ニューラルネットワークの性能を決定づけるデータの量と質
Summary
TLDRThis video script by Sony's Kobayashi discusses the pivotal role of data quantity and quality in determining the performance of deep learning technologies. It outlines the three key steps in developing intelligent functions: preparing a dataset, designing a neural network architecture, and training the model. The script emphasizes that while neural network architecture can be reused or optimized, data must be specifically curated for each function. It also highlights the direct correlation between data volume and deep learning performance, suggesting that more data leads to better performance without a ceiling, contrary to traditional machine learning approaches. The script concludes by stressing the importance of both data volume and quality in achieving high performance in deep learning applications.
Takeaways
- 🧠 Deep Learning Performance: The script discusses how the performance of deep learning technologies is determined by the quantity and quality of data, emphasizing the importance of data in the development of intelligent features.
- 📈 Data Quantity and Quality: It highlights that both the amount and the quality of data are crucial for deep learning, with more data leading to better performance without an apparent ceiling.
- 🔍 Data Needs: The script mentions the need for a large and diverse dataset to train neural networks effectively, comparing it to human learning through various experiences.
- 🛠️ Neural Network Architecture: The architecture of the neural network is a key factor in performance, alongside the dataset, and can be improved using various techniques or automated exploration.
- 📚 Data Preparation: The process of preparing the dataset, such as collecting pairs of input images and their classifications, is a foundational step in developing deep learning models.
- 📈 Data Scale Impact: The script provides evidence that deep learning performance scales linearly with the logarithm of the data amount, with no visible limit even at 3.5 billion images.
- 🌐 Data Growth Rate: It points out that the world's data volume is growing exponentially, suggesting that deep learning performance will continue to improve as more data becomes available.
- 🔧 Data Quality Considerations: The quality of data is multifaceted, including factors like diversity, noise levels, and whether the data is representative of the real-world distribution.
- 🔬 Data Evaluation: The script suggests evaluating the quality of data by whether humans can make accurate judgments from it, as a benchmark for surpassing human performance.
- 📉 Data Overhead: It notes that while higher resolution data can improve performance, it may also lead to increased computational requirements, necessitating a balance between resolution and practicality.
- 📝 Data Collection Strategy: The amount of data needed depends on the desired performance level and the complexity of the problem, with the script suggesting starting with a proof of concept and then scaling up.
Q & A
What is the main topic discussed in this video script?
-The main topic discussed in this video script is the importance of data quantity and quality in determining the performance of deep learning technologies.
Why is the amount of data important for deep learning models?
-The amount of data is important for deep learning models because it directly correlates with the performance of the model. More data allows for the model to learn more effectively and achieve higher accuracy.
What is the role of data quality in deep learning?
-Data quality is crucial in deep learning as it ensures that the data is representative, diverse, and free from noise and errors, which can significantly impact the model's performance.
What are the three steps involved in developing intelligent features using deep learning?
-The three steps involved are: 1) Preparing a dataset for training the neural network, 2) Designing the neural network architecture, and 3) Training the network using the prepared dataset.
How does the performance of deep learning models change with the increase in data quantity?
-The performance of deep learning models tends to improve linearly with the increase in data quantity, with no apparent ceiling even with extremely large datasets.
What is the significance of the graph mentioned in the script that shows classification accuracy versus the number of images?
-The graph is significant as it visually demonstrates the direct relationship between the amount of data used for training and the classification accuracy achieved, indicating that performance scales with data quantity.
How does the increase in the world's data volume contribute to the advancement of deep learning techniques?
-The increase in the world's data volume provides more data for training deep learning models, which is a key factor in the continuous improvement of their performance.
What are some of the techniques used to achieve high performance with limited data?
-Techniques such as data augmentation, transfer learning, and semi-supervised learning are used to achieve high performance with limited data.
Why is it necessary to consider both the quantity and quality of data when developing deep learning models?
-Both the quantity and quality of data are necessary because they jointly influence the model's ability to learn effectively and generalize well to new, unseen data.
What is the recommended approach when deciding on the resolution of data for deep learning models?
-The recommended approach is to collect data at the highest possible quality within the constraints of storage and computational resources, as higher resolution data can be downscaled if necessary but cannot be upscaled without loss of quality.
How can one estimate the required amount of data to achieve a certain performance level?
-One can estimate the required amount of data by first collecting a small dataset, training the model, and then incrementally reducing the data quantity while evaluating performance. This process helps to understand the relationship between data quantity and model performance.
Outlines
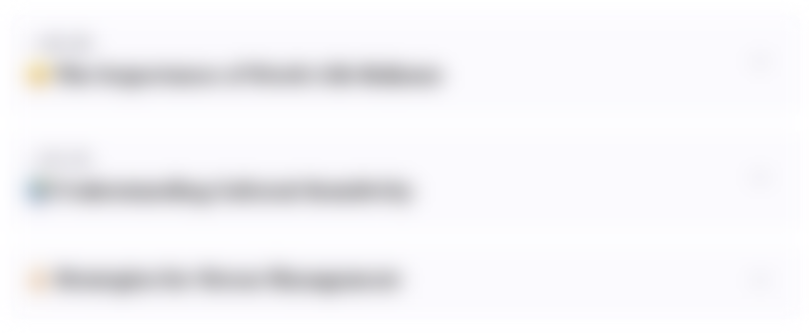
Dieser Bereich ist nur für Premium-Benutzer verfügbar. Bitte führen Sie ein Upgrade durch, um auf diesen Abschnitt zuzugreifen.
Upgrade durchführenMindmap
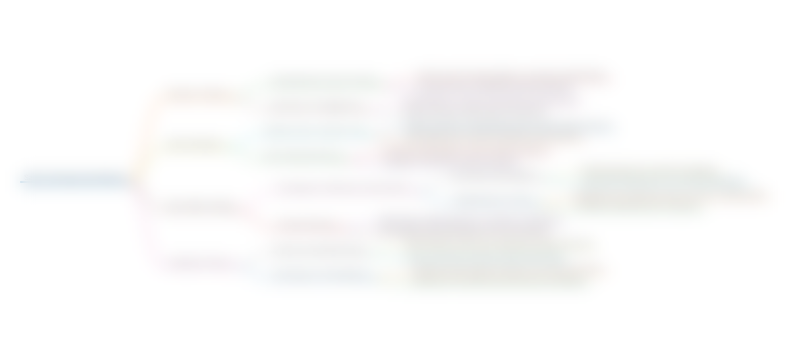
Dieser Bereich ist nur für Premium-Benutzer verfügbar. Bitte führen Sie ein Upgrade durch, um auf diesen Abschnitt zuzugreifen.
Upgrade durchführenKeywords
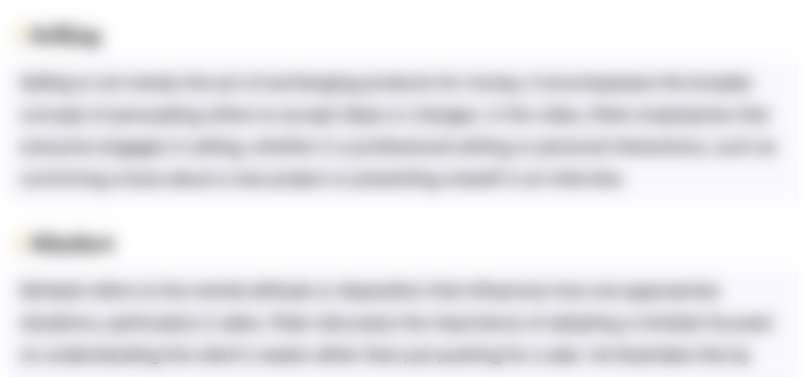
Dieser Bereich ist nur für Premium-Benutzer verfügbar. Bitte führen Sie ein Upgrade durch, um auf diesen Abschnitt zuzugreifen.
Upgrade durchführenHighlights
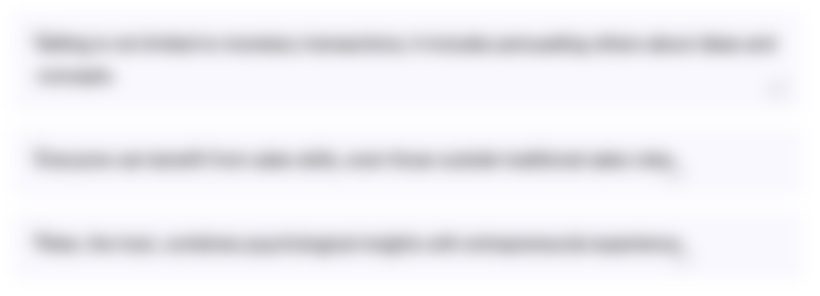
Dieser Bereich ist nur für Premium-Benutzer verfügbar. Bitte führen Sie ein Upgrade durch, um auf diesen Abschnitt zuzugreifen.
Upgrade durchführenTranscripts
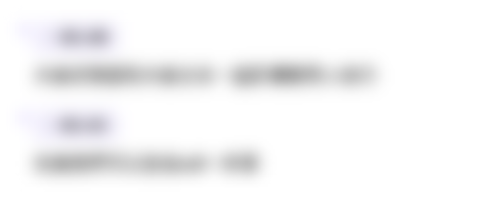
Dieser Bereich ist nur für Premium-Benutzer verfügbar. Bitte führen Sie ein Upgrade durch, um auf diesen Abschnitt zuzugreifen.
Upgrade durchführen5.0 / 5 (0 votes)