Interpreting Output for Multiple Regression in SPSS
Summary
TLDRIn this informative video, Dr. Grande teaches viewers how to interpret multiple regression output using SPSS. With a fictitious dataset involving career limitations and work experience as predictors, and days until employment as the outcome, the video guides through the process of conducting a regression analysis. It explains the significance of R-square, ANOVA, and p-values, and how to understand the impact of each predictor on the outcome. The video concludes with a clear explanation of the confidence intervals for the coefficients, offering a helpful guide for those new to regression analysis.
Takeaways
- đšââïž Dr. Grande introduces a video tutorial on interpreting multiple regression output in SPSS.
- đ The video uses fictitious data with four variables: an ID variable, two predictor variables, and one outcome variable.
- đ The predictor variables are 'career limitations', an index reflecting potential barriers to employment, and 'experience', measured in years of work.
- đ The outcome variable is 'days until employed', tracking the time it takes for participants to find work post-training.
- â Hypotheses suggest that higher career limitations may increase unemployment duration, while more experience may decrease it.
- đ The video emphasizes checking assumptions before running multiple regression to ensure data suitability for the analysis.
- đ The SPSS process involves selecting 'Analyze', 'Regression', and 'Linear', with 'days until employed' as the dependent variable.
- đ The model includes career limitations and experience as independent variables, with options for statistics like R-squared change and confidence intervals.
- đ The model summary reveals an adjusted R-square of 0.237, indicating that 23.7% of variance in employment time is explained by the predictors.
- đ The ANOVA table shows a statistically significant result (p < .05), validating the model's predictive power.
- đ The coefficients table provides unstandardized and standardized coefficients, with p-values indicating the statistical significance of each predictor.
- đ Career limitations have a positive impact on unemployment duration, with each unit increase associated with 2.658 more days until employed.
- đ Experience has a negative impact, with each additional year reducing the days until employed by approximately 4 days.
- đ Standardized coefficients indicate the effect size in terms of standard deviations, with career limitations increasing days until employed by 0.233 and experience decreasing it by 0.436.
- đ Confidence intervals provide a range within which the true effect of each predictor is likely to fall, offering a measure of precision for the estimates.
- đ€ The video concludes by encouraging viewers to reach out with questions, emphasizing support for further understanding.
Q & A
What is the purpose of the video by Dr. Grande?
-The purpose of the video is to explain how to interpret the output from a multiple regression analysis using SPSS.
What are the four variables mentioned in the SPSS data editor in the video?
-The four variables mentioned are an ID variable, two predictor variables (career limitations and experience), and one outcome variable (days until employed).
What does the career limitations variable represent in the study?
-The career limitations variable represents an index of potential barriers to employment, such as criminal history, low educational level, active substance use disorder, etc., with higher values indicating more severe limitations.
How is the experience variable measured in the study?
-The experience variable is measured in years, representing the number of years a participant had either a full-time or part-time job.
What is the maximum number of days considered for the 'days until employed' variable?
-The maximum number of days considered for the 'days until employed' variable is 365 days.
What are the hypotheses regarding the relationship between the predictor variables and the outcome variable?
-The hypotheses are that a higher number on the career limitations variable would be associated with a longer time to become employed, and more experience would be associated with fewer days until employment.
What does the adjusted R-square value of 0.237 indicate about the model in the video?
-An adjusted R-square of 0.237 indicates that 23.7% of the variance in the outcome variable (days until employed) is explained by the independent variables (career limitations and experience).
What does a statistically significant p-value in the ANOVA table suggest?
-A statistically significant p-value (less than 0.05) in the ANOVA table suggests that the overall model is significant and that at least one predictor variable has a statistically significant effect on the outcome variable.
What is the interpretation of the unstandardized coefficient for career limitations being 2.658?
-The unstandardized coefficient of 2.658 for career limitations means that for every one-unit increase in the career limitations index, there is an expected increase of 2.658 days until employed.
How does the experience variable affect the outcome variable according to the unstandardized coefficients?
-The unstandardized coefficient for experience is -4.044, indicating that for every additional year of experience, the number of days until employed decreases by approximately 4 days.
What do the standardized coefficients tell us about the effect of each variable in terms of standard deviations?
-The standardized coefficients indicate that for every one standard deviation increase in career limitations, the dependent variable increases by 0.233 standard deviations, and for every one standard deviation increase in experience, the dependent variable decreases by 0.436 standard deviations.
What is the purpose of the confidence intervals provided for the unstandardized coefficients?
-The confidence intervals provide a range within which we can be 95% confident that the actual value of the unstandardized coefficient lies, indicating the precision of the estimates.
Outlines
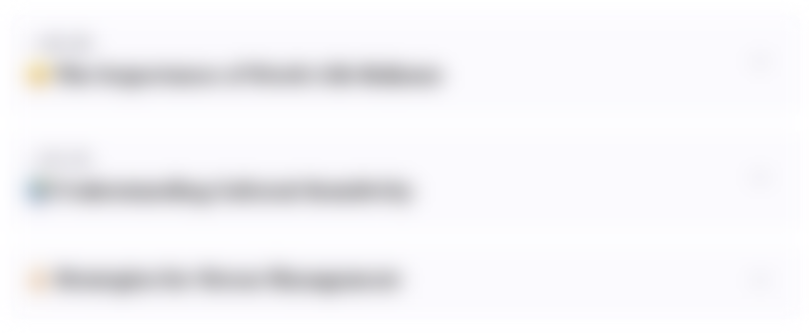
Dieser Bereich ist nur fĂŒr Premium-Benutzer verfĂŒgbar. Bitte fĂŒhren Sie ein Upgrade durch, um auf diesen Abschnitt zuzugreifen.
Upgrade durchfĂŒhrenMindmap
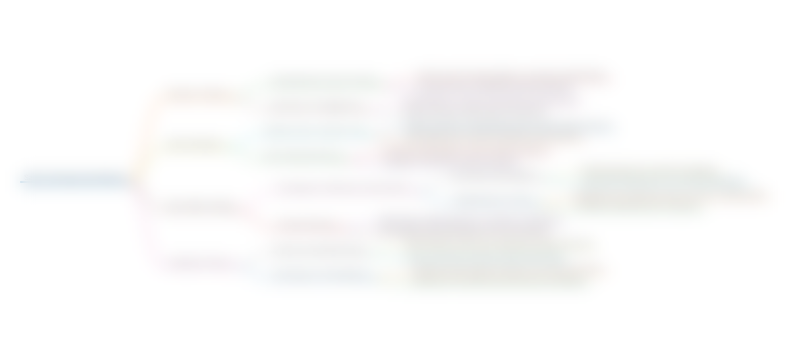
Dieser Bereich ist nur fĂŒr Premium-Benutzer verfĂŒgbar. Bitte fĂŒhren Sie ein Upgrade durch, um auf diesen Abschnitt zuzugreifen.
Upgrade durchfĂŒhrenKeywords
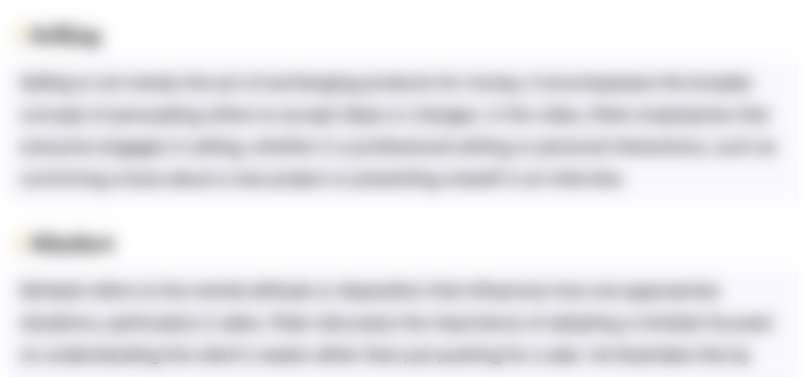
Dieser Bereich ist nur fĂŒr Premium-Benutzer verfĂŒgbar. Bitte fĂŒhren Sie ein Upgrade durch, um auf diesen Abschnitt zuzugreifen.
Upgrade durchfĂŒhrenHighlights
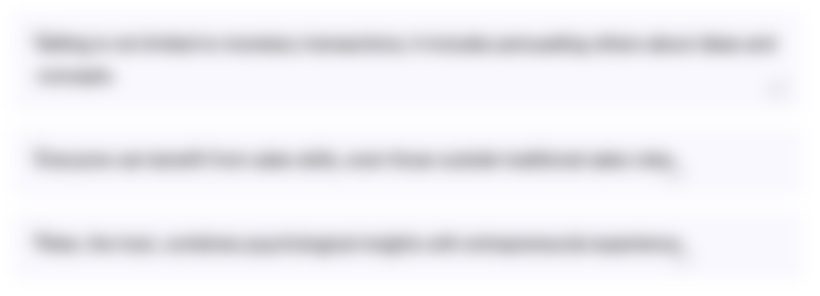
Dieser Bereich ist nur fĂŒr Premium-Benutzer verfĂŒgbar. Bitte fĂŒhren Sie ein Upgrade durch, um auf diesen Abschnitt zuzugreifen.
Upgrade durchfĂŒhrenTranscripts
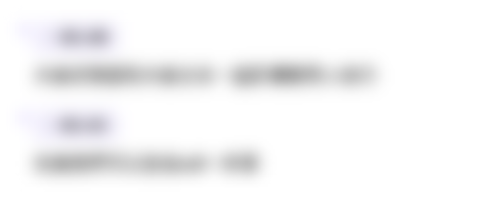
Dieser Bereich ist nur fĂŒr Premium-Benutzer verfĂŒgbar. Bitte fĂŒhren Sie ein Upgrade durch, um auf diesen Abschnitt zuzugreifen.
Upgrade durchfĂŒhrenWeitere Ă€hnliche Videos ansehen
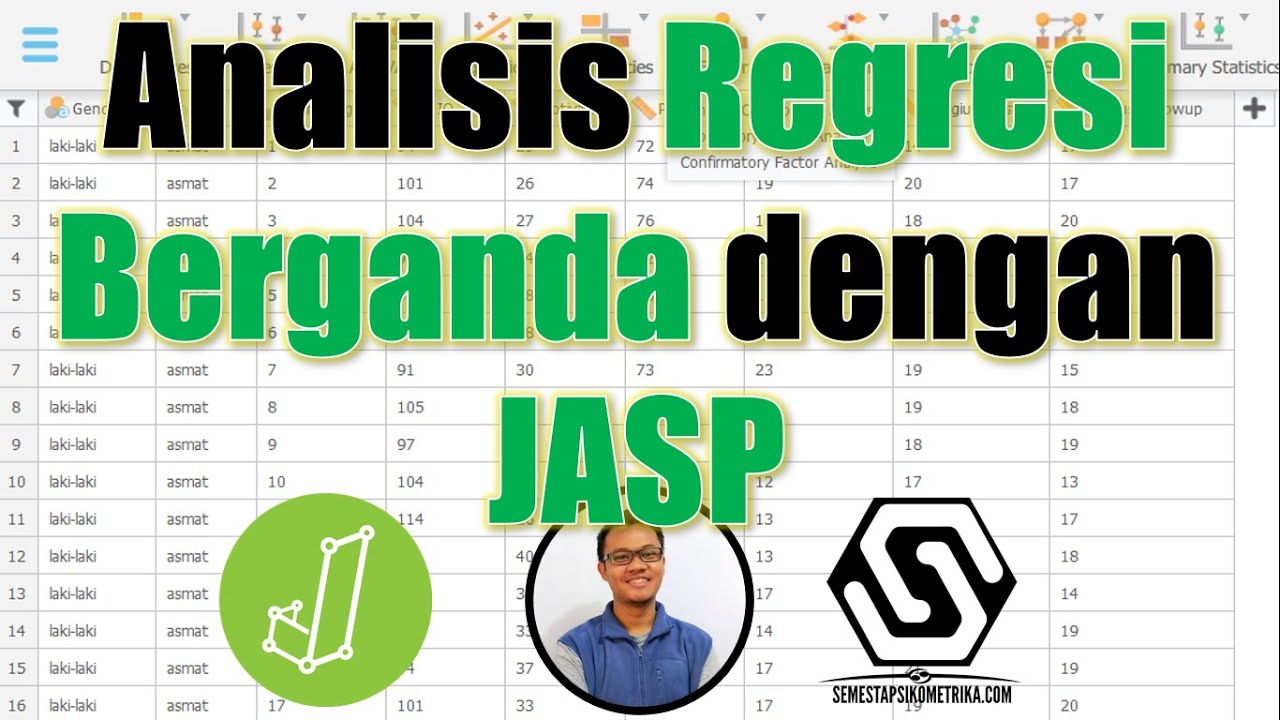
Cara Analisis Regresi Berganda dengan JASP
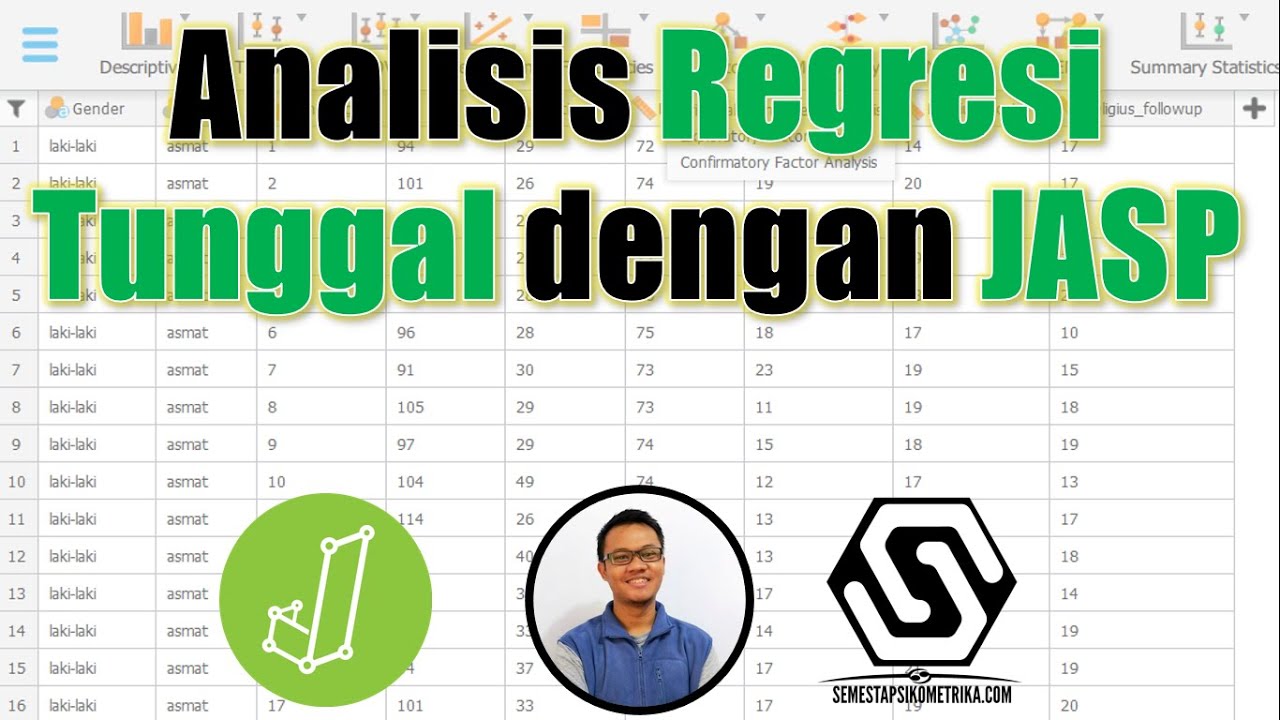
Tutorial Analisis Regresi Tunggal dengan JASP
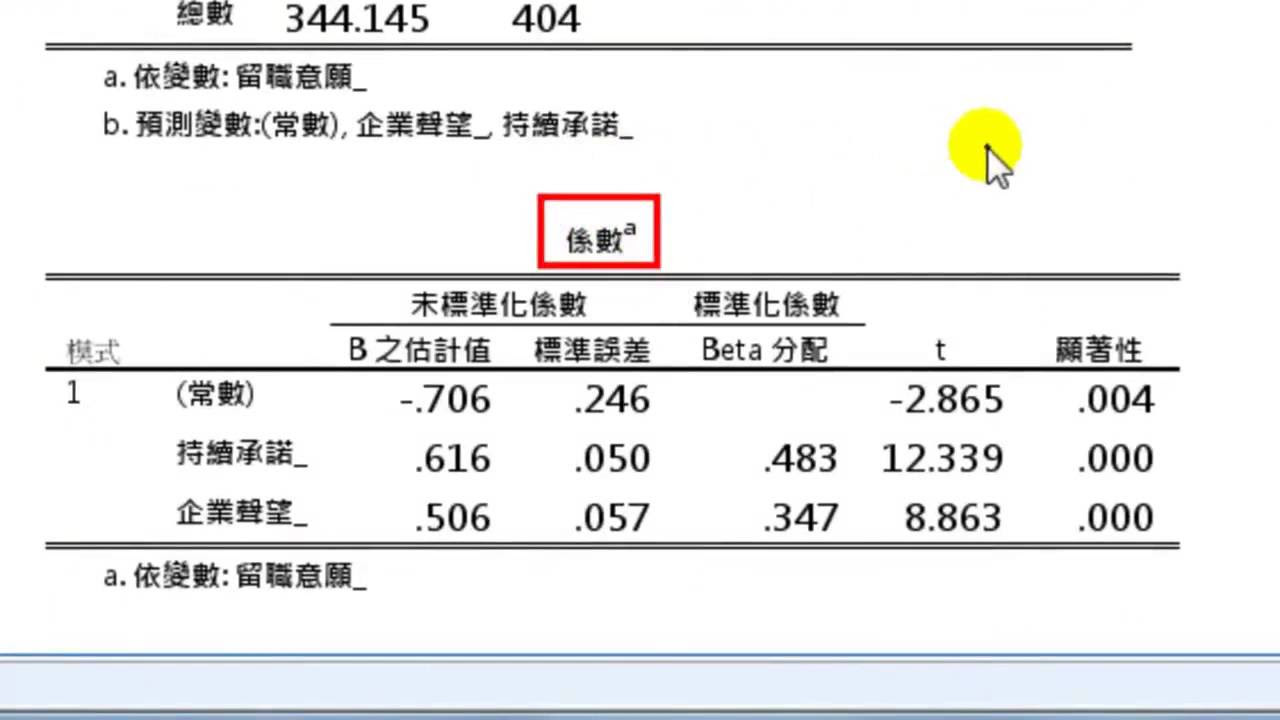
äžć€ăç”±èšćžïŒèżŽæžćæ

Excel Regression Analysis through the Toolpak
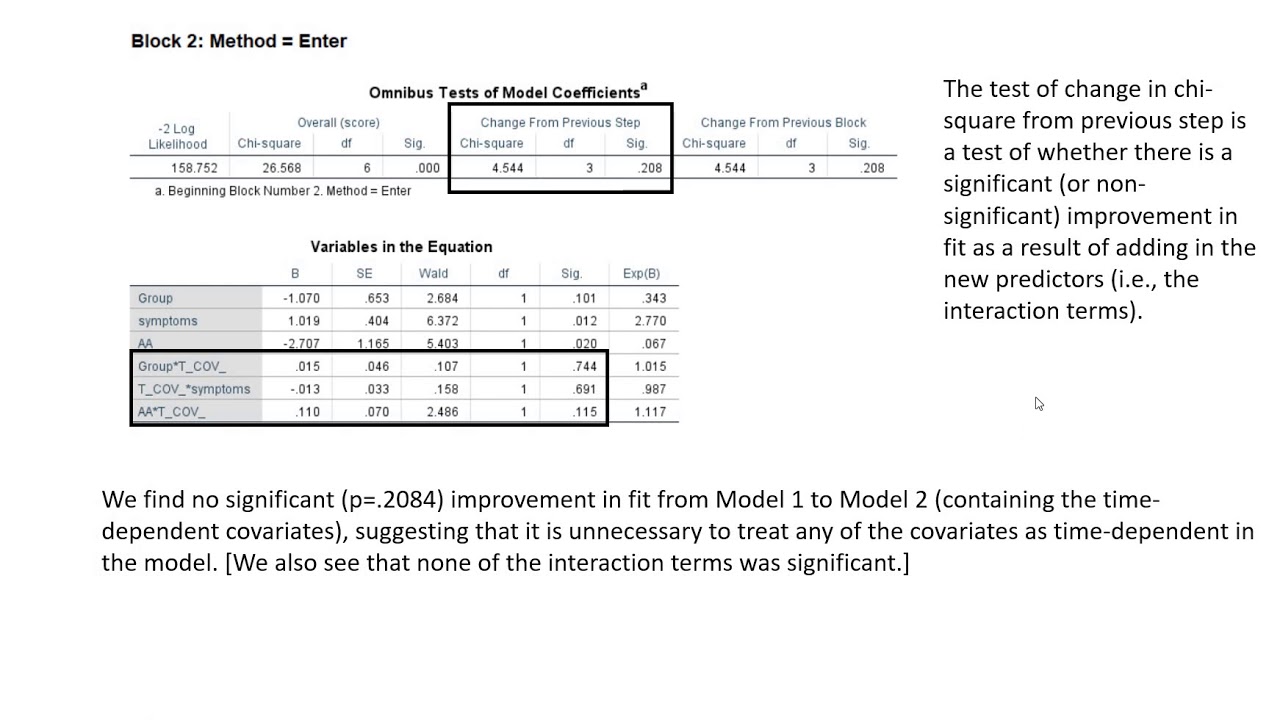
Survival analysis using Cox regression SPSS demo (new, July 2019)
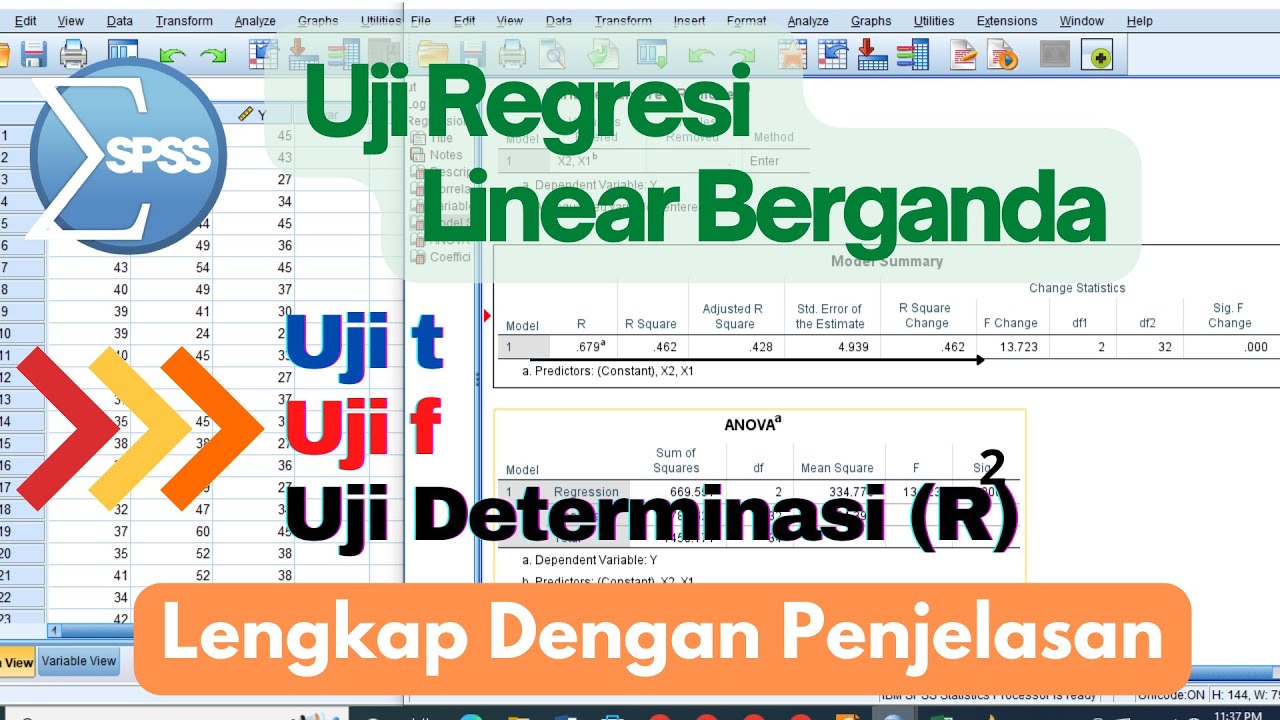
Cara Uji Regresi Linear Berganda ( Uji t, Uji f dan Uji Determinasi) menggunakan aplikasi SPSS
5.0 / 5 (0 votes)