Binomial Distribution
Summary
TLDRThis video introduces the binomial distribution, focusing on its key characteristics, including binary outcomes, independent trials, a fixed number of events, and constant probability of success. It explains how these elements are encapsulated in the acronym BINS. Using practical examples, such as tossing a fair coin and rolling a die, the video illustrates how to calculate probabilities using the binomial formula in R. The concepts of mean and standard deviation for binomial random variables are also addressed, providing viewers with a comprehensive understanding of how to analyze binomial distributions effectively.
Takeaways
- 😀 A binomial distribution involves counting the occurrences of a binary outcome (success or failure) across a fixed number of trials.
- 📊 The four criteria for a binomial setting can be remembered with the acronym BINS: Binary outcome, Independent trials, Fixed number of trials, and a constant Probability of success.
- 💡 Each trial in a binomial distribution must be independent, meaning the outcome of one trial does not affect another.
- 🔢 The total number of trials (n) and the probability of success (P) are essential parameters for defining a binomial distribution.
- 🪙 An example of a binomial distribution is flipping a fair coin, where each flip has two possible outcomes and a consistent probability of success.
- 📉 The probability of obtaining a certain number of successes can be calculated using the `dbinom` function in R for exact values.
- 📈 Cumulative probabilities (e.g., the probability of getting less than or equal to a certain number of successes) can be calculated using the `pbinom` function in R.
- 🔍 To calculate the probability of getting at least one success, you can use the complement rule: 1 minus the probability of getting zero successes.
- 📏 The mean and standard deviation of a binomial random variable can be calculated using specific formulas: mean = n * P and standard deviation = √(n * P * (1 - P)).
- ✅ Understanding the conditions for a binomial distribution is crucial for applying it correctly in real-world scenarios, such as quality control in manufacturing.
Q & A
What is the main concept of a binomial distribution?
-A binomial distribution deals with counting the number of successes in a fixed number of trials, where each trial has a binary outcome (success or failure).
What does the acronym 'BINS' stand for in relation to binomial distribution?
-BINS stands for Binary outcome, Independent trials, a Fixed number of trials, and a Fixed probability of success.
Why must trials be independent in a binomial distribution?
-Trials must be independent to ensure that the outcome of one trial does not influence the outcome of another, allowing each trial to be evaluated on its own merits.
How can we represent a random variable that follows a binomial distribution?
-A random variable X that follows a binomial distribution can be denoted as X ~ Binomial(n, p), where n is the number of trials and p is the probability of success.
What is the significance of the probability notation P(X > k)?
-The notation P(X > k) signifies the probability that the random variable X is greater than k. This can be calculated using the complement rule: P(X > k) = 1 - P(X ≤ k).
What function is used in R to calculate the probability of getting exactly k successes?
-In R, the function 'dbinom' is used to calculate the probability of getting exactly k successes in n trials, given a probability p of success.
How can you find the cumulative probability of successes less than or equal to k?
-To find the cumulative probability of successes less than or equal to k, you can use the 'pbinom' function in R, which provides the cumulative probability up to k successes.
What are the formulas for calculating the mean and standard deviation of a binomial random variable?
-The mean (μ) of a binomial random variable is calculated as μ = n * p, and the standard deviation (σ) is calculated as σ = √(n * p * (1 - p)).
In the context of rolling a fair die, what is the probability of getting exactly three sixes in ten rolls?
-Using the 'dbinom' function in R with n = 10, k = 3, and p = 1/6, the probability of getting exactly three sixes is approximately 0.155, or 15.5%.
What is the probability of rolling at least one six in ten rolls of a die?
-The probability of rolling at least one six can be calculated as 1 minus the probability of rolling zero sixes. This is approximately 0.838, or 83.8%.
Outlines
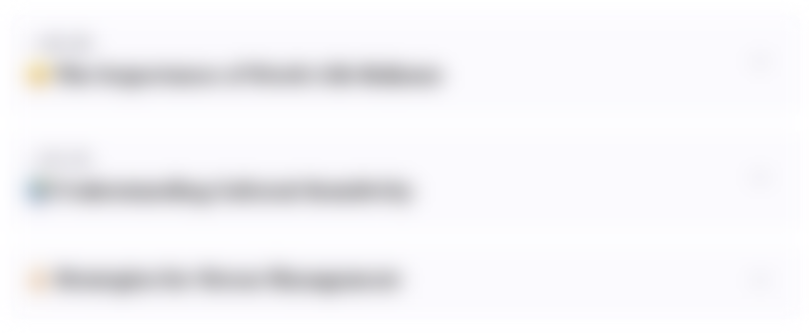
Dieser Bereich ist nur für Premium-Benutzer verfügbar. Bitte führen Sie ein Upgrade durch, um auf diesen Abschnitt zuzugreifen.
Upgrade durchführenMindmap
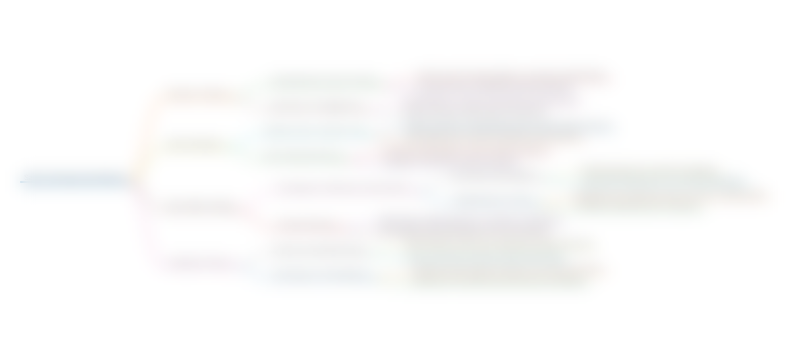
Dieser Bereich ist nur für Premium-Benutzer verfügbar. Bitte führen Sie ein Upgrade durch, um auf diesen Abschnitt zuzugreifen.
Upgrade durchführenKeywords
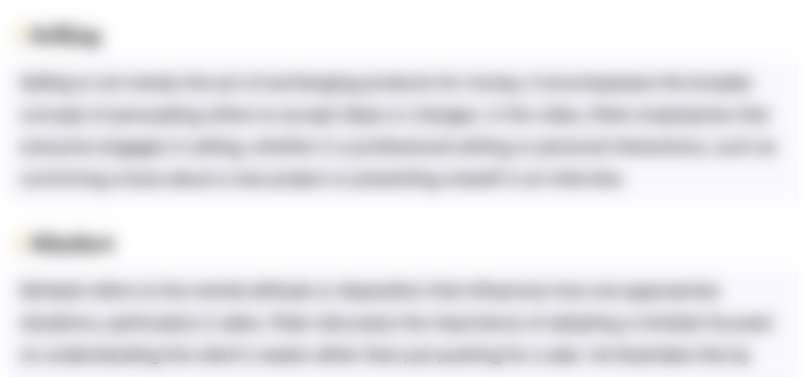
Dieser Bereich ist nur für Premium-Benutzer verfügbar. Bitte führen Sie ein Upgrade durch, um auf diesen Abschnitt zuzugreifen.
Upgrade durchführenHighlights
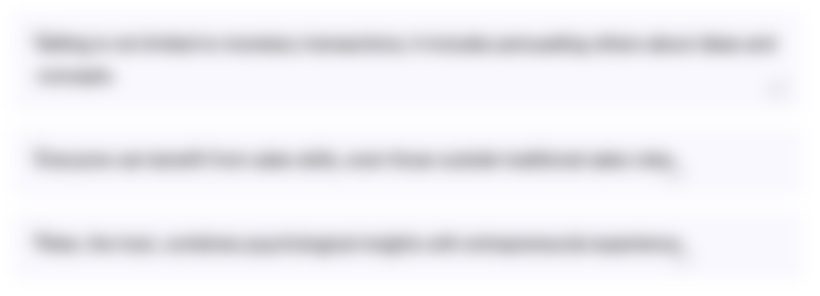
Dieser Bereich ist nur für Premium-Benutzer verfügbar. Bitte führen Sie ein Upgrade durch, um auf diesen Abschnitt zuzugreifen.
Upgrade durchführenTranscripts
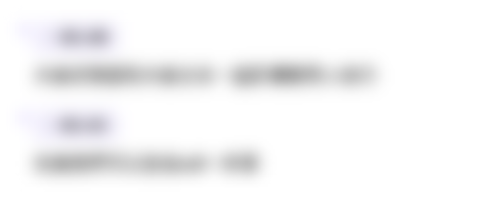
Dieser Bereich ist nur für Premium-Benutzer verfügbar. Bitte führen Sie ein Upgrade durch, um auf diesen Abschnitt zuzugreifen.
Upgrade durchführenWeitere ähnliche Videos ansehen
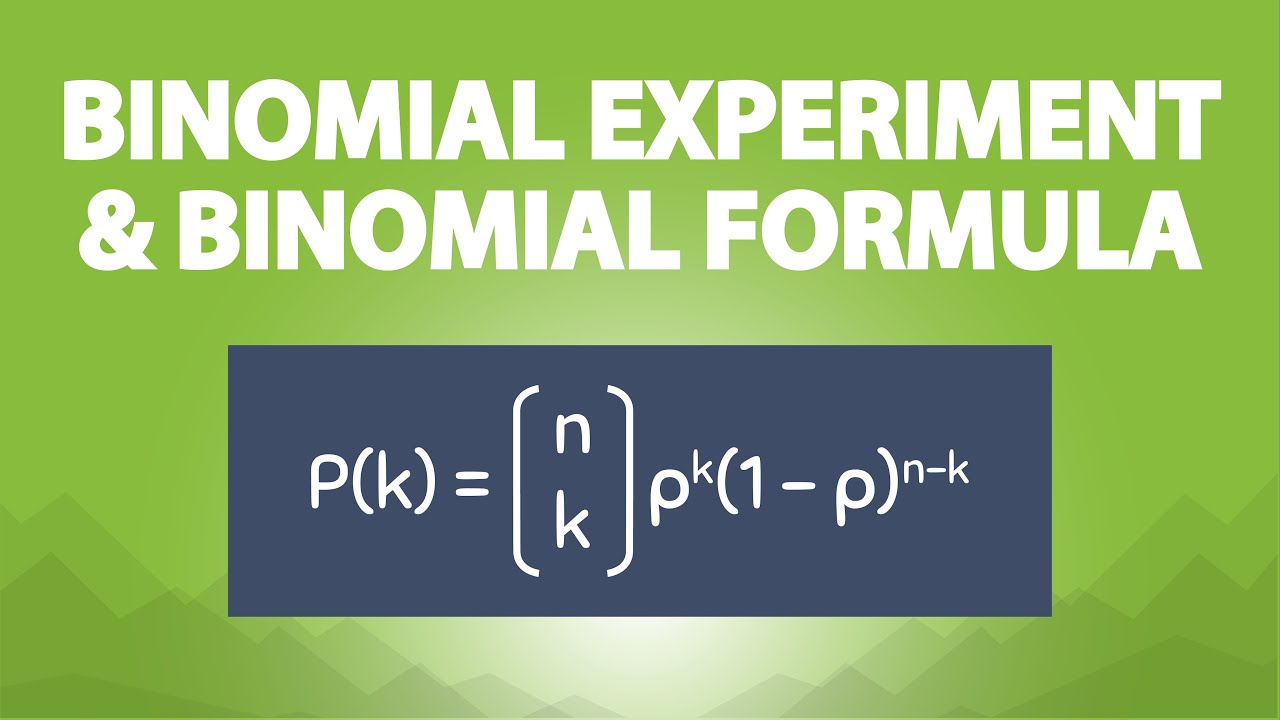
The Binomial Experiment and the Binomial Formula (6.5)
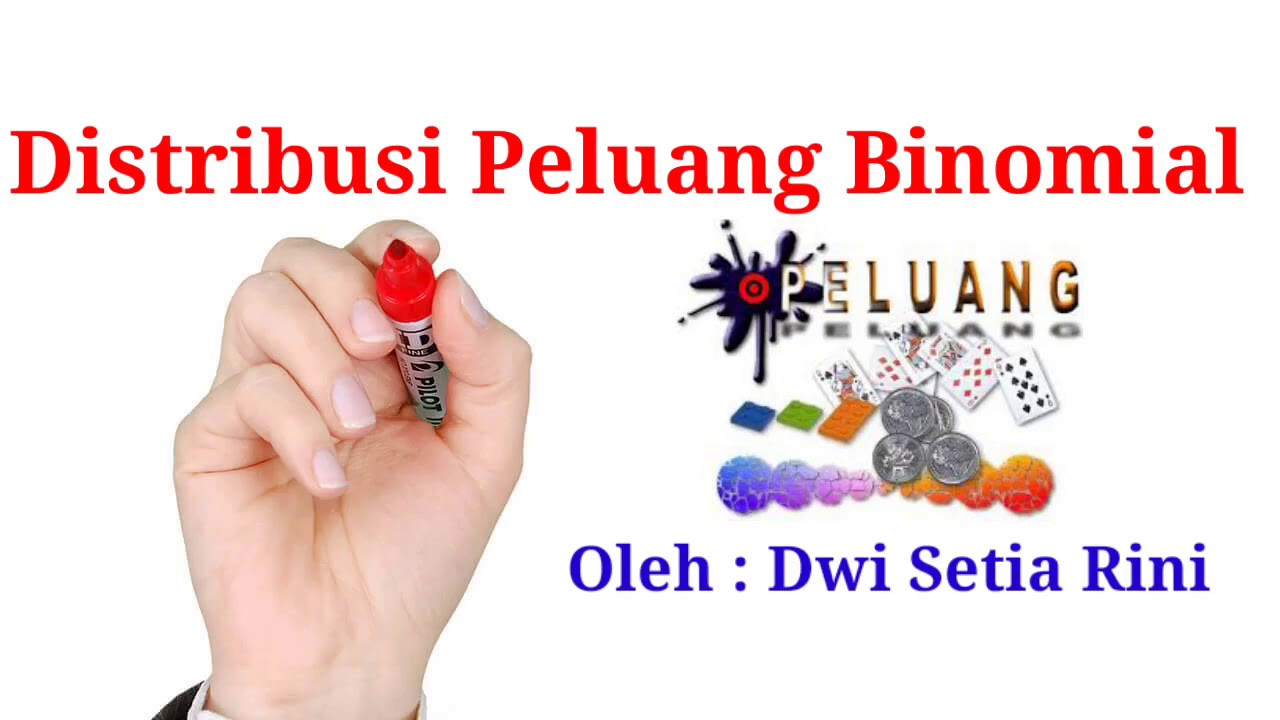
Kelas XII / Distribusi Peluang Binomial

Metode Statistika | Sebaran Peluang Diskrit | Bernoulli | Binomial | Poisson

Sesi 5 3 Distribusi Probabilitas Bagian 3
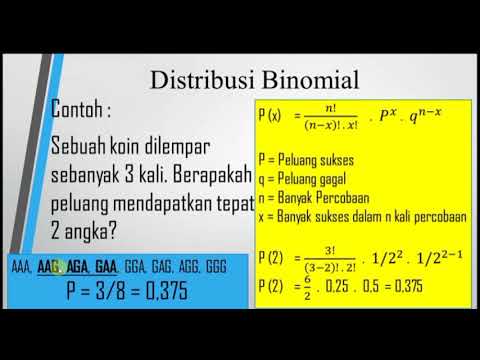
Distribusi Probabilitas Diskrit - Sesi 7 & 8 Statistik Bisnis
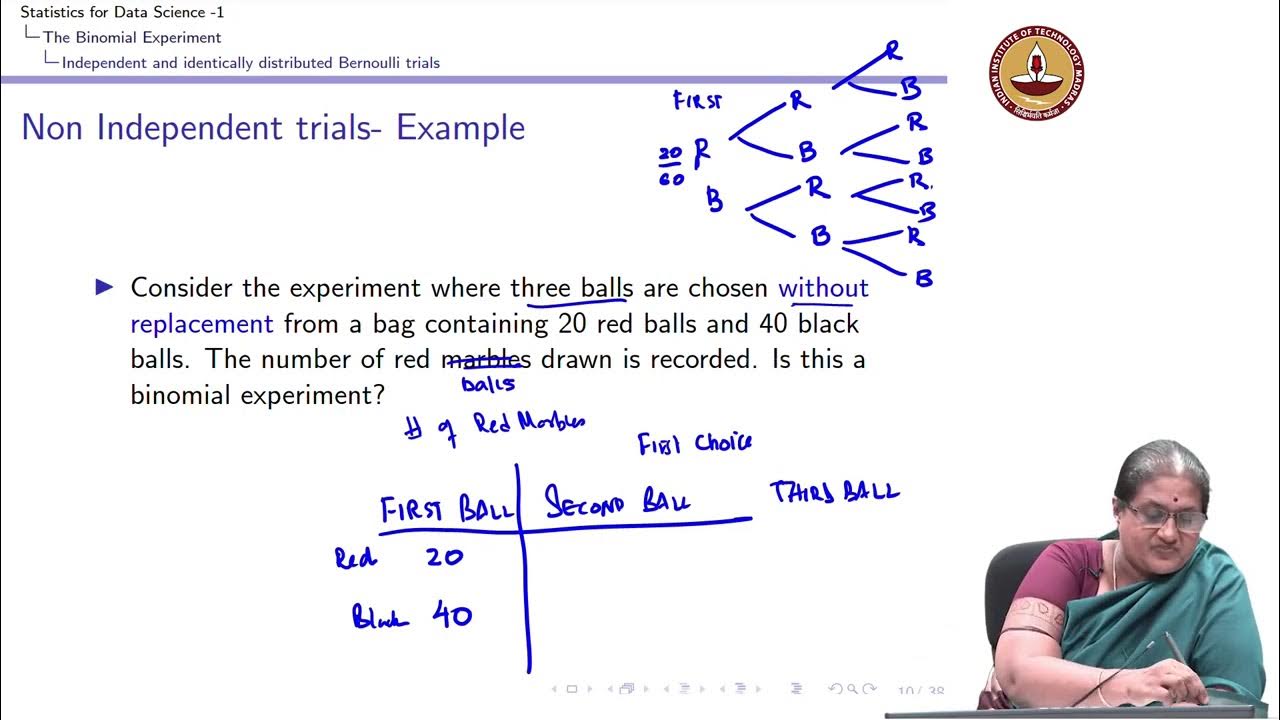
Lecture 10.2 - Binomial distribution - IID Bernoulli trials
5.0 / 5 (0 votes)