Watch how a Pro develops AI Agents in real-time
Summary
TLDRDieses Skript demonstriert die Erstellung eines AI-Agents, der Webinhalte extrahiert und in eine Tabelle formatiert. Es wird die 'agent Ops'-Plattform verwendet, um die Agenten-Performance zu überwachen und zu analysieren. Durch den Einsatz von 'fir crawl' für das Scraping und OpenAI für die Textsummarisierung werden die Schritte des Prozesses veranschaulicht. Zusätzlich wird gezeigt, wie man Fehler mit der Agent-Dashboard-Funktion diagnostiziert und wie man die Kosten- und Leistungsanalyse von verschiedenen Agenten-Laufzeiten einblendet.
Takeaways
- 🤖 Die Diskussion umfasst das Erstellen eines AI-Agents, der Webinhalte extrahieren und in Tabellenform präsentieren kann.
- 🔍 Der Agent soll in der Lage sein, Websites zu crawlen und Informationen zu sammeln, indem er die Funktion 'crawl web' nutzt.
- 📝 Die Zusammenfassung der Webinhalte erfolgt durch die 'summarize text'-Funktion, die noch zu implementieren ist.
- 🛠 Für das Web-Crawling wird das Open-Source-Framework 'fir crawl' verwendet, das Markdown-Texte liefert.
- 🔑 Es wird auf die Verwendung von API-Schlüsseln und Anforderungsbibliotheken wie 'requests' für die Kommunikation mit dem 'fir crawl' hingewiesen.
- 🔄 Die Umgebungsvariablen müssen geladen werden, um sicherzustellen, dass sie im Python-Skript zur Verfügung stehen, wenn es ausgeführt wird.
- 📊 Der Agent 'Ops' bietet Dashboards an, die es ermöglichen, den Ablauf und die Kosten von AI-Agents zu verfolgen und zu analysieren.
- 🔎 Agent 'Ops' kann die Interaktionen und Kosten von verschiedenen großen Sprachmodellen (LLMs) verfolgen und analysieren.
- 🚀 Durch den Einsatz von 'Agent Ops' können Entwickler ihre AI-Agents überwachen, was zu einer verbesserten Zuverlässigkeit und Leistung bei der Problembehandlung führt.
- 💡 Der Einsatz von 'Agent Ops' ermöglicht es, Fehler schnell zu identifizieren und zu beheben, indem man die Schritte und Entscheidungen der Agents im Dashboard nachvollziehen kann.
- 🔗 'Agent Ops' ist in verschiedenen Agenten-Frameworks integriert und kann leicht in bestehende Projekte einbezogen werden.
Q & A
Was ist das Hauptziel des in der Diskussion beschriebenen Projekts?
-Das Hauptziel des Projekts ist es, einen AI-Agenten zu erstellen, der Webseiten crawlen und Informationen sammeln kann, um sie dann in einer Tabelle zu formatieren.
Welche Funktionen soll der AI-Agent haben?
-Der AI-Agent soll zwei Hauptfunktionen haben: Erstens soll er in der Lage sein, Inhalte von Webseiten zu crawlen, und zweitens soll er diese Inhalte in einer übersichtlichen Tabelle zusammenfassen.
Welches Tool wird für das Webcrawling verwendet?
-Für das Webcrawling wird das Tool 'Fir Crawl' verwendet, das Open Source ist und eine einfache Möglichkeit bietet, Inhalte von Webseiten zu sammeln.
Was ist 'Augment' und wie wird es in diesem Projekt eingesetzt?
-Augment ist ein alternativer Code-Assistent, vergleichbar mit Co-Pilot, der in diesem Projekt verwendet wird, um den Code-Schreibprozess zu beschleunigen und zu verbessern.
Wie wird die Zusammenfassung der Webinhalte erstellt?
-Die Zusammenfassung der Webinhalte wird durch einen separaten Funktionsaufruf namens 'summarize text' erstellt, der die gesammelten Daten in einer übersichtlichen Form präsentiert.
Welche Rolle spielt die Agent-Ops-Plattform in diesem Projekt?
-Die Agent-Ops-Plattform dient zur Überwachung und Analyse der Aktivitäten des AI-Agents, einschließlich Kosten-, Latenz- und Erfolgsverfolgung der durchgeführten Anfragen.
Wie wird sichergestellt, dass die Umgebungsvariablen im Python-Skript geladen werden?
-Um sicherzustellen, dass die Umgebungsvariablen geladen werden, wird die Funktion 'load.env' aufgerufen, die die Umgebungsvariablen in das Python-Skript einbindet.
Welche Bedeutung haben die Dekoratoren in der Agent-Ops-Funktion?
-Die Dekoratoren in der Agent-Ops-Funktion, wie z.B. 'agent_ops.record_function', dienen dazu, die von den Funktionen durchgeführten Aktionen zu verfolgen und im Dashboard nachzuvollziehen.
Was ist der Zweck des OpenAI-Clients in der 'summarize text'-Funktion?
-Der OpenAI-Client in der 'summarize text'-Funktion wird verwendet, um die gesammelten Webinhalte an einen Large Language Model (LLM) zu senden und eine Zusammenfassung zu erhalten.
Wie wird die Leistung der Agents im Laufe der Zeit gemessen und visualisiert?
-Die Leistung der Agents wird durch das Agent-Ops-Dashboard gemessen und visualisiert, das Informationen über die Kosten, die Dauer und den Erfolg der durchgeführten Aktionen anzeigt.
Was sind die Vorteile des Agent-Ops-Dashboards bei der Fehlerbehebung und -analyse?
-Das Agent-Ops-Dashboard ermöglicht eine detaillierte Analyse von Fehlern und die Überwachung der Agentenleistung, indem es eine zeitliche Aufschlüsselung der Ereignisse und eine Visualisierung der Aktionen bietet.
Outlines
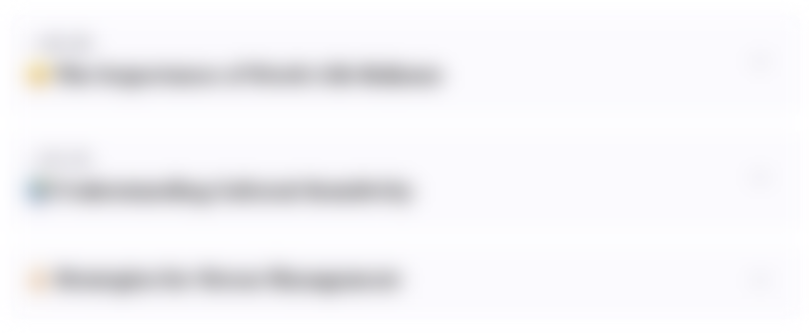
Dieser Bereich ist nur für Premium-Benutzer verfügbar. Bitte führen Sie ein Upgrade durch, um auf diesen Abschnitt zuzugreifen.
Upgrade durchführenMindmap
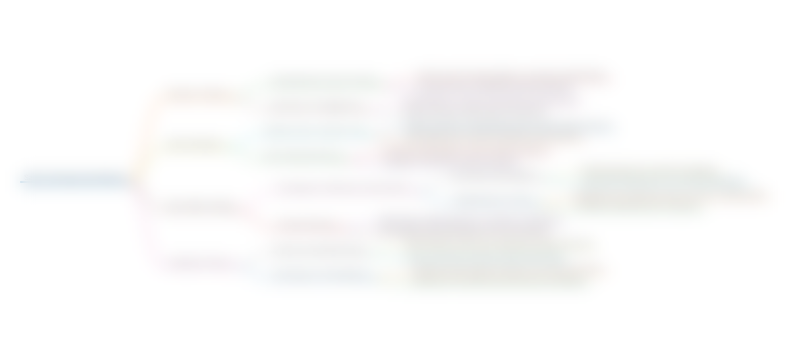
Dieser Bereich ist nur für Premium-Benutzer verfügbar. Bitte führen Sie ein Upgrade durch, um auf diesen Abschnitt zuzugreifen.
Upgrade durchführenKeywords
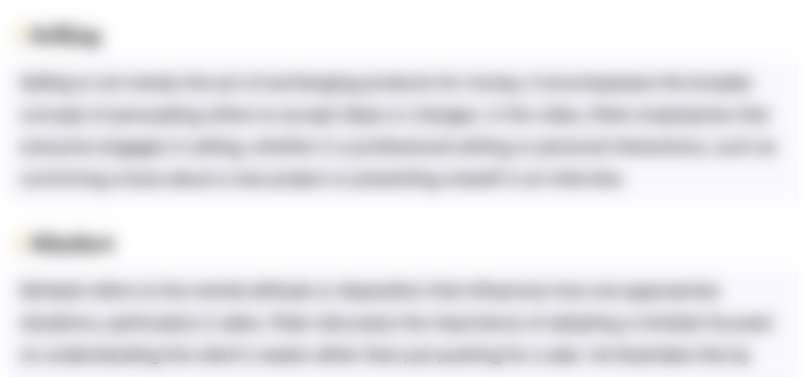
Dieser Bereich ist nur für Premium-Benutzer verfügbar. Bitte führen Sie ein Upgrade durch, um auf diesen Abschnitt zuzugreifen.
Upgrade durchführenHighlights
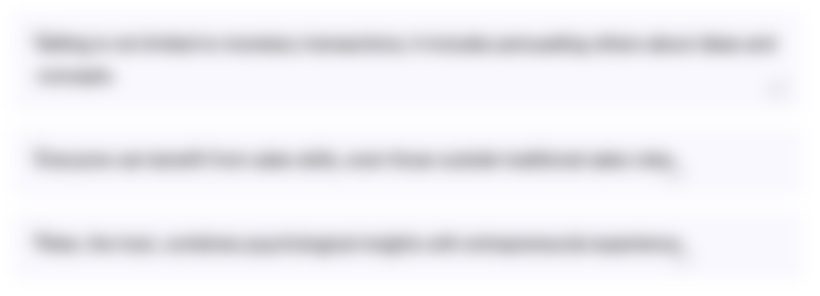
Dieser Bereich ist nur für Premium-Benutzer verfügbar. Bitte führen Sie ein Upgrade durch, um auf diesen Abschnitt zuzugreifen.
Upgrade durchführenTranscripts
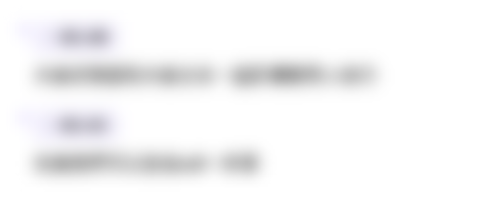
Dieser Bereich ist nur für Premium-Benutzer verfügbar. Bitte führen Sie ein Upgrade durch, um auf diesen Abschnitt zuzugreifen.
Upgrade durchführenWeitere ähnliche Videos ansehen
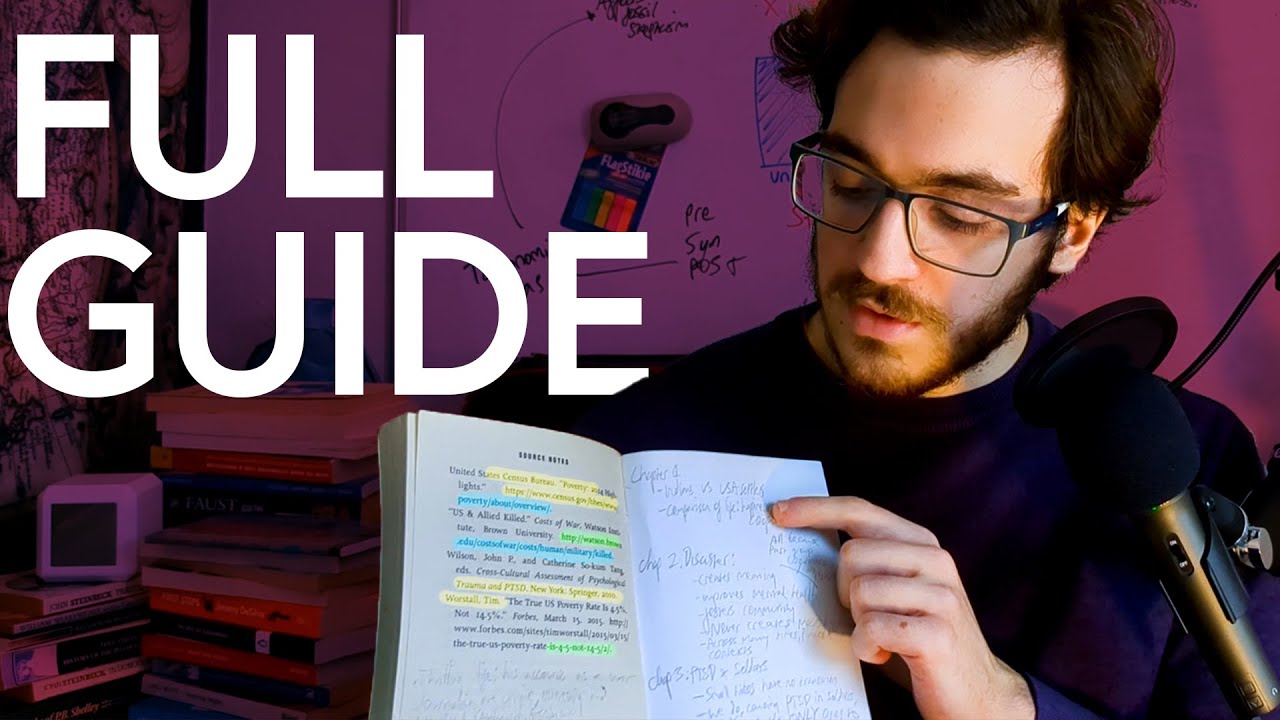
How to Analyze a Book - 101
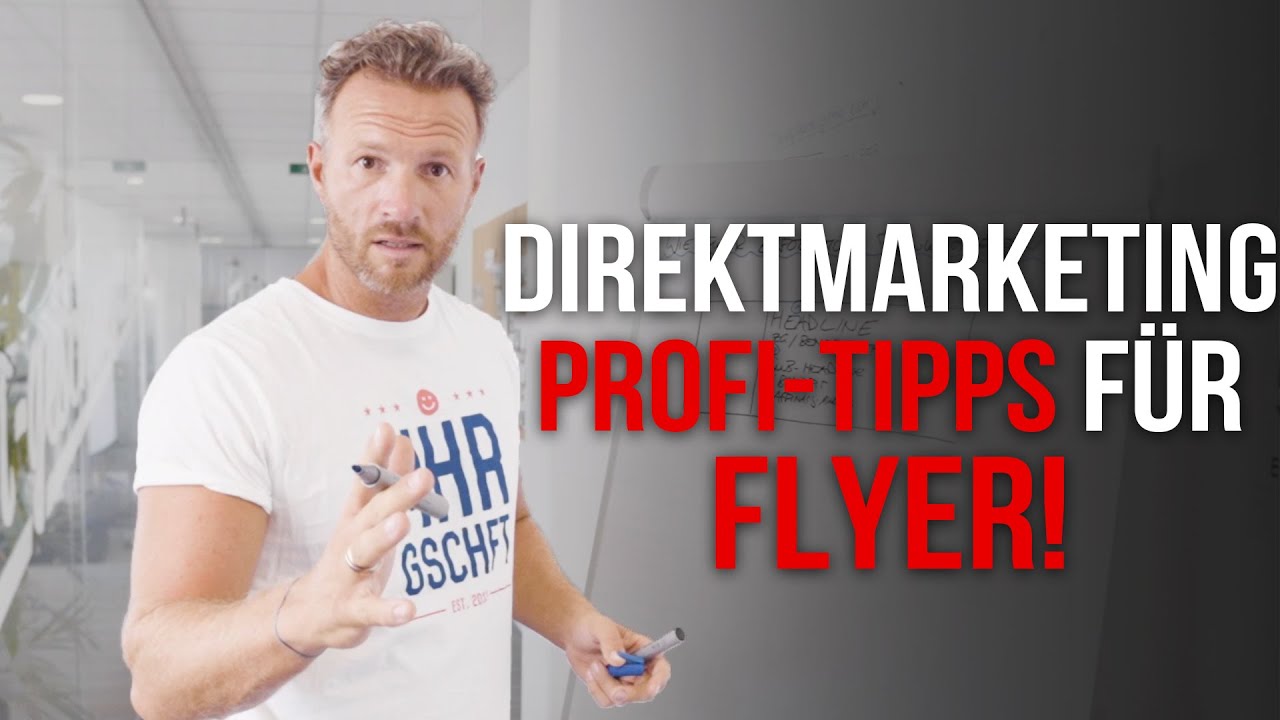
Mehr Geschäft - Wie du Flyer baust, die sofort funktionieren!
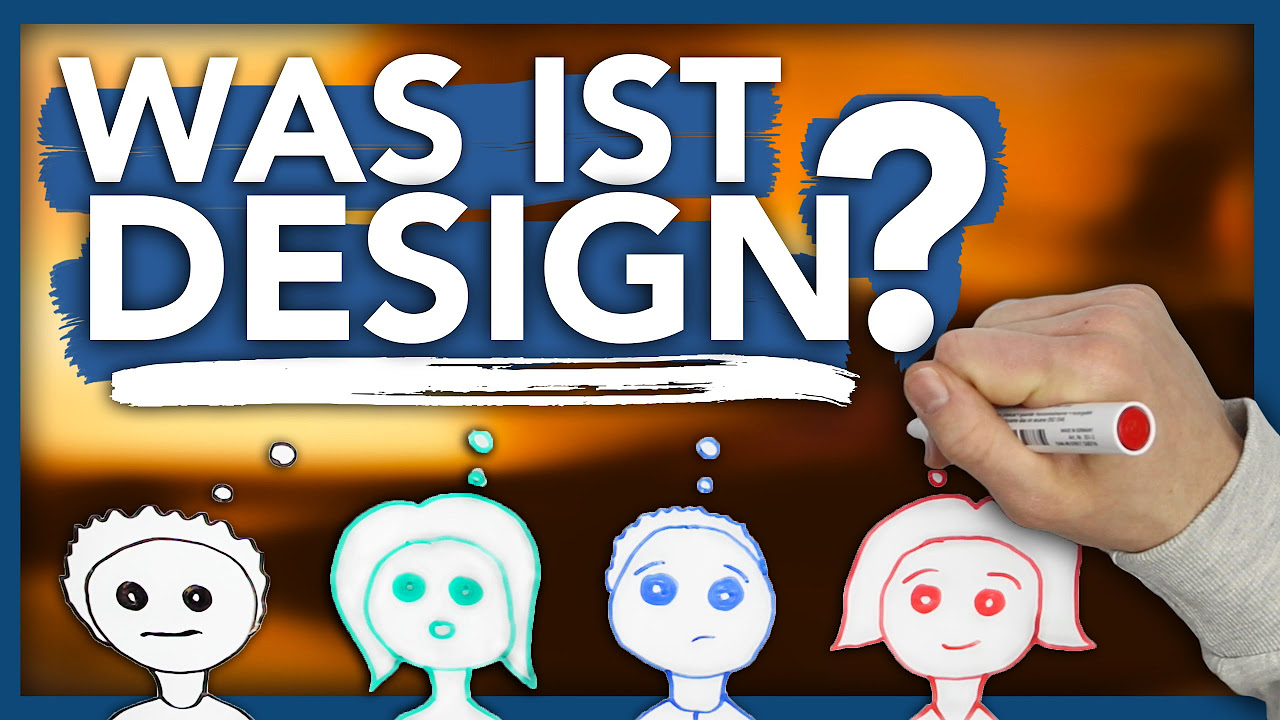
WAS IST DESIGN? - Einfach und verständlich erklärt!

Serienbrief Teil I in Word 2016 - einfach erklärt mit Beispiel
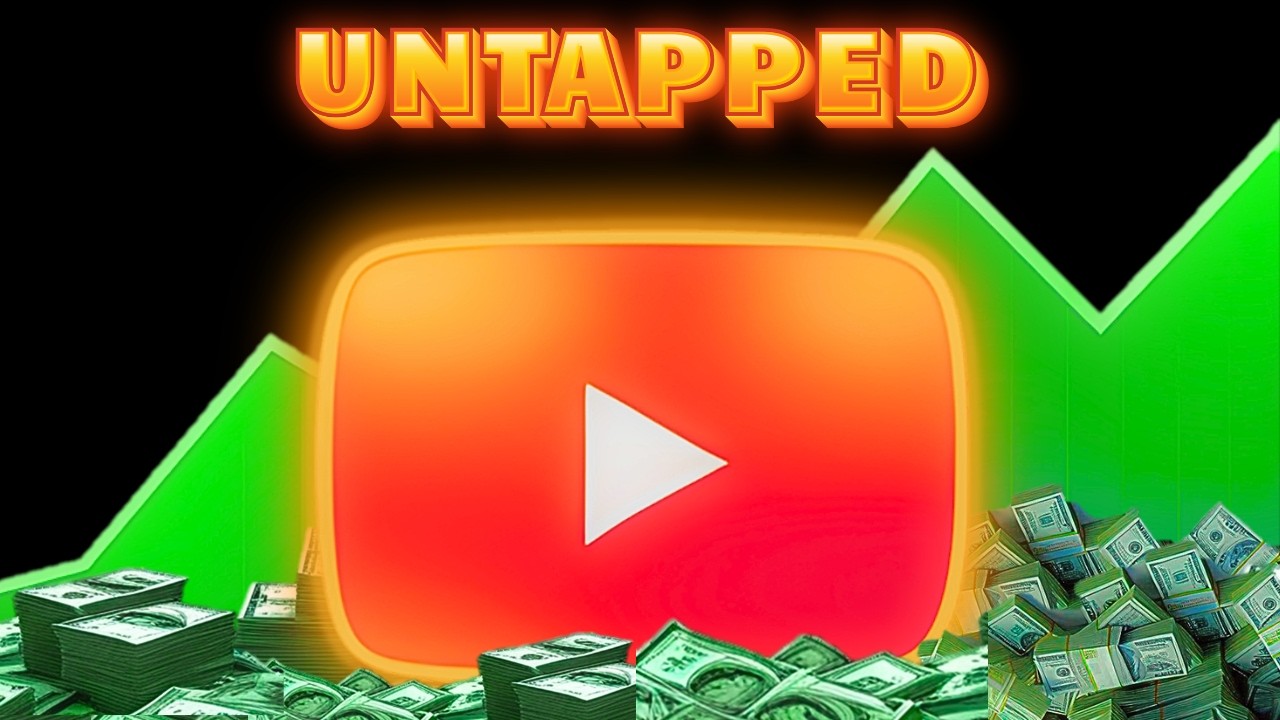
I Found a $10K+ Viral AI Niche & Created a Competing YouTube Channel in Minutes!
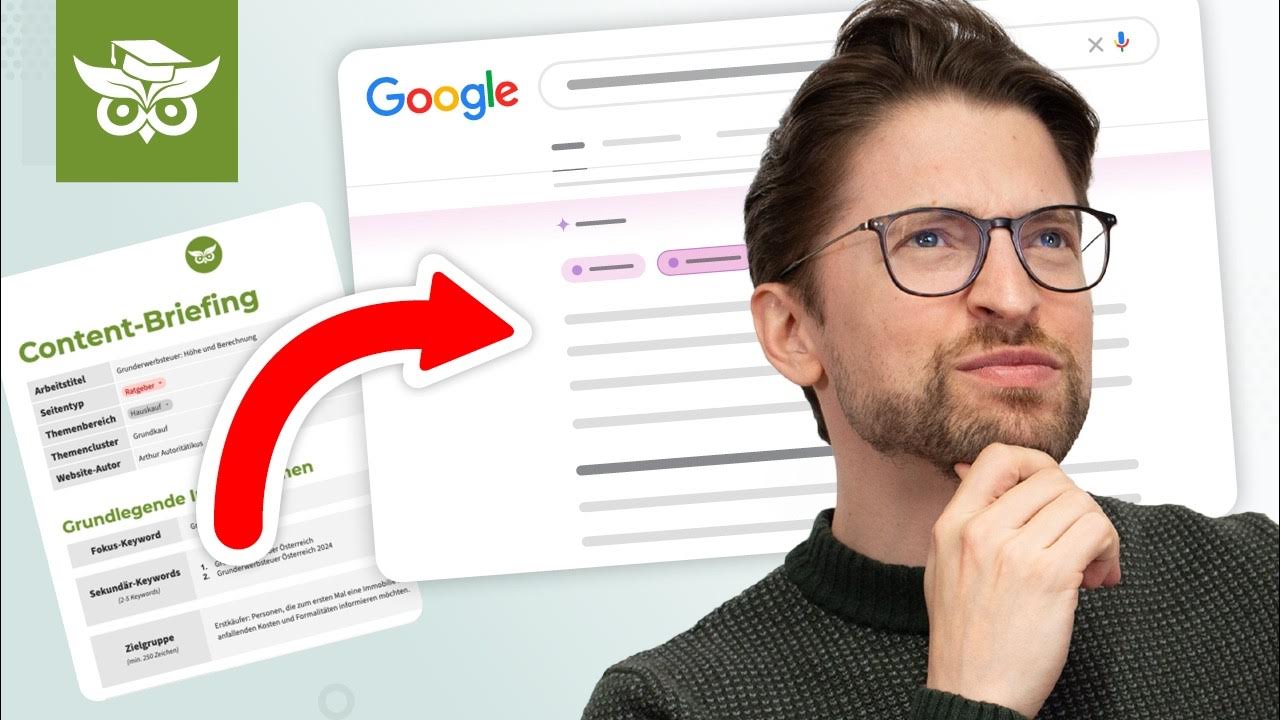
Content-Briefing inkl. Vorlage (AI Overviews ready)
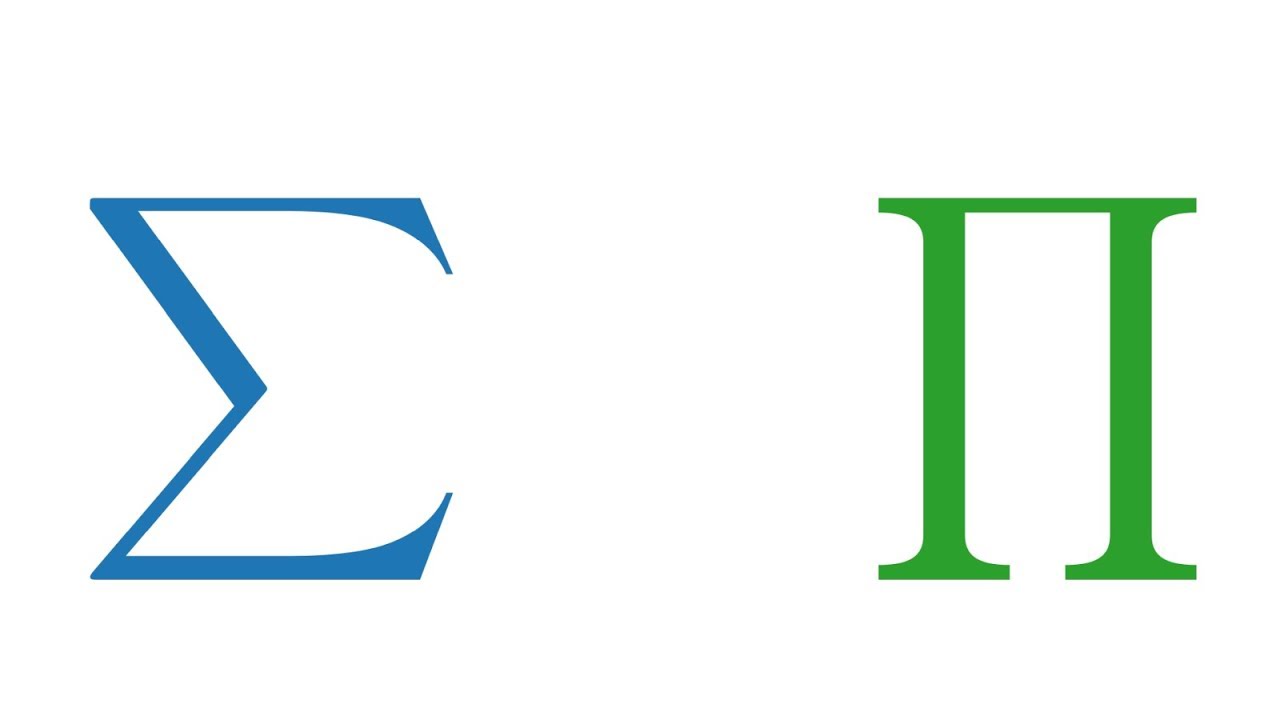
Rechnen mit Summen- und Produktzeichen
5.0 / 5 (0 votes)