Intelligence in the Age of AI with new CTO of the CIA
Summary
TLDRIn a thought-provoking discussion, a16z General Partner Martin Casado and a16z Enterprise Editor Derick Harris converse with Nund Mulchandani, the first-ever CTO of the CIA, about the future of defense and intelligence in the age of AI. The conversation delves into the evolving relationship between AI and analysts, the challenges governments face in keeping pace with exponential technology, and the impact on both offense and defense strategies. The discussion highlights the transformative potential of AI in intelligence work, emphasizing the need for a balanced approach to leveraging this powerful tool while maintaining the human element crucial to the field.
Takeaways
- 🌐 The rapid advancement of AI technology is transforming various industries, including defense and intelligence, with the potential to reimagine job roles and tasks.
- 💡 AI's impact on the intelligence community is not just about automating existing tasks but also about the potential for new, creative applications that were previously unimaginable.
- 🔄 The CIA is adapting to the AI era by focusing on three key areas: operational efficiency, analytical support, and external engagement through platforms like podcasts and videos.
- 🛡️ While AI offers new capabilities, it also brings challenges such as the need to detect and counter deepfakes and other AI-generated deceptions.
- 🤖 Generative AI, like large language models, can be used as a tool for analysts, but there is still a need for human oversight and decision-making, especially in critical areas.
- 🚀 The US government and intelligence agencies are at a crossroads with AI, needing to balance the adoption of new technologies with security, ethical, and policy considerations.
- 🌟 AI's role in national security is not just about offense but also defense, and the technology's democratization means that it can be used by anyone, not just sophisticated agencies.
- 🤔 The intelligence community is grappling with the question of how AI will change the equilibrium between offense and defense in the context of national security.
- 🔍 The CIA is exploring the use of AI in a measured way, recognizing the need for experimentation and learning while also understanding the limitations and risks of the technology.
- 🌐 The future of AI in intelligence is not just about the technology itself but also about how humans interact with it, and the cultural shifts needed within agencies to fully leverage its potential.
Q & A
What is the main focus of the CIA's technology shift under Director Burns?
-The main focus of the CIA's technology shift under Director Burns is from CT to great power competition, with a significant emphasis on dealing with the amorphous entity called technology.
What are the three key areas the CIA is focusing on with the new emphasis on technology?
-The three key areas the CIA is focusing on are the creation of the China Mission Center, the transnational technology Mission Center (T2MC), and the establishment of the CTO role.
How is AI impacting the intelligence community?
-AI is impacting the intelligence community by enabling the detection of patterns in large amounts of data, automating routine tasks, and potentially transforming the way analysts gather and process information.
What is the 'pull model' versus the 'push model' in the context of AI and data analysis?
-The 'pull model' refers to the traditional approach where analysts had to come up with queries to find information, whereas the 'push model' involves AI technologies pushing relevant information to analysts based on their needs and focus areas.
What is the concern regarding AI's ability to amplify biases in the intelligence community?
-The concern is that AI algorithms, which are designed to please users, may inadvertently amplify the biases of analysts, leading to a 'rabbit hole' effect where analysts are drawn into confirmation biases and远离from objective analysis.
How does the CIA plan to use AI in its operations and analysis?
-The CIA plans to use AI as a tool to enhance the effectiveness of its case officers and operations teams, as well as to aid analysts in discovering patterns in data. However, it emphasizes that AI will not replace the need for human intelligence and critical thinking.
What is the significance of the 'co-pilot model' in the context of AI and intelligence work?
-The 'co-pilot model' refers to the use of AI to assist analysts with routine tasks and to provide support in their work, without replacing the human element of intelligence gathering and analysis.
How does the CIA view the potential for AI to create new information?
-The CIA believes that while AI can find and routinize existing information, it is unclear whether current AI systems can truly produce new information or thought, especially in the context of intelligence work which often involves 'tail reasoning' or thinking outside the norm.
What is the 'hallucination problem' in AI and how does it relate to intelligence work?
-The 'hallucination problem' refers to AI's tendency to generate responses or information that are not commonly represented in its training data, leading to errors. In intelligence work, this level of uncertainty is unacceptable, especially in operational contexts where explainability and accuracy are crucial.
How does the CIA approach the challenge of analysts reimagining their jobs in the face of AI advancements?
-The CIA encourages its analysts to think beyond mere automation and efficiency gains, challenging them to consider how they can reimagine their roles in 5 to 10 years as AI technologies continue to develop and become more integrated into their work.
What is the role of open source technology in the CIA's AI strategy?
-Open source technology plays a significant role in the CIA's AI strategy, allowing for flexibility and customization to meet specific agency needs. However, the CIA also recognizes the challenges of integrating and securing open source solutions in their operational environment.
Outlines
🚀 Introduction to AI's Impact on Jobs and National Security
The paragraph discusses the transformative power of AI, emphasizing its potential to redefine jobs and national security. It highlights the shift from traditional job automation concerns to the need for reimagining roles in light of AI advancements. The conversation also touches on the American dynamism and the collaborative effort between Silicon Valley, the government, and the defense sector to harness AI's capabilities.
🤖 AI's Disruption and Its Role in the Intelligence Community
This section delves into AI's disruption across industries, with a focus on its impact on the intelligence community. It discusses the historical significance of AI in intelligence work and explores the implications of generative AI on intelligence agencies. The speakers consider the ease of detecting AI-generated content and the shift towards more human-focused, less technology-centric approaches to intelligence problems.
🌐 AI's Impact on Analytic and Operational Sides of Intelligence
The paragraph examines the dual impact of AI on the analytic and operational aspects of intelligence work. It contrasts the operational side, characterized by spy-versus-spy dynamics, with the analytic side, which involves big data and pattern recognition. The conversation highlights the revolutionary potential of AI in uncovering patterns and the challenges of avoiding bias in analysis.
🔄 The Evolution of AI in the Intelligence Agency's Strategy
This section discusses the integration of AI within the intelligence agency's strategy, focusing on its use cases and the experimental approach to its implementation. It emphasizes the importance of not spreading AI applications indiscriminately and the need for thoughtful consideration of its applications. The paragraph also touches on the agency's public acknowledgment of using AI and the push for employees to reimagine their roles with AI's assistance.
🤔 Reflections on AI's Future Role in Intelligence Analysis
The paragraph contemplates the future role of AI in intelligence analysis, considering the current limitations of AI models and their potential to complement analysts' work. It discusses the concept of 'tail reasoning' and the challenges of applying AI to unique, exceptional scenarios. The speakers also reflect on the transformation within the intelligence community and the cultural shifts necessitated by the advent of AI.
🤝 Encouraging Collaboration Between Tech and Government
This section emphasizes the importance of fostering collaboration between the tech industry and the government, particularly in the context of AI and national security. It discusses the need for a cultural shift within the intelligence community to embrace technology and the challenges of integrating commercial technologies into government operations. The conversation also touches on the potential for public-private partnerships and the importance of understanding and adapting to emerging technologies.
📈 Policymaking in the Era of Emerging Technologies
The paragraph explores the complexities of policymaking in the face of emerging technologies like AI. It highlights the challenges of building policy on top of rapidly evolving tech landscapes and the need for an iterative, long-term process involving both industry and regulators. The conversation underscores the importance of understanding the unique characteristics of AI and the potential for it to be used for both beneficial and malicious purposes.
🎥 Inside Look at a16z American Dynamism Summit
This final section provides an overview of the a16z American Dynamism Summit, offering insights into the event's exclusive stage talks and the opportunity to engage with policy makers, founders, and funders. It encourages viewers to visit the a16z website for more information and to access the summit's content, which aims to foster a dialogue on American dynamism and innovation.
Mindmap
Keywords
💡Artificial Intelligence (AI)
💡American Dynamism
💡Generative AI
💡CTO of the CIA
💡Human Intelligence
💡Defense Intelligence
💡Pattern Recognition
💡Reimagine Jobs
💡Asymmetric Superpower
💡Compute Projects
Highlights
Human intelligence operation's capability to make anyone be anybody, sound like anybody, and look like anybody.
The shift in focus from CT to great power competition and technology under Director Burns' leadership at the CIA.
The creation of the China Mission Center and Transnational Technology Mission Center within the CIA.
The role of the CTO in the CIA being a new position to handle the agency's technological focus and external engagement.
Artificial Intelligence's impact on various industries and its role in the defense of the country.
The challenge of detecting AI-generated content and the potential for AI to amplify biases in analysis.
The importance of human intelligence and the all-source approach in the CIA's operations.
The potential of AI to revolutionize pattern recognition and data analysis in the intelligence community.
The limitations of current AI models like LLMs and their suitability for intelligence work.
The need for the intelligence community to reimagine job roles in light of AI advancements.
The comparison of AI's impact on the intelligence community to the internet's impact, and the importance of not applying old lessons directly.
The cultural shift within the CIA towards embracing technology and the challenges it presents.
The discussion on the potential of open source AI and the trade-offs between using base algorithms and creating bespoke solutions.
The importance of public-private partnerships and the government's role in driving innovation and addressing the needs of national security.
The transformation and modernization efforts within the CIA to become a better customer for technology and innovation.
The unique challenges of policymaking in the context of emerging technologies and the need for an iterative, long-term approach.
Transcripts
[Music]
we're a human intelligence operation we
can now make anybody be anybody and
sound like anybody and look like anybody
stop thinking about automating getting a
10% 20% 30% on your job tell me in 5 to
10 years how you going to reimagine your
job this is no like asymmetric
superpower which by the way is very very
different than the internet well it's a
American dynamism it's this idea of
Silicon Valley leaning in and the
government having to lean in together
for us to meet in the middle to be a
better supplier and a great customer
these are the largest compute projects
mankind has ever done before like we've
never done anything close to this life
finds a way which if there's demand for
it people will supply it artificial
intelligence has taken this world by
storm just think about it here in 2024
anyone with an internet connection and a
few minutes to spare can literally spin
up a Disney Avatar of themselves
translate a foreign podcast into their
native language and even get help
writing their vows but artificial
intelligence is not just impacting the
creative spheres in fact you'll be
hardpressed to identify an industry
that's not touched by this technology
and the defense of our country is no
exception in today's episode originally
recorded in the heart of Washington DC
back in January during a16 Z's American
dynamism Summit a6z General partner
Martin cassado and a16z Enterprise
editor derck Harris are joined by first
ever CTO of the CIA yes that is the
Central Intelligence Agency and we're
joined by CTO nund mulchandani to
discuss the future of Defense
intelligence in this wide- ranging
conversation they discussed the evolving
relationship between Ai and analysts how
governments can keep up with this
exponential technology and finally how
it's impacting not just offense but also
defense oh and if you'd like to get an
inside look into a16 Z's American
dynamism Summit you can watch several of
the stage talks from the event featuring
policy makers like Congressman Jake aen
Claus or Senator Todd young and of
course both Founders and funders
building toward American dynamism you
can find all of the above at
az.com ad Summit all right let's get
started Martino most people watching
listening to this are fairly familiar
with with you and your role at a16z but
n because the CIA CTO role is relatively
new can you give us like a quick
background on that role and kind of what
your objective is so uh my journey so
there really two stories here one is the
agency needing a CTO and kind of what
what created that and then my own
journey to it uh the big thing it all
all starts with director Burns taking
over the agency uh at the beginning of
the administration and he just like any
great business leader sat down and and
did a business review which is you know
what what business are we in what are
the big threats and and and the other
pieces and the conclusion was actually
fairly uh interesting uh and not a
surprise was that we had it pivot from
CT which had been the big sort of focus
for the agency for a couple of decades
to great power
competition and then the interesting
unusual sort of thing was this big
amorphous thing called technology which
is something that we had to sort of deal
with
uh there was huge interest obviously
from policy makers in technology that we
needed to start looking into and build
policymaking around that I think it
might be helpful to the listeners to
understand kind of what CIA actually
does because I had to actually learn a
lot about the agency when I came on
board uh and sort of what what Amy zard
calls uh spy tainment to like what we
actually do and so this pivot uh in
terms of rethinking the agency's focus
on technology uh there were three things
that happened one is we created the
China Mission Center which is how we
actually uh focus on on on on threats
and opportunities things t2mc which is
transnational technology Mission Center
and this weird thing called a CTO we're
a 76y old spy agency so we've been doing
technology for a long long long long
time but technology has been somewhat
latent what happened is with this focus
on Tech we basically needed to focus on
these sort of three different things
that I think were different from what we
were doing before number one is we are
as an agency fairly um focused
vertically focused and the CTO function
really gives us the luxury a little bit
of looking a little bit out rather than
Focus sort of Inward and um the third
thing is a little more external versus
internal so engaging with the outside
world uh doing things like this
particular podcast and and video cast
and other pieces so those are kind of
the big Dimensions there and iess I
guess Martin people know you as investor
and founder of but I guess maybe it's
worth noting you also s worked in the
intelligence Community for a little bit
right so you you come to this with a
little bit of background huh yeah that's
right yeah 20 years ago let's start with
talking about AI which is kind of
disrupting I think everything at the
moment I would love to get both of your
perspectives maybe Martin we can start
with you on like how you thinking about
AI as it relates to the intelligence
Community like where we're at and and
probably where we're headed you know AI
has been around for a very long time um
and I will say that um even when I was
part of the intelligence Community 20
years ago we talked a lot about if you
have all of this information how do you
kind of detect signal a lot of this was
like very significant big data
processing and a lot of the more kind of
advanced Notions actually came out of
the intelligence Community I mean a lot
of what the intelligence Community deals
with is you know things like enty and
covert comms Etc a lot of these ideas
are just fundamentally tied with AI um
and so I just think there's a
long-standing history what's interesting
to ask is is how does this this kind of
new generative AI World impact the
intelligence agency and and and or like
intelligence in general and one idea is
like well okay so we can we can now make
anybody be anybody and sound like
anybody and look like anybody and oh
that could be a huge problem because now
you know like this kind of deep face
could be a problem and then I'll tell
you my kind kind of conclusion in a lot
of this is um you know it actually turns
out that if a computer generates
something the ability to kind of
fingerprint that isn't that difficult
it's actually not that hard right so I
actually think it becomes much much
easier to detect if people are using Ai
and tooling actually is the reality but
that also means that we can't use them
as tools and then we've got to go much
more to the fundamentals so this kind of
weird irony like we've got this set of
new tools that allows anybody to sound
like anybody or be anybody and that's
going to be heavily heavily used around
the world but like for those that are
sufficiently sophisticated it's going to
be quite possible to detect them and I
think that in some ways this is kind of
this nice cover in chaos for what you
know our agencies and many agencies are
very good at which is kind of much more
you know human Focus less than you know
core technical approach to these
intelligence problems I guess it's
probably fair to say yes like we've been
ai's been around for a long time machine
learning even you know deep learning
back a decade ago now but like yeah
generative AI LMS and that sort of thing
are
definitely do you think do you think
about it like Martin referenced kind of
like the defense and the offensive side
of that right yeah so exactly that and
I'll just complement what Martin said
was you know really when you look at the
two big functions that we we we have as
an agency right we've got the
operational side and we've got the
analytic side and of course those are
composed in to different things but
those are really broadly the two big
functions so what Martin absolutely
nailed is the operational side of it
it's spy versus spy it's cat and mouse
it's all the usual stuff right the
democratization of this stuff and the
availability we know is going to drive
each one of our competitors to be
driving this up we're going to be aware
of that we're going to drive our own
stuff up so there's this aspect of we
don't know where this is going to go but
it's definitely not pulling back this is
where you know the the stops are off um
however the thing we've always got to
keep at least we keep in
mind we're a human intelligence
operation we're a foreign intelligence
operation we're all source so these are
the things so we have particular Focus
within the 18 intelligence agencies to
focus on a particular thing so
everything he said in terms of using or
wielding or scaling this Ai and
operations all is within the context of
taking our case officers and operations
teams and making them successful right
because any good sort of team sport we
play a particular role on the field and
applying this Tac to scaling and making
our our teams basically our folks more
effective and win is basically the game
now on the analytic side it's a
complimentary but a different problem
and this is where the big data and the
other pieces come in is to me what's
revolutionary about this is underlying
AI is the the the promise and excitement
always is the pattern patterning right
the ability to discover patterns in
large amounts of data that typically
humans can't see right it's not easy for
them to see what's different now in the
older days of when we were doing big
data analytics and things I call this
the pull model versus the push model
which is the analyst had to come up with
creative stuff to think about first and
say I'm going to put a query in to go
find the information the problem is is
the conceptual boundary of an analyst to
hit the query that hits the data was it
was like spear fishing right you're like
going in and trying to get this one anal
analytic idea out of this massive data
what's beautiful about this Tech is
nowaday now this stuff can actually push
stuff right you can almost invert the
process where a lot of the stuff gets
pushed to you because it starts to
understand what you're looking for in
some sense and starts tailoring the
stuff and gets deeper and deeper and
deeper over time right this is the uh uh
stuff you've been driving to now that
has a uh evil twin problem to it that I
I've been spending time on thinking
about just as it relates to our work is
what I call the sort of uh rabbit ho
holding problem which is the very thing
that makes these these uh products and
Technologies so effective which is
learning about you and knowing and I
think on one of your previous podcasts I
think you or Mark talked about this idea
of these these algorithms are built to
please you they want to make you happy
well it can then also unfortunately take
the things that you as an analyst are
weakest at or it's your uh your Weak
Knee or your thing that that it can get
into your head and start Rabbit holding
you down this thing which amplifies your
biases we've got to be very careful very
smart about where we apply it when and
how modul all these all these particular
things that are out there how close are
we actually I mean because I feel like
we've heard maybe going back to the
early days of talking about Big Data
like how personalized something will be
or how how easy it's going to be to sort
Stu I mean or are we are we still away a
ways away from like you know very much
automating like maybe in a CIA analyst
sort of so so I I I think I think we're
now understanding the kind of power and
limits of of this technology I think
well first off I think there's a very
important topic for us to to add like
like maybe prediction to make which is
will this change the existing
equilibrium that you know that is now an
intelligence between kind of like
offense and defense and and Etc and like
my deep belief is exactly what Nan says
which is like it doesn't change the
equilibrium in the sense that that you
know there's going to be more toing for
offense there going be more toing for
defense this is no like asymmetric
superpower which by the way is very very
different than the internet and this is
why this is such a bad analog the
internet was asymmetric which is like
the more capabilities you had with the
internet the more vulnerable you were
which is why like when we were working
on the terrorist threat we're like you
know we're the most vulnerable nation in
the world and like you know many of the
people that we were focused on didn't
even have laptops it's nothing like that
you know AI is something that anybody
can use um it doesn't change some
fundamental equilibrium in an a
asymmetric way so so so that's the first
answer to your question is does it allow
for personalization or does it allow for
kind of anything specific I think that
you know it's kind of you know it
doesn't create any EMB balance between
two opposing sides so the second answer
that is I think we're pretty clear about
like even the individual limits of let's
say llms right now which is they're very
very good for a few use cases they're
very very good for like you ask one
question they give you one response and
if that question is in the Corpus of
training data it'll give you a good
response the problem is if the question
is out of the Corpus of the training
data like you don't really know if the
response is good or not which is fine if
you're just asking one question because
most humans will be able to back check
that right so it's very good if you like
have like a co-pilot for an analyst and
you help them with their job the thing
that we've not seen any evidence of is
agentic behavior and by agentic Behavior
it means like you ask one question then
you step away and you go get a coffee
and it does its own thing and the reason
is is if it generates anything out of
distribution like and by the way out of
distribution means it's not commonly
represented in the training set then
that error is going to acre and it tends
to ur exponentially provably right and
so I think right now we view these as
new tools that you use you know side by
side but they don't become their own
separate kind of entity yeah and that
that absolutely brilliant point is this
idea of the acral of the prob
probabilities times probabil
probabilities which gets the
hallucination Stuff Etc when it comes to
my 11-year-old drawing unicorns that's a
feature not a no problem no problem
awesome hallucinate away that's awesome
that's great uh it playing games doing
all kinds of crazy like that's amazing
when it comes to analytic capabilities
when it comes to operations we cannot
have this level of uncertainty and and
not you know knowing and explainability
I mean I think that we're in such these
sort of early stages of this game right
so everything we're talking about here
is like we were in 1990 five show up two
years ago exactly I mean this thing just
happened and we were just talking before
this like all of us old F three old
folies here you know sitting around the
porch this very well could be a porch
with you know rocking chairs and I
sitting around talking about the early
days of the internet we could not have
possibly imagined the stuff that's
happened over the past years
so at the agency what we're doing is
saying great there's a whole bunch of
these basic use cases that are just
there's no question this stuff can get
applied and by the way we're all in on
it right so I didn't ever wanted to give
the impression that this is something
that is is uh you know we're slow
rolling it or thinking it but to
martine's point the applications on a
peruse basis we have to think them
through this is not peanut butter that
you can just spread everywhere and you
get goodness everywhere with with no
thinking so for instance we're public
with the fact that we actually have llms
in production at the agency we have it
in production in the open source team uh
you know so we're so those easy use
cases business automation other pieces
we're experimenting we're trying we're
doing stuff now the co-pilot piece the
way I view it is typically people jump
immediately to the hardest of hard
problems and say we're just going to go
replace this we're going to go do this
that what what we're challenging
everyone to do inside the agency though
is it's one thing to like look at the
low hanging fruit get that stuff
automated get the value the thing that's
most interesting though is can and we're
challenging fix to say stop thinking
about automating getting a 10% 20% 30%
on your job tell me in 5 to 10 years how
you going to reimagine your job now
here's where problems here's where
things get tricky because many of the
people who are challenged to reimagine
their
job are looking at this technology and
learning it right for the first time and
understanding the power of it I'm still
like I mean all of us are still very far
away from knowing where this going to go
so it's really hard for them to imagine
this prototype thing that's like still
playing around how's it going to impact
or rethink my job so this is where you
know we're pushing and experimenting and
encouraging everyone try stuff learn
stuff get up the experience curve but
we're not going to settle on an answer
CU that's not going to just appear
magically off that so I I read something
recently a CIA analyst like imagining
their job I don't know what the time
frame was like five or 10 years out and
it it was very much like it was very
much what you describe right like enter
a prompt go get a cup of coffee and and
then by the end of the day we've
arrested someone in France for something
right that's how people it's uh uh we
took the kids to Disney World and you go
on the Epcot ride
which was the imagination of it's like
ride of the future it was from the 1950s
or 60s imagining what the world would be
like in
2024 and it's
like a a handheld phone with a video
monitor on it like okay you know so it's
it's one of these things which is it's
really hard to see where the Stu is go
do you guys have a sense of like where
how the analyst I mean again again
noting that it's early I mean
realistically like it's just a thing
where like analysts just have different
skill sets going forward they have just
this have different tools at their
disposal I mean how does it well I think
we can say
something relatively specific on this
and then there's a bunch of stuff we
don't know but here's the thing that's
relatively specific so the way that
these large language models in
particular work is they get a whole
bunch of data and then they basically
have a distribution of the you know how
common the data was represented like
that's what they do right they they do
basically what's called kernel smoothing
over positional embeddings which is just
like aing a bunch of like words so it
averages a bunch of words and then for
any time that you ask it a question it
kind of gives you like the most common
outcome so what does this mean this
means for like for mean things you want
to do for the a for the the average
thing you want to it would be very good
to get you an answer so for any kind of
like standard rote thing you want to do
it's going to give you an answer the
problem is is if you want to do
something in the tail or in something
that's new or an exception it doesn't
know how to do that like there's no
mechanism within it that will do that
and so much of like intelligence work I
would argue is actually in the tail
right I mean it's like these are the
problems the intelligence agency is
particularly good at and so I think we
can believe that there's a world with
you know every analyst will have
strapped on an llm that'll help them
with the routine stuff but like so much
of the job is tail reasoning reasoning
in the tail that this is not going to
remove the humans and by the way this
isn't just the intelligence Community I
think this is most work but I think it's
particularly acute in the intelligence
Community yeah and I
break it up into the first piece is uh
does this technology replace the analyst
the second piece that Martin talked
about is the co-pilot model which is I
I'm I do my work and I have a little
wing person that helps me with all the
routine stuff or scaling but it's
exactly his point which is it's not the
creation of new information from old
information like human beings uniquely
create new things new information it's
unclear whether these systems actually
produce new information information or
new thought it's just finding it or or
routinizing it the third one is what I
call the sort of crazy drunk friend
problem or other pieces which is the
hallucinating or the piece which has a
role in some disciplines right making
new art poetry and in the analyst
function it's this point of finding that
point on the distribution if the average
policy maker could think through the
average use case and what's the role of
the analy
the role of the analyst is to have this
holistic piece of thinking through
probabilities I mean it's kind of what
an AI program would do to some extent if
you start modulating where on the sort
of distribution you want to go here's a
good analog imagine you're an analyst
and imagine and if you get paired up
with like some person that's been in the
agency for like 40 years so this person
doesn't know new technology it just
knows what everybody's done a whole
bunch is that the entire perspective of
course not like you have to evolve but
it's a very important perspective so
llms are very good at being that old
person they're very good at being like
well this is how we've done it in the
past here's a recommendation but like
that's why we have people to make a
decision like do I want to do something
new something that's in the tail or do I
want to listen to this person that's
been around for a very long time so it's
a it's a very concrete kind of mental
book end for doing things but the
majority of the value which is the new
stuff will still remain with the person
one interesting thing for me has been
being you know having spent 25 years in
the valley done a bunch of startups and
now being on the on the government side
is a lot of the tech discussion around
this is about the possibilities and all
the great stuff on creation side which
is awesome Innovation invention getting
great people to do group awesome stuff
but you have to flip over to the buy
side of Technology this is my first the
second run Pentagon was two and a half
years and now it's CIA of being on the
bu side of technology and seeing all the
stuff happening I just actually took a
red eye in from California and like you
over there it's all about possibilities
and here it's about how do we take the
job the function that we have to operate
in with all the constraints and things
which are by the way not uh constraints
that are artificially imposed I mean uh
you know the CTO office at the agency
right next door is where the pdb gets
made for the president and so by the
time some hits that threshold of getting
into the presidential Daily Brief you
can imagine the level of scrutiny and
Analysis and focus that our analysts put
into this work and so it's funny because
like the excitement and hype about this
technology versus US absorbing it and
making it battle ready is a long long
distance and so I I don't hopefully you
know uh portraying or representing that
side of the equation which it doesn't
happen rapidly right it takes a long
time for folks in their own disciplines
and life and careers to understand
what's the actual impact the absorption
of this technology does take a long time
and for it to disrupt a particular
individual or an individual discipline
or a you know whatever these things do
you know so that so I so I experienced
when I was in the intelligence Community
something very similar but but but
modestly different which is the
following which is um I I know I was an
Ops and we would have you know missions
and stuff to do um and they would have
very specific requirements and and the
the piece of technology that came from
like the public SE or the private sector
just were not suited and in fact little
know story so my you know my PhD work
was in software defi networking before
it was done in Hardware to tough to
program we need to make like honestly
the insight for that work all came from
my time working with the intelligence
agencies because we had to build a
network that had to like deal with
certain things and I'm like you know I
actually came from the Computing side of
the world I'm like these things aren't
programmable and and to do what we need
to do here I have to program them
because you know Cisco just doesn't know
what we need to do so there's also a
flip side so everything you're saying I
I totally agree it takes a long time to
be adopted but also your requirements
and needs are a bit different than what
they are than what the market ising for
yeah yeah yeah well actually you know
what's funny is uh first uh it will sh
lessly say I I stole uh I've been a huge
software Define networking fan for a
very long time I know we overlapped at
VMware you know when you were acquired
in I had got acquired in uh before but
uh uh I stole it for a paper uh that I
coote with Shan General Shanahan called
s find Warfare I love it and the idea
that this strongly Endo ex there you go
yeah uh you can go download download uh
early and often but yeah basically this
idea of like how do you actually take
something that's on a hardware Hardware
curve and push it onto a software curve
how do you do this Pro reprogramming Etc
and so the question we've been asking
inside is what does software defined
intelligence look like I love it right
so what's the next level of like stuff
where to point it's maybe more push
versus pull it's this idea of going
across that distribution curve and
starting to understand uh one other
piece that that's been sort of uh right
in the middle of this whole thing is the
whole policymaking debate inside you
know Etc uh one key point I wanted to
make was it's very interesting so the
work that each of us do has encoded in
it the policies and outlines of what we
have to do as part of a job function and
I call this code AS law which is that
when you look at the applications that
you probably use at the agency and that
we we we use there we encode all of
those rules and regulations inside of it
and I call this the thresholding problem
to some extent which is inside a line of
code in our application there is
something that says if probability of X
happens Beyond this then do this versus
that right right we have lines and lines
and millions of lines of code that has
those if statements in there now it's
interesting because what that means is
we've been implicitly taking human
decisions that a programmer or a
policymaker made it and code it into
code now with this new sort of AI based
systems both both the previous sort of
supervised learning and unsupervised and
now with these uh newer algorithms um
these are still probabilistic algorithms
except now the probabilities actually
stare you in the face in a way that
previous systems didn't push right so
previous application systems never came
up with said do you want the 49% the 69%
answer and now you decide whether 69 is
high enough or not right it would
basically would encode it and say great
there's an ARB number 50 and anything
above versus below now why this is
becoming such a debate is because the
probabilities are now surfaced to the
user's face and if they aren't we have
to train people to start thinking about
when the system punches out a number how
do you make a decision on a probability
so I think that that's that's the big
difference between before and now that
we're having to retrain everybody and
why it's become a policy issue all of a
sudden it's because policy makers now
have knob where we now have to decide
explicitly this or that so to me it's
it's it's actually we're in a kind of
the same world but just more an explicit
world is there I mean before we get into
policy specifically like is is there a
sense where like I mean the Advent of
Open Source and then just the general
acceptance of Open Source now does that
make it easier does that ease the
adoption to some open source Tech right
because in an Intel we have the open-
source intell
question I would say open source are
sort of technology and in terms of like
you want to adopt something right so
there's commercial technology not up not
up to power but you know you need to be
able to to remake it in your own image
and and and get something that actually
is actually fun functional for
you um I would love to hear Nan's answer
actually I've got a historic perspective
on this but I'd like to hear your
current perspective so the thing we're
challenged with on on just this whole
landscape right now
is um each of the large each of the
companies is offering right a particular
llm and and to me it's turning into sort
of like different types of wine or
different varietal and I'm not a a wine
snobber or know why that well but I'm
imagining it's it's it's it has
something the lineage and the you know
the data Exquisite data this one was
grown in the hills of you know Normandy
and this one comes from you know you
know this part of Italy you're saying
it's all
nonsense exactly ultimately ultimately
right so so you're getting these llms
with these you know different lineages
and different vintages and different
data you know Etc and uh each of these
behaves you know these systems are going
to behave very differently over time
right uh now the question is you know
the the going big problem of like Well
everybody's going to train on the whole
internet so everything's just going to
get look the same right this versus that
but I think there's a second question
that we're having to to to do is um the
open source Fe and this is where the
open source question comes in is the
ability to start training with your own
data and the question becomes do I take
a base algorithm or system that somebody
has built that by the way has ingested
the entire internet which both good and
garbage that has been ingested in and
now I use that as a base platform and it
may have a certain set of biases
that it brought along with the garbage
and Cesspool that the internet is and
all of a sudden now I'm I'm using that
as my base or do I want my pristine you
know
handcrafted uh you know sort of made in
in in in uh uh in bespoke fashion thing
that's where the availability of these
algorithms becomes really interesting
however it has the base the the opposite
problem of it doesn't have the imperatur
and the stamp of a large company that
has the experence building large
software systems and training and
verification other pieces so we then
have to sort of know and understand the
stuff ourselves and do all that work so
I think that's a trade-off that we're
dealing with yeah yeah yeah yeah that
makes a ton of sense here here's a bit
of a here's a bit of a historical
perspective on this which is similar to
what I touched on before which is um you
know like in my experience the
government the intelligence agencies
have to solve problems that like just
that market forces don't really solve
for in order to do that there has to be
some sort of you know flexibility
programmability and open source has
always been like a a key component of
this right I mean quite famously SE
Linux came out of the NSA you know and
they use kind of Linux to do because
they required kind of like whatever and
like there's been a number of chaining
to to algorithms like um like crypto
right like like the I don't remember the
details but remember like the NSA was
like oh like quadratic linear
programming will break this so go ahead
and do this kind of change in the
algorithm then it's better I mean so
these are types of things that have been
coming out of the agencies for
intelligence for quite a while now I
remember 20 years ago when I was in the
depths kind of working on one of these
problems and there's kind of an Oldtimer
there and he he goes to me he's like man
he was a tech Oldtimer he's like it's so
great you have this open source because
we can work with it he says I remember
in the time when all we would get was
supercomputers and they would come out
of IBM or come out of SDI or come out of
ksr or whatever it is we'd buy one of
everything but like we only got what
like the vendors created um and
something that I do think about it be
great to hear perspective Nan which is
it's one thing to open source weights
and biases that's one thing but these
are the largest compute projects that H
like mankind has ever done before like
we've never done anything close to this
and so even if the weights and biases
are open source I don't know how much
you can modify it right so it almost
feels like we're going back to this old
Mainframe day where like it's great to
have it and you can operationalize it
but you're not going to have the same
level of flexibility as you know you
have with like traditional software at
least this would be my guess no AB
absolutely
um have you're totally right the the
weights and biases are anybody's ability
to do something with that those with
that information is limited because it's
not just the numbers you have to have
the expertise you need to compute you
need all the other pie how many these
training runs are hundreds of millions
that's my point which is sure great I I
have that information I just don't have
the compute Cycles to be able to do
anything with it right or or modify it
or change it so you're totally right it
it will help it with verification it'll
help with training testing it'll train
help with all that stuff that's fine but
you're totally right feel like we're
going back into this kind of almost
super computer but which which by the
way the government is the best at like
100% the best at procurement using I
mean there's a long history of that but
it's a very different it it's a the ball
game is completely different um the
other piece by the way with open source
that we have to deal with a lot by the
way is the uh is the supply chain issues
of course yeah right so
um just you know and this is a whole new
level of paranoia that you need to have
working in a spy agency is it's one
thing for your program to not run or you
know some some e-commerce customer
having a bug or something uh these
issues are are still again also really
really important for us I don't ask so
so why does that look like that if you
get back if you make the supercomputer
te analogy right like what does a new
let's say public private DC Silicon
Valley partnership look like in terms of
like actually implementing and
operationalizing AI models and AI
systems so I I'll take a shot at that
because it's something that uh that
we're well I think the US government is
thinking about this at large is so
um uh when you go talk to universities
right now one point they make is they
don't have the compute power to be able
to rival Microsoft and open Ai and these
companies which is mindboggling right
because to your point the supercomputer
systems and everything I I when I was at
Cornell we remember we had a
supercomputing site on campus with money
that you know the government had put put
in place to have these supercomputing
centers right Illinois had one uh
Cornell Etc and we don't have that
equivalent now so now the National
Science Foundation I think some of the
uh money that the government's allocated
I'm assuming has been is going towards
building these large scale or not or the
opposite so we're not ay making
organizations actively trying to keep
people from building them I think there
needs to be this model of it has to come
out from uh you know away from just
corporate uh organizations doing this to
you know and and you know we're part of
government so it's a different thing but
the question is is whether this needs to
get sort of opened up in a bigger way I
mean so so prior to being in the so the
thing that brought me into the
intelligence Community is I was doing
computational physics on supercomputers
at a National Lab in the weapons program
when 911 happened right and they're like
you have all the clearances like you
need to move like to like this kind of
new area and they moved me to the
intelligence community and I learned a
bunch of stuff that way um you know I I
will say
um the work that we did on those super
computers the government was the best it
created entire new disciplines of of of
you know scientists and career
Professionals in universities it leaned
totally into this and that's why we
maintained a leadership position in the
world through that and in in in compute
and we still do um I do think that it's
a risk that we don't take the same
attitude like I don't listen I'm a VC
here and I'm saying I don't think the
private markets solve everything I do
believe in like public private
Partnerships I do believe in
institutions I do believe that the
government has a big role to play here
but I think that role to play is
investing heavily in in people and Tech
and careers and reaching out and my fear
is that they're kind of doing the
opposite where is in my time you know my
time but back back in the 90s was
exactly what you said I mean I mean I
went to like Northern Arizona University
Small Mountain School and we had like
asy program where they would come out
and kind of like invest in us and I just
feel that now even though we have this
new technology it's very powerful and we
are the leader and it came from the
United States instead we're kind of
pulling back from it and so I do think
that this is a moment of kind of you
know I think that the US has to kind of
take pause and and and and understand if
are we undergoing a Doctrine change
where when new technologies come we run
away from it instead of towards it and
you know I think it's a it's a real
quandry yeah it does seem like that like
may maybe there was a shift at some
point too maybe it was the internet
internet I'm not sure where like in the
supercomputer days yes like you you
bought a system from IBM or SGI whoever
was selling
this and but that was a hardware system
right running some very specialized
software but like today yes everything
comes out of these huge companies that
have access to all the data and all the
computing power and like I don't know if
that how how that affected like the the
pow shift or whatever but is is there a
way to like I mean do you do you sense a
way to bridge C does it and can I like
well go ahead yeah I mean so so here's
the other side of the argument which is
the very dynamics that have led us to
this point of creating these algorithms
and and these systems these
breakthroughs uh there's also hundreds
of companies that you you and other VCS
are funding hacking away at the problem
to make these things available right
there's huge amounts of work going on in
AI specific chipsets uh both on the
training side and the inferencing side
um whole bunch of algorithmic changes
that are going to happen that refactor
these algorithms to do better job in
terms of scaling and being able to
shrink them without a loss of a dramatic
loss of performance so again we're such
in the early stages and innings of this
game that we don't know what the next
five years is going to bring but for
sure you've got thousands of really
really smart people hacking away at the
problem that I think will come to some
medium where yes hopefully the
government or maybe the government funds
or Academia ends up with these large
compute places to be able to rival um uh
commercial and at the same time the
availability of you know hardware
commoditization and other pieces will
get to a point where we'll be able to
run all kinds of interesting algorithms
at scale with really cheap readily
available Hardware right so that's The
Optimist that's a sort of techno
Optimist aspect of it which is you know
as I say life finds a way which is if
there's demand for it people will supply
it yeah yeah it just it just the
question is is will will the the the
private markets solve the problems
needed for things like Global defense or
like you know national security stuff
like that and just
historically the the government has
played a role in innovation in training
I mean you know like think about like
nuclear Engineers like a lot of this
actually came up from government
programs so this is a different slightly
different topic is this idea of what are
we doing in government to do a better
job of working with industry right and
that so uh a large portion of this job
that I have is this idea this new idea
of you know well it's American dynamism
it's this idea of Silicon Valley leaning
in and the government having to lean in
together for us to meet in the middle to
be uh you know a supp better supplier
and a great customer so in my role at
the agency one of the big areas of Focus
for us is how do we become a better
customer you know a dramatically
different customer I spent two and a
half years at the Pentagon which was its
own gigantic problem in terms of that's
where software Define Warfare ideas came
and in all honesty we don't we don't do
a great job in certain things where we
could be world class or better and we
are working really really hard to change
that so for instance
um there is no as we point out in inside
many cases there is no ready app store
for Spy software so there are absolutely
certain things that we need to build and
right inside the agency that's very
specific it's also our competitive
Advantage right which is we're not going
to be buying this stuff that's readily
available for everyone we have our
secret sauce we build it it's our
competitive Advantage however what we
don't do sometimes is analyze it we take
that too far which is there's stuff
that's readily available outside from
commercial land that we don't think
think about buying deploying and
implementing at scale and in the past
year and a half we have actually spent a
lot of I've personally spent a lot of
time focusing on what I call Commercial
first right is this idea that we need to
be rethinking our strategy that if
something is available on the outside
how we bring it in however we have
procurement processes we have atto
processes we have security processes
that don't lend themselves well for
Rapid acquisition in pieces so we're
trying to hack away at those on top of
it the other issue is that the security
needs and requirements to run the stuff
on the high side is very expensive and
for commercial vendors to provide and go
through that process is expensive and an
investment and so we have to create
incentive structures to be able to bring
them in so it's not a it's not simply we
can will it into into existence but it's
a systemic problem that we're trying to
attack and and and hack away at um
there's certain things that have been
big breakthroughs inside the agency over
the past year and a half I can say
probably can't tell you what those are
but we've made huge huge progress in
rethinking and other other ways there
and as an agency you know there's a
number of cultural shifts that we're
going through internally right so I
actually before even the S wrote up kind
of like a couple of these sort of like
this versus that so I'll throw a bunch
of this so first is the human versus
Tech right is this idea that as a human
intelligence organization it's very
interesting because of the changes in
Tech outside that our case officers and
we operate in right we're publicly talk
about what we call ubiquitous technical
surveillance right UTS video cameras
Biometrics Etc so we as a spy agency
hate Tech when it's applied against us
but we also wield it right so it's that
aspect the other interesting cultural
thing in the agency that's been
fascinating is the the power of the
individual which is we train individuals
to uh go do heroic efforts and things
which is part of our our that that's our
job right that's the the agency's job is
to go into foreign countries and and spy
the the applying Tech what is the big
change in Tech that we've seen over the
past couple of decades scale and so this
idea of the individual versus scale is a
cultural thing that we're trying to
rationalize right is applying Enterprise
large- scale Tech to an an organization
that teaches individuals to take
basically have agency to go do things so
that's a very interesting one the
short-term versus longterm which is the
idea of you know us being an agency
that's ready to go at a moment's notice
which the agency does incredibly well
but again to do large scale Enterprise
Wide Technology Transformations and
things takes takes time uh it's the uh
open versus sort of clandestine which is
as an agency our um folks AR not trained
to be out there in public and director
Burns has has made this a big priority
in terms of Engagement with the outside
world engagement with the technology
industry which happens out in the open
right it's it's it's stuff the idea of
carrying a business card and being
present and being on podcasts these are
all culturally new things for the agency
to deal with so we ourselves are going
through this huge huge transformation
dealing with tech how do we actually
change the thinking in this new world uh
and then the AI stuff on top of it which
then is another layer of complexity in
terms of changing how we operate what we
do in the discipline so it's a it's it's
a very interesting exciting but also
somewhat you know uh confusing and
transformational time for for for for
the for the agency do you think that I
mean given so American dynamism is
having a moment right now right the you
know DC and Silicon Valley is going to
be talking at least maybe I don't know
if more than ever certainly not since
the early days but like you know people
are talking like you guys both have both
Intel and software experience startup
experience intersection right government
Tech yeah does that do you think that
starts to help maybe ease some of the
friction in terms of I don't know
whether it's making procurement process
is faster making adoption a little
easier making it easier to kind of hack
on stuff and you know just have a
startup type mentality like yeah so
maybe I'll I'll I'll say something that
it would be great if you took us home
which is um the issues you just talked
about have been around for a very long
time and I don't think that's the hirer
bit right like of course we can make
procurement better of course we can make
communication better of course we can
have better public private partnership I
remember talking about this whenever in
the 2000s in the 90s Etc here's what I
think is the most important and you
alluded it to it before and I think it's
so important which is um the internet
caught the US flat footed a bit um there
was this notion of
asymmetry um it ended up having
exponential effects because there's so
much you know kind of activity and so
when it came out it took us a while to
come to griffs with it and what I fear
my biggest fear is with
AI people are fighting the last war and
it's to our detriment which is which is
a lot of things people are concerned
about with AI were really internet
things and we've kind of gotten on top
of so you can't take that mindset you
can't take this mindset of you know
investing in this stuff is bad because
it's asymmetric you can't take the
mindset of like this is inherently
exponential you can't take the mindset
of like this is core critical
infrastructure because it's just very
very different this is a new type of
Technology it's as useful for us for
doing good as it is for changing the
thread environment which I actually
don't think it changes the threat
environment a lot and so I think that
both the government and Us in Industry
need to come together and acknowledge
this is a you know a new technology
that's beneficial and then we're better
learning about it than running away from
it and we can't take these old lessons
from the internet and somehow kind of
roote apply them because then we're
going to miss the train and so for me
this is kind of like the the higher bit
the most important thing and then a lot
of the things you've talked about are
important but they will kind of follow
in due
course wow I'm not sure I'm going to top
that uh and and I I and I and I do
actually have to be careful in the sense
that again sort of the uh the big
disclaimer I have to patch on this is
you know CIA needs you know we're a
we're not a policymaking shop our job is
to support policy makers with very
objective uh you know by the book
analytic support on the questions that
they're asking what Martine I think just
just outlined is exactly the
policymaking debate and discussion going
on is this idea of how do you how do you
regulate versus incentivize right
because I think the thing is is
that
uh you know what happened with things
like privacy and security and other
things uh has had has impacted consumers
and therefore it impacts lawmakers and
so we got pulled into leaning and and
you know now along with that whatever is
happening in that area right um the
issue around 5G right was a big big
National Security concern all of a
sudden uh then all of a sudden now the
chips act in terms of
semiconductors uh look at what happened
in crypto and now ai so so I'm l in them
because at CI we
track you can list 5 10 15 you know
there there's all these emerging Tech
areas that we follow now and we've got
World leading domain experts that follow
each emerging Tech
area uh because again there's demand and
support uh support from downtown on on
those questions Okay so we've I listed
what four um four and then the fifth one
is privacy security regulation around
social media Etc so these five areas of
Technology now have been you know
there's a spotlight on them uh to
Martin's Point where it lands and ends
up that's purely a policymaker domain
our job though in that's tricky in many
of these situations is that these are by
definition emerging Tech areas I mean 5G
and semiconductors are scaled uh
Industries but the rest of these
industries are emergent
Industries and emergent Industries is
having been in the industry in startups
you know we don't know where this
stuff's going to land and so all of a
sudden becomes really hard to understand
who to talk to who to believe uh do we
forecast do we not forecast uh where we
think it's going to land and there are
no solid answers to any of these
questions because every six months it's
going to be something new and so how do
you build policymaking on top of
emerging Tech areas that is an art and I
don't know how I mean again it's up to
law makers and policy makers to figure
this out which is where then you end up
with things like for instance executive
orders rather than laws right it's very
interesting how the policy-making
apparatus Works where you know you you
end up with 100 pages of executive order
stuff that outlines generally some ideas
and thoughts and questions and I think
there's going to be this leaning in and
convergence that happens between
industry and regulators and stuff
because this stuff's moving the tech is
moving policy makers are learn learning
more they learn more they ask more
questions the tech industry moves this
way so it's an iterative long-term
process it's up to the players including
you know the uh the folks with the money
and the investors uh having a seat at
the table to to play this uh the good
news is we the agency is playing very
much a you know we're friends with
everyone we talk to everybody that's our
job gather a lot of intelligence analyze
it hopefully with AI
and then help our policy makers sort of
create this we very much appreciate what
you do and and thank you for that thank
you now if you have made it this far
don't forget that you can get an inside
look into a16z American dynamism Summit
at
az.com Summit there you can catch
several of the exclusive stage talks
feing policy makers like deputy
secretary of defense Kathleen Hicks or
Governor Westmore of Marland plus both
Founders from companies like andril and
coinbase and funders like Mark all
building toward American dynamism again
you can find all of the above at
az.com admit and we'll include a link in
the show
notes
Weitere ähnliche Videos ansehen

Georgia L. Anderson, Rory Sutherland, Stephan Pretorius and Antonis Kocheilas – AI | Nudgestock 2024
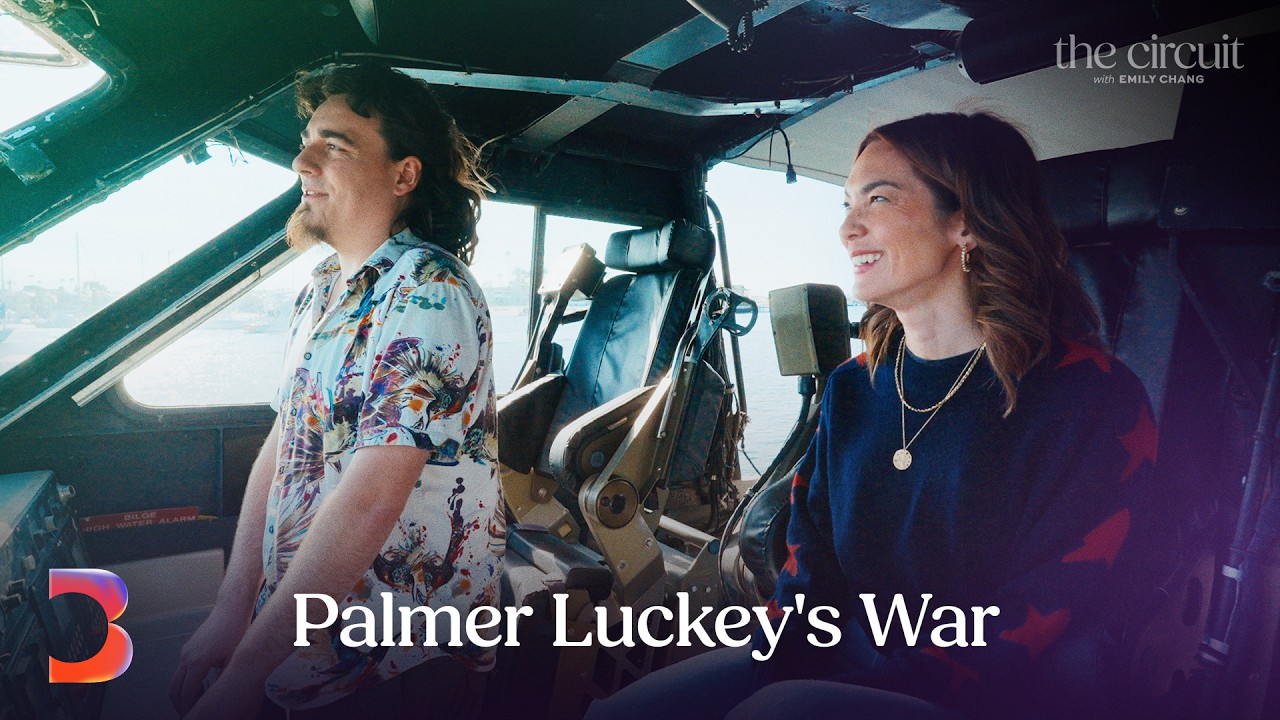
Palmer Luckey Wants to Be Silicon Valley's War King | The Circuit
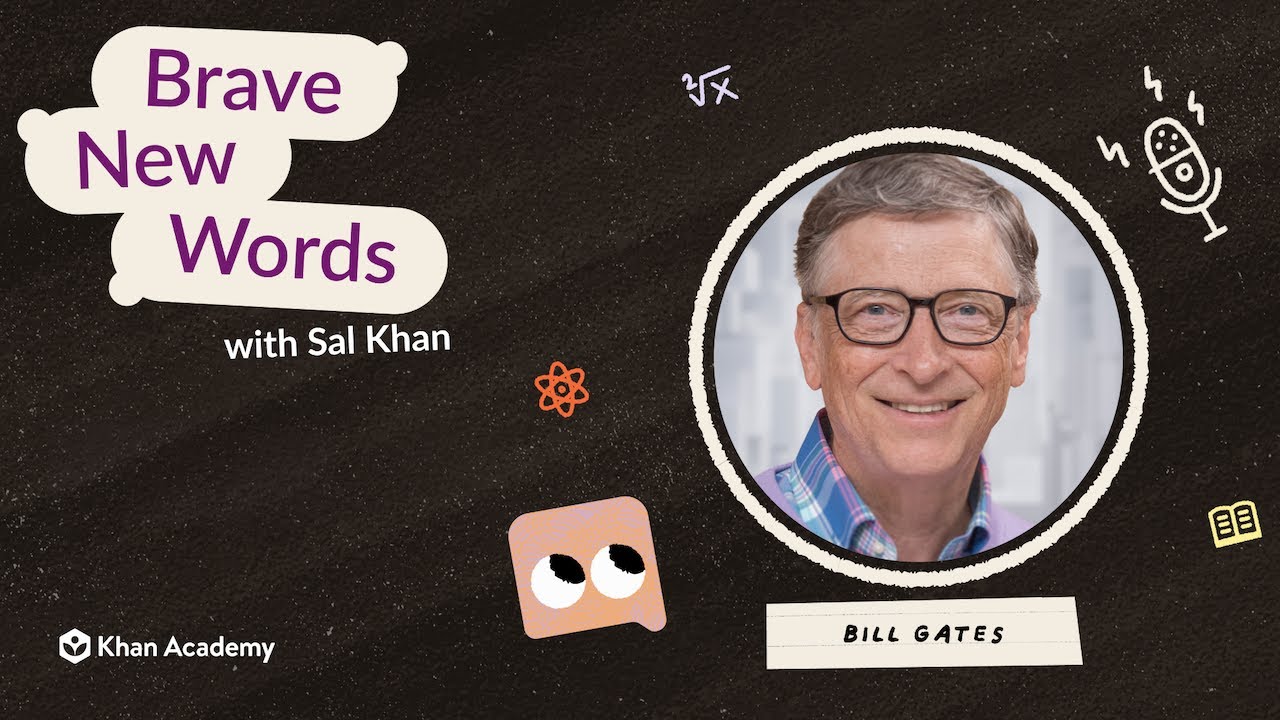
Brave New Words - Bill Gates & Sal Khan

About 50% Of Jobs Will Be Displaced By AI Within 3 Years
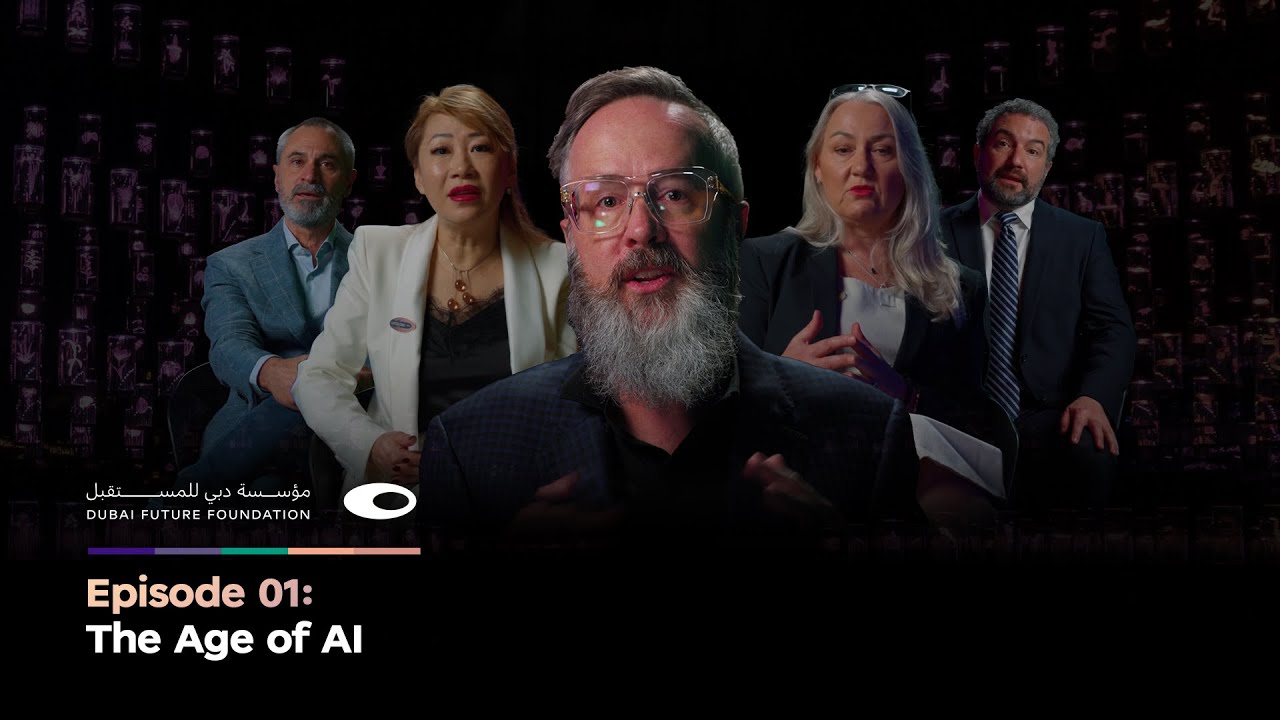
Ep. 01: The Age of AI I Docuseries: What Does the Future Hold ? - Season 2
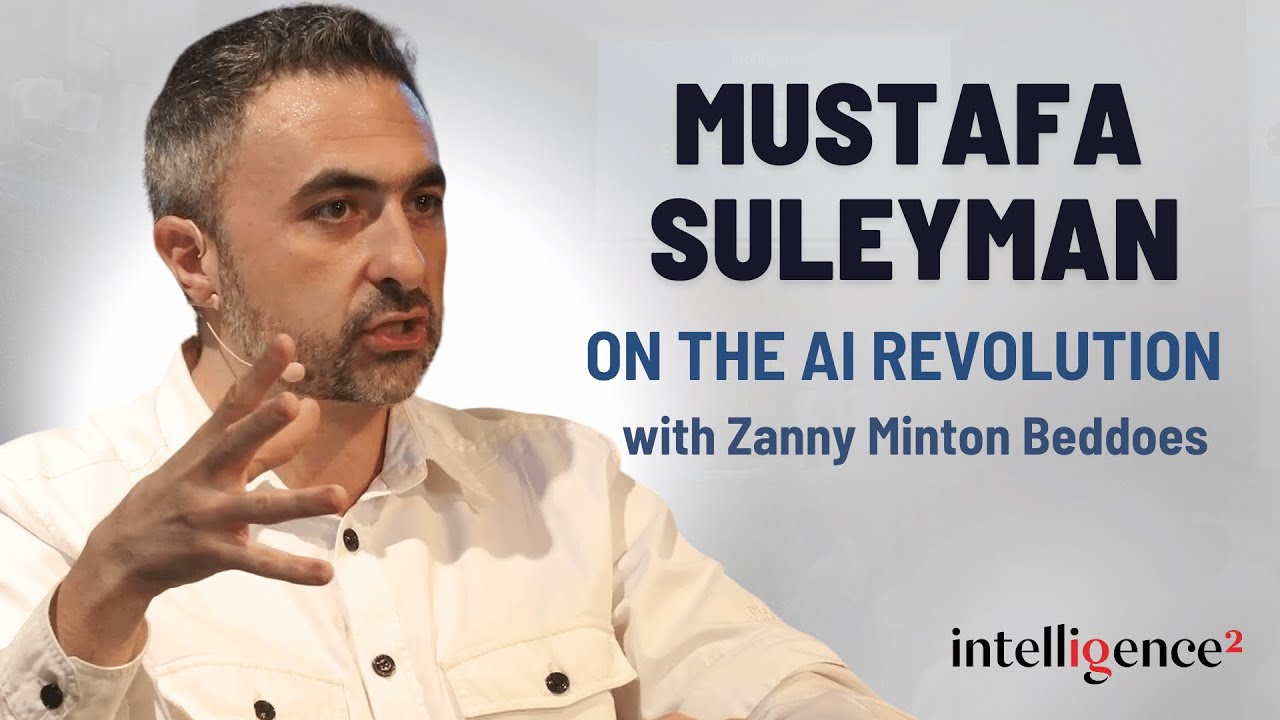
Mustafa Suleyman on The Coming Wave of AI, with Zanny Minton Beddoes
5.0 / 5 (0 votes)