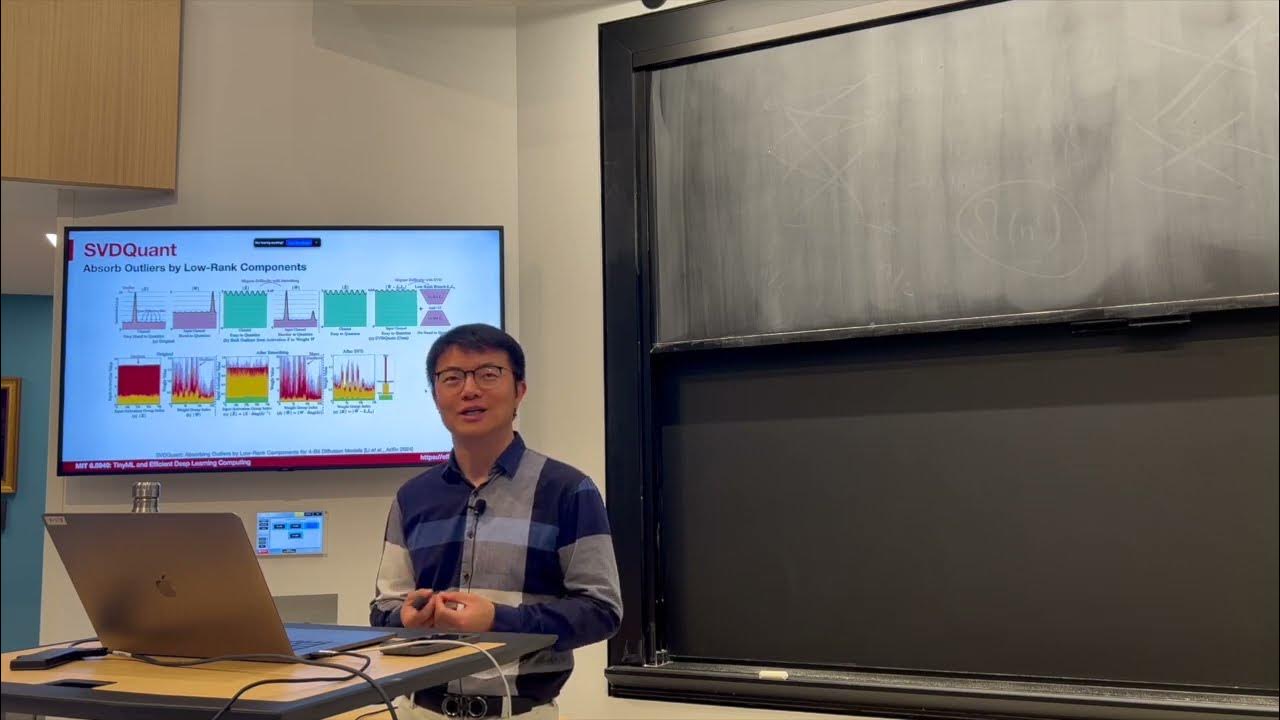
EfficientML.ai 2024 | Introduction to SVDQuant for 4-bit Diffusion Models
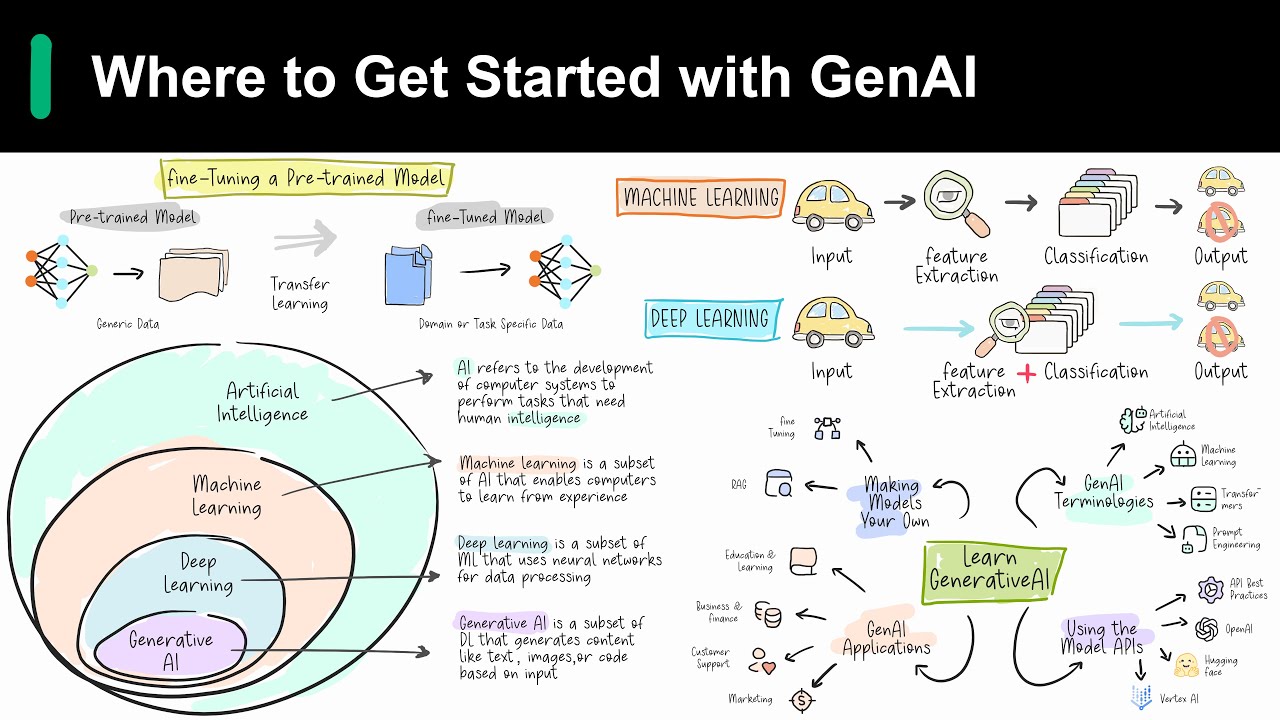
Introduction to Generative AI
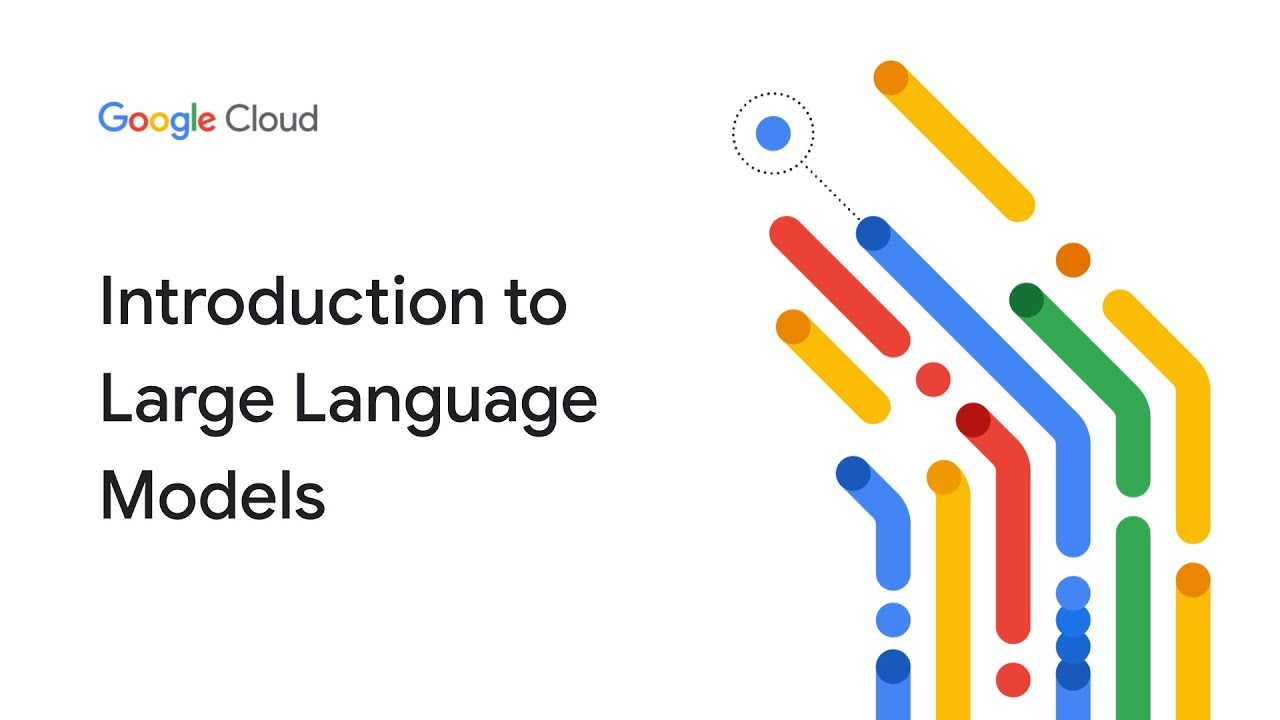
Introduction to large language models

A basic introduction to LLM | Ideas behind ChatGPT
5.0 / 5 (0 votes)