are we cooked w/ o3?
Summary
TLDRThe speaker discusses the latest GPT-3 model's performance on the ARC AGI Benchmark, which has led to claims of AGI nearing reality. While acknowledging GPT-3's impressive results, the speaker cautions against overhyped claims, pointing out the high computational costs and limitations of current AI models. The speaker emphasizes that despite AI's potential to solve certain tasks, it still requires significant human oversight. The future of AI, while promising, faces challenges in scaling and affordability. Ultimately, the speaker stresses the importance of technical depth and expertise in leveraging AI effectively.
Takeaways
- đ GPT-03 has outperformed previous AI models in the Arc AGI Benchmark, scoring 82% on the public dataset, showing significant progress towards AGI but not yet achieving full AGI.
- đ€ Despite impressive performance on tasks, GPT-03's ability to solve simple puzzles does not necessarily translate to solving more complex real-world problems like large-scale code debugging.
- đž The cost of running AI tasks, particularly with high compute, is prohibitively expensive for most individuals and businesses, making AI tools like GPT-03 impractical for everyday use.
- đ§ The high compute version of GPT-03 is 172 times more expensive than the low compute version, which limits its accessibility for most people and organizations.
- đ§ Technical depth and hard skills will remain crucial, as AI tools like GPT-03 cannot replace the need for deep understanding of systems and software development.
- đ AI is still in its early stages and, while promising, is far from revolutionizing work processes in the short term. It requires substantial advancements in efficiency and affordability to become widely useful.
- đ» AI's current limitations include both high computational costs and hardware bottlenecks, meaning that widespread adoption of models like GPT-03 is not feasible yet due to resource constraints.
- â ïž The hype around AGI and AI achievements is largely driven by investors and companies seeking to fundraise, rather than a genuine, immediate revolution in the field.
- đ AI is not a silver bullet for tasks like bug fixing or software development. It can assist, but it often requires manual oversight and can still produce errors, adding to the overall workload.
- âł Despite AI's potential, the rapid pace of change in technology will only amplify the value of individuals with technical expertise, who can leverage AI effectively while understanding the underlying systems.
Q & A
What is the ARC AGI test, and how did GPT-3 perform on it?
-The ARC AGI test is designed to evaluate AI models on logical puzzles to measure progress towards AGI (Artificial General Intelligence). GPT-3 (03) performed significantly better than its predecessors, with scores of 75% on private tasks and 82% on public tasks. It outperformed previous models, like GPT-1 and GPT-1 Mini, in terms of accuracy, showcasing its enhanced reasoning capabilities.
What does the 03 model's performance on the ARC AGI test suggest about its potential for AGI?
-While GPT-3's strong performance on the ARC AGI test is impressive, it's important to note that the test is a litmus test for AGI rather than definitive proof of achieving AGI. The results indicate significant progress but are not conclusive evidence of true AGI, as the test involves solving logical puzzles and not real-world, complex tasks.
What are the major concerns regarding the cost of using models like GPT-3 for practical tasks?
-The cost of using models like GPT-3 is a significant barrier to widespread adoption. On low compute, a task costs around $20 and takes 1.3 minutes, while high compute models could cost upwards of $140,000 for marginally improved accuracy. This makes such models impractical for everyday use, especially for large-scale, real-world problems.
How does the performance of GPT-3 on smaller tasks compare to its ability to solve more complex, real-world problems?
-GPT-3 excels at solving smaller, toy-like tasks, but its performance on larger, real-world problems, like bug fixes in large codebases, remains limited. The challenge lies in scaling the model to handle more intricate, context-rich problems that require deeper understanding and reasoning.
Why is the high compute option for GPT-3 considered impractical for most use cases?
-The high compute option for GPT-3 offers better accuracy, but it is 172 times more computationally expensive than low compute, making it prohibitively costly. For example, solving tasks with high compute could cost up to $140,000 for a slight improvement in accuracy, which is unsustainable for most applications.
What are the limitations of using AI for tasks like bug fixing in large codebases?
-AI can help identify small bugs in code, but when dealing with large codebases, the AI may struggle to understand the full context and fail to provide accurate fixes. Additionally, even when AI suggests fixes, human oversight is still needed to verify and adjust the solutions, reducing the time savings it might offer.
How does the cost of using AI models like Devon for code-related tasks compare to the value it provides?
-Using models like Devon for code-related tasks can be very expensive, with limited compute hours available for a high cost. At $500 per month for just 62.5 hours of compute, the cost may not justify the benefit, especially considering that the AI-generated code still requires significant human intervention to correct errors.
What is the key takeaway regarding the role of human technical skills in the age of AI?
-Despite the advancements in AI, human technical skills remain crucial. As AI tools become more integrated, the ability to understand and leverage them effectively will be more valuable than ever. Those with deep technical knowledge will be better equipped to navigate the rapid changes in technology, while those without it may struggle.
What is the speakerâs prediction about the future impact of AI on human workers and skills?
-The speaker predicts that as AI continues to evolve, the gap between those with deep technical expertise and those who rely on AI without understanding it will widen. Professionals who understand the technical depth of systems will become more valuable, while those without this knowledge may see their value diminish as AI takes over more tasks.
How does the speaker view the current hype around AGI and the claims of AI's capabilities?
-The speaker is skeptical about the current hype around AGI and believes much of it is driven by investor interests. While AI has made significant progress, the claims of achieving AGI or near AGI are likely exaggerated, serving more to attract funding than to reflect the actual capabilities of current AI models.
Outlines
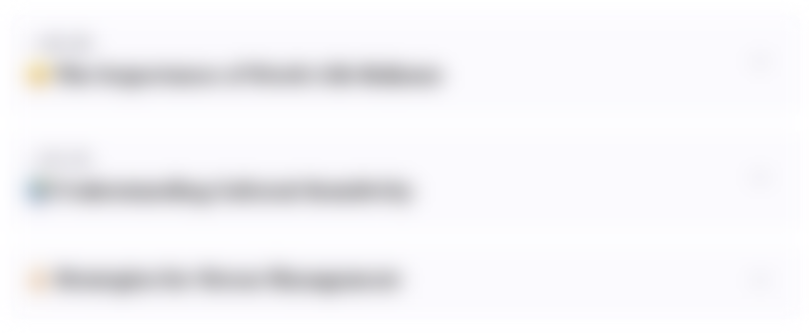
Dieser Bereich ist nur fĂŒr Premium-Benutzer verfĂŒgbar. Bitte fĂŒhren Sie ein Upgrade durch, um auf diesen Abschnitt zuzugreifen.
Upgrade durchfĂŒhrenMindmap
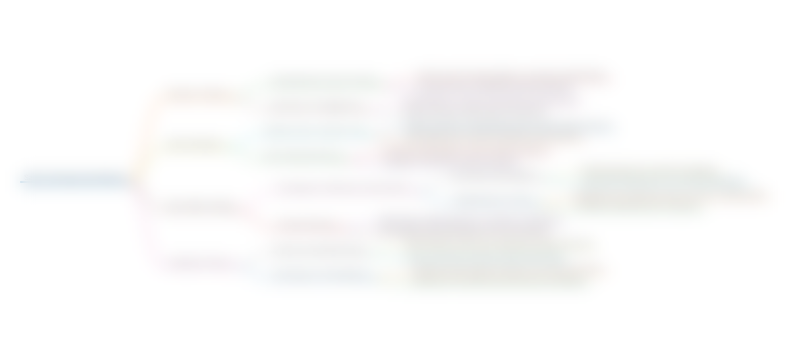
Dieser Bereich ist nur fĂŒr Premium-Benutzer verfĂŒgbar. Bitte fĂŒhren Sie ein Upgrade durch, um auf diesen Abschnitt zuzugreifen.
Upgrade durchfĂŒhrenKeywords
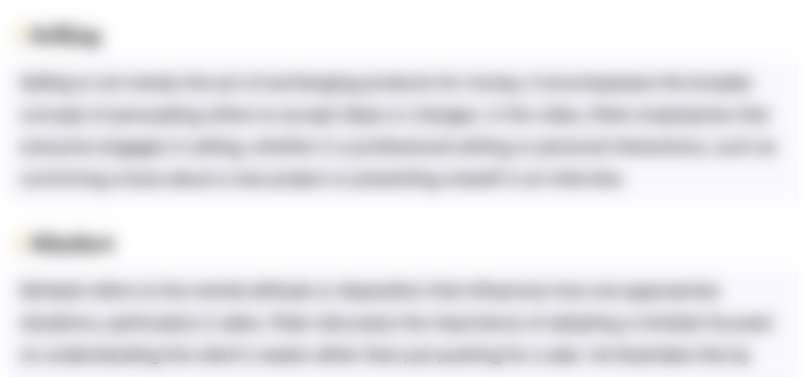
Dieser Bereich ist nur fĂŒr Premium-Benutzer verfĂŒgbar. Bitte fĂŒhren Sie ein Upgrade durch, um auf diesen Abschnitt zuzugreifen.
Upgrade durchfĂŒhrenHighlights
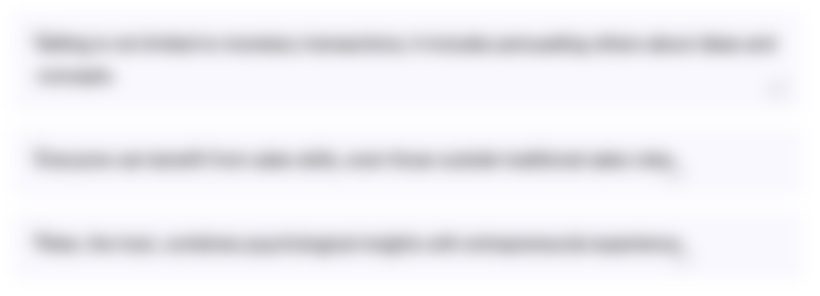
Dieser Bereich ist nur fĂŒr Premium-Benutzer verfĂŒgbar. Bitte fĂŒhren Sie ein Upgrade durch, um auf diesen Abschnitt zuzugreifen.
Upgrade durchfĂŒhrenTranscripts
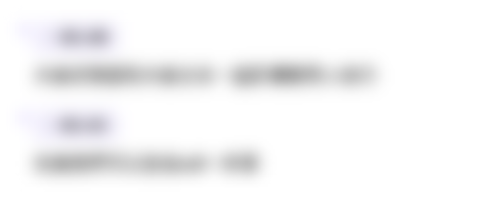
Dieser Bereich ist nur fĂŒr Premium-Benutzer verfĂŒgbar. Bitte fĂŒhren Sie ein Upgrade durch, um auf diesen Abschnitt zuzugreifen.
Upgrade durchfĂŒhrenWeitere Ă€hnliche Videos ansehen
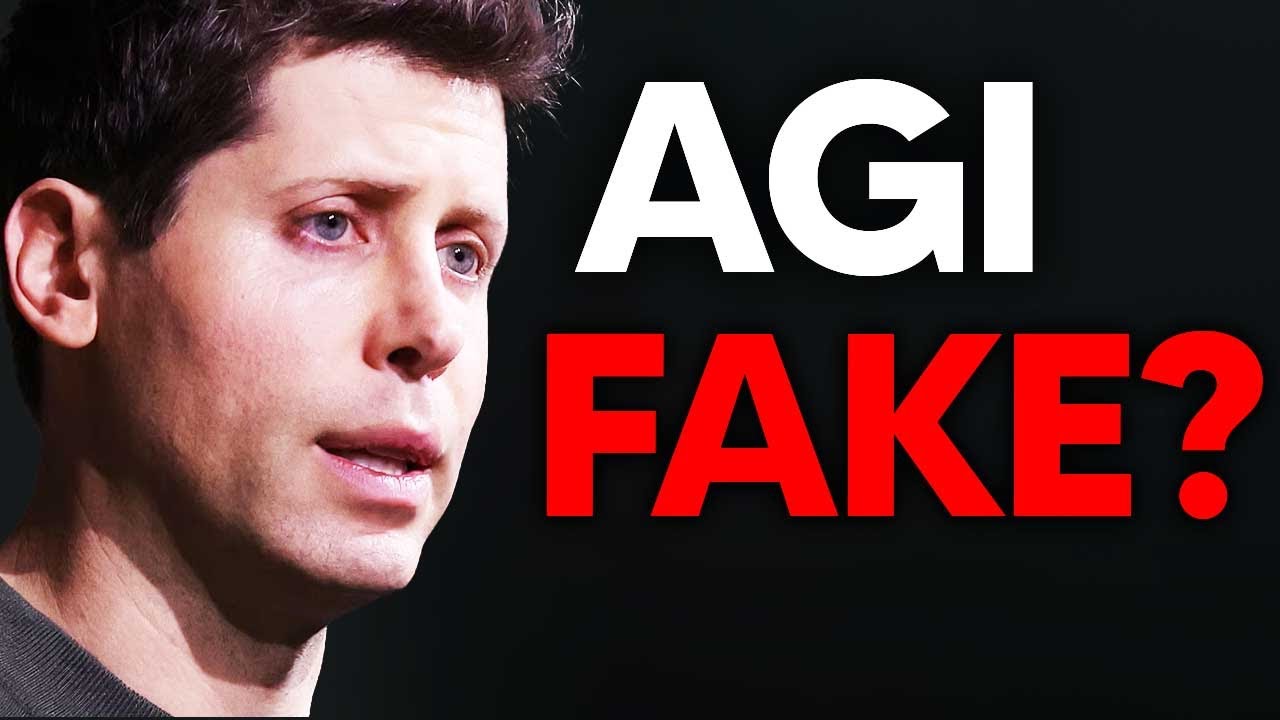
Did OpenAI FAKE AGI ? (Controversy Explained)
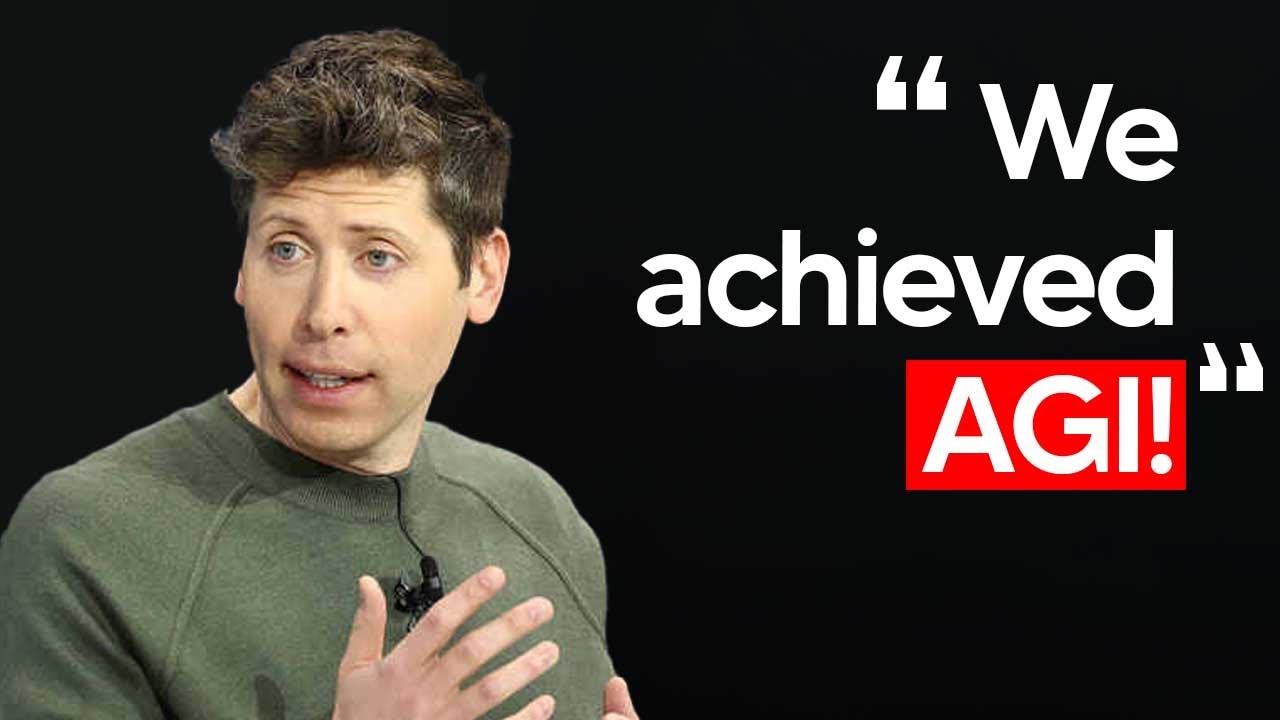
OpenAI Just Revealed They ACHIEVED AGI (OpenAI o3 Explained)
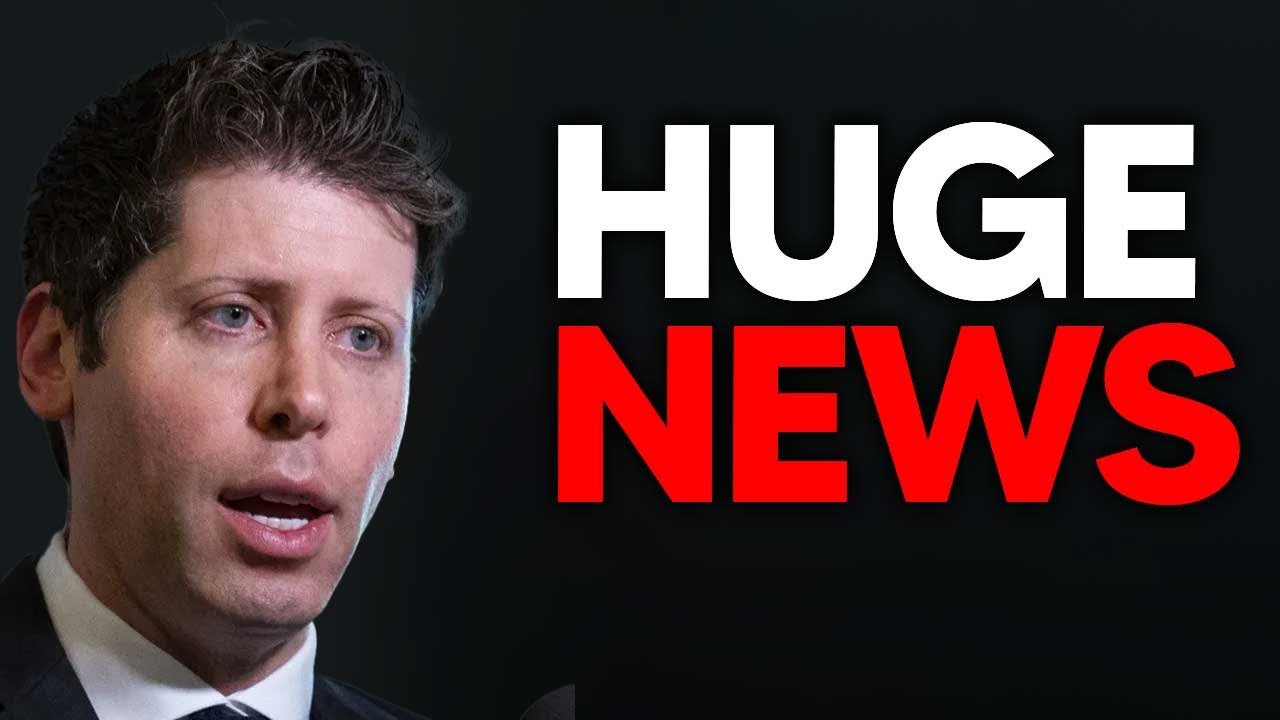
HUGE AI NEWS: AGI Benchmark BROKEN ,OpenAIs Agents Leaked , Automated AI Research And More
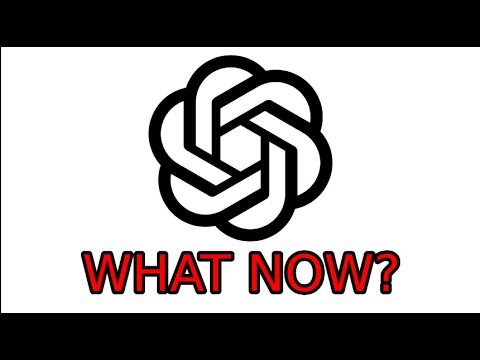
AI is about to get way worse (AGI in development)

BOMBA: Elon Musk ha ragione, GPT-4 E' UNA AGI. (Guai seri per Microsoft?)
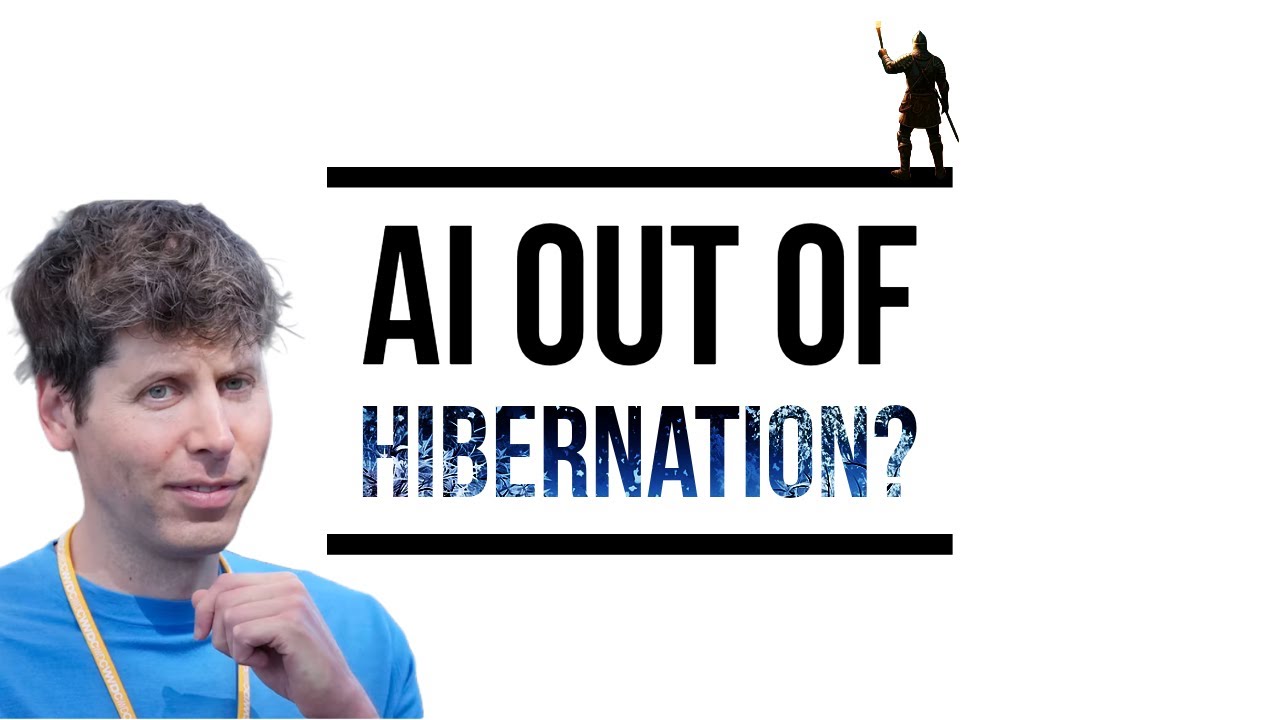
AI Breaks Its Silence: OpenAIâs âNext 12 Daysâ, Genie 2, and a Word of Caution
5.0 / 5 (0 votes)