The pros and cons of GWAS
Summary
TLDRThis video introduces genome-wide association studies (GWAS), which identify gene loci associated with traits or phenotypes. The host explains the pros and cons of GWAS, emphasizing its hypothesis-free nature and its potential therapeutic benefits. Limitations such as missing heritability and a lack of diversity in the studies are discussed, along with the need for further experiments to enhance accuracy. The video also touches on polygenic risk scores, which predict the likelihood of traits like obesity, and stresses the importance of making these scores actionable and independent of existing information.
Takeaways
- 🧬 Genome-wide Association Studies (GWAS) are used to identify gene loci associated with a trait or phenotype of interest.
- 🔍 GWAS involves scanning the entire genome to find single nucleotide polymorphisms (SNPs) that may be associated with a particular trait.
- 🌟 One of the main benefits of GWAS is that it is hypothesis-free, allowing researchers to identify potential gene associations without prior knowledge.
- 💊 GWAS can have therapeutic potential, particularly when combined with other data like polygenic risk scores, which can predict the likelihood of certain conditions.
- 📈 The data for GWAS is becoming more accessible due to the prevalence of sequencing data, making these studies technically easier to conduct.
- 🔄 Combining GWAS with other studies, like single-cell RNA sequencing, can help overcome some of the limitations and provide a fuller understanding of gene expression.
- ⚠️ A key limitation of GWAS is the issue of 'missing heritability', where identified genetic variants only account for a small percentage of the genetic influence on a phenotype.
- 🔎 GWAS often identifies loci rather than specific genes, which can be challenging as it's the gene function that researchers are typically more interested in understanding.
- 🌐 There is a lack of diversity in many GWAS, which can limit the applicability of findings to different populations and may contribute to 'missing heritability'.
- 🏥 Polygenic risk scores generated from GWAS can be used to predict an individual's risk for certain diseases or conditions, potentially enabling early intervention.
Q & A
What are genome-wide association studies (GWAS)?
-Genome-wide association studies (GWAS) are used to identify gene loci associated with a specific trait or phenotype by analyzing genetic variations across the entire genome. These studies look for single nucleotide polymorphisms (SNPs) that may be linked to certain traits.
What is the main advantage of genome-wide association studies?
-One major advantage of GWAS is that they are hypothesis-free. Researchers do not need prior knowledge about the genome, making it possible to identify genetic variants that are likely associated with a particular trait.
What are some limitations of genome-wide association studies?
-Key limitations include the inability to establish causation, the issue of missing heritability, the challenge of identifying rare variants, and the potential for identifying non-coding regions or loci without clearly linked genes.
What does 'missing heritability' mean in the context of GWAS?
-Missing heritability refers to the gap between the genetic variants identified through GWAS and the full genetic explanation for a trait. The variants typically explain only a small percentage of the heritability, leaving most of the genetic factors unaccounted for.
How can combining GWAS with other studies improve results?
-Combining GWAS with studies such as single-cell RNA sequencing can help researchers better understand which genes are expressed in specific tissues and confirm the association between genetic variants and phenotypes, enhancing the reliability of findings.
Why is the lack of diversity in GWAS a problem?
-Most GWAS have used sequencing data primarily from European populations, which may limit the generalizability of results. Including more diverse ethnic groups could help address missing heritability and provide more comprehensive insights into genetic associations.
What is a polygenic risk score, and how is it related to GWAS?
-A polygenic risk score is a measure that combines the effects of multiple genetic variants identified through GWAS to estimate an individual's likelihood of developing a specific trait or disease. It can be used for early disease prediction and prevention.
What are some potential applications of polygenic risk scores?
-Polygenic risk scores can help predict the likelihood of developing conditions such as obesity, cancer, or other complex diseases. By identifying individuals at higher risk, early intervention and preventive measures can be implemented.
What is the main challenge with using polygenic risk scores for actionable insights?
-The challenge lies in ensuring that polygenic risk scores provide independent and actionable information beyond what is already known. For example, if someone is already obese, a risk score confirming their likelihood of obesity offers little new information.
How can rare genetic variants influence the effectiveness of GWAS?
-GWAS typically focus on common genetic variants, but rare variants may have a greater effect size on traits. These rare variants might be missed in standard GWAS but could play a significant role in explaining missing heritability.
Outlines
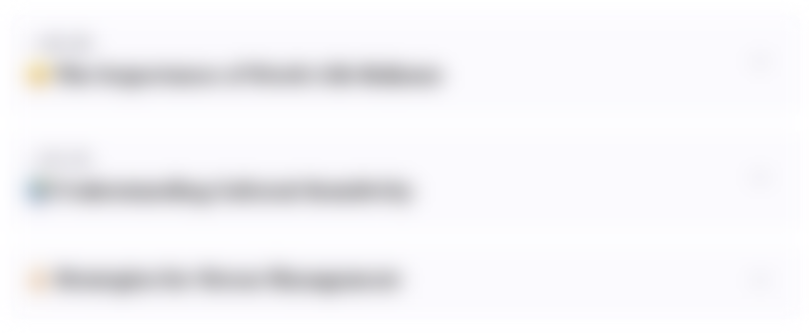
Dieser Bereich ist nur für Premium-Benutzer verfügbar. Bitte führen Sie ein Upgrade durch, um auf diesen Abschnitt zuzugreifen.
Upgrade durchführenMindmap
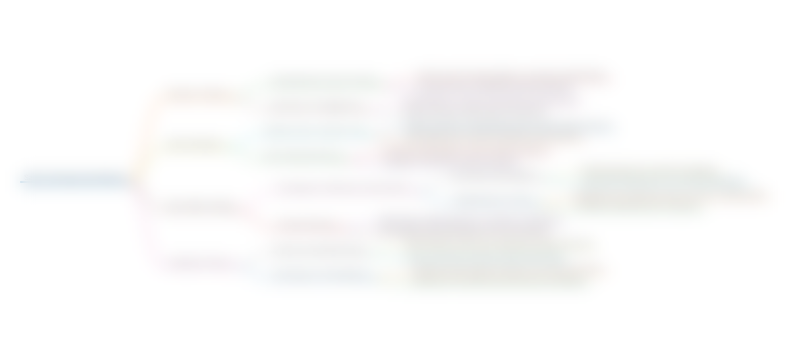
Dieser Bereich ist nur für Premium-Benutzer verfügbar. Bitte führen Sie ein Upgrade durch, um auf diesen Abschnitt zuzugreifen.
Upgrade durchführenKeywords
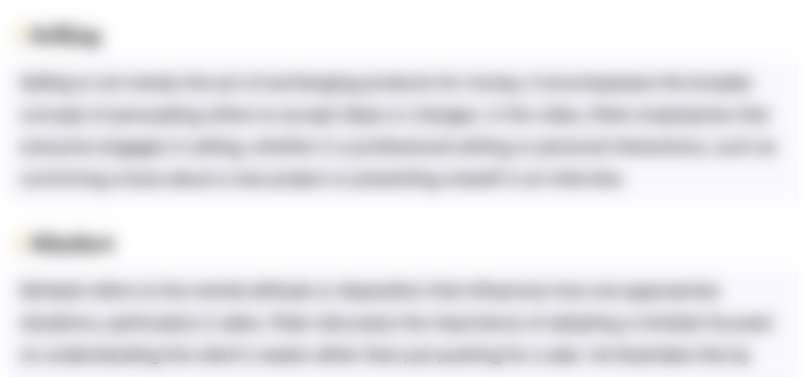
Dieser Bereich ist nur für Premium-Benutzer verfügbar. Bitte führen Sie ein Upgrade durch, um auf diesen Abschnitt zuzugreifen.
Upgrade durchführenHighlights
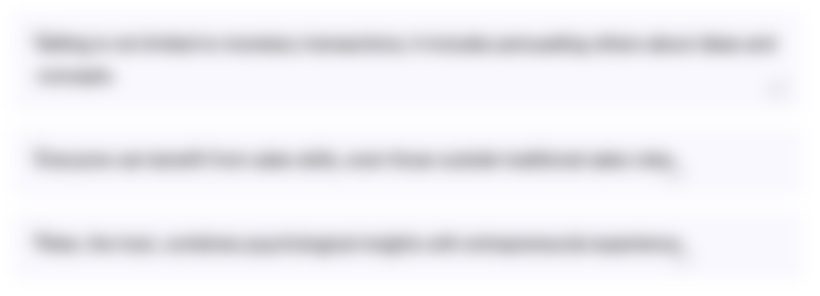
Dieser Bereich ist nur für Premium-Benutzer verfügbar. Bitte führen Sie ein Upgrade durch, um auf diesen Abschnitt zuzugreifen.
Upgrade durchführenTranscripts
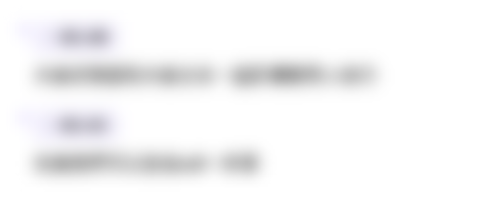
Dieser Bereich ist nur für Premium-Benutzer verfügbar. Bitte führen Sie ein Upgrade durch, um auf diesen Abschnitt zuzugreifen.
Upgrade durchführenWeitere ähnliche Videos ansehen

Genome-Wide Association Study - An Explanation for Beginners

PGC: Genetics of Bipolar Disorder - Brandon Coombes & Kevin O'Connell
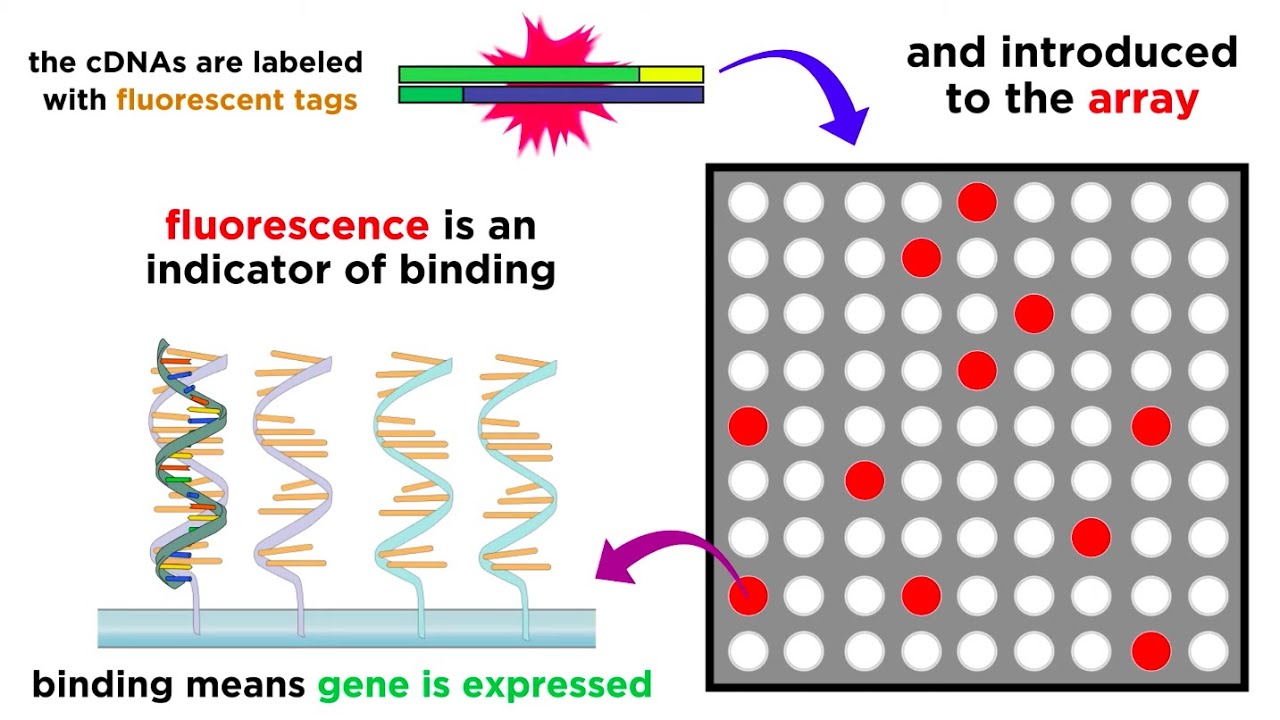
Gene Expression Analysis and DNA Microarray Assays

Does your DNA make you popular?

DNA and Behavioral Genetics - Robert Plomin

Chromatin structure, gene regulation, and epigenomic mapping assays
5.0 / 5 (0 votes)