Tutorial 34- Performance Metrics For Classification Problem In Machine Learning- Part1
Summary
TLDRتشرح القناة اليوتيوبية في هذا الفيديو عن معايير التصنيف في مسائل التصنيف، ويغطي الفهرس الخلطي والدقة والأخطاء النوع 1 والنوع 2، والاسترجاع والدقة والF beta. سيناقش القناة في الأجزاء القادمة المزيد من المعايير مثل ROC المنحنى وAUC النتيجة والمنحنى PR، ويشرح التطبيق الفني في الجزء الثالث مع بيانات مكتوبة.
Takeaways
- 📊 الemetrika في مشاكل التصنيف: النص يناقش مجموعة واسعة من الemetrika التي يمكن استخدامها لتقييم الخوارزميات الذكاء الاصطناعي في المشاكل التصنيفية.
- 🔍 الفهرس الخلطي: يشرح النص مفهوم الفهرس الخلطي ويشرح القيم الحقيقية والمتوقعة والعلاقات بينها.
- ✅ الدقة: يناقش النص كيف يتم حساب الدقة في المشاكل التصنيفية وكيف يعتمد على القيم ال对角 في الفهرس الخلطي.
- 🔺 الأخطاء النوع 1 والنوع 2: يحدد النص الأخطاء النوع 1 (falase positive) والأخطاء النوع 2 (falase negative) وتأثيرهما على الدقة.
- 🔍 التذكير: يشدد النص على أهمية فهم الemetrika الصحيحة للتقييم الصحيح للمودل الذكاء الاصطناعي.
- 📉 التوازن في المجموعة البيانات: يناقش النص الفرق بين مجموعات بيانات متوازنة وغير متوازنة وتأثير ذلك على الemetrika المختارة.
- 🔎 الذاكرة (Recall): يشرح النص معنى الذاكرة وتوضح كيف يعتمد على عدد القيم الصحيحة المحددة بشكل صحيح.
- 🎯 الدقة (Precision): يناقش النص الدقة ويشرح كيف يعتمد على نسبة القيم الصحيحة من بين القيم المتوقعة بشكل إيجابي.
- 🤖 F beta: يشرح النص مفهوم F beta ويشرح كيف يجمع بين الدقة والذاكرة لتقييم المودل بشكل متوازن.
- 📈 الاستخدام الصحيح للemetrika: يشدد النص على ضرورة اختيار الemetrika الصحيحة بناءً على أهمية الأخطاء الfalase positive والfalase negative في ال.problem.
- 👨🏫 تطبيق الemetrika: يوعد النص بتطبيق الemetrika التي تمت مناقشتها في حل مشكلة تصنيف مع مجموعة بيانات غير متوازنة في الجزء الثالث من الفيديو.
Q & A
ما هي المعايير التي تناقشها في هذا الفيديو؟
-يناقش هذا الفيديو مجموعة من المعايير التي تتضمن مصفوفة الخلط، دقة، خطأ النوع 1، خطأ النوع 2، تذكر (True Positive Rate)، دقة (Positive Prediction Value)، وF beta.
ما هي الفرق بين الدقة والتذكر؟
-الدقة تشير إلى عدد النتائج الصحيحة الإيجابية من بين النتائج المتوقعة الإيجابية، بينما التذكر تشير إلى عدد القيم الصحيحة الإيجابية التي تم توقعها بشكل صحيح من بين جميع القيم الفعلية الإيجابية.
لماذا لا يُنصح باستخدام الدقة لتقييم النماذج في المجموعة غير التوازنة؟
-في المجموعة غير التوازنة، يمكن أن تؤدي الدقة إلى تقييم خاطئ للنموذج، حيث يمكن أن يتوقع النموذج جميع الإدخالات كفئة واحدة، مما يؤدي إلى دقة عالية ولكن لا تعكس الأداء الحقيقي للنموذج.
ما هي مصفوفة الخلط؟
-مصفوفة الخلط هي مصفوفة 2x2 تستخدم في التصنيف لتحديد عدد القيم الصحيحة الإيجابية، القيم الصحيحة السلبية، القيم الخاطئة الإيجابية، والقيم الخاطئة السلبية.
ما هو F beta النتيجة؟
-F beta النتيجة هي معيار يجمع بين الدقة والتذكر، ويتيح تحديد الأهمية النسبية للأخطاء الخاطئة والأخطاء السلبية استنادًا إلى قيمة beta، التي يمكن أن تحدد الأهمية المرجوة للأخطاء الخاطئة والأخطاء السلبية.
كيف يمكننا استخدام معيار F beta لتحسين تقييم النموذج؟
-يمكننا استخدام معيار F beta لتحسين تقييم النموذج عن طريق ضبط قيمة beta بناءً على الأهمية النسبية للأخطاء الخاطئة والأخطاء السلبية في المسألة المحددة، مما يتيح لنا الحصول على معيار يعكس بشكل أفضل أداء النموذج.
ما هي الفرق بين الأخطاء النوع 1 والأخطاء النوع 2؟
-أخطاء النوع 1 هي الأخطاء الخاطئة الإيجابية، التي تعني توقع أن العنصر هو من فئة معينة عندما يكون في الواقع من فئة أخرى. الأخطاء النوع 2 هي الأخطاء الخاطئة السلبية، التي تعني توقع أن العنصر ليس من فئة معينة عندما يكون في الواقع من تلك الفئة.
لماذا يُنصح بتقليل الأخطاء الخاطئة الإيجابية والأخطاء الخاطئة السلبية في التصنيف؟
-تقليل الأخطاء الخاطئة الإيجابية والأخطاء الخاطئة السلبية يساعد على تحسين دقة التصنيف وتقليل التأثير السلبي للأخطاء في القرار النهائي، مما يؤدي إلى تحسين أداء النموذج بشكل عام.
ما هي الأهمية من استخدام ROC المنحنى والنقاط AUC في التصنيف؟
-ROC المنحنى والنقاط AUC هي معايير تحليلية تساعد في تقييم القدرة على التمييز بين الفئات المختلفة في التصنيف، مما يوفر نظرة شاملة على أداء النموذج في مختلف القيم العتبة.
ما هي الخطوات التالية التي سيتم تغطيتها في الفيديوهات القادمة؟
-في الفيديوهات القادمة، سيتم تغطية معايير Kohan Kappa، ROC المنحنى، نقاط AUC، PR المنحنى، والمزيد من المعايير التي لم يتم تغطيتها في هذا الفيديو.
Outlines
📊 Introduction to Classification Metrics
Krishna introduces the video series focusing on classification metrics in machine learning. He outlines the importance of using the right metrics to evaluate a model's performance and mentions that incorrect metrics can lead to poor model performance in production. The video will cover confusion matrix, accuracy, type 1 and 2 errors, recall, precision, and F-beta score. The series will also discuss advanced metrics like Cohen's Kappa, ROC curve, AUC score, and PR curve in subsequent parts.
🔍 Understanding Confusion Matrix and Accuracy
The paragraph explains the concept of a confusion matrix in binary classification and its components: true positive, false positive, false negative, and true negative. It discusses the importance of reducing type 1 and type 2 errors. The paragraph then delves into calculating accuracy for balanced datasets, emphasizing that accuracy may not be a reliable metric for imbalanced datasets due to the potential for biased predictions.
📈 Dealing with Imbalanced Datasets
Krishna addresses the challenge of imbalanced datasets, where one class significantly outnumbers the other. He explains that accuracy is not a suitable metric in such cases and introduces recall, precision, and F-beta score as more appropriate metrics. The paragraph discusses the importance of these metrics in evaluating model performance when the dataset is skewed.
🛠 Precision and Recall: When to Use Them
This section provides a detailed explanation of precision and recall, including their formulas and significance. Krishna uses the example of spam detection to illustrate the importance of precision in minimizing false positives. Conversely, he uses the example of cancer diagnosis to highlight the critical nature of recall in avoiding false negatives, emphasizing the need to choose between precision and recall based on the specific impact of false positives and false negatives in a given problem.
🎯 The F-Beta Score: Balancing Precision and Recall
Krishna introduces the F-beta score as a way to balance precision and recall, especially when both false positives and false negatives are significant. He explains the formula for the F-beta score and how the beta value can be adjusted to emphasize either precision or recall, depending on the problem statement. The paragraph also discusses the selection of beta values and the scenarios in which they are applied.
🔚 Conclusion and Future Content
In the concluding paragraph, Krishna summarizes the video's content and previews upcoming topics in the series, including Cohen's Kappa, ROC curve, AUC score, PR curve, and additional metrics. He encourages viewers to subscribe to the channel and to review the material to fully understand the concepts presented. Krishna also hints at a practical implementation in part 3 of the series to solidify the viewers' understanding of the discussed metrics.
Mindmap
Keywords
💡Metrics
💡Confusion Matrix
💡Accuracy
💡Type 1 Error
💡Type 2 Error
💡Recall
💡Precision
💡F Beta Score
💡ROC Curve
💡AUC Score
Highlights
Introduction to a variety of metrics used in classification problems in machine learning.
Explanation of the importance of selecting the right metrics for evaluating machine learning models.
Discussion on the use of confusion matrix as a fundamental tool for classification problem evaluation.
Clarification of the difference between type 1 and type 2 errors in classification.
Introduction to recall, also known as true positive rate, as a key metric for classification problems.
Definition and importance of precision, also known as positive predictive value, in classification.
Explanation of F beta score as a balance between precision and recall for classification problems.
Introduction to the concept of ROC curve and AUC score for evaluating classification models.
Discussion on the challenges of using accuracy as a metric in imbalanced datasets.
Emphasis on the need for different metrics like recall, precision, and F beta in imbalanced datasets.
Illustration of how to interpret the confusion matrix for binary classification problems.
Explanation of the implications of threshold values in classification, especially in healthcare.
Introduction to the concept of balanced and imbalanced datasets in the context of classification.
Discussion on the impact of dataset imbalance on the bias of machine learning models.
Explanation of how to compute accuracy for balanced datasets in classification problems.
Introduction to the concept of false positive rate and its calculation.
Emphasis on the goal of minimizing type 1 and type 2 errors in classification problems.
Introduction to the F beta score formula and its significance in combining precision and recall.
Discussion on the selection of beta value in F beta score based on the importance of false positives and false negatives.
Preview of upcoming videos covering additional metrics and practical implementation on imbalanced datasets.
Transcripts
hello my name is Krishna and welcome to
my youtube channel now this was one of
the most requested video by you all guys
so in this video we'll be discussing
about all the metrics in a
classification problem statement guys
this is just the part one and I have
listened down all the important metrics
that you can actually use for
understanding whether your machine
learning algorithm is predicting well or
not
okay so some of the metrics of a
confusion matrix okay
then we'll understand about accuracy
then we'll understand about type 1 error
type 2 error
then we have concepts like recall which
is also called as true positive red then
we will discuss about precision which is
also called as positive prediction value
okay
then we'll understand about F beta and
in the next part in the next video we'll
basically be understanding about Kohan
Kappa ROC curve AUC score and something
called as PR curve there are two more
metrics again which will I'll discuss in
the part two because I do not have space
to write it down so we'll just be
discussing about that in our next part -
in the part three I'll try to implement
a problem statement considering an
imbalance data set and I'll try to apply
all this particular matrix and I'll show
you that how the accuracy will look like
so make sure guys you watch this
particular video completely and make
sure that you understand things okay the
reason why I am saying even though you
are a very very good data scientist and
you know how to actually use a machine
learning algorithm with respect to your
data but if you are not using the
correct kind of metrics to find out how
good your model is then it is completely
a waste of time you know because if you
are not selected the right metrics and
then you are deployed your model to the
production right you'll be able to see
that because of the metrics because of
the wrong metrics that you have chosen
you have chosen that will actually give
you a very very bad accuracy again when
the model is actually deployed in the
production so let us go ahead and try to
understand the metrics and in this
particular video I will be making like a
story ok so part 1 will just be like a
story and then I will continue and I
will explain you each and every matrix
Omega now understand one thing that
suppose we have a problem statement so
this is a problem statement specifically
a classification problem statement ok
classification problem statement now in
classification problem statement right
there are two ways how you can solve the
classification problem one way is
basically through class labels suppose
you want to predict class labels ok the
next way is through probabilities
probabilities now suppose I if you let
me just consider a binary classification
in a binary classification I know there
will be two different classes A or B
suppose this is my a or B so my output
will either be a or B ok
by default the threshold value will be
taken as 0.5 what does this basically
mean suppose I am predicting with some
of my machine learning model like
logistic regression by default if I
predict if it is greater than 0.5 then
it would become a B class if it is less
than 0.5 then it becomes a a class I
mean less than or equal to 0.5 then it
would become a a class but in case of
probabilities here we have to also find
out the class labels how we have to
basically select the right threshold
value which is this p value okay and
lots a p value but i instead say some
threshold value in some of the health
care sector this threshold value may
decrease you will be saying that suppose
if a person is having cancer or not at
that time this threshold value should be
chosen in a proper way if it is not
chosen in the proper way
the person who is having cancer will be
missed out right so in probabilities we
will be discussing and in probabilities
what we have we have basically ROC curve
au seeker as a score at PR curve which
we'll be discussing in the part 2 in the
part 1 we'll be focusing more on this
class labels where our default
probability is 0.5 okay so I hope you
are getting it right so understand that
thing if we have a classification
problem usually what we do is that they
have two types of problem statements
over here with respect to the class
labels we need to find out what what is
the output of that particular record or
based on probabilities where we have to
first of all find out a threshold value
okay in logistic regulation I may find
out
the threshold value maybe 0.3 maybe 0.4
that basically means that if my output
is less than or equal to 0.4 it becomes
Class A if my output is greater than 0.4
then it becomes Class B right and this I
will be showing you how you can actually
find out with the help of ROC curve and
P arca okay so that will come in the
part two now let us go ahead one more
thing now over here now with respect to
this problem statement we have two
problem statement based on the output
okay based on the output suppose in in
this particular problem statement I have
thousand records okay
I have thousand records okay now with
respect to thousand records suppose this
is the binary classification problem
that basically means suppose I have 500
yes that basically 500 records which has
a yes as an output and I have 500 no as
my other output or I may have 600 years
or 400 no right now in this case what I
can suggest is that this looks like an
in this looks like a balanced data set
okay balanced data set basically means
that yes you have almost same number of
years and same number of no so both then
the output labels are almost same
similarly if you could take seven
hundred years and three hundred no this
is also fine this looks like a balanced
data set
okay now understand one thing guys why
why I am saying balanced data set over
here the number of years and no are
almost equal in this case you may be
suggesting Chris there is a difference
of four hundred but it is fine why I'll
tell you if we have this kind of data
set also and if we try to provide this
kind of data points to a machine
learning algorithm my machine learning
algorithm will not get biased based on
the maximum number of output okay but if
we have scenarios where in our data set
ranges and this is basically 70 to 30
right 70 to 30 percent basically 70 to
30 ratio here you have basically like a
60/40 ratio here you have 50/50 ratio
right but now if I go one more level
down like 8020 ratio 8020 ratio
basically means that suppose I have over
here 800 record and here I have
200 right now in this case when I
provide this kind of imbalance dataset
to my machine learning algorithm some of
the machine learning algorithm will get
biased based on the maximum number of
output okay now if we have a balanced
data set if we have a balanced data set
the type of metrics that is basically
used is something called as accuracy if
we have an imbalance data set at that
time we do not consider accuracy instead
we consider something called as recall
precision and F beta I'll explain you
about what exactly is a beta score which
is also called as the f1 score if you
have heard of most of it but this f1
score is derived by this beta value that
will it be discussing about now this
consider guys initially let us take that
suppose my data set is balanced okay at
that time I'll try to explain your
accuracy and then we will then
understand if our data becomes
imbalanced how do we solve this
particular problem okay so let us go
ahead I am just going to rub this thing
and if you have not understood just you
know just go back again see this
particular explanation what I've given
okay now first of all if we have a
binary classification problem guys we
need to understand what exactly is the
confusion matrix now understand one
thing guys concision matrix is nothing
but it is a 2 cross 2 matrix in case of
binary classification problem where the
top values are actually the actual
values okay the top values are the
actual values over here actual values
like 0 or 1 1 or 0 suppose I have to
consider this as 1 and 0 and similarly
in the left hand side this all mehar my
predicted values so this basically
values indicates that what my model has
actually predicted so this will also
become 1 and 0 so usually what we do is
that each and every field we specify
with some notations so the first field
in this case is something called as true
positive the second field is something
called as true false positive and the
third field is something called as false
negative and the fourth field is
something called as true negative we'll
try to understand more in depth what
exactly this of these fields mean okay
what is false positive what is false
negative and by default if I consider
this type 1 this is called as
type one error okay
so if I want to consider this false
positive this is basically called as a
type 1 error we we can also compute the
type 1 error with the help of false
positive rate okay false positive rate I
will define the formula in just a while
this FN is basically called as a type 2
error and this is also mentioned like
false negative rate now what is what do
what does this false positive mean now
if you want to define false positive
error how do we do is that we basically
consider this false values with respect
to your actual and predicted and the
false positive rate is basically given
by FB / FP plus TN okay this true
negative and this and always remember
guys these are your most accurate
results okay TP and DL and always your
aim should be okay in any classification
problem to reduce your type 1 error and
to reduce your type 2 error okay you
always have to focus on reducing your
type 1 error and reducing your type 2
error okay now understand one thing guys
since this is a balanced problem
statement right so what we do is that we
directly compute the accuracy now how
does we how do we compute the accuracy
is that we simply add T P plus TN PP +
TN / TP plus FP + SN + TN that basically
means I am just saying that this
diagonal elements which will actually be
giving me the right result divided by
total number of residents and this will
give us the accuracy why I am following
this particular stuff because I'm
considering this problem statement is my
balance data set okay
and during the balance data set usually
my model does not get biased based on
the different types of categories that
we have in this binary classification
problem okay but now what is what if my
data set is not balanced what if my data
set is not balanced let me give you a
very good example suppose one category 1
category I have some one hundred nine
hundred values suppose out of the
thousand records
I have nine hundred one category and one
hundred as my another category okay 100
as my another category now if I apply
just understand over here guys if I just
say that my model will bluntly say that
everything belongs to category A and not
to category B okay not to category B but
instead it will say that every I mean
every values or every touch data set
belongs to my category a instead of
category B okay so out of this I am just
suggesting that okay 900 values and I am
considering this is my touch data
suppose days okay suppose I am having
this test data or just consider that I
had my train data in my train did I had
1500 records out of which 1200 was my a
Class C category and 300 was my Class B
category and now I have divided that
into train - split my in my inside my
test data suppose I had around I'm just
considering an example in my test data I
had Class A as 900 records and Class B
has 100 records now suppose if my model
predicted all the classes over Class A
now in that specific place if I said TP
plus TN I'm just going to get the 90%
accuracy right because they're true
positive I will be getting 900 true
negative I will be getting 0 and if I do
the summation of all of this this will
be some thousand and this is in short
90% dozen of my accuracy right so this
is a problem right if we have an
imbalanced data set we cannot just use
accuracy because it will give you a very
very bad meaning about that particular
model you know you're just blindly
saying that it belongs to just one
category so if you have an imbalanced
data set you basically go with something
called as recall precision right and
something called as FB toughs code now
let us go ahead and try to understand
about recall and precision okay guys now
let us go ahead and just understand what
exactly is recall and precision
now guys here is my confusion matrix
here you have through positive false
positive false negative and true
negative I understand why do we use this
for an imbalanced data set now
understand one thing guys any kind of
data set that you have you should always
try to reduce your type
one error and type 2 error okay you
should always try to reduce this now
specifically when your data set is
imbalanced we should either focus on
recall and precision now what does
recall over here formula says recall
basically says that TP TP so these are
my actual values right these are my
actual these are my predicted values so
this basically says that TP / TP plus FN
okay TP / TP plus FN now what does this
says that out of the total positive
actual values how many values did be
correctly predicted positively okay this
is what this recall basically says again
I am repeating it guys out of the total
actual positive values one is positive
right I can say true or positive
anything out of all this how many
positive did we predict correctly that
is what this recall basically says
recall is also given by something called
as true positive rate it is also
mentioned by true positive rate or it is
also mentioned by sensitivity okay it is
also mentioned by sensitivity now
similarly if I consider about precision
it basically says TP / TP + SB okay now
what does this basically say out of the
total predicted positive result how many
results are actual positives okay here
we are actually focusing on the false
positives here in the record we were
actually focusing on false negatives
right so again I am repeating it what
does precision basically say out of the
total actual positive predicted results
how many were actually positive and what
is the prop proportion that were actual
positive that is what this particularly
precision basically say and for
precision we also specify another name
which is called as positive prediction
value suppose in your interview they
asked you what is actually positive
prediction value you have to explain the
same thing understand this thing guys
now when should we use a recall and
precision understand guys let me just
give you some very good example of
recall and precision so I have a use
case which is called as spam detection
in spam detection I have to focus on
precision why consider that suppose in
this false positive which basically says
that suppose this this mail is not a
spam okay suppose it is mail is not a
spam but the model has predicted that it
is a spam that is your false positive
okay this again I'm telling you guys the
mail is not a spam but it has been
predicted that it is a spam so this is
what it is saying zero basically means
not a spammer
but it has predicted that it is a spam
now in this particular scenario what
will happen is that we should try to
reduce this false positive value we
should try to reduce it false positive
value because I understand in this case
in a spam mail detection if it is not a
spam and if it is specified or predicted
as a spam the customer is going to miss
that particular mail which may be a very
important mail itself right so because
of that we should always try to reduce
this false positive value in the case of
this kind of use case that is spam
detection but what about recall suppose
I say that whether the person is having
cancer or not cancer or not okay so my
one value basically specifies that he is
having cancer 0 specifies that he is not
having cancer now in this particular
case we should try to reduce false
negative
why understand this guys this one now
consider that if the person is having a
cancer actually is having a cancer but
he is predicted as that the person is
not having by the model okay so that is
what say so yeah these are natural
values suppose is he he is suffering
from cancer so that what what exactly
the actual value says right but the
model has predicted that the person is
not having a cancer so this may be a
disaster because I can understand if I
get an error in false positive then the
person will go with another for the test
to understand whether he is having
cancer or not but if my model predicted
that even though he had a cancer but
here so he was predicted as he was not
having a cancer so this is the disaster
at that time we should specifically use
recall now in short guys whenever your
false positive is much more important
whenever your false positive is much
more important go and blind
use precision whenever with respect to
your problem statement if your recall
with your false negative is important at
that time you go and use recall I gave
you an example guys okay cancer whether
a person is having cancer or not some
more examples whether tomorrow the stock
market is going to crash or not some
example of precision spam detection
right consider this particular example
try to think always our aim should be to
reduce false positive and false negative
but whether false in the positive is
playing a greater impact or role in that
specific model if we displayed go and
use precision focus on precision if
false negative is actually playing a
greater role or if greater impact it is
having this go and use recall now I want
to introduce you to something bad as f
beta now sometimes in some of the
problem statements guys false positive
and false negative both are very very
important okay in an imbalance data set
I'm saying okay both will be important
at that time we have to consider both
recall and precision and if you want to
reconsider both recall and precision we
basically use FB does score okay FB does
code and sometimes in some of the
problem statements guys even though
recall play a major role that is like
false negative player is major role or a
false positive play a major role you
know some of the problem statement you
should try to combine both precision and
recall to get the most accurate value so
for that particular case we use a as
beta score now here I'm going to define
a F beta school formula for you so that
you'll be able to understand so now let
us go ahead and understand what exactly
is the F beta score guys in F beta
usually the main aim is to select this
beta value okay now how we should go
ahead and select the beta value I'll
just tell you in a minute
but just understand one thing guys this
exactly if I just consider my beta value
is one okay this basically becomes an f1
score okay
this basically becomes an f1 score how
to select when to select beta is equal
to one
I'm just going to tell you in a while
similarly beta values can vary it can
also be less than one
one it can be 0.5 it can be - you know
if it is 0.5 we basically say this as f
0.5 score if it is 2 means basically say
that F 2 score okay now I hope I don't
know whether you have seen this
particular formula guys but just
understand this is my F beta and
initially we need to select this
particular beta value now consider that
it's my beta values 1 now this formula
basically becomes 2 into precision
multiplied by recall divided by
precision plus recall okay and this is
basically called as a if you don't know
about this guy this is called as a
harmonic mean harmonic mean if I replace
this precision recall by X X - x + y so
this will become 2 X Y divided by x + y
I hope you have been formula with this
particular formula itself this was we've
used in some of the linear algebra in
your school days and in your college
days if you remember - XY / x + y I'm
considering precision and X recall as Y
precision over AR x x + y okay now
understand over here
when should we select beta is equal to 1
now understand guys if you have a
problem statement where wherever through
positive sorry not true positive false
positive and false negative both are
equally important both are having a
greater impact at that time you go and
select beta is equal to 1 okay now in
some of the scenarios suppose you're
false positive is having more impact
than the false negative that is then the
type 2 error okay false positive is the
type 1 error at that time you reduce
your beta value to 0.5 between between 0
to 1
usually people select it as 0.5 at that
time this beta value will get converted
to 0.5 so it will be 1 point 1 plus 0.25
this is nothing but 1 point 2 5
multiplied the precision in to recall
divided by this will basically become
0.25 into precision + record so whenever
your false positive is more important
we reduce this beta value now similarly
if my false negative is high false
negative impact is high right that is
for recall if you remember guys okay if
that is high at that time we
specifically increase my beta value
greater than 1 suppose I consider it as
2 right at that time what will happen
again I'll have to try to apply in this
beta value this particular 2 value this
is nothing will but 5 multiplied by
appreciation into recall divided by 4
multiplied by precision plus recall so
if if false negative and false positive
are both important we consider beta is
equal to 1 if false positive is more
important at that time what we do we
reduce the beta value if false negative
is having an higher impact we increase
the beta value and that is how we select
this F beta value and sometimes we say
it as f1 score sometimes we say it as f2
score sometimes we say say it as f 0.5
score okay considering this particular
values and again guys
based on this false negative also
sometimes your beta value ranges between
1 to 10 okay considering where your
false negative is having a greater
impact when you have false visit
positive is having a greater impact you
basically select a value somewhere
between 0 to 1 and that is why you
specifically use F beta whether you want
to combine both precision recall and try
to showcase a particular problem
statement and try to select the right
kind of metrics this each and every
parameter is very very important guys so
I hope you understood this particular
videos guys in the part 2 will be
discussing about Kohan Kappa ROC curve
AUC score PR curve and there are some
more two more metrics which I am going
to discuss in the next part 2 in the
part 3 we will basically be implementing
a practical problem statement to make
you understand all these particular
things so I hope you like this
particular video I know this is too much
just go revise it you get to know each
and everything so yes this was all about
this particular video I hope you liked
it please do subscribe the channel if
you have not already subscribe in the
next video have a great day ahead thank
you one at all
تصفح المزيد من مقاطع الفيديو ذات الصلة
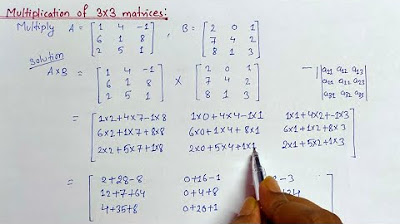
Matrix Multiplication || Multiplication of 3X3 matrices

تعلم replit في 18 دقيقة

We Bought Over 100 Laptops...Can We Profit?

ماذا يحدث لجسدك عند النوم عاريا ؟ ستنصدم ! حذرنا منه النبى ﷺ أشد تحذير !

Earn Money From Mobile | Copy Paste Job 😍| Part Time Job | Online Jobs | Work From Home Jobs 2024

صعدت للڨراند ماستر بدون خسارة 🔥
5.0 / 5 (0 votes)